#bayesian theorem
Explore tagged Tumblr posts
Text
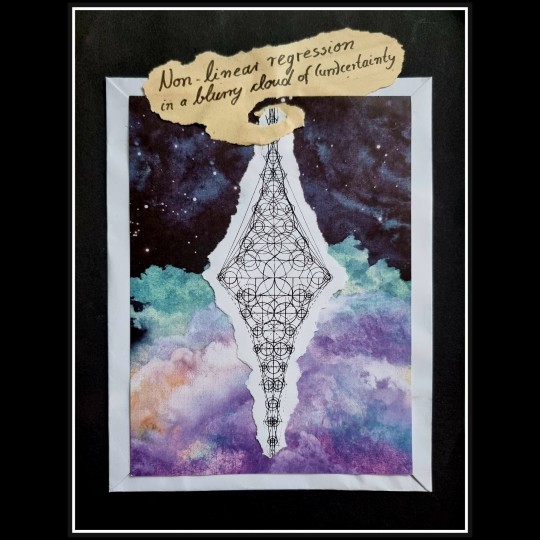
Title: "Non-linear regression in a blurry cloud of (un-) certainty"
Date: 2023/06/12 - Size: DIN A4
Collage made with torn pieces of paper, printed background paper (top is rather dark night sky, bottom is mererly clouds in pastel-colors)
I resized and printed the non-linear regression visualisation/illustration and put it on top of the watercolour background paper.
I included a scrap piece of paper with the title of the picture and have torn it with a spiral-shaped jag at the bottom, which I bent around the top part of the non-linear regression illustration.
#art#math art#mathy art#collage#non linear regression#regression#stats#statistics#bayesian stuff#artsy#my art#math#mathematics#STEM art#STEMmy art#colors and shapes#uncertainty#bayesian theorem#bayesian inference#ml#machine learning#probability
37 notes
·
View notes
Text
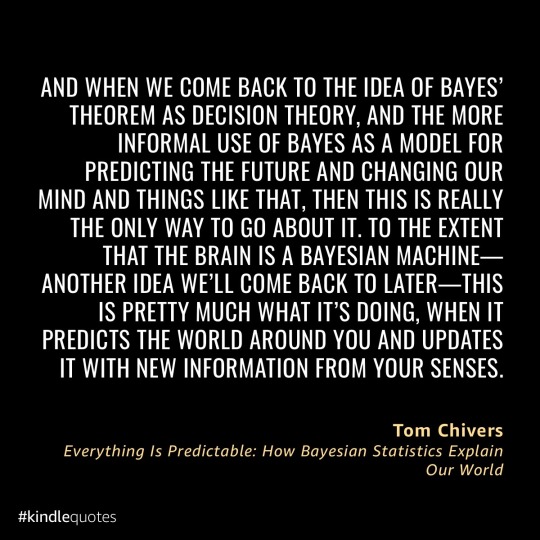
#quotes#kindle quotes#psychology#bayesian#statistics#prediction#bayes’ theorem#Tom Chivers#the brain is a Bayesian machine
2 notes
·
View notes
Text

i used to be a rationalist. roko's basilisk... bayesian theorems... ai research at yudkowsky's. you would have not liked me back then
6K notes
·
View notes
Text
Bayesian Active Exploration: A New Frontier in Artificial Intelligence
The field of artificial intelligence has seen tremendous growth and advancements in recent years, with various techniques and paradigms emerging to tackle complex problems in the field of machine learning, computer vision, and natural language processing. Two of these concepts that have attracted a lot of attention are active inference and Bayesian mechanics. Although both techniques have been researched separately, their synergy has the potential to revolutionize AI by creating more efficient, accurate, and effective systems.
Traditional machine learning algorithms rely on a passive approach, where the system receives data and updates its parameters without actively influencing the data collection process. However, this approach can have limitations, especially in complex and dynamic environments. Active interference, on the other hand, allows AI systems to take an active role in selecting the most informative data points or actions to collect more relevant information. In this way, active inference allows systems to adapt to changing environments, reducing the need for labeled data and improving the efficiency of learning and decision-making.
One of the first milestones in active inference was the development of the "query by committee" algorithm by Freund et al. in 1997. This algorithm used a committee of models to determine the most meaningful data points to capture, laying the foundation for future active learning techniques. Another important milestone was the introduction of "uncertainty sampling" by Lewis and Gale in 1994, which selected data points with the highest uncertainty or ambiguity to capture more information.
Bayesian mechanics, on the other hand, provides a probabilistic framework for reasoning and decision-making under uncertainty. By modeling complex systems using probability distributions, Bayesian mechanics enables AI systems to quantify uncertainty and ambiguity, thereby making more informed decisions when faced with incomplete or noisy data. Bayesian inference, the process of updating the prior distribution using new data, is a powerful tool for learning and decision-making.
One of the first milestones in Bayesian mechanics was the development of Bayes' theorem by Thomas Bayes in 1763. This theorem provided a mathematical framework for updating the probability of a hypothesis based on new evidence. Another important milestone was the introduction of Bayesian networks by Pearl in 1988, which provided a structured approach to modeling complex systems using probability distributions.
While active inference and Bayesian mechanics each have their strengths, combining them has the potential to create a new generation of AI systems that can actively collect informative data and update their probabilistic models to make more informed decisions. The combination of active inference and Bayesian mechanics has numerous applications in AI, including robotics, computer vision, and natural language processing. In robotics, for example, active inference can be used to actively explore the environment, collect more informative data, and improve navigation and decision-making. In computer vision, active inference can be used to actively select the most informative images or viewpoints, improving object recognition or scene understanding.
Timeline:
1763: Bayes' theorem
1988: Bayesian networks
1994: Uncertainty Sampling
1997: Query by Committee algorithm
2017: Deep Bayesian Active Learning
2019: Bayesian Active Exploration
2020: Active Bayesian Inference for Deep Learning
2020: Bayesian Active Learning for Computer Vision
The synergy of active inference and Bayesian mechanics is expected to play a crucial role in shaping the next generation of AI systems. Some possible future developments in this area include:
- Combining active inference and Bayesian mechanics with other AI techniques, such as reinforcement learning and transfer learning, to create more powerful and flexible AI systems.
- Applying the synergy of active inference and Bayesian mechanics to new areas, such as healthcare, finance, and education, to improve decision-making and outcomes.
- Developing new algorithms and techniques that integrate active inference and Bayesian mechanics, such as Bayesian active learning for deep learning and Bayesian active exploration for robotics.
Dr. Sanjeev Namjosh: The Hidden Math Behind All Living Systems - On Active Inference, the Free Energy Principle, and Bayesian Mechanics (Machine Learning Street Talk, October 2024)
youtube
Saturday, October 26, 2024
#artificial intelligence#active learning#bayesian mechanics#machine learning#deep learning#robotics#computer vision#natural language processing#uncertainty quantification#decision making#probabilistic modeling#bayesian inference#active interference#ai research#intelligent systems#interview#ai assisted writing#machine art#Youtube
4 notes
·
View notes
Text

Dr. David Harold Blackwell (April 24, 1919 – July 8, 2010) was a statistician and mathematician who made significant contributions to game theory, probability theory, information theory, and Bayesian statistics. He is one of the eponyms of the Rao–Blackwell theorem. He was the first African American inducted into the National Academy of Sciences, the first African American tenured faculty member at UC Berkeley, and the seventh African American to receive a Ph.D. in Mathematics.
He was a pioneer in textbook writing. He wrote one of the first Bayesian textbooks, his 1969 Basic Statistics. By the time he retired, he had published over 90 books and papers on dynamic programming, game theory, and mathematical statistics.
He entered the University of Illinois at Urbana-Champaign with the intent to study elementary school mathematics and become a teacher. He earned his BS, MS, and Ph.D. in Mathematics all by the age of 22. He was a member of Alpha Phi Alpha Fraternity.
He took a position at UC Berkeley as a visiting professor in 1954 and was hired as a full professor in the newly created Statistics Department in 1955, becoming the Statistics department chair in 1956. He spent the rest of his career at UC Berkeley, retiring in 1988. #africanhistory365 #africanexcellence #alphaphialpha
7 notes
·
View notes
Text
The Data Scientist's Toolkit: 20 Essential Statistical Approaches - A Deep Dive
Data science is more than just wielding powerful machine learning algorithms. It's fundamentally built on a strong foundation of statistical principles. To truly extract meaningful insights, build robust models, and make impactful decisions, a data scientist must possess a comprehensive understanding of statistical approaches. Let's delve deeper into 20 crucial statistical concepts that form the bedrock of a successful data science career.
1. Descriptive Statistics: Unveiling the Story Within the Data
Descriptive statistics are the first step in any data analysis. They provide a concise summary of your data, allowing you to understand its central tendencies and variability. Key measures include:
Mean: The average value, representing the center of the data.
Median: The middle value, less sensitive to outliers than the mean.
Mode: The most frequent value, useful for categorical data.
Variance: Measures the spread of data around the mean.
Standard Deviation: The square root of variance, providing a more interpretable measure of spread.
2. Probability Distributions: Modeling the World's Randomness
Probability distributions describe the likelihood of different outcomes. Understanding them is crucial for modeling real-world phenomena:
Normal Distribution: The bell-shaped curve, representing many natural phenomena.
Binomial Distribution: Models the probability of successes in a fixed number of trials.
Poisson Distribution: Models the probability of events occurring in a fixed interval of time or space.
Exponential Distribution: Models the time between events in a Poisson process. 1
3. Hypothesis Testing: Drawing Conclusions from Data
Hypothesis testing allows us to make statistically sound inferences about populations based on sample data. Common tests include:
T-tests: Compare the means of two groups.
Chi-square tests: Examine relationships between categorical variables.
ANOVA (Analysis of Variance): Compare the means of multiple groups.
4. Confidence Intervals: Estimating the Unknown
Confidence intervals provide a range within which a population parameter (e.g., the mean) is likely to fall, with a certain level of confidence.
5. Correlation Analysis: Measuring Relationships
Correlation analysis quantifies the strength and direction of the linear relationship between two variables.
6. Regression Analysis: Predicting the Future
Regression analysis builds models to predict a dependent variable based on one or more independent variables:
Linear Regression: Models linear relationships.
Polynomial Regression: Models curved relationships.
Logistic Regression: Models binary outcomes.
7. Analysis of Variance (ANOVA): Comparing Group Differences
ANOVA extends t-tests to compare the means of more than two groups.
8. Time Series Analysis: Unraveling Temporal Patterns
Time series analysis focuses on data collected over time, identifying trends, seasonality, and other patterns:
ARIMA (Autoregressive Integrated Moving Average): Models time series based on past values.
Exponential Smoothing: Forecasts future values based on weighted averages of past values.
9. Bayesian Statistics: Updating Beliefs with Evidence
Bayesian statistics uses Bayes' theorem to update beliefs based on new evidence.
10. Sampling Techniques: Making Inferences from Subsets
Sampling techniques allow us to select representative samples from a population to make inferences about the entire population.
11. Non-Parametric Tests: Dealing with Non-Normal Data
Non-parametric tests are used when data does not meet the assumptions of parametric tests:
Mann-Whitney U test: Compares two independent groups.
Wilcoxon signed-rank test: Compares two related groups.
12. Principal Component Analysis (PCA): Reducing Dimensionality
PCA reduces the dimensionality of data by identifying the principal components that explain the most variance.
13. Cluster Analysis: Finding Hidden Groups
Cluster analysis groups similar data points together based on their characteristics:
K-means clustering: Partitions data into k clusters.
Hierarchical clustering: Builds a hierarchy of clusters.
14. Outlier Detection: Identifying Anomalies
Outlier detection identifies data points that deviate significantly from the rest of the data.
15. Survival Analysis: Modeling Time-to-Event Data
Survival analysis analyzes time-to-event data, such as time until failure or time until recovery.
16. Resampling Techniques: Estimating Model Performance
Resampling techniques, like bootstrapping and cross-validation, estimate the performance of a model.
17. Statistical Power: Detecting Real Effects
Statistical power determines the probability of detecting a statistically significant effect when it exists.
18. A/B Testing: Comparing Two Options
A/B testing compares two versions of a product or feature to determine which performs better.
19. Statistical Modeling: Representing Reality
Statistical modeling involves creating mathematical representations of real-world phenomena.
20. Experimental Design: Planning Effective Studies
Experimental design focuses on planning and conducting experiments to collect data and test hypotheses.
The Indispensable Role of Statistics in Data Science:
These statistical approaches are not mere theoretical concepts; they are the practical tools that empower data scientists to:
Extract meaningful insights from raw data.
Build accurate and reliable predictive models.
Make informed, data-driven decisions that drive business value.
Communicate complex findings clearly and effectively to stakeholders.
Xaltius Academy's Data Science and AI Program: Building a Strong Statistical Foundation:
Xaltius Academy's Data Science and AI program recognizes the paramount importance of a solid statistical foundation. The program provides a comprehensive understanding of these essential statistical approaches, ensuring that you graduate with the practical skills and theoretical knowledge needed to excel in the data science field. The program's hands-on approach will make these difficult concepts easier to understand.
0 notes
Text
Sensor Fusion Market Size, Share & Industry Trends Analysis Report by Algorithms (Kalman Filter, Bayesian Filter, Central Limit Theorem, Convolutional Neural Networks), Technology (MEMS, Non-MEMS), Offering (Hardware, Software), End-Use Application and Region - Global Forecast to 2028
0 notes
Text
I finally figured out what's been missing from all the discussions about this: Bayesian Statistics.
The original question, as worded, is NOT: "which would you be more likely to find at your doorstep?"
It is: "which would you be more SURPRISED to find at your doorstep?"
In considering both options, we are asked to believe, for a moment, that there IS a fairy or a walrus at our door. The possibility of hallucinations or pranks or wordplay nonwithstanding, it is GIVEN in that question that there IS a fairy at your door.
Accepting this, let's look at Bayes' Theorem for a moment:

First, let's look at the question of walruses. We will let condition B be "there is a walrus at my door" and condition A be "walruses are real." Most of us have a high degree of certainty that walruses are real, so we can confidently set our prior at 1. Our unknowns and uncertainties then revolve entirely around the likelihood of seeing a walrus at our front door. This depends on location, tendency towards visual hallucinations, and other factors, which many people have discussed in other posts.
Now, let's look at the question of fairies.
We'll use the same setup: condition B is "there is a fairy at my door" and condition A is "fairies are real." Now the prior becomes more interesting. Many people would set it at zero - though I suspect not all as confidently as we set the probability of walruses to 1 - but not everyone would. After all, it's much harder to prove a negative. As for the likelihood, I think many people agree that given fairies existed, it wouldn't be all that surprising to find one at your door. There are still many factors to consider - the type of fairy, for one - but I think most of us could agree that P(B|A) is greater than 0. As for the marginalization, even the staunchest of fairy disbelievers could allow for mild hallucinations, making P(B) small, but still nonzero.
Now let's look at the posterior. Given that we see a fairy at our doorstep, what are the chances that fairies are real? Stanch P(A) = 0 believers would insist that the chance is still 0, and that what we think we see is a hallucination or prank. However, those who allow for greater uncertainty in their prior are more likely to restructure their worldview to allow for fairies, if they were to observe one at their door. In addition, not everyone experiences frequent hallucinations, and - most critically - the wording of the original poll predisposes many of us to assume that our eyes are giving us the objective truth in this scenario.
Now let's look at the final element of the puzzle: surprise. Which result would be more statistically anomalous to find?
The probability of walruses is well-defined. It is well-understood, using measures that have been handed down for generations. The prior is well-constrained, the likelihood is a function of similarly well-constrained factors. For most of us, the probability of a walrus at our door is significant well beyond 3 sigma. In my own case, I'd put it well past 5 sigma, without question.
For most people, the probability of fairies is less constrained. In this case, when we are presented with a fairy at our door, we must ask ourselves a question: "Am I dreaming, hallucinating, being pranked, or is there truly a fairy at my door?" The first 3 possibilities are not that hard to believe, and are thus unsurprising. However, if the evidence of our eyes is real, then our prior knowledge of fairies was incorrect, and is much closer to one than to 0. In this case, than the likelihood of a real fairy being at our door is less constrained than the likelihood of a real walrus - there is far more variation in fairies than in walruses, after all. Thus, given that fairies are apparently real, the appearance of one at my door is not significant beyond the 3 sigma level, within the allotted uncertainties.
Though I would still publish the paper, as a case study and to update the prior for future calculations by others.
fuck it let's try this again
#statistics#walruses#fairies#described#there's a lot more nuance that you can get into here#I only did fairly rudimentary work with Bayesians#but I was surprised that I hadn't seen anyone bring it up yet#math#mathematics
34K notes
·
View notes
Text
Sensor Fusion Market Trends and Innovations to Watch in 2024 and Beyond

The global sensor fusion market is poised for substantial growth, with its valuation reaching US$ 8.6 billion in 2023 and projected to grow at a CAGR of 4.8% to reach US$ 14.3 billion by 2034. Sensor fusion refers to the process of integrating data from multiple sensors to achieve a more accurate and comprehensive understanding of a system or environment. By synthesizing data from sources such as cameras, LiDAR, radars, GPS, and accelerometers, sensor fusion enhances decision-making capabilities across applications, including automotive, consumer electronics, healthcare, and industrial systems.
Explore our report to uncover in-depth insights - https://www.transparencymarketresearch.com/sensor-fusion-market.html
Key Drivers
Rise in Adoption of ADAS and Autonomous Vehicles: The surge in demand for advanced driver assistance systems (ADAS) and autonomous vehicles is a key driver for the sensor fusion market. By combining data from cameras, radars, and LiDAR sensors, sensor fusion technology improves situational awareness, enabling safer and more efficient driving. Industry collaborations, such as Tesla’s Autopilot, highlight the transformative potential of sensor fusion in automotive applications.
Increased R&D in Consumer Electronics: Sensor fusion enables consumer electronics like smartphones, wearables, and smart home devices to deliver enhanced user experiences. Features such as motion sensing, augmented reality (AR), and interactive gaming are powered by the integration of multiple sensors, driving market demand.
Key Player Strategies
STMicroelectronics, InvenSense, NXP Semiconductors, Infineon Technologies AG, Bosch Sensortec GmbH, Analog Devices, Inc., Renesas Electronics Corporation, Amphenol Corporation, Texas Instruments Incorporated, Qualcomm Technologies, Inc., TE Connectivity, MEMSIC Semiconductor Co., Ltd., Kionix, Inc., Continental AG, and PlusAI, Inc. are the leading players in the global sensor fusion market.
In November 2022, STMicroelectronics introduced the LSM6DSV16X, a 6-axis inertial measurement unit embedding Sensor Fusion Low Power (SFLP) technology and AI capabilities.
In June 2022, Infineon Technologies launched a battery-powered Smart Alarm System, leveraging AI/ML-based sensor fusion for superior accuracy.
These innovations aim to address complex data integration challenges while enhancing performance and efficiency.
Regional Analysis
Asia Pacific emerged as a leading region in the sensor fusion market in 2023, driven by the presence of key automotive manufacturers, rising adoption of ADAS, and advancements in sensor technologies. Increased demand for smartphones, coupled with the integration of AI algorithms and edge computing capabilities, is further fueling growth in the region.
Other significant regions include North America and Europe, where ongoing advancements in autonomous vehicles and consumer electronics are driving market expansion.
Market Segmentation
By Offering: Hardware and Software
By Technology: MEMS and Non-MEMS
By Algorithm: Kalman Filter, Bayesian Filter, Central Limit Theorem, Convolutional Neural Network
By Application: Surveillance Systems, Inertial Navigation Systems, Autonomous Systems, and Others
By End-use Industry: Consumer Electronics, Automotive, Home Automation, Healthcare, Industrial, and Others
Contact:
Transparency Market Research Inc.
CORPORATE HEADQUARTER DOWNTOWN,
1000 N. West Street,
Suite 1200, Wilmington, Delaware 19801 USA
Tel: +1-518-618-1030
USA - Canada Toll Free: 866-552-3453
Website: https://www.transparencymarketresearch.com Email: [email protected]
0 notes
Text
TVF - Performatively creative
Dear Caroline:
This little snippet is from Adam Yedidia, one of the first witnesses at the FTX trial. In just a few brushstrokes, he manages to capture some of your most admirable virtues beyond the selflessness mentioned in your relative’s letters: your hard work, intelligence, kindness, creativity, joyfulness, and fun-loving spirit. I smiled at his oxymoronic mention of your “spare time”—a rarity, I imagine, given the intensity of your commitments. Yet from your blog, I already knew a bit about your LARP talents (like your notes from the Hong Kong game) and a touch of your culinary skills. These gems were tucked away on worldoptimization - life advice posts with tips about vinegars, shrub syrups, and scone recipes. I’m tempted to try those scones myself, if the links are still active.
Mr. Yedidia’s letter concludes with a suggestion that you might channel your empathy and skill with words into future careers in writing and education. I wholeheartedly agree. But I would add that the range of your abilities and interests is so broad that whatever you choose to focus on, I’m certain you’ll excel—not only for your own fulfillment but for the world around you as well.
Thinking about your future and the high likelihood of a positive outcome brough to mind the book I am currently reading: Everything is Predictable, by Tom Chivers. It is a light primer in Bayesianism (the subtitle is 'How Bayes' Remarkable Theorem Explains the World). It would be light reading for you, but I think you would enjoy it - you reviewed one of Chivers's other books in your blog and goodreads before.
May these reflections bring you encouragement as you consider the possibilities awaiting you.
0 notes
Text
Fuck it, full list + review:
First year
Linear Algebra: easy, boring.
Group theory: marginally harder, significantly more interesting.
Real analysis: one of the hardest, but only because you do it right at the start. Really one you only appreciate once it's over.
Probability: I really enjoyed this actually - not too hard if you're ok at analysis.
Statistics: it's like they took probability and took out the interesting parts.
Intro calculus: mostly very very boring, but some neat problems I guess. Also badly named, this means intro to differential equations.
Multivariable calculus: honestly one of the easiest courses I've taken. Slightly more interesting than intro.
Fourier analysis: terrible course, only taught for applied people. Everyone else should be allowed to wait until they've done functional analysis.
Geometry: mostly linear algebra tbh, with some random calculus problems thrown in. Fine, but felt very thrown together.
Dynamics. This was the hardest course I ever took. Wtf was happening. How did I get a first in this. I had no idea what I was meant to do at any point.
Algorithms: can you learn an algorithm and apply it by hand? Then you can pass this course!
Second year:
More linear algebra: Spectral theorem is useful, otherwise by far the easiest second year course.
Ring theory: vital for any pure mathematician. The course was very hard, but mostly because the lecturer was the kind of person to define a subring as "an injective morphism" to second years. Modules are cool tho.
Complex analysis: also very very hard, but mostly because it was badly taught. I see why people like it; I didn't.
Measure theory: the end of real analysis! Nice to finally define an integral, and definitely nicer arguments than other analysis courses. Overall fun if tricky.
Metric spaces: sick. It was taught by Ben Green. Need I say more?
Topology: I am doing a PhD in topology, so very biased. You spend a lot of time doing fairly boring analysis-y stuff, but the payoff is great.
More differential equations: this was fantastically taught and actually very fun, although partly because I was good at it. That said, actually solving the damn things wasn't always that fun compared to the theory.
More probability: Markov chains are cool and also just easier than everyone thinks they're going to be? Ended up being my best second year exam somehow lol, but fairly mid-tier for interest.
More statistics: I only took this because I felt I should know Bayesian stats. I have forgotten Bayesian stats.
Quantum theory: did you know that everything is a Hilbert space? And do you know your trig identities? If yes, you might like this course! More seriously, this is just "intro to Lie algebra representation theory" but they don't tell you that.
Short courses (more group theory, number theory, projective geometry, multivariable analysis): these courses were badly designed so no comment
Third year
Representation theory: sick. Do this. It's just algebra but done better.
Commutative algebra: ill. is how I felt. Don't do this. Well do it's very important in pure maths but make sure you find a good teacher. You probably should do it though I guess.
Galois theory: everyone interested in pure maths should do this, but personally I hated it. I do not care sufficiently about polynomials.
Algebraic number theory: if you care about numbers, this is cool. I found it fairly easy/boring since it wasn't aimed at people who'd done as much algebra as I had, but was nice to see.
Surfaces: a weird sort of intro to classical differential geometry, focusing on smooth surfaces and Riemann surfaces. It was nice and very easy, but the course was very weirdly designed imo. Other unis also seem to have similar courses though, which confuses me, since I feel it makes more sense to just teach general manifolds then Riemannian geometry, and use surfaces as simple examples throughout.
Algebraic curves: massive overlap with surfaces since both needed Riemann surfaces but neither was a prereq for the other. Then also covered all of projective geom, and rushed through the interesting stuff. Having now relearnt it though, it's super cool and I would recommend to everyone pure or not.
Baby AlgTop: basically Ch0+1 of Hatcher, dealing with cell complexes and the fundamental group. Everyone should know what the fundamental group is, but tbh I don't think everyone needs to sit through a course that proves the simplicial approximation theorem.
Functional Analysis: quite easy if you have finally internalised the lessons that undergrad analysis was trying to teach you, very hard if not. Basically did everything you've already seen more generally, imo should be compulsory.
More Functional Analysis: this time it's topology! Seriously though. Do it if you like topology, otherwise just trust people.
More quantum theory: this time it was "intro to Lie group representation theory". Then it became perturbation theory and I stopped going.
Master's year:
All of these reviews will be useless because the masters I did was weird.
Homological algebra: why was this taught before category theory??? Useful tool, but a hard course. Especially before category theory.
Category theory: should be compulsory for pure mathematicians. Also should be an undergrad course, since all my other masters courses assumed you knew basic category theory. (And so did some 3rd year algebra courses).
Algebraic geometry: I dropped it after 3 lectures bc the lecturer was bad. But if you have a good lecturer, maybe it might not be? Im unconvinced.
Proper Algebraic topology: the classic course covering Ch2+3 of Hatcher. If you like pictures and sign errors you should do it. If not, you probably still should.
Manifolds: this could also be an undergrad course tbh. Do you love vector bundles? You should love vector bundles. I love vector bundles. De Rham cohomology is underwhelming though, sorry @lipshits-continuous.
Lie groups: I think there is no good way to teach Lie groups to geometers. At least to me. I have absolutely no idea how I got 70% in this exam I did not deserve it.
Riemannian geometry: my best exam ever I think, somehow. It's kinda just analysis, except for geometers so there's much more handwaving and fewer δs. It's pretty boring at points, but the interplay between topology and curvature is fascinating imo.
Low-dimensional topology: this is now my PhD, but also the course was atrocious. Do with that what you will.
Uhh that was a good use of time while I waited for my rice to cook
Math enthusiasts of tumblr. What math subjects have you studied and which ones were your favorite? Which ones were your least favorite? Which ones were the hardest?
177 notes
·
View notes
Text
Tenth workshop:
OBSERVATION AND MEASUREMENT
Venue: Institute of Philosophy, Research Center for the Humanities, Budapest, 1097 Tóth Kálmán u. 4, Floor 7, Seminar room (B.7.16)
Date: October 17, 2024
Organizer: Philosophy of Physics Research Group, Institute of Philosophy
Contact: Gábor Hofer-Szabó and Péter Vecsernyés
The language of the workshop is Hungarian.
The slides of the talks can be found here.
Program:
10.20: Welcome and introduction
10.30: József Zsolt Bernád: De Finetti's representation theorem in both classical and quantum worlds
Throughout this talk, I will adopt a committed Bayesian position regarding the interpretation of probability. First, the concept of exchangeability with some examples is discussed. This is followed by the classical de Finetti representation theorem and its consequences. As we establish justification for using Bayesian methods, it becomes an interesting question of how to carry over the results to the quantum world. In the last part of the talk, a de Finetti theorem for quantum states and operations is presented. Then, I will discuss the operational meaning of these results. Only key elements of the proofs will be given, and the focus lies on the implications.
11.30: Coffee
11.45: András Pályi: Qubit measures qubit: A minimal model for qubit readout
On the most elementary level, measurement in quantum theory is formulated as a projective measurement. For example, when we theoretically describe the measurement of a qubit, then the probabilities of the 0 and 1 outcomes, as well as the corresponding post-measurement states, are calculated using two orthogonal projectors. If, however, we perform a qubit readout experiment, then the physical signal we measure to infer the binary outcome is usually more informative (`softer’) than a single bit: e.g., we count the number of photons impinging on a photodetector, or measure the current through a conductor, etc. In my talk, I will introduce a minimal model of qubit readout, which produces such a soft signal. In our model — following an often-used scheme in the generalised formulation of quantum measurements —, the qubit interacts with a coherent quantum system, the `meter’. Many subsequent projective measurements are performed on the meter, and the outcomes of those generate the soft signal from which the single binary outcome can be deduced. Our model is minimal in the sense that the meter is another qubit. We apply this model to understand sources of readout error of single-electron qubits in semiconductors.
12.45: Lunch
14.00: Győző Egri: Records and forces
I review the mechanism of how objective reality emerges from unitary evolution. The system evolves into a mixture of pointer states, while the environment is populated with records holding redundant information about the pointer state. Then we will notice that the pointer states are overlapping, which opens up the possibility of the appearance of classical forces among the system particles. These two mechanisms, the generation of records and the emergence of classical forces are usually discussed separately, but actually have the very same origin: interaction with the environment. Thus they may interfere. I will argue that we should worry about two or even more dust particles, instead of just one.
15.00: Coffee
15.15: László E. Szabó: On the algebra of states of affairs
This talk will be a reflection on why in physics, be it classical or quantum, we think that there is an ontological content in talking about the "algebra of physical quantities".
16.45: Get-together at Bálna terasz
0 notes
Text
Understanding Techniques and Applications of Pattern Recognition in AI
Summary: Pattern recognition in AI involves identifying and classifying data patterns using various algorithms. It is crucial for applications like facial recognition and predictive analytics, employing techniques such as machine learning and neural networks.

Introduction
Pattern recognition in AI involves identifying patterns and regularities in data using various algorithms and techniques. It plays a pivotal role in AI by enabling systems to interpret and respond to complex inputs such as images, speech, and text.
Understanding pattern recognition is crucial because it underpins many AI applications, from facial recognition to predictive analytics. This article will explore the core techniques of pattern recognition, its diverse applications, and emerging trends, providing you with a comprehensive overview of how pattern recognition in AI drives technological advancements and practical solutions in various fields.
Read Blogs:
Artificial Intelligence Using Python: A Comprehensive Guide.
Unveiling the battle: Artificial Intelligence vs Human Intelligence.
What is Pattern Recognition?
Pattern recognition in AI refers to the process of identifying and classifying data patterns based on predefined criteria or learned from data. It involves training algorithms to recognize specific structures or sequences within datasets, enabling machines to make predictions or decisions.
By analyzing data patterns, AI systems can interpret complex information, such as visual images, spoken words, or textual data, and categorize them into meaningful classes.
Core concepts are:
Patterns: In pattern recognition, a pattern represents a recurring arrangement or sequence within data. These patterns can be simple, like identifying digits in a handwritten note, or complex, like detecting faces in a crowded image. Recognizing patterns allows AI systems to perform tasks like image recognition or fraud detection.
Features: Features are individual measurable properties or characteristics used to identify patterns. For instance, in image recognition, features might include edges, textures, or colors. AI models extract and analyze these features to understand and classify data accurately.
Classes: Classes are predefined categories into which patterns are grouped. For example, in a spam email filter, the classes could be "spam" and "not spam." The AI system learns to categorize new data into these classes based on the patterns and features it has learned.
Explore: Big Data and Artificial Intelligence: How They Work Together?
Techniques in Pattern Recognition
Pattern recognition encompasses various techniques that enable AI systems to identify patterns and make decisions based on data. Understanding these techniques is essential for leveraging pattern recognition effectively in different applications. Here’s an overview of some key methods:
Machine Learning Approaches
Supervised Learning: This approach involves training algorithms on labeled data, where the desired output is known. Classification and regression are two main techniques. Classification assigns input data to predefined categories, such as identifying emails as spam or not spam. Regression predicts continuous values, like forecasting stock prices based on historical data.
Unsupervised Learning: Unlike supervised learning, unsupervised learning works with unlabeled data to discover hidden patterns. Clustering groups similar data points together, which is useful in market segmentation or social network analysis. Dimensionality reduction techniques, such as Principal Component Analysis (PCA), simplify complex data by reducing the number of features while retaining essential information.
Statistical Methods
Bayesian Methods: Bayesian classification applies probability theory to predict the likelihood of a data point belonging to a particular class based on prior knowledge. Bayesian inference uses Bayes' theorem to update the probability of a hypothesis as more evidence becomes available.
Hidden Markov Models: These models are powerful for sequential data analysis, where the system being modeled is assumed to follow a Markov process with hidden states. They are widely used in speech recognition and bioinformatics for tasks like gene prediction and part-of-speech tagging.
Neural Networks
Deep Learning: Convolutional Neural Networks (CNNs) excel in pattern recognition tasks involving spatial data, such as image and video analysis. They automatically learn and extract features from raw data, making them effective for object detection and facial recognition.
Recurrent Neural Networks (RNNs): RNNs are designed for sequential and time-series data, where the output depends on previous inputs. They are used in applications like natural language processing and financial forecasting to handle tasks such as language modeling and trend prediction.
Other Methods
Template Matching: This technique involves comparing a given input with a predefined template to find the best match. It is commonly used in image recognition and computer vision tasks.
Feature Extraction and Selection: These techniques improve pattern recognition accuracy by identifying the most relevant features from the data. Feature extraction transforms raw data into a more suitable format, while feature selection involves choosing the most informative features for the model.
These techniques collectively enable AI systems to recognize patterns and make informed decisions, driving advancements across various domains.
Read: Secrets of Image Recognition using Machine Learning and MATLAB.
Applications of Pattern Recognition in AI

Pattern recognition is a cornerstone of artificial intelligence, driving innovations across various sectors. Its ability to identify and interpret patterns in data has transformative applications in multiple fields. Here, we explore how pattern recognition techniques are utilized in image and video analysis, speech and audio processing, text and natural language processing, healthcare, and finance.
Facial Recognition
Pattern recognition technology plays a pivotal role in facial recognition systems. By analyzing unique facial features, these systems identify individuals with high accuracy. This technology underpins security systems, user authentication, and even personalized marketing.
Object Detection
In autonomous vehicles, pattern recognition enables object detection to identify and track obstacles, pedestrians, and other vehicles. Similarly, in surveillance systems, it helps in monitoring and recognizing suspicious activities, enhancing security and safety.
Speech Recognition
Pattern recognition techniques convert spoken language into text with remarkable precision. This process involves analyzing acoustic signals and matching them to linguistic patterns, enabling voice-controlled devices and transcription services.
Music Genre Classification
By examining audio features such as tempo, rhythm, and melody, pattern recognition algorithms can classify music into genres. This capability is crucial for music streaming services that recommend songs based on user preferences.
Sentiment Analysis
Pattern recognition in NLP allows for the extraction of emotional tone from text. By identifying sentiment patterns, businesses can gauge customer opinions, enhance customer service, and tailor marketing strategies.
Topic Modeling
This technique identifies themes within large text corpora by analyzing word patterns and co-occurrences. Topic modeling is instrumental in organizing and summarizing vast amounts of textual data, aiding in information retrieval and content analysis.
Medical Imaging
Pattern recognition enhances diagnostic accuracy in medical imaging by detecting anomalies in X-rays, MRIs, and CT scans. It helps in early disease detection, improving patient outcomes.
Predictive Analytics
In healthcare, pattern recognition predicts patient outcomes by analyzing historical data and identifying trends. This predictive capability supports personalized treatment plans and proactive healthcare interventions.
Fraud Detection
Pattern recognition identifies unusual financial transactions that may indicate fraud. By analyzing transaction patterns, financial institutions can detect and prevent fraudulent activities in real-time.
Algorithmic Trading
In stock markets, pattern recognition algorithms analyze historical data to predict price movements and inform trading strategies. This approach helps traders make data-driven decisions and optimize trading performance.
See: What is Data-Centric Architecture in Artificial Intelligence?
Challenges and Limitations
Pattern recognition in AI faces several challenges that can impact its effectiveness and efficiency. Addressing these challenges is crucial for optimizing performance and achieving accurate results.
Data Quality and Quantity: High-quality data is essential for effective pattern recognition. Inadequate or noisy data can lead to inaccurate results. Limited data availability can also constrain the training of models, making it difficult for them to generalize well across different scenarios.
Computational Complexity: Advanced pattern recognition techniques, such as deep learning, require significant computational resources. These methods often involve processing large datasets and executing complex algorithms, which demand powerful hardware and substantial processing time. This can be a barrier for organizations with limited resources.
Overfitting and Underfitting: Overfitting occurs when a model learns the training data too well, including its noise, leading to poor performance on new data. Underfitting, on the other hand, happens when a model is too simple to capture the underlying patterns, resulting in inadequate performance. Balancing these issues is crucial for developing robust and accurate pattern recognition systems.
Addressing these challenges involves improving data collection methods, investing in computational resources, and carefully tuning models to avoid overfitting and underfitting.
Frequently Asked Questions
What is pattern recognition in AI?
Pattern recognition in AI is the process of identifying and classifying data patterns using algorithms. It helps machines interpret complex inputs like images, speech, and text, enabling tasks such as image recognition and predictive analytics.
How does pattern recognition benefit AI applications?
Pattern recognition enhances AI applications by enabling accurate identification of patterns in data. This leads to improved functionalities in areas like facial recognition, speech processing, and predictive analytics, driving advancements across various fields.
What are the main techniques used in pattern recognition in AI?
Key techniques in pattern recognition include supervised learning, unsupervised learning, Bayesian methods, hidden Markov models, and neural networks. These methods help in tasks like classification, clustering, and feature extraction, optimizing AI performance.
Conclusion
Pattern recognition in AI is integral to developing sophisticated systems that understand and interpret data. By utilizing techniques such as machine learning, statistical methods, and neural networks, AI can achieve tasks ranging from facial recognition to predictive analytics.
Despite challenges like data quality and computational demands, advances in pattern recognition continue to drive innovation across various sectors, making it a crucial component of modern AI applications.
0 notes
Text
6 Challenges in Solving Nash Equilibrium Assignment Problems in Macroeconomics
The Nash equilibrium is a concept from game theory that is very relevant to economics, particularly macroeconomics. Nash equilibrium was defined by the mathematician John Nash as a state in a game where no player can benefit by altering their strategies if the other player’s strategies remain unaltered. This concept of economics helps in comprehending different aspects related to oligopolistic markets and trading between countries.
From a learners’ perspective, Questions based on Nash equilibrium is commonly asked in exams and assignment. It is critical for comprehending how strategic decision are made. These problems may prove somewhat complicated because of the mathematics and the concepts of economics involved. Seeking assistance from macroeconomics assignment help expert can prove to be beneficial. These experts can explain the confusing concepts, explain principles in layman terms, and even provide guidance on the optimal ways to solve specific mathematical problems. We will talk about these services in more detail later, but let us elaborate the challenges first.
6 Challenges in Solving Nash Equilibrium Assignment Problems in Macroeconomics
1. Complexity of Multi-Player Games
Nash equilibrium problems are difficult due to the complexity involved in multi-player games. The games which include many participants are much more complex than the one having just two participants, as the former can have many possible strategies and outcomes. Each player’s returns are determined by the strategies of the other players. Hence, it is difficult to calculate the equilibrium due to multi-player complexity.
Example: In macroeconomics, let us consider an example of coordination of fiscal policies among multiple nations. The best policy of one nation essentially depends on the policies of other nation making the analysis extensive and complex.
2. Existence and Uniqueness of Equilibria
Some of the games may not possess Nash equilibrium and a game may possess more than one equilibria. Determining whether there exists an equilibrium and if it is unique can be quite challenging, particularly in continuous strategy contexts where traditional approaches are not effective.
Case Study: It was noted that, according to the Cournot competition model, which is inherent to oligopoly, there may be multiple Nash equilibria, especially with regard to firms having distinct production costs. To outline the most probable state of equilibrium one must deeply study in context of economic assumptions and stability tests.
3. Mathematical Rigor and Proof Techniques
Establishing the existence of a Nash equilibrium may require sophisticated concepts from mathematics, for instance, the fixed-point theorems. Most of the students faced difficulties in understanding the said concepts as well as relating them to issues in economics.
Textbook Reference: "Game Theory for Applied Economists" by Robert Gibbons is a useful book that breaks down these mathematical techniques in a way that’s easier for economics students to understand.
Fact: According to the "Journal of Economic Education", out of 100 percent of students over 70 percent have difficulties solving problems that require advanced mathematics for game theory.
4. Dynamic Games and Time Consistency
Changing scenarios involves different decisions and strategies that are adopted by players over different time periods. In such cases, one has to consider strategies over time which brings another complication of time consistencies.
Recent Example: Monetary policy of the European Central Bank must take into consideration the responses of other central banks and financial markets in the long-run. This dynamic aspect creates extra difficulties in accomplishing equilibrium computations.
5. Incomplete Information and Bayesian Equilibria
In most of the cases, players are unaware of the strategies and payoffs of other players. The underlying ideas for analyzing Nash equilibrium in these situations include Bayesian Nash equilibrium concepts which are more complicated.
Example: In labor markets, there is an unavailability of information where firms and workers do not know each other’s productivity and preferences. To use Nash equilibrium in these contexts, Bayesian equilibria can be used by the players to take decisions based on their beliefs.
Helpful Reference: "Microeconomic Theory" by Andreu Mas-Colell, Michael D. Whinston, and Jerry R. Green explores Bayesian equilibria in detail.
6. Behavioral Considerations and Bounded Rationality
The conventional Nash equilibrium is based on the assumption that prospective players are precisely rational. But in real life players may display bounded rationality where their strategies are limited by cognitive bias and heuristics.
Insight: Nash equilibrium analysis that incorporates behavioral economics is an upcoming field. It is necessary to understand how the real-world behavior deviates from rationality and impacts the equilibrium for attaining precise economic modelling.
Case Study: The 2008 financial crisis showed that due to the bounded rationality and tendency to follow herd behavior amongst investors led to poor equilibria resulting in economic instability.
Importance of Macroeconomics Assignment Help Service for Students Struggling with Nash Equilibrium
With dynamic market and changing scenarios, solving various Nash equilibrium problems can present quite a number of challenges to many students. Acknowledging this, our macroeconomics assignment help service is committed to delivering the expert assistance that is required to address such needs. It is for this reason that we provide students with custom services from our professional team who provide easy-to-follow directions when solving complex problems like Nash equilibrium.
Common Exam Questions on Nash Equilibrium and How to Approach Them
Exam questions on Nash equilibrium can vary in complexity, but they typically fall into a few common categories. Here are some examples and the correct approach to solving them:
1. Identifying Nash Equilibrium in Simple Games:
Question: Given a payoff matrix for a two-player game, identify the Nash equilibrium.
Approach:
Construct the Payoff Matrix: Clearly outline the strategies and corresponding payoffs for each player.
Best Response Analysis: For each player, identify the best response to every possible strategy of the other player.
Equilibrium Identification: Determine where the best responses intersect, indicating no player can improve their payoff by unilaterally changing their strategy.
2. Solving for Nash Equilibrium in Continuous Strategy Spaces:
Question: Given a duopoly model with continuous strategies, find the Nash equilibrium.
Approach:
Set Up the Problem: Define the profit functions for each firm based on their production quantities.
First-Order Conditions: Derive the first-order conditions for profit maximization for each firm.
Simultaneous Equations: Solve the resulting system of simultaneous equations to find the equilibrium quantities.
3. Dynamic Games and Subgame Perfect Equilibrium:
Question: Analyze a sequential game and determine the subgame perfect Nash equilibrium.
Approach:
Game Representation: Use extensive form to represent the game, highlighting decision nodes and payoffs.
Backward Induction: Apply backward induction to solve the game, starting from the final decision node and working backwards to the initial node.
4. Games with Incomplete Information:
Question: Find the Bayesian Nash equilibrium for a game with incomplete information.
Approach:
Define Types and Payoffs: Specify the types of players and their respective payoff functions.
Belief Formation: Establish the beliefs each player has about the types of the other players.
Bayesian Equilibrium Analysis: Solve for the strategies that maximize each player's expected payoff, given their beliefs.
Benefits of Our Macroeconomics Assignment Help Service
Our macroeconomics homework assistance service provides numerous benefits to students struggling with Nash equilibrium problems:
Expert Guidance: Our team includes exclusively skilled and experienced tutors in game theory and macroeconomics that are eager to perform their job. They can simplify a problem into achievable sub-tasks, guaranteeing that the students fully understand the issue.
Customized Solutions: We provide one-on-one tutoring services where students get assistance according to their learning difficulties they may encounter in their academic achievement.
Practical Problem-Solving Techniques: The guidance given by our tutors contain step-by-step solutions and strategies to solve Nash equilibrium problems as well as effective ways to develop strong problem-solving skills among the students.
Comprehensive Support: Our service provides high quality homework assignment solutions, as well as exam preparation help for all Nash equilibrium problem to prepare students throughout their coursework.
Conclusion
In macroeconomics, there are many assignment problems that are built around understanding the Nash equilibrium concepts. Due to the reasons such as, presence of multiple players in a game, involvement of mathematical modeling and analysis, existence of incomplete information, behavioral properties, such problems are quite hard. But, with proper guidance, helpful study material and personalized students can understand these concepts well.
Furthermore, other resources like textbooks “Game Theory for Applied Economists” written by Robert Gibbons, “Microeconomic Theory” by Andreu Mas-Colell, Michael D. Whinston, and Jerry R. Green can also be helpful in extending the understanding and competence of the students solving the Nash equilibrium problems.
Our macroeconomics assignment support service is equipped with all the teaching tools, techniques and material needed to overcome such challenges. With our services, students not only do well on the assignments and exams but also understand how strategy is played out in different economic interactions.
0 notes
Link
This book introduces students to probability, statistics, and stochastic processes. It can be used by both students and practitioners in engineering, various sciences, finance, and other related fields. It provides a clear and intuitive approach to these topics while maintaining mathematical accuracy. The book covers: Basic concepts such as random experiments, probability axioms, conditional probability, and counting methods Single and multiple random variables (discrete, continuous, and mixed), as well as moment-generating functions, characteristic functions, random vectors, and inequalities Limit theorems and convergence Introduction to Bayesian and classical statistics Random processes including processing of random signals, Poisson processes, discrete-time and continuous-time Markov chains, and Brownian motion Simulation using MATLAB, R, and Python (online chapters) The book contains a large number of solved exercises. The dependency between different sections of this book has been kept to a minimum in order to provide maximum flexibility to instructors and to make the book easy to read for students. Examples of applications—such as engineering, finance, everyday life, etc.—are included to aid in motivating the subject. The digital version of the book, as well as additional materials such as videos, is available at www.probabilitycourse.com. roduct details Publisher : Kappa Research, LLC (August 24, 2014) Language : English Paperback : 746 pages ISBN-10 : 0990637204 ISBN-13 : 978-0990637202
0 notes
Text
Bayesian Statistics: A Powerful Tool for Uncertainty Modeling
Bayesian statistics is a framework for handling uncertainty that has become increasingly popular in various fields. It provides a flexible and systematic approach to modeling and quantifying uncertainty, allowing us to make better-informed decisions. In this article, we will delve into the foundations of Bayesian statistics, understand its significance in uncertainty modeling, and explore its applications in real-world scenarios.
Defining Bayesian Statistics
Bayesian statistics can be defined as a framework for reasoning about uncertainty.
It is based on Bayes’ theorem, which provides a mathematical formula for updating our beliefs in the presence of new evidence.
Bayesian statistics allows us to incorporate prior knowledge and update it with data to obtain posterior probabilities.
Historical Context of Bayesian Statistics
In the 18th century, the Reverend Thomas Bayes introduced the theorem that forms the backbone of Bayesian statistics. However, it was not until the 20th century that Bayesian methods started gaining prominence in academic research and practical applications. With the advent of computational tools and increased recognition of uncertainty, Bayesian statistics has evolved into a powerful tool for modeling and decision-making.
Significance of Uncertainty Modeling
Uncertainty is intrinsic to many real-world phenomena, from complex biological systems to financial markets. Accurately modeling and quantifying uncertainty is crucial for making informed decisions and predictions. Bayesian statistics plays a vital role in addressing uncertainty by providing a probabilistic framework that allows us to account for inherent variability and incorporate prior knowledge into our analysis.
Foundations of Bayesian Statistics
A. Bayes’ Theorem
Bayes’ theorem is at the core of Bayesian statistics and provides a formula for updating our beliefs based on new evidence. It enables us to revise our prior probabilities in light of observed data. Mathematically, Bayes’ theorem can be expressed as:
P(A|B) = (P(B|A) * P(A)) / P(B)
Bayes’ theorem allows us to explicitly quantify and update our beliefs as we gather more data, resulting in more accurate and precise estimates.
B. Prior and Posterior Probability
In Bayesian inference, we begin with an initial belief about a parameter of interest, expressed through the prior probability distribution. The prior distribution represents what we believe about the parameter before observing any data. As new data becomes available, we update our beliefs using Bayes’ theorem, resulting in the posterior distribution. The posterior probability distribution reflects our updated beliefs after considering the data.
The prior probability distribution acts as a regularization term, influencing the final estimates. It allows us to incorporate prior knowledge, domain expertise, or informed assumptions into the analysis. On the other hand, the posterior distribution represents our refined knowledge about the parameter, considering both the prior beliefs and the observed data.
Bayesian Inference Process
A. Likelihood Function
The likelihood function plays a pivotal role in Bayesian statistics as it captures the relationship between the observed data and the unknown parameters. It quantifies the probability of obtaining the observed data under different parameter values. By maximizing the likelihood function, we can estimate the most probable values for the parameters of interest.
The likelihood function is a key component in Bayesian inference, as it combines the data with the prior information to update our beliefs. By calculating the likelihood for different parameter values, we can explore the range of potential parameter values that are consistent with the observed data.
B. Posterior Distribution
The posterior distribution is the ultimate goal of Bayesian inference. It represents the updated distribution of the parameters of interest after incorporating the prior beliefs and the observed data. The posterior distribution provides a comprehensive summary of our uncertainty and captures the trade-off between prior knowledge and new evidence.
Bayesian updating involves multiplying the prior distribution by the likelihood function and normalizing it to obtain the posterior distribution. This process allows us to continually refine our estimates as more data becomes available. The posterior distribution represents the most up-to-date knowledge about the parameters and encompasses both uncertainty and variability.
Bayesian Models and Applications
A. Bayesian Parameter Estimation
Bayesian statistics offers a robust framework for parameter estimation. It allows us to estimate unknown parameters and quantify the associated uncertainty in a principled manner. By incorporating prior knowledge in the form of prior distributions, Bayesian parameter estimation can make efficient use of limited data.
In fields such as finance, Bayesian parameter estimation has found applications in option pricing, risk management, and portfolio optimization. In healthcare, Bayesian models have been utilized for personalized medicine, clinical trials, and disease prognosis. The ability to incorporate prior information and continuously update estimates makes Bayesian parameter estimation a powerful tool in various domains.
B. Bayesian Hypothesis Testing
Bayesian hypothesis testing provides an alternative to frequentist methods by offering a way to quantify the evidence in favor of different hypotheses. Unlike frequentist methods that rely on p-values, Bayesian hypothesis testing uses posterior probabilities to assess the likelihood of different hypotheses given the data.
By incorporating prior information into the analysis, Bayesian hypothesis testing allows for more informative decision-making. It avoids some of the pitfalls of frequentist methods, such as the reliance on arbitrary significance levels. Bayesian hypothesis testing has found applications in research, industry, and policy-making, providing a more intuitive and flexible approach to drawing conclusions.
Uncertainty Propagation
A. Uncertainty Quantification
Uncertainty quantification is a fundamental aspect of Bayesian modeling, enabling us to understand and communicate the uncertainty associated with model outputs. It provides a means to quantify the inherent variability and lack of perfect information in our predictions.
Methods for uncertainty quantification in Bayesian modeling include calculating credible intervals or using Bayesian hierarchical models to capture uncertainty at different levels of the modeling process. Uncertainty quantification allows decision-makers to account for ambiguity and risk when interpreting and utilizing model outputs.
B. Monte Carlo Methods
Monte Carlo methods are widely used for uncertainty propagation in Bayesian analysis. These techniques, including Markov Chain Monte Carlo (MCMC), allow for efficient sampling from complex posterior distributions, which often have no closed-form analytic solution.
MCMC algorithms iteratively draw samples from the posterior distribution, exploring the parameter space to approximate the true distribution. These samples can then be used to estimate summary statistics, compute credible intervals, or perform model comparison. Monte Carlo methods, especially MCMC, have revolutionized Bayesian analysis and made it feasible to handle complex and high-dimensional models.
Bayesian Machine Learning
A. Bayesian Neural Networks
Bayesian statistics can be integrated into neural networks, resulting in Bayesian neural networks (BNNs). BNNs provide a principled way to incorporate uncertainty estimation within the neural network framework.
By placing priors on the network weights, BNNs enable us to capture uncertainty in the network’s predictions. Bayesian neural networks are particularly useful when data is limited, as they provide more realistic estimates of uncertainty compared to traditional neural networks.
The benefits of Bayesian neural networks extend to a wide range of applications, including anomaly detection, reinforcement learning, and generative modeling.
B. Bayesian Model Selection
Model selection is a critical step in statistical modeling and Bayesian techniques offer reliable approaches to tackle this challenge. Bayesian model selection allows for direct comparison of different models and quantifying the evidence in favor of each model based on the observed data.
Bayesian Information Criterion (BIC) is one of the widely used metrics in Bayesian model selection. It balances the goodness-of-fit of the model with model complexity to avoid overfitting. By accounting for the uncertainty in model selection, Bayesian methods provide a principled approach for choosing the most appropriate model.
Challenges and Considerations
A. Computational Complexity
Bayesian analysis often involves complex models with a high dimensional parameter space, which presents computational challenges. Sampling from and exploring the posterior distribution can be computationally expensive, especially when dealing with large datasets or intricate models.
To overcome these challenges, researchers have developed advanced sampling algorithms such as Hamiltonian Monte Carlo and variational inference techniques. Additionally, the availability of high-performance computing resources has made it easier to tackle computationally demanding Bayesian analyses.
B. Data Requirements
Bayesian modeling relies on the availability of sufficient data to reliably estimate parameters and quantify uncertainty. In cases where data is limited, such as in rare diseases or in emerging fields, Bayesian approaches need to be supplemented with expert knowledge and informative priors.
However, even with limited data, Bayesian techniques can be valuable. By incorporating external information through prior distributions, Bayesian models can leverage existing knowledge and provide reasonable estimates even in data-scarce settings.
Real-World Examples
A. Bayesian Statistics in Finance
Bayesian methods have demonstrated their utility in various financial applications. In risk assessment, Bayesian statistics allows for the incorporation of historical data, expert knowledge, and subjective opinions to estimate the probabilities of market events. Bayesian portfolio optimization considers both expected returns and uncertainty to construct portfolios that balance risk and return.
Credit scoring also benefits from Bayesian statistics, enabling lenders to make accurate predictions by incorporating information from credit bureaus, loan applications, and other relevant sources. Bayesian statistics in finance provides a flexible and rigorous framework for decision-making in uncertain financial markets.
B. Bayesian Statistics in Healthcare
Bayesian statistics has made significant contributions to healthcare decision-making. In medical diagnosis, Bayesian models can combine patient symptoms, test results, and prior information to estimate the probability of disease. Bayesian approaches to drug development utilize prior knowledge, clinical trial data, and animal studies to optimize drug dosage and minimize risks.
In epidemiology, Bayesian statistics is employed to estimate disease prevalence, evaluate the effectiveness of interventions, and forecast future disease trends. Bayesian statistics enhances healthcare decision-making by integrating various sources of information and addressing uncertainty in medical research and practice.
Advancements and Tools
A. Bayesian Software and Packages
Several software packages and libraries have been developed to facilitate Bayesian analysis. Popular tools include:
Stan: A probabilistic programming language that allows for flexible modeling and efficient computation of Bayesian models.
PyMC3: A Python library that provides a simple and intuitive interface for probabilistic programming with Bayesian inference.
JAGS: Just Another Gibbs Sampler, a program for Bayesian analysis using Markov chain Monte Carlo (MCMC) algorithms.
These tools provide user-friendly interfaces, efficient sampling algorithms, and a wide range of pre-built models, making Bayesian analysis accessible to researchers and practitioners across different domains.
B. Recent Developments
Bayesian statistics that continues to evolve with ongoing research and technological advancements. Recent developments include advancements in scalable Bayesian computation, hierarchical modeling, and deep learning with Bayesian approaches. Emerging applications in fields such as autonomous driving, natural language processing, and Bayesian optimization highlight the versatility and expanding reach of Bayesian statistics.
As researchers continue to innovate, Bayesian statistics will remain a powerful tool for uncertainty modeling, providing decision-makers with more accurate estimates, better predictions, and improved risk assessment.
In conclusion, Bayesian statistics offers a compelling framework for uncertainty modeling that has wide-ranging applications across various disciplines. Through the use of prior knowledge, data updating, and careful estimation of posterior distributions, Bayesian statistics enables us to make informed decisions in the face of uncertainty. By acknowledging and quantifying uncertainty, Bayesian statistics empowers decision-makers to account for risk and make better-informed choices. Its flexibility, ability to handle complex models, and emphasis on incorporating prior knowledge make Bayesian statistics an invaluable tool for uncertainty modeling in today’s data-driven world. Embracing the Bayesian approach can unlock new insights, provide more accurate predictions, and enable proactive decision-making. With the advancement of computational tools and the availability of user-friendly software, exploring Bayesian statistics has become more accessible and practical for researchers and practitioners alike. To assist people in their pursuit of a Data Science education, ACTE Institute offers a variety of Data Science courses, boot camps, and degree programs. Let us embrace Bayesian statistics and harness its power for robust uncertainty modeling in our respective fields.
0 notes