#cagra
Explore tagged Tumblr posts
Text
少しづつ
札幌中心部は雪が溶けてきては少し湿った雪が降ったりと、いつものように綺麗とは言えない季節がやってきてます。 そうやって来る春は嬉しいものです。早くあったかくならないかな。 雪が溶けるとようやく革底の靴の季節です。 次の雪が積もるまでの楽しみ。
cagraの靴作り教室でもチラホラ靴が出来上がってきました。 まずはFさん、初めての靴作り。 趣味で革製品をたくさん作っていて、念願の靴作りは家での作業もたくさんやってきて頑張りました。 きれいに出来て、サイズも合っていて、春になったらたくさん履いてくださいね。 2足目もゴリゴリと進めています。



こちらも初めての靴作りのYさん。 お仕事で来られない日々もありましたが無事完成。サイズ良し、艶感バッチリ、上手に出来ました。 次の靴は自前の革を準備できるでしょうか?? どんな革を買ってくるのか楽しみにしてます。



前回に続き、今度は自分のサンダルをサクッと作ったMさん。 サクッと。と言っても試作の繰り返しでそれなりに時間はかかってます。 テストテストテスト テストテスト 革はパイソンのゴールド!ピント合ってませんが… 一歩進むごとに運気が上がりそうですね。



Aさんは3足目が完成! お仕事で遅れてしまうことが多く、日数がかかってしまいましたが見事に作り上げました。 紫系のキッド(山羊)で雰囲気の良いチャッカブーツ。 インソックで全体のフィット感も一段と良くなって歩きやすそうに見えますね。



みなさん忙しい中も靴作りの時間を作って楽しんでくれています。 完成時は靴作りの教室を続けていて良かったと思う瞬間の一つ。幸せだなあと思います。
2 notes
·
View notes
Text
Names generated from Italian forenames and Greek islands, excluding the letter "U"
Achakros Aeggia Aenie Aenza Afiako Agianteros Aglimno Agriandra Agrifonisi Aindi Aitheri Alevyti Alori Altanisi Andes Andro Anisi Anisikoni Anissaros Anolza Anteramili Anteras Antia Antikono Antilakidi Antipsada Antisi Antita Apandioea Araso Areganti Armada Armochalli Aroinos Aronisili Arono Arsaros Askoni Asome Asorigelas Astrina Atallo Atecchaki Atroi Avathodos Avelino Avenza Avicia Avryoni...
Bagine Bagolo Barakolos Baravonto Berio Bisilia Bistallos Biton Boealos Boemisto Bolli Bolza Briganto Cafon Cagos Cagra Calio Callognos Calos Carimo Carra Casonysi Ceglia Cellia Cellos Cenos Cenza Cerisymnos Chachilos Chiesi Chila Chilios Chionia Chriakio Chrotivia Colia Comegana Comodos Comythia Credeste Creviti Dento Desci Dianissa Diceschisi Disientino Dones Donisi Drakinos Dravra Elenza Eloggia Ercollos Eseros Esetamos Faegavgos Faleiriza Faros Flegano Flonikale Folagiria Folfeta Folfettoni Fonia Fontza Forga Forna Freloni Galerano Gantonisti Ganza Gavala Gavgona Gavros Gelaxi Gelonis Genti Gentithra Ginistra Giochiza Giochoinos Giono Glios Graplolza Greno Griana Grisi Grothio Gyaiona Gyarenza Gylio Halasa Halia Halydos Hasora Herissos Hragos Hrakara Hteremici Hydranis Ikefolano Ikros Ikyro Imara Imilvana Imoliarda Itakittani Kakini Kakisto Kaleonysi Kalonisi Kalta Kammoni Kandi Kandros Kanos Kanti Karienisia Karkoneira Karno Karteria Kasklo Kastesena Kateros Kavana Kavicia Kavonis Kefaenza Kefaretsos Kefatipsa Kelino Kellegalki Kelos Kerace Kerison Kidica Klidia Klina Kolia Kolianto Konisi Kontolos Konzari Koplamos Korigna Krognoi Kyntos Kyrno Kyros Kythala Kythodos Kythos Kythra Kyttakyra Labrimadia Laiogia Landi Lavigno Lavrovi Laximiloni Lazandio Leccin Legamoli Legia Legno Lendi Lento Lenza Lertece Leses Letalia Letsos Lifonisi Liomo Madaso Mades Madesi Maios Makino Makioinisi Maklitros Makoniace Makra Manti Manysa Marakra Marapla Maravenis Marina Maros Marsa Maskaros Massalki Mastalos Mateloni Matersoi Mates Matharia Mathasses Matimnos Mavdos Mavelia Megalades Megampra Meglia Megna Megno Mescampoli Mezia Mikyros Minies Ministento Modes Modos Molida Monavas Monisia Moros Motikros Mykonisi Mythri Mythrinoa Nalina Nerga Nesta Omada Omone Omytha Oterra Otischia Oxeissos Palaza Pales Palio Palos Paltandos Pandronali Papla Paredeses Paterifon Paterra Pathakra Patikida Patine Paxima Paximorisi Paxos Pergoli Peria Perigno Perinaxos Peropeza Peros Pescatano Pescin Petalevyti Petso Pettoinisa Pides Piesca Pissaresa Pitossa Pomonisos Ponespes Ponianissa Ponisto Ponos Porago Porifona Pothrakiso Potithodi Pozzo Prafoni Pragri Prakorpia Pranisi Prantia Proko Pronisi Psada Psalos Psaminos Psaros Psarteri Psasi Pserasa Psiaeiros Psikati Psylimo Psyrno Psyros Ragontitra Rakines Redesani Redos Regainos Regavatia Reggia Reisidia Relara Relencegno Rezianza Rhachia Rhino Romezia Rovisi Sacas Sachio Sadelli Sades Salkis Salkona Sanissani Sapla Sathiloro Scara Scatherra Sceggyaio Scela Schalafra Schinisi Schisi Sidilia Sikos Skada Skakamo Skala Skalati Skani Skarissano Sklefoklos Skleggia Sklia Skyntosa Skytherio Skythnos Sondrona Speti Sphaliesi Spidio Sponzia Stessa Stornanisi Strano Strephanos Stros Symanos Syrathonto Syria Syromegio Sytis Telos Tentzo Thace Tharana Thatera Therona Theros Theto Thnos Thonimioea Thria Thydra Tikopomfon Tipse Tretecci Treteccia Troggisi Tronikos Tsona Valatika Valydole Vandios Vandra Vandri Varonissa Varos Vatenzak Vathacteno Velloniste Velos Venos Venza Vicallia Vicatisos Vides Vitenos Vithos Vitola Vitos Vitta Vrastosa Vronisilos Vryoni Zakrosaros
0 notes
Photo
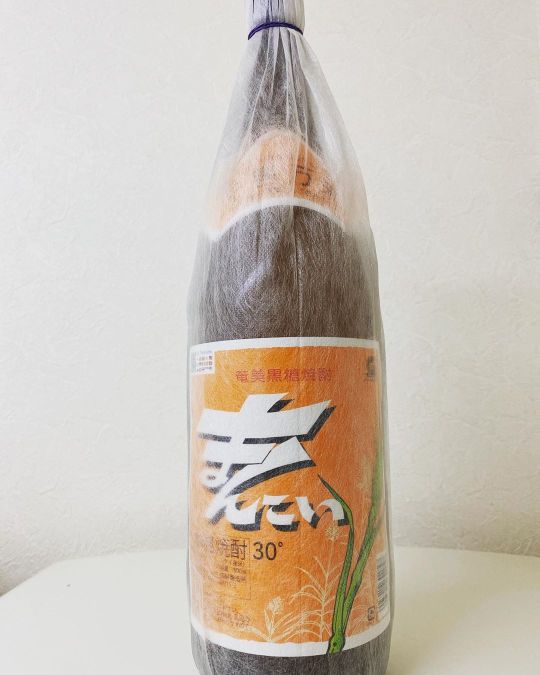
cagraさんから周年祝いいただきました!ありがとうございます。 靴修理店で働きながら靴作り教室に通ってた時からお世話になってます。 独立の相談を初めてしたのもcagraさんだった事を思い出しました。 北海道でビスポークシューズをお考えの方は迷わずcagraさんにGO!ですよ。 自分で靴を作ってみたい人は靴作り教室もあるので問い合わせてみて下さい (Shoe Repair Ânon(アノン)) https://www.instagram.com/p/CAy55WYJYki/?igshid=1fs1jl9mat8e2
5 notes
·
View notes
Text
Esta vez no es tan mala como las anteriores, pero estoy cansada. Y aunque ho ha sido tan mal como otras veces... No sería mejor renunciar a todo esto?
Siento que el corazón pesa más que antes, que las traiciones duelen menos y los amigos se van alejando. Pero ahora eso es tolerable.
Más bien es cansancio y dolor por mi, por no aceptarme y quererme. Por seguir con lazos a mi familia, cuando es de donde siempre salgo herida, y aún así no es tan malo...
Sólo que con los años se vuelve mas pesado llevar esta cagra. Es complicado...
0 notes
Text
YOSHITOMO NARA 北海道写真展
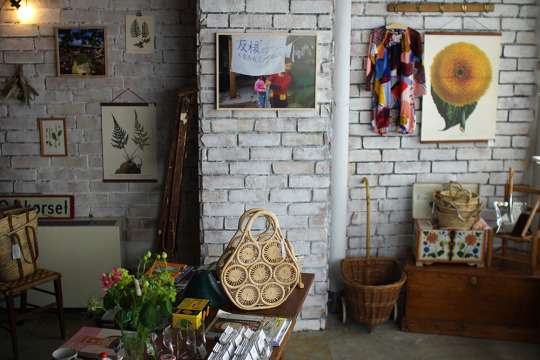
本当に久しぶりのブログ更新となります、当店は期間中 通常通り 火・水曜休みで営業致します。コロナ禍遠出もままならないですが、無理のない範囲でお立ち寄りお待ちしております。
https://atyhs.net
奈良美智さんによる、北海道初の写真展を、 札幌、小樽の小さなお店全13店舗で同時開催いたします。
それぞれのお店に似合う写真を、奈良美智さんがセレクト。 全会場違う作品が展示されます。
会期2021年4月29日(��・祝)〜5月9日(日)
会場Ach so ne 石田珈琲店 cagra カスタネット Cafuné 喫茶とギャラリー なみなみ
サロン チロル 台湾料理ごとう 庭ビル vivre sa vie+mi-yyu
フラワーショップ四季&やかん by みちみち種や 北欧雑貨piccolina MACRO
入場料無料(飲食店の場合は1オーダー必須)
主催YOSHITOMO NARA 北海道写真展 実行委員会
・・・・・・・・・・・・・・・・・・・・・・・・・・・・・
見えない敵と戦った2020年。 今、大切にすべきことを守りながら、また、これからの未来が明るく楽しみなものであるように。一日、一日が大切な日常になってほしいと思い、2021年、札幌と小樽の小さなお店13店舗で、現代美術作家の奈良美智さんの写真展『Though no one may notice, the world knows all that you have seen.』を開催します。
「誰も気が付かないかもしれないけど、君がずっと見てきたことを、ちゃんと知っているよ。」
写真には、その人が何を感じてシャッターを切ったのかが鏡のように映るものです。奈良さんの写真からは優しさ、温かさ、その街、その時の瞬間が伝わってきます。これまで、奈良さんの写真が北海道で紹介されることはなかったのですが、その魅力に惚れ込んだ私たちは、たくさんの方にも観てもらえたらと、写真展を企画しました。
札幌と小樽にある雑貨店、喫茶店、靴工房、美容室、お花屋さん。毎日に彩りを添えてくれるお店に、奈良さんの写真が加わるとどんな変化が起きるのか、それも楽しみの1つです。そして、奈良さんの写真が待ってるのならと、気になっていたあのお店に行ってみようと勇気が湧いてくるかもしれません。
新しい町を散歩する気持ちで、ちょっとワクワク。奈良さんがファインダー越しに見た世界を、一緒にのぞいてみましょう。
皆さまに会える日を楽しみにしています。
YOSHITOMO NARA 北海道写真展 実行委員会
0 notes
Text
Best PMS Products for Wealth Creation
Equities are the one of the best ways to create wealth over longer periods of time. And, Portfolio Management Services are one of the most effective ways to invest in equities.
In the last decade (2010-2020 ) equity market delivered very average returns less than 5 % CAGRas SENSEX went up from 20000 to 40000, and the decade prior to that ( 2000-2010 ), was one of the best decades for equities as SENSEX went up from 3000 level to around 21000 level. If the pattern is to be extrapolated the decade next 2020-2030 could be to be another stellar decade for wealth creation. There are many fundamental reasons for that. Let’s understand them.
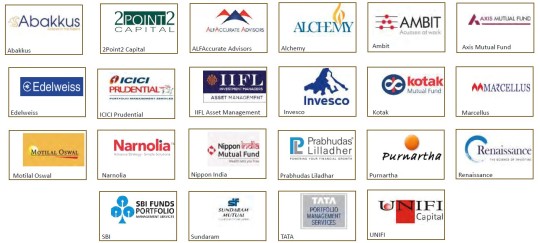
With historic fall in interest rates, cost of capital has gone down significantly. This presents a good business case which many entrepreneurs have always chased in India over years. Also, fall in interest rates, from highs of 9% to almost 5% currently, makes the case for valuations to be seen differently, and hence some high valued companies could command even higher valuations as they would be seen for the potential to generate higher return on capital employed over next decade.
Indian Economy as measured from Gross Domestic Product ( GDP ) has grown from US $ 1.6 tn in 2010 to US $ 2.8 tn in 2020. An absolute performance of 75%. Significantly higher than performance of equity market as Sensex growth was only 50% during last 10 years. This has led to a multi-year GAP between equity market cap and GDP. Currently market cap - to - GDP stands at ~ 60 %. This ratio has fallen swiftly from ~80% as in FY 19 to ~60% in FY 20. It is today, much below long - term average of 75% and closer to levels last seen during FY 09. The ratio has been quite stable over FY15-19 in the 70-80% band. The lowest in the last two decades has been ~45% seen in FY04. The ratio hit a peak of 149% in December 2007 during the 2003-08 bull run. This GAP in Market Cap to GDP ought to be filled and Market Cap to GDP could surpass 100% mark over next decade.
India is an agrarian economy with nearly 50% of Indians livelihood dependent on agriculture and allied sectors. This year has seen a good Rabi crop and the India meteorological department (IMD) announced that it expects monsoon rainfall to be normal this year. Rollout of long pending Agri reforms like scraping of essential commodities act, allowing farms to sell their produce anywhere in the country etc shall pave a way for corporatization of agriculture sector and should lead to growth of this sector in medium to long term.
FII holdings today stands at the lowest level since 2013, at ~20%, govt holding is also at a record low of at ~ 6.6%, DII holding is at 14%, and retail holding is also at 14%. But, at the same time, Indian promotors have increased their holding and are on the buying Spree where they see their franchises under-valued.
China which is largest manufacturing hub to many multinational companies is facing distress wave. And, most of these companies are looking for another alternative, today. CII & Indian Govt. has made representation to around 1500 global companies for moving production to India. Besides, last 10 years have paved way too many policy moves as well as reforms at fiscal, monetary and tax levels. Current scenario is becoming highly conducive for India to attract potential FDI over next decade.
Fall in global crude oil prices is a huge positive for India. Remember 85% of oil is what we import, and every 1 dollar fall in its price, leads to 1 bn dollars of saving on our import bill. This has cascading effect on lower inflation, lower current account deficit, and accommodative monetary stance.
So , investing in Equity is going to see some interesting times ahead and PMS managed by experienced fund managers are going to be one of the best ways to WEALTH CREATION in the coming decade.
Best 5 PMSesbased on the recent past performance (Year 2018 & 2019 ) are
1) Marcellus Consistent Compounders,
2) Stallion Asset Core Fund,
3) IIFL Multi Cap PMS,
4) Ambit Coffee CAN,
5) ASK India Entrepreneurial Portfolio.
0 notes
Text
Ugur Mumcu Boulevard, Girne and road upgrading continues
Ugur Mumcu Boulevard, Girne and road upgrading continues
Work has been initiated in the east of Girne to move road traffic smoothly and safely.
Girne Traffic Transportation Master Plan prepared by the Municipality of Girne for the reduction of traffic intensity on Ugur Mumcu Boulevard called for 4 lanes to be constructed and the work is continuing. A roundabout has been constructed in front of Cagra Ltd in Karakum for the safe and fluid flow of local…
View On WordPress
0 notes
Video
instagram
#tirulipa #TiktokRondonia https://www.instagram.com/p/CAgRA-bHuav/?igshid=1dfzbhshbrqut
0 notes
Text
カグラヅキ
もう11月でしたね。
お客様の靴です、ビスポーク���
旧イルチアの革です。(イタリアのイルチアというタンナーですが、何年か前に買収されて革が結構変わってしまったように思います。界隈では旧とニューでわけて呼ばれていたりします)
スワッチで見た時に、マットな茶系ですが好みが分かれそうな色なので、なかなか靴になりませんでした。
ただ、革質ものすごく良さそうに見えるのですよね。


そこを見抜いたのかNさま。
初めてこの革での製作となりました。
普段、仮靴の時には本番と同じ革の本番には使えない部分で作ることが多いのですが、今回もそうしました。
仮合わせ時にちょっとした提案をしようと思い、片足だけアッパーの色を抜いてみました。いわゆる脱色。
自分の中では脱色���ても良さそうな革とダメな革は何となく分けているのですが、この革はいけるやつです。もちろん端切れで試します。
革は場所によって繊維の違いがあるので、同じやり方で脱色しても同じ結果にはなりません。
片足の中でも色がなかなか落ちないところがあれば落ち過ぎてしまうところもある。ほぼ一発勝負で緊張します。
さあ仮合わせの日。
お客様は脱色した方をものすごく気に入ってくださって、本番もそうして欲しいとのご依頼。
しかもある一部分を指して、この感じが好みだと、、、
自分で勝手にハードルを上げてしまいました、、、
・
・
・





Nさま、この度はどうもありがとうございました。
2 notes
·
View notes
Link
Cloud Eco recrute 100 collaborateurs en 2018 #ChrisTec #France #Lille #Marseille L’opérateur Cloud Eco affirme ses ambitions et annonce sa campagne de recrutement 2018 qui marquera une étape-clé dans sa stratégie de croissance. C’est dans ce contexte que Cloud Eco va investir massivement pour renforcer ses équipes dans toute la France au sein de ses agences régionales et particulièrement dans la région nord (Lille et Rouen) et sud (Aix-en-Provence, Marseille et Montpellier). Cloud Eco est un opérateur global de téléphonie fixe et mobile et un fournisseur d’accès Internet. Ses équipes garantissent le déploiement de solutions performantes auprès des PME et TPE et accompagnent au quotidien de nombreuses structures dans la mise en œuvre d’infrastructures télécom adaptées à leurs attentes. Au regard de ces éléments, Cloud Eco souhaite muscler ses équipes commerciales en recrutant cent nouveaux candidats. Plus que des candidats diplômés ou expérimentés, Cloud Eco recherche des collaborateurs engagés, passionnés et désireux de s’investir pleinement dans la société. Une fois recrutés, les collaborateurs bénéficieront de nombreuses formations et d’un soutien actif de leurs managers. Rejoindre Cloud Eco est l’assurance d’évoluer dans un groupe où la méritocratie est une valeur centrale. Ainsi, le groupe permet aux collaborateurs dynamiques et motivés d’accéder rapidement à des postes de management. Un autre élément à prendre en compte tient à la possibilité d’évoluer dans l’ensemble des agences grâce à une politique de mobilité, mais également de manière transversale dans les différents départements du groupe. Cloud Eco bénéficie donc de nombreux fondamentaux qui le positionnent comme l’un des opérateurs les plus attractifs du marché. Elif Cagras, Chargé de recrutement et formation chez Cloud Eco « Notre marque employeur bénéficie d’un réel potentiel d’attractivité. Nous allons investir de très nombreuses ressources pour renforcer nos équipes commerciales régionales qui accompagnent au quotidien nos clients dans leurs projets Telecom et réseaux. Nous recherchons des personnalités enthousiastes et désireuses de commercialiser des services à très forte valeur ajoutée dédiés aux TPE et PME. Cloud Eco permet donc de vivre une expérience professionnelle unique où les notions d’engagement, de dépassement de soi et de passion sont des points essentiels pour évoluer dans l’entreprise. » Catégorie actualité: Carrière emploi Image actualité AMP: #France #Lille #Marseille
0 notes
Photo
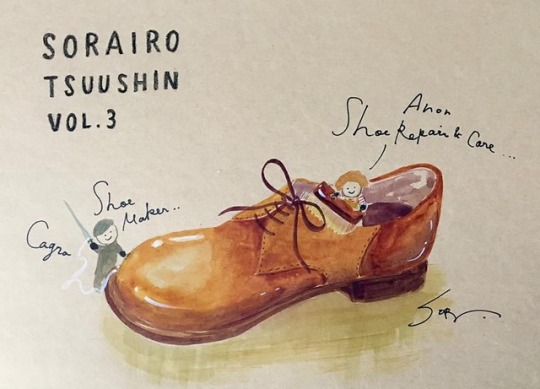
先日取材していただいたNHKの番組今日放送されたみたいです。(見逃した) cagraさんと僕のイラスト(天パ感\(^o^)/)そらさんありがとうございました! (Shoe Repair Ânon(アノン))
3 notes
·
View notes
Photo
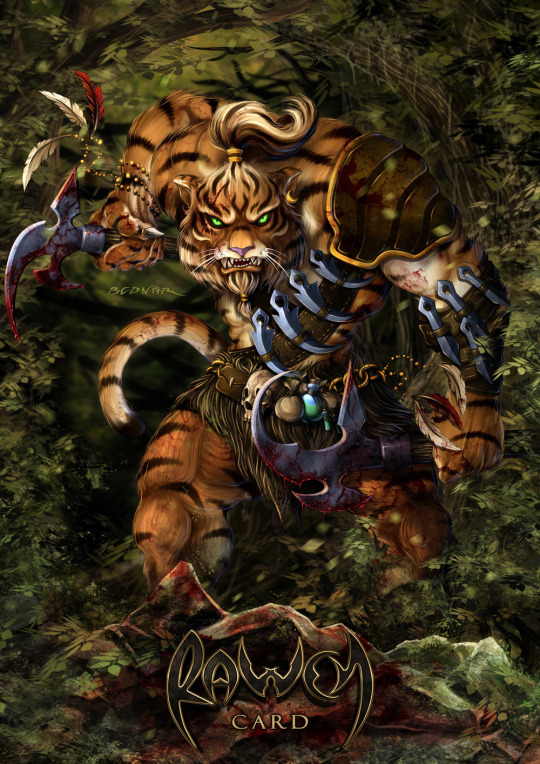
Cagra, the Demon Claw by ~Symerinart
---x---
More: | Animals | Random |
16 notes
·
View notes
Text
HP
そうそう。 ホームページのトップ画面からinstagramとショップページに行けるようになりました。 もう少し更新せねば、、、とは思ってます。
cagra
今年は暑くていい夏って感じの札幌です。今のところ去年よりは過ごしやすい。 各地で水害もあって浮かれてばかりではいられませんが、素早い支援が届きますように。
2 notes
·
View notes
Text
DEEP
更新を少し開けてしまうと、あれも書いてこれも書いて、あ、あれも書かないとなとか、それで、結局書かなくなってしまうことがあります。 誰が見てるかも分からないし、自分にとってはどうでもいいこと書いても良い場所なのに 笑 少し病んでるかのような書き出しになりましたが通常運転です。 今日も素敵な靴のお話になったら良いなと思い書き出します。
なんと、全く同じデザインで2足目のご注文でした。 私が自分のために揃えるとしたら、革の靴と鞄に関しての理想は気に入ったデザインを2色ずつ持つこと。
靴に関しては欲しいデザインが大体決まっていますが、靴屋をやっていてもなかなか願いは叶いません。なぜでしょう??
鞄に関してもデザインがもちろん大切ですが、大きさは第一条件。小さなものから大きなものまでで内容物と行動に合わせて2色ずつ。
私に関しては、まだまだ物欲は収まらないようです。
さて、Yさまの2足目。なんと!全く同じデザインで2足目のご注文でした。






アッパーはドイツのカーフ。とても深い緑のきれいな革です。 底材は全てイギリスのオークバークを使用しました。
素敵です。 どうもありがとうございました。
2 notes
·
View notes
Text
教室
毎年の花粉とはちょっと違うここ最近。 目が痒すぎて、瞼が腫れてます。その割に鼻水が出ないしなんだか変な感じ。 花粉じゃないのかな? この春から教室も新たなメンバーを迎えてます。来月も新たに1名来ていただく予定。まあ、春に限らず募集していますが、自分は春というと何か始めたくなります。最近始めたのは週一銭湯通い。 靴作りは先が長いのですが、まずは楽しみながら1足作り上げて欲しいですね。
最近、教室で完成した2足。
まずはBさん。 教室のメンバーと共同購入したホーウィンのクロムエクセルで作った2足目。 底付けは2回目の作業も多いので、前回を思い出していただきながらコバやヒールの削りや磨きをしっかり目にやっていただきました。 コツが少し掴めたようで、きれいに出来たように思います。 完成おめでとうございます。


Sさん5足目は少し変則のTストラップ。 とてもきれいなカーフで柔らかい足あたりでしょう。 本番の前に仮靴も作りましたが、完成後履いてみると少し大きかったよう。 残念ながらこういうこともありますが、そこは気を取り直してサイズ調整を学ぶことに。 こちらからはいくつかの方法とか考え方を説明し、Sさん自らのアイデアを組み合わせて素晴らしい調整となりました。 履きなれてみてからもまだ調整があるかもしれませんが、こういった発想と実際に手を動かすことこそが、靴作りだけではなく他の多くのことに活かせる力となると思っていて、自分が教室をやっている意味の一つでもあります。 Sさんも完成おめでとうございます。


2 notes
·
View notes