#ampere cpu
Explore tagged Tumblr posts
Text
Ampere CPUs Processor Overview
Ampere CPUs are advanced processors designed for high performance and energy efficiency. They are based on ARM architecture and are used in various applications, including cloud computing, AI, and edge computing. Ampere CPUs are known for their high core count, which allows them to handle many tasks simultaneously, making them ideal for modern, scalable computing environments.They use less energy and lower costs, making them a popular choice for businesses and cloud providers who need efficient and powerful computing.
In this article, we are talking about Ampere CPUs, covering their architecture, key product lines, and notable performance characteristics. We’ll explore the energy efficiency that sets Ampere apart, its unique market positioning, and rapid adoption within cloud environments. We’ll also look into the software ecosystem built around these processors, their manufacturing processes, and customization options. Finally, we discuss Ampere's future developments and their impact on the server CPU market.
👉 Read the full article here
1 note
·
View note
Note
h. how about. hackerman j0hn in mushroom forest
This took too long cause I kept getting distracted.
80 notes
·
View notes
Text
AMPERE Altra Q64-22 CPU on ASRock micro-ATX motherboard

In addition to the Altra Q64-22 CPU that comes with the motherboard, the ASRock ALTRAD8UD-1L2T supports the much more expensive Altra Max lineup, which has CPUs with 96–128 cores.
Ampere Altra Q64-22 CPU Compatibility with ASRock’s micro-ATX
ALTRAD8UD-1L2T, a 10-inch micro-ATX motherboard, is packed with features. Four PCIe 4.0 x16 slots, two NVMe slots, ten gigabit Ethernet, and eight 256GB DDR4-3200 RAM slots are highlights. Although it doesn’t support PCIe 5.0 or DDR5, this motherboard isn’t old.
The package includes Ampere’s first-generation Altra CPU’s 64-core Altra Q64-22 CPU. Since Ampere didn’t launch the Q64-22 in 2020, its launch date is unknown.
But with Ampere’s Altra Max CPUs, which have up to 128 cores, why even bother with the Q64-22? These 2021 second-generation chips are mostly meant for cloud workloads, but they also provide significantly better multi-threaded performance and excellent value per core. They are strikingly similar to AMD’s 128-core Zen 4c-based Bergamo chips, which are supposedly designed with cloud servers and data centers in mind.
Naturally, Altra Max chips are also getting a bit dated now. As they were only marginally faster than 64-core Epyc Milan CPUs in 2021, we can probably assume AMD’s more recent 96-core and 128-core Epyc Genoa and Bergamo server CPUs are significantly faster. Even 128-core Altra Max CPUs should not stand a chance against Intel’s 64-core Emerald Rapids chips, particularly when it comes to AI workloads. Ampere has also configured its own 192-core AmpereOne CPU to overclock Altra Max.
Getting this ASRock motherboard and Ampere CPU bundle is a question that needs to be answered. Since both the Altra Q64-22 CPU and the ALTRAD8UD-1L2T motherboard aren’t available for retail purchase, it’s difficult to estimate how much they would cost separately. But the bundle only cost half of the $3,090 MSRP of the slightly more expensive Q64-24 in 2020. Even end users can afford the bundle, which comes with $1,500, but it does not cover the price of RAM, storage, or GPU.
Ampere AC-106409502 64-Bit Multi-Core Q64-22 2.20GHz 64-Core Processor – Altra
Offers 2.20GHz speed maximum with 64-core.
Ampere Altra with 64-Bit Multi-Core Processor.
Include Advanced Configuration Power Interface.
1 MB L2 cache per core, with 7 nm process technology.
Read more on Govindhtech.com
#AMPERE#AltraQ6422#CPU#motherboard#asrock#micro#ASRockmotherboard#AmpereCPU#technews#technology#govindhtech
1 note
·
View note
Text
Share Your Anecdotes: Multicore Pessimisation
I took a look at the specs of new 7000 series Threadripper CPUs, and I really don't have any excuse to buy one, even if I had the money to spare. I thought long and hard about different workloads, but nothing came to mind.
Back in university, we had courses about map/reduce clusters, and I experimented with parallel interpreters for Prolog, and distributed computing systems. What I learned is that the potential performance gains from better data structures and algorithms trump the performance gains from fancy hardware, and that there is more to be gained from using the GPU or from re-writing the performance-critical sections in C and making sure your data structures take up less memory than from multi-threaded code. Of course, all this is especially important when you are working in pure Python, because of the GIL.
The performance penalty of parallelisation hits even harder when you try to distribute your computation between different computers over the network, and the overhead of serialisation, communication, and scheduling work can easily exceed the gains of parallel computation, especially for small to medium workloads. If you benchmark your Hadoop cluster on a toy problem, you may well find that it's faster to solve your toy problem on one desktop PC than a whole cluster, because it's a toy problem, and the gains only kick in when your data set is too big to fit on a single computer.
The new Threadripper got me thinking: Has this happened to somebody with just a multicore CPU? Is there software that performs better with 2 cores than with just one, and better with 4 cores than with 2, but substantially worse with 64? It could happen! Deadlocks, livelocks, weird inter-process communication issues where you have one process per core and every one of the 64 processes communicates with the other 63 via pipes? There could be software that has a badly optimised main thread, or a badly optimised work unit scheduler, and the limiting factor is single-thread performance of that scheduler that needs to distribute and integrate work units for 64 threads, to the point where the worker threads are mostly idling and only one core is at 100%.
I am not trying to blame any programmer if this happens. Most likely such software was developed back when quad-core CPUs were a new thing, or even back when there were multi-CPU-socket mainboards, and the developer never imagined that one day there would be Threadrippers on the consumer market. Programs from back then, built for Windows XP, could still run on Windows 10 or 11.
In spite of all this, I suspect that this kind of problem is quite rare in practice. It requires software that spawns one thread or one process per core, but which is deoptimised for more cores, maybe written under the assumption that users have for two to six CPU cores, a user who can afford a Threadripper, and needs a Threadripper, and a workload where the problem is noticeable. You wouldn't get a Threadripper in the first place if it made your workflows slower, so that hypothetical user probably has one main workload that really benefits from the many cores, and another that doesn't.
So, has this happened to you? Dou you have a Threadripper at work? Do you work in bioinformatics or visual effects? Do you encode a lot of video? Do you know a guy who does? Do you own a Threadripper or an Ampere just for the hell of it? Or have you tried to build a Hadoop/Beowulf/OpenMP cluster, only to have your code run slower?
I would love to hear from you.
13 notes
·
View notes
Text
You don't need to be an engineer to make that


Look what horrible contraption I have created without any engineering degree whatsoever - it is a desktop CPU cooler, adapted so it can cool a Xeon (and it barely covers the whole heat spreader)
And yes that fan IS tapped directly into motherboard 12V because for some reason it draws 2 amperes
many inventions…. must continue research
11K notes
·
View notes
Text
Comprehensive Guide to Choosing the Right Graphics Card for Your PC
Importance of a Graphics Card for Your PC
A graphics card is a critical component in any PC, especially for those interested in gaming, content creation, or running demanding applications. It plays a pivotal role in rendering images, video, and animations, making it essential for achieving optimal performance and visual quality.
Most Expensive Graphics Card
Most Expensive Calculator
Overview of What This Guide Covers
This comprehensive guide will walk you through everything you need to know about graphics cards, from understanding the basics to choosing the right one for your needs. We’ll explore different types of graphics cards, key specifications, compatibility issues, performance considerations, and more.
2. Understanding Graphics Cards
What is a Graphics Card?
A graphics card, also known as a GPU (Graphics Processing Unit), is a specialized electronic circuit designed to accelerate the creation of images and videos on a display device. It offloads the graphical processing tasks from the CPU, enabling smoother and faster rendering of high-quality visuals.
Key Components of a Graphics Card
Graphics cards consist of several key components:
GPU: The core processor of the card responsible for rendering graphics.
VRAM: Video RAM used for storing graphical data and textures.
Cooling System: Fans and heat sinks to manage the GPU’s temperature.
Power Connectors: Provide the necessary power from the PSU to the GPU.
3. Types of Graphics Cards
Integrated vs. Dedicated Graphics Cards
Integrated Graphics Cards: Built into the CPU and share system memory. They are sufficient for basic tasks but lack the power for intensive applications.
Dedicated Graphics Cards: Standalone units with their own VRAM and processing power, ideal for gaming, video editing, and other demanding tasks.
Consumer vs. Professional Graphics Cards
Consumer Graphics Cards: Designed for gaming and general use, focusing on performance and affordability.
Professional Graphics Cards: Built for tasks like 3D modeling, CAD, and AI, offering superior precision and stability but at a higher cost.
4. Key Specifications to Consider
GPU Architecture and Cores
The architecture of the GPU and the number of cores directly influence its performance. Modern GPUs like NVIDIA’s Ampere or AMD’s RDNA 2 are designed for high efficiency and power.
VRAM: Memory Size and Bandwidth
VRAM is crucial for storing textures, frame buffers, and other data. More VRAM allows for better performance at higher resolutions and with more demanding textures.
Clock Speed and Overclocking
Clock speed determines how fast the GPU processes data. Overclocking can boost performance but may require better cooling solutions.
Power Consumption and Cooling Solutions
High-performance GPUs consume more power and generate more heat, necessitating adequate power supply units (PSUs) and effective cooling systems.
5. Compatibility Considerations
Motherboard Compatibility
Ensure that your graphics card is compatible with your motherboard’s PCIe slots. Most modern GPUs require PCIe x16 slots.
Power Supply Requirements
Check the power requirements of your chosen GPU and ensure your PSU can supply enough power, including the necessary connectors.
Case Size and Physical Dimensions
Graphics cards come in various sizes, so it’s essential to verify that your PC case can accommodate the card, particularly in terms of length, width, and height.
6. Performance vs. Price
Benchmarking: Understanding Performance Metrics
Benchmarking tools like 3DMark provide performance scores that help compare different GPUs. These scores can be crucial when balancing price and performance.
Price-to-Performance Ratio
Consider the price-to-performance ratio to ensure you get the best value for your money. Sometimes, mid-range cards offer better performance per dollar than high-end models.
7. Popular Graphics Card Brands
NVIDIA: GeForce Series
NVIDIA’s GeForce series is known for its high performance, particularly in gaming. The latest GeForce RTX 30 series offers cutting-edge technology like ray tracing.
AMD: Radeon Series
AMD’s Radeon series provides strong competition to NVIDIA, often offering better value at lower prices. The Radeon RX 6000 series is known for its excellent performance in both gaming and content creation.
Intel: Arc Series
Intel’s Arc series is a newcomer, focusing on offering a balanced mix of performance and affordability, targeting the mid-range market.
8. Gaming Graphics Cards
Best GPUs for 1080p Gaming
For 1080p gaming, GPUs like the NVIDIA GeForce RTX 3060 or AMD Radeon RX 6600 provide excellent performance at a reasonable price.
Best GPUs for 1440p and 4K Gaming
Higher resolutions require more powerful GPUs like the NVIDIA GeForce RTX 3080 or AMD Radeon RX 6800 XT.
Ray Tracing and DLSS Technology
Ray tracing enhances lighting and reflections, creating more realistic visuals. DLSS (Deep Learning Super Sampling) uses AI to upscale lower resolutions, improving performance without sacrificing image quality.
9. Graphics Cards for Content Creation
GPUs for Video Editing
For video editing, a GPU with high VRAM and CUDA cores (for NVIDIA cards) is ideal. The NVIDIA RTX 3070 or AMD Radeon RX 6700 XT are good options.
GPUs for 3D Rendering
3D rendering demands powerful GPUs with high CUDA or Stream Processor counts. The NVIDIA RTX 3090 or AMD Radeon RX 6900 XT are top choices.
GPUs for Machine Learning and AI
For AI and machine learning tasks, GPUs with Tensor cores (NVIDIA) or high compute performance are essential. The NVIDIA A100 or AMD Instinct series are designed for such workloads.
10. Buying Guide
New vs. Used Graphics Cards
While new cards come with warranties and the latest technology, used cards can offer significant savings. However, used cards may have reduced performance due to wear.
Where to Buy: Online vs. Retail
Online stores often offer a wider selection and better prices, but physical stores allow you to inspect the card before purchase.
Warranties and Return Policies
Ensure the card comes with a good warranty and understand the return policy, especially if purchasing used or refurbished products.
11. Expert Insights
Interview with a Hardware Specialist
We interviewed a hardware specialist who emphasized the importance of understanding your specific needs before choosing a GPU. They also highlighted the common mistake of overestimating power needs, leading to unnecessary spending.
Common Mistakes to Avoid When Choosing a GPU
Overpaying for a card that far exceeds your needs.
Ignoring compatibility with existing components.
Focusing solely on brand names without comparing actual performance metrics.
12. Future Trends in Graphics Cards
The Rise of AI-Powered GPUs
AI is increasingly integrated into GPUs, with NVIDIA’s Tensor cores leading the way. This technology enhances rendering, upscaling, and real-time processing.
Advances in Ray Tracing Technology
Ray tracing continues to evolve, becoming more accessible in mid-range cards, allowing more gamers to experience enhanced graphics.
Sustainability and Energy Efficiency in Future GPUs
Future GPUs are likely to focus more on energy efficiency, reducing the environmental impact of high-performance computing.
13. Practical Tips
How to Properly Install a Graphics Card
Ensure your PC is powered off and unplugged before installation. Carefully insert the card into the PCIe slot and secure it with screws. Connect the necessary power cables and close your case.
Maintaining and Upgrading Your GPU
Regularly clean your GPU to prevent dust buildup. Consider upgrading your GPU when it can no longer meet your performance needs, but balance this with the cost of new technology.
14. FAQs
What is the Difference Between VRAM and RAM?
VRAM is specifically used by the GPU for handling graphical data, while RAM is used by the CPU for general tasks. VRAM is crucial for high-resolution gaming and rendering.
Can I Use a Gaming GPU for Professional Work?
Yes, gaming GPUs can handle professional tasks like video editing or 3D rendering, but professional GPUs are optimized for such tasks and offer better stability and support.
How Often Should I Upgrade My Graphics Card?
It depends on your usage and the advancement of technology. Typically, upgrading every 3-5 years is sufficient unless you need to keep up with cutting-edge applications or games.
15. Conclusion
Summary of Key Points
Choosing the right graphics card depends on understanding your needs, considering specifications like VRAM and GPU cores, and ensuring compatibility with your system. Balancing performance and price is crucial, as is staying informed about future trends.
0 notes
Text
Ampere AmpereOne Aurora 512 Core AI CPU Announced
https://www.servethehome.com/ampere-ampereone-aurora-512-core-ai-cpu-announced-arm/
0 notes
Text
Setting Up a Training, Fine-Tuning, and Inferencing of LLMs with NVIDIA GPUs and CUDA
New Post has been published on https://thedigitalinsider.com/setting-up-a-training-fine-tuning-and-inferencing-of-llms-with-nvidia-gpus-and-cuda/
Setting Up a Training, Fine-Tuning, and Inferencing of LLMs with NVIDIA GPUs and CUDA
The field of artificial intelligence (AI) has witnessed remarkable advancements in recent years, and at the heart of it lies the powerful combination of graphics processing units (GPUs) and parallel computing platform.
Models such as GPT, BERT, and more recently Llama, Mistral are capable of understanding and generating human-like text with unprecedented fluency and coherence. However, training these models requires vast amounts of data and computational resources, making GPUs and CUDA indispensable tools in this endeavor.
This comprehensive guide will walk you through the process of setting up an NVIDIA GPU on Ubuntu, covering the installation of essential software components such as the NVIDIA driver, CUDA Toolkit, cuDNN, PyTorch, and more.
The Rise of CUDA-Accelerated AI Frameworks
GPU-accelerated deep learning has been fueled by the development of popular AI frameworks that leverage CUDA for efficient computation. Frameworks such as TensorFlow, PyTorch, and MXNet have built-in support for CUDA, enabling seamless integration of GPU acceleration into deep learning pipelines.
According to the NVIDIA Data Center Deep Learning Product Performance Study, CUDA-accelerated deep learning models can achieve up to 100s times faster performance compared to CPU-based implementations.
NVIDIA’s Multi-Instance GPU (MIG) technology, introduced with the Ampere architecture, allows a single GPU to be partitioned into multiple secure instances, each with its own dedicated resources. This feature enables efficient sharing of GPU resources among multiple users or workloads, maximizing utilization and reducing overall costs.
Accelerating LLM Inference with NVIDIA TensorRT
While GPUs have been instrumental in training LLMs, efficient inference is equally crucial for deploying these models in production environments. NVIDIA TensorRT, a high-performance deep learning inference optimizer and runtime, plays a vital role in accelerating LLM inference on CUDA-enabled GPUs.
According to NVIDIA’s benchmarks, TensorRT can provide up to 8x faster inference performance and 5x lower total cost of ownership compared to CPU-based inference for large language models like GPT-3.
NVIDIA’s commitment to open-source initiatives has been a driving force behind the widespread adoption of CUDA in the AI research community. Projects like cuDNN, cuBLAS, and NCCL are available as open-source libraries, enabling researchers and developers to leverage the full potential of CUDA for their deep learning.
Installation
When setting AI development, using the latest drivers and libraries may not always be the best choice. For instance, while the latest NVIDIA driver (545.xx) supports CUDA 12.3, PyTorch and other libraries might not yet support this version. Therefore, we will use driver version 535.146.02 with CUDA 12.2 to ensure compatibility.
Installation Steps
1. Install NVIDIA Driver
First, identify your GPU model. For this guide, we use the NVIDIA GPU. Visit the NVIDIA Driver Download page, select the appropriate driver for your GPU, and note the driver version.
To check for prebuilt GPU packages on Ubuntu, run:
sudo ubuntu-drivers list --gpgpu
Reboot your computer and verify the installation:
nvidia-smi
2. Install CUDA Toolkit
The CUDA Toolkit provides the development environment for creating high-performance GPU-accelerated applications.
For a non-LLM/deep learning setup, you can use:
sudo apt install nvidia-cuda-toolkit However, to ensure compatibility with BitsAndBytes, we will follow these steps: [code language="BASH"] git clone https://github.com/TimDettmers/bitsandbytes.git cd bitsandbytes/ bash install_cuda.sh 122 ~/local 1
Verify the installation:
~/local/cuda-12.2/bin/nvcc --version
Set the environment variables:
export CUDA_HOME=/home/roguser/local/cuda-12.2/ export LD_LIBRARY_PATH=/home/roguser/local/cuda-12.2/lib64 export BNB_CUDA_VERSION=122 export CUDA_VERSION=122
3. Install cuDNN
Download the cuDNN package from the NVIDIA Developer website. Install it with:
sudo apt install ./cudnn-local-repo-ubuntu2204-8.9.7.29_1.0-1_amd64.deb
Follow the instructions to add the keyring:
sudo cp /var/cudnn-local-repo-ubuntu2204-8.9.7.29/cudnn-local-08A7D361-keyring.gpg /usr/share/keyrings/
Install the cuDNN libraries:
sudo apt update sudo apt install libcudnn8 libcudnn8-dev libcudnn8-samples
4. Setup Python Virtual Environment
Ubuntu 22.04 comes with Python 3.10. Install venv:
sudo apt-get install python3-pip sudo apt install python3.10-venv
Create and activate the virtual environment:
cd mkdir test-gpu cd test-gpu python3 -m venv venv source venv/bin/activate
5. Install BitsAndBytes from Source
Navigate to the BitsAndBytes directory and build from source:
cd ~/bitsandbytes CUDA_HOME=/home/roguser/local/cuda-12.2/ LD_LIBRARY_PATH=/home/roguser/local/cuda-12.2/lib64 BNB_CUDA_VERSION=122 CUDA_VERSION=122 make cuda12x CUDA_HOME=/home/roguser/local/cuda-12.2/ LD_LIBRARY_PATH=/home/roguser/local/cuda-12.2/lib64 BNB_CUDA_VERSION=122 CUDA_VERSION=122 python setup.py install
6. Install PyTorch
Install PyTorch with the following command:
pip install torch torchvision torchaudio --index-url https://download.pytorch.org/whl/cu121
7. Install Hugging Face and Transformers
Install the transformers and accelerate libraries:
pip install transformers pip install accelerate
The Power of Parallel Processing
At their core, GPUs are highly parallel processors designed to handle thousands of concurrent threads efficiently. This architecture makes them well-suited for the computationally intensive tasks involved in training deep learning models, including LLMs. The CUDA platform, developed by NVIDIA, provides a software environment that allows developers to harness the full potential of these GPUs, enabling them to write code that can leverage the parallel processing capabilities of the hardware. Accelerating LLM Training with GPUs and CUDA.
Training large language models is a computationally demanding task that requires processing vast amounts of text data and performing numerous matrix operations. GPUs, with their thousands of cores and high memory bandwidth, are ideally suited for these tasks. By leveraging CUDA, developers can optimize their code to take advantage of the parallel processing capabilities of GPUs, significantly reducing the time required to train LLMs.
For example, the training of GPT-3, one of the largest language models to date, was made possible through the use of thousands of NVIDIA GPUs running CUDA-optimized code. This allowed the model to be trained on an unprecedented amount of data, leading to its impressive performance in natural language tasks.
import torch import torch.nn as nn import torch.optim as optim from transformers import GPT2LMHeadModel, GPT2Tokenizer # Load pre-trained GPT-2 model and tokenizer model = GPT2LMHeadModel.from_pretrained('gpt2') tokenizer = GPT2Tokenizer.from_pretrained('gpt2') # Move model to GPU if available device = torch.device("cuda" if torch.cuda.is_available() else "cpu") model = model.to(device) # Define training data and hyperparameters train_data = [...] # Your training data batch_size = 32 num_epochs = 10 learning_rate = 5e-5 # Define loss function and optimizer criterion = nn.CrossEntropyLoss() optimizer = optim.Adam(model.parameters(), lr=learning_rate) # Training loop for epoch in range(num_epochs): for i in range(0, len(train_data), batch_size): # Prepare input and target sequences inputs, targets = train_data[i:i+batch_size] inputs = tokenizer(inputs, return_tensors="pt", padding=True) inputs = inputs.to(device) targets = targets.to(device) # Forward pass outputs = model(**inputs, labels=targets) loss = outputs.loss # Backward pass and optimization optimizer.zero_grad() loss.backward() optimizer.step() print(f'Epoch epoch+1/num_epochs, Loss: loss.item()')
In this example code snippet, we demonstrate the training of a GPT-2 language model using PyTorch and the CUDA-enabled GPUs. The model is loaded onto the GPU (if available), and the training loop leverages the parallelism of GPUs to perform efficient forward and backward passes, accelerating the training process.
CUDA-Accelerated Libraries for Deep Learning
In addition to the CUDA platform itself, NVIDIA and the open-source community have developed a range of CUDA-accelerated libraries that enable efficient implementation of deep learning models, including LLMs. These libraries provide optimized implementations of common operations, such as matrix multiplications, convolutions, and activation functions, allowing developers to focus on the model architecture and training process rather than low-level optimization.
One such library is cuDNN (CUDA Deep Neural Network library), which provides highly tuned implementations of standard routines used in deep neural networks. By leveraging cuDNN, developers can significantly accelerate the training and inference of their models, achieving performance gains of up to several orders of magnitude compared to CPU-based implementations.
import torch import torch.nn as nn import torch.nn.functional as F from torch.cuda.amp import autocast class ResidualBlock(nn.Module): def __init__(self, in_channels, out_channels, stride=1): super().__init__() self.conv1 = nn.Conv2d(in_channels, out_channels, kernel_size=3, stride=stride, padding=1, bias=False) self.bn1 = nn.BatchNorm2d(out_channels) self.conv2 = nn.Conv2d(out_channels, out_channels, kernel_size=3, stride=1, padding=1, bias=False) self.bn2 = nn.BatchNorm2d(out_channels) self.shortcut = nn.Sequential() if stride != 1 or in_channels != out_channels: self.shortcut = nn.Sequential( nn.Conv2d(in_channels, out_channels, kernel_size=1, stride=stride, bias=False), nn.BatchNorm2d(out_channels)) def forward(self, x): with autocast(): out = F.relu(self.bn1(self.conv1(x))) out = self.bn2(self.conv2(out)) out += self.shortcut(x) out = F.relu(out) return out
In this code snippet, we define a residual block for a convolutional neural network (CNN) using PyTorch. The autocast context manager from PyTorch’s Automatic Mixed Precision (AMP) is used to enable mixed-precision training, which can provide significant performance gains on CUDA-enabled GPUs while maintaining high accuracy. The F.relu function is optimized by cuDNN, ensuring efficient execution on GPUs.
Multi-GPU and Distributed Training for Scalability
As LLMs and deep learning models continue to grow in size and complexity, the computational requirements for training these models also increase. To address this challenge, researchers and developers have turned to multi-GPU and distributed training techniques, which allow them to leverage the combined processing power of multiple GPUs across multiple machines.
CUDA and associated libraries, such as NCCL (NVIDIA Collective Communications Library), provide efficient communication primitives that enable seamless data transfer and synchronization across multiple GPUs, enabling distributed training at an unprecedented scale.
</pre> import torch.distributed as dist from torch.nn.parallel import DistributedDataParallel as DDP # Initialize distributed training dist.init_process_group(backend='nccl', init_method='...') local_rank = dist.get_rank() torch.cuda.set_device(local_rank) # Create model and move to GPU model = MyModel().cuda() # Wrap model with DDP model = DDP(model, device_ids=[local_rank]) # Training loop (distributed) for epoch in range(num_epochs): for data in train_loader: inputs, targets = data inputs = inputs.cuda(non_blocking=True) targets = targets.cuda(non_blocking=True) outputs = model(inputs) loss = criterion(outputs, targets) optimizer.zero_grad() loss.backward() optimizer.step()
In this example, we demonstrate distributed training using PyTorch’s DistributedDataParallel (DDP) module. The model is wrapped in DDP, which automatically handles data parallelism, gradient synchronization, and communication across multiple GPUs using NCCL. This approach enables efficient scaling of the training process across multiple machines, allowing researchers and developers to train larger and more complex models in a reasonable amount of time.
Deploying Deep Learning Models with CUDA
While GPUs and CUDA have primarily been used for training deep learning models, they are also crucial for efficient deployment and inference. As deep learning models become increasingly complex and resource-intensive, GPU acceleration is essential for achieving real-time performance in production environments.
NVIDIA’s TensorRT is a high-performance deep learning inference optimizer and runtime that provides low-latency and high-throughput inference on CUDA-enabled GPUs. TensorRT can optimize and accelerate models trained in frameworks like TensorFlow, PyTorch, and MXNet, enabling efficient deployment on various platforms, from embedded systems to data centers.
import tensorrt as trt # Load pre-trained model model = load_model(...) # Create TensorRT engine logger = trt.Logger(trt.Logger.INFO) builder = trt.Builder(logger) network = builder.create_network() parser = trt.OnnxParser(network, logger) # Parse and optimize model success = parser.parse_from_file(model_path) engine = builder.build_cuda_engine(network) # Run inference on GPU context = engine.create_execution_context() inputs, outputs, bindings, stream = allocate_buffers(engine) # Set input data and run inference set_input_data(inputs, input_data) context.execute_async_v2(bindings=bindings, stream_handle=stream.ptr) # Process output # ...
In this example, we demonstrate the use of TensorRT for deploying a pre-trained deep learning model on a CUDA-enabled GPU. The model is first parsed and optimized by TensorRT, which generates a highly optimized inference engine tailored for the specific model and hardware. This engine can then be used to perform efficient inference on the GPU, leveraging CUDA for accelerated computation.
Conclusion
The combination of GPUs and CUDA has been instrumental in driving the advancements in large language models, computer vision, speech recognition, and various other domains of deep learning. By harnessing the parallel processing capabilities of GPUs and the optimized libraries provided by CUDA, researchers and developers can train and deploy increasingly complex models with high efficiency.
As the field of AI continues to evolve, the importance of GPUs and CUDA will only grow. With even more powerful hardware and software optimizations, we can expect to see further breakthroughs in the development and deployment of AI systems, pushing the boundaries of what is possible.
#ai#AI development#AI research#AI systems#AI Tools 101#amp#applications#approach#apt#architecture#artificial#Artificial Intelligence#benchmarks#BERT#Bias#challenge#clone#CNN#code#Code Snippet#Collective#command#communication#communications#Community#complexity#comprehensive#computation#computer#Computer vision
0 notes
Text
Nvidia HGX vs DGX: Key Differences in AI Supercomputing Solutions

Nvidia HGX vs DGX: What are the differences?
Nvidia is comfortably riding the AI wave. And for at least the next few years, it will likely not be dethroned as the AI hardware market leader. With its extremely popular enterprise solutions powered by the H100 and H200 “Hopper” lineup of GPUs (and now B100 and B200 “Blackwell” GPUs), Nvidia is the go-to manufacturer of high-performance computing (HPC) hardware.
Nvidia DGX is an integrated AI HPC solution targeted toward enterprise customers needing immensely powerful workstation and server solutions for deep learning, generative AI, and data analytics. Nvidia HGX is based on the same underlying GPU technology. However, HGX is a customizable enterprise solution for businesses that want more control and flexibility over their AI HPC systems. But how do these two platforms differ from each other?




Nvidia DGX: The Original Supercomputing Platform
It should surprise no one that Nvidia’s primary focus isn’t on its GeForce lineup of gaming GPUs anymore. Sure, the company enjoys the lion’s share among the best gaming GPUs, but its recent resounding success is driven by enterprise and data center offerings and AI-focused workstation GPUs.
Overview of DGX
The Nvidia DGX platform integrates up to 8 Tensor Core GPUs with Nvidia’s AI software to power accelerated computing and next-gen AI applications. It’s essentially a rack-mount chassis containing 4 or 8 GPUs connected via NVLink, high-end x86 CPUs, and a bunch of Nvidia’s high-speed networking hardware. A single DGX B200 system is capable of 72 petaFLOPS of training and 144 petaFLOPS of inference performance.
Key Features of DGX
AI Software Integration: DGX systems come pre-installed with Nvidia’s AI software stack, making them ready for immediate deployment.
High Performance: With up to 8 Tensor Core GPUs, DGX systems provide top-tier computational power for AI and HPC tasks.
Scalability: Solutions like the DGX SuperPOD integrate multiple DGX systems to form extensive data center configurations.
Current Offerings
The company currently offers both Hopper-based (DGX H100) and Blackwell-based (DGX B200) systems optimized for AI workloads. Customers can go a step further with solutions like the DGX SuperPOD (with DGX GB200 systems) that integrates 36 liquid-cooled Nvidia GB200 Grace Blackwell Superchips, comprised of 36 Nvidia Grace CPUs and 72 Blackwell GPUs. This monstrous setup includes multiple racks connected through Nvidia Quantum InfiniBand, allowing companies to scale thousands of GB200 Superchips.
Legacy and Evolution
Nvidia has been selling DGX systems for quite some time now — from the DGX Server-1 dating back to 2016 to modern DGX B200-based systems. From the Pascal and Volta generations to the Ampere, Hopper, and Blackwell generations, Nvidia’s enterprise HPC business has pioneered numerous innovations and helped in the birth of its customizable platform, Nvidia HGX.
Nvidia HGX: For Businesses That Need More
Build Your Own Supercomputer
For OEMs looking for custom supercomputing solutions, Nvidia HGX offers the same peak performance as its Hopper and Blackwell-based DGX systems but allows OEMs to tweak it as needed. For instance, customers can modify the CPUs, RAM, storage, and networking configuration as they please. Nvidia HGX is actually the baseboard used in the Nvidia DGX system but adheres to Nvidia’s own standard.
Key Features of HGX
Customization: OEMs have the freedom to modify components such as CPUs, RAM, and storage to suit specific requirements.
Flexibility: HGX allows for a modular approach to building AI and HPC solutions, giving enterprises the ability to scale and adapt.
Performance: Nvidia offers HGX in x4 and x8 GPU configurations, with the latest Blackwell-based baseboards only available in the x8 configuration. An HGX B200 system can deliver up to 144 petaFLOPS of performance.
Applications and Use Cases
HGX is designed for enterprises that need high-performance computing solutions but also want the flexibility to customize their systems. It’s ideal for businesses that require scalable AI infrastructure tailored to specific needs, from deep learning and data analytics to large-scale simulations.
Nvidia DGX vs. HGX: Summary
Simplicity vs. Flexibility
While Nvidia DGX represents Nvidia’s line of standardized, unified, and integrated supercomputing solutions, Nvidia HGX unlocks greater customization and flexibility for OEMs to offer more to enterprise customers.
Rapid Deployment vs. Custom Solutions
With Nvidia DGX, the company leans more into cluster solutions that integrate multiple DGX systems into huge and, in the case of the DGX SuperPOD, multi-million-dollar data center solutions. Nvidia HGX, on the other hand, is another way of selling HPC hardware to OEMs at a greater profit margin.
Unified vs. Modular
Nvidia DGX brings rapid deployment and a seamless, hassle-free setup for bigger enterprises. Nvidia HGX provides modular solutions and greater access to the wider industry.
FAQs
What is the primary difference between Nvidia DGX and HGX?
The primary difference lies in customization. DGX offers a standardized, integrated solution ready for deployment, while HGX provides a customizable platform that OEMs can adapt to specific needs.
Which platform is better for rapid deployment?
Nvidia DGX is better suited for rapid deployment as it comes pre-integrated with Nvidia’s AI software stack and requires minimal setup.
Can HGX be used for scalable AI infrastructure?
Yes, Nvidia HGX is designed for scalable AI infrastructure, offering flexibility to customize and expand as per business requirements.
Are DGX and HGX systems compatible with all AI software?
Both DGX and HGX systems are compatible with Nvidia’s AI software stack, which supports a wide range of AI applications and frameworks.
Final Thoughts
Choosing between Nvidia DGX and HGX ultimately depends on your enterprise’s needs. If you require a turnkey solution with rapid deployment, DGX is your go-to. However, if customization and scalability are your top priorities, HGX offers the flexibility to tailor your HPC system to your specific requirements.
Muhammad Hussnain Facebook | Instagram | Twitter | Linkedin | Youtube
0 notes
Text
30.6. CUDA 업데이트
CUDA 업데이트와 관련된 각 단계를 더 구체적으로 살펴보겠습니다. 1. CUDA 업데이트 개요 1.1. CUDA란 무엇인가? CUDA는 NVIDIA가 개발한 병렬 컴퓨팅 플랫폼이자 프로그래밍 모델입니다. 이를 통해 개발자는 GPU를 사용하여 컴퓨팅 작업을 병렬로 수행할 수 있습니다. 이는 특히 과학 계산, 인공지능, 머신러닝, 데이터 분석, 게임 그래픽 등의 분야에서 매우 유용합니다. 2. CUDA 업데이트 절차 2.1. CUDA Toolkit 다운로드 (1) NVIDIA 공식 웹사이트 방문: - (https://developer.nvidia.com/cuda-downloads) 페이지로 이동합니다. (2) 운영체제 및 아키텍처 선택: - Windows, Linux, MacOS 중 사용 중인 운영체제를 선택합니다. - CPU 아키텍처 (예: x86_64, arm64 등)를 선택합니다. (3) CUDA Toolkit 버전 선택: - 사용하려는 CUDA Toolkit 버전을 선택합니다. 최신 버전이 권장되지만, 호환성 문제로 특정 버전을 선택할 수도 있습니다. (4) 설치 파일 다운로드: - 선택한 설정에 맞는 설치 파일을 다운로드합니다. 이 파일은 설치 마법사 또는 패키지 매니저를 통해 설치를 진행할 수 있습니다. 2.2. 설치 (1) 설치 파일 실행: - 다운로드한 설치 파일을 실행합니다. 설치 마법사가 시작되며, 안내에 따라 설치를 진행합니다. (2) CUDA Toolkit 구성 요소 선택: - 기본 설정대로 설치하거나, 설치할 구성 요소 (예: 드라이버, 샘플 코드, 라이브러리 등)를 선택합니다. (3) 드라이버 설치: - 최신 NVIDIA 드라이버가 필요합니다. 설치 과정에서 필요한 드라이버가 포함되어 있지 않은 경우, NVIDIA 드라이버 페이지에서 최신 드라이버를 다운로드하여 설치해야 합니다. 2.3. 환경 변수 설정 (1) 환경 변수 추가 (Linux/MacOS): - CUDA Toolkit의 bin 디렉토리를 PATH 환경 변수에 추가합니다. 예를 들어, `~/.bashrc` 파일에 다음 줄을 추가합니다: export PATH=/usr/local/cuda/bin:$PATH - 라이브러리 경로를 추가합니다: export LD_LIBRARY_PATH=/usr/local/cuda/lib64:$LD_LIBRARY_PATH (2) 환경 변수 추가 (Windows): - 시스템 속성 -> 고급 시스템 설정 -> 환경 변수 -> 시스템 변수에서 `Path`를 선택하고 편집합니다. - 새 항목으로 CUDA bin 디렉토리 (예: `C:Program FilesNVIDIA GPU Computing ToolkitCUDAvX.Xbin`)를 추가합니다. 3. 주요 업데이트 내용 3.1. 새로운 기능 (1) Tensor Cores 지원: - AI 및 딥러닝 작업을 가속화하기 위한 Tensor Cores가 추가됩니다. 이는 행렬 연산을 매우 빠르게 수행할 수 있도록 도와줍니다. (2) CUDA Graphs: - 복잡한 작업 흐름을 최적화하여 성능을 향상시키기 위한 CUDA Graphs 기능이 추가됩니다. 이를 통해 커널 실행 순서 및 동기화를 효율적으로 관리할 수 있습니다. (3) 새로운 라이브러리: - 특정 작업을 최적화하기 위한 새로운 라이브러리가 추가됩니다. 예를 들어, cuSPARSE는 희소 행렬 연산을 가속화하기 위한 라이브러리입니다. 3.2. 성능 향상 (1) 커널 실행 최적화: - 새로운 커널 실행 기술을 통해 GPU 자원을 효율적으로 사용하고, 병렬 실행을 최적화하여 성능을 향상시킵니다. (2) 메모리 관리 개선: - 더 빠른 메모리 할당 및 해제를 통해 메모리 사용의 효율성을 높이고, 전반적인 성능을 개선합니다. 3.3. 버그 수정 (1) 이전 버전의 버그 수정: - 이전 버전에서 발견된 여러 버그가 수정됩니다. 이는 안정성을 높이고, 예기치 않은 오류를 줄이는 데 도움이 됩니다. (2) 안정성 및 호환성 향상: - 새로운 기능 및 기존 기능의 호환성을 개선하여, 다양한 하드웨어 및 소프트웨어 환경에서 더 나은 성능을 제공합니다. 4. 호환성 및 지원 4.1. 하드웨어 호환성 - 최신 CUDA 버전은 최신 NVIDIA GPU를 지원합니다. 특정 GPU 아키텍처 (예: Volta, Turing, Ampere 등)에 대한 최적화가 포함됩니다. - 일부 구형 GPU는 지원되지 않을 수 있으므로, 업데이트 전에 호환성 목록을 확인해야 합니다. 4.2. 소프트웨어 호환성 - 최신 CUDA Toolkit은 최신 운영 체제 버전과 호환됩니다. 이는 주기적으로 운영 체제를 업데이트해야 함을 의미합니다. - 특정 소프트웨어나 라이브러리와의 호환성 문제를 피하기 위해, 개발 환경을 주기적으로 업데이트해야 합니다. 5. 업데이트 시 고려 사항 5.1 백업: - 중요한 데이터나 코드를 업데이트 전에 백업하십시오. 이는 업데이트 중 발생할 수 있는 문제를 예방하기 위함입니다. 5.2 테스트: - 업데이트 후 기존 코드가 정���적으로 작동하는지 테스트하십시오. 새로운 기능을 사용하기 전에 기존 기능의 정상 작동을 확인하는 것이 중요합니다. 5.3 문서 확인: - 새로 추가된 기능이나 변경된 사항에 대해 문서를 꼼꼼히 확인하십시오. (https://docs.nvidia.com/cuda/)를 참고하여 최신 정보와 사용법을 숙지하십시오. 이와 같은 절차를 통해 CUDA 업데이트를 성공적으로 수행할 수 있습니다. 최신 기술과 최적화를 반영한 업데이트를 통해, GPU를 최대한 활용하여 더 나은 성능을 얻을 수 있습니다. Read the full article
0 notes
Link
Ampere afirma que el chip de 256 núcleos se fabricará en el nodo de proceso de 3 nm de última generación de TSMC y ofrecerá un aumento de rendimiento del 40 por ciento en comparación con cualquier otra CPU actualmente en el mercado. La compañía ha diseñado varias características nuevas para un rendimiento eficiente, administración de memoria, almacenamiento en caché y capacidades informáticas de IA.
0 notes
Text
GIGABYTE AmpereOne CPU Servers For Cloud Workloads

Ampereone CPU
Using the AmpereOne family of processors for cloud native workloads, GIGABYTE announces the general availability of its new servers and joins Yotta 2024.
Today, GIGABYTE subsidiary Giga Computing launched its first wave of GIGABYTE servers that support the AmpereOne family of processors’ entire stack. Giga Computing is a pioneer in the industry for servers for x86 and ARM platforms as well as sophisticated cooling technologies.
AmpereOne CPU was revealed last year, and GIGABYTE servers supporting the platform were accessible to a limited number of users. With single and twin socket servers already in production and more planned in late Q4, GIGABYTE servers are now generally available.
From October 7–9, Yotta 2024 in Las Vegas will feature GIGABYTE servers for Ampere Altra and AmpereOne processors at both the GIGABYTE booth and the Ampere pavilion.
Up to 192 specially created Ampere cores, DDR5 memory, and 128 PCIe Gen5 lanes per socket are features of the AmpereOne family of CPUs, which is intended for cloud-native computing. All things considered, this series of processors aims to provide outstanding performance per watt for cloud instances with high virtual machine density. With expanded applications in AI inference, data analytics, and other areas, this whole stack of CPUs offers more cores, IO, memory, performance, and cloud features.
AmpereOne Family Of Processors Servers
R163 Series: General-purpose, single-socket 1U servers
R163-P32: 12x 2.5″ Gen5 NVMe/SATA drives or 12x 2.5″ SATA drives
R163-P30: 4x 3.5″/2.5″ Gen5 NVMe/SATA drives
R263 Series: General-purpose, single-socket 2U servers
R263-P30: 4x 3.5″/2.5″ Gen5 NVMe/SATA and 8x 3.5″/2.5″ SATA drives
E163 Series: Edge, single-socket servers with a depth of 520mm
E163-P30: 2x 2.5″ Gen5 NVMe/SATA drives
R183 Series: General-purpose, dual-socket 1U servers
R183-P90: 4x 3.5″/2.5″ Gen5 NVMe/SATA drives
R183-P92: 12x 2.5″ Gen5 NVMe/SATA drives
R283 Series: General-purpose, dual-socket 2U servers
R283-P90: 12x 3.5″/2.5″ Gen5 NVMe/SATA drives
R283-P92: 12x 2.5″ Gen5 NVMe and 12x 2.5″ Gen5 NVMe/SATA drives
Vincent Wang, vice president of sales at Giga Computing, stated, “GIGABYTE firmly believe that the new product offerings for AmpereOne CPU will meet customer needs because the servers have been tailored to their intended workloads and because they have listened to the customers feedback.” “And the abundance of new SKUs for this high core count processor that meets all cloud native compute needs also reflects this.”
Glenn Keels, vice president of product marketing at Ampere, stated, “With the general availability of AmpereOne CPU servers at GIGABYTE, It is expanding portfolio of products to offer even more compute density per rack at unmatched energy efficiency.” “AMPERE Altra and AmpereOne product families offer servers with a broad range of core counts, giving GIGABYTE customers a broad portfolio of Ampere-based products to meet all of their Cloud Native and AI Compute needs.”
In summary
The AmpereOne CPU Servers from GIGABYTE are engineered to manage demanding cloud workloads with exceptional performance, scalability, and energy economy. Driven by AmpereOne processors, they meet the increasing needs of data centers and cloud-native applications. These servers offer cost-effective operations and power efficiency due to their multi-core processing optimization, which guarantees strong support for workloads involving AI, machine learning, and parallel processing.
Read more on Govindhtech.com
#GIGABYTEAmpereOne#AmpereOne#AmpereOneCPUServers#CPUServers#CloudWorkloads#GIGABYTEservers#GigaComputing#AmpereAltra#News#Technews#Technology#Technologynews#Technologytrends#Govindhtech
0 notes
Text
Linus Torvalds Ampere Arm işlemcili bilgisayar kullanıyor

Linux çekirdeği yaratıcısı Linus Torvalds yakın zamanda Arm64 Linux'u test etme konusunda sistemini geliştirdi. Daha önce Arm64 Linux'u Apple Silicon MacBook Air üzerinde geliştiriyordu, ancak şimdi daha güçlü Ampere AArch64 sistemi sayesinde daha da fazla Arm64 testi yapıyor. Sadece Linux çekirdeğini değil Git'i de yaratmasıyla tanınan Torvalds, yıllarca yalnızca Intel donanımı üzerinde çalıştı. Daha sonra ana sistemi için AMD Ryzen Threadripper iş istasyonuna geçti . MacBook'unu eline aldığında onu düzenli olarak yeni Arm64 çekirdek yapılarını derlemek için kullandı. Linux çekirdeği 5.19'dan başlayarak Torvalds, Arm64 yapılarını Apple'ın M2 Çip Üzerinde Sistem (SoC) ile 2022 MacBook Air üzerinde derliyordu. Ancak bu model sadece 8 CPU çekirdeği içeriyor. Linux 5.19 piyasaya sürüldüğünde Torvalds, "bir sonraki seyahatimde bununla bir dizüstü bilgisayar olarak seyahat edebileceğimden ve sonunda Arm64 tarafının test sürümünü de kullanabileceğimden emin olmaya çalıştığını" yazdı. Geliştiricinin artık yüksek sayıda Armv8 çekirdeğine sahip bir Ampere iş istasyonu/sunucusu var. Torvalds hangi Ampere sistemine sahip olduğunu belirtmiyor ancak bazıları bunun muhtemelen Ampere Altra ailesinin bir çeşidi olduğuna inanıyor . Evet, AmpereOne daha fazla CPU çekirdeğini destekliyor ancak Altra iş istasyonları daha kolay temin edilebilir. 128 çekirdeğe kadar destek sunan Ampere Altra, ağır Arm64 Linux testleri için kesinlikle yeterince sağlam. Torvalds, ileride pek çok Arm64 Linux yapısı yapmaya devam edeceğini umduğunu açıkladı. Halen M2 MacBook'u kullanıyor ancak bunun günlük bir sürücüden ziyade haftalık test derlemeleri için olduğunu açıkladı. Günlük sürücü muhtemelen sürüm notlarında bahsettiği Ampere sistemidir. kaynak:https://www.tomshardware.com/software/linux/linus-torvalds-now-favors-arm-powered-ampere-chip-over-apple-silicon-mac-for-building-linux-kernels-says-the-more-powerful-system-is-why-hes-doing-more-arm64-linux-testing Read the full article
0 notes
Text
Intel, Ampere show running LLMs on CPUs isn't as crazy as it sounds
http://securitytc.com/T6GKyD
0 notes
Photo

La empresa de chips fundada por el ex presidente de Intel planea una CPU masiva de 256 núcleos para navegar la ola de inferencia de IA y darle a Nvidia B100 una oportunidad por su dinero: Ampere Computing AmpereOne-3 probablemente sea compatible con la tecnología PCIe 6.0 y DDR5 Ampere Computing presentó su famil... https://ujjina.com/la-empresa-de-chips-fundada-por-el-ex-presidente-de-intel-planea-una-cpu-masiva-de-256-nucleos-para-navegar-la-ola-de-inferencia-de-ia-y-darle-a-nvidia-b100-una-oportunidad-por-su-dinero-ampere-compu/?feed_id=606985&_unique_id=662ca8999d16c
0 notes
Text
MSI DESKTOP MS-B915
Experience the pinnacle of gaming performance with MSI desktop MS-B915. With its contemporary look and traces of customizable electric circuitry, the Gaming CPU is the perfect choice for gamers. Furthermore, this gaming CPU's RTX GPU operates quickly and with less power consumption. In addition, the NVIDIA Ampere architecture makes use of several processors to produce a realistic gaming experience.
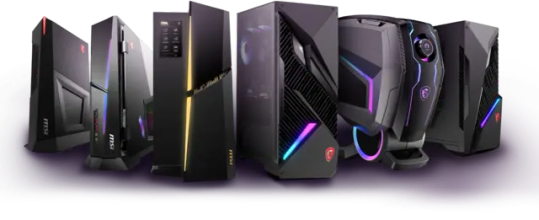
. Adaptable Style
. Effective Operation
. Processing Unit for Graphics
. Silent Storm Cooling 2
. Convenient Upgrading
. Mystic Light Customisation
1 note
·
View note