#How To Visualize Data Using Tableau
Explore tagged Tumblr posts
Text
DATA VISUALIZATION USING TABLEAU | USING TABLEAU TO VISUALIZE DATA | VISUALIZATION USING TABLEAU | TABLEAU FOR BEGINNERS DATA VISUALISATION | HOW TO VISUALIZE DATA USING TABLEAU | DATA VISUALIZATION USING TABLEAU TUTORIAL | TABLEAU VISUALISATION | DATA VISUALISATION WITH TABLEAU
Data Visualization Using Tableau,Using Tableau To Visualize Data,Visualization Using Tableau,Tableau For Beginners Data Visualisation,How To Visualize Data Using Tableau,Data Visualization Using Tableau Tutorial,Tableau Visualisation,Data Visualisation With Tableau
Visit : https://cognitec.in/course/data-visualization-using-tableau-40-hrs
#Data Visualization Using Tableau#Using Tableau To Visualize Data#Visualization Using Tableau#Tableau For Beginners Data Visualisation#How To Visualize Data Using Tableau#Data Visualization Using Tableau Tutorial#Tableau Visualisation#Data Visualisation With Tableau
0 notes
Text
How to Visualize Data using Tableau
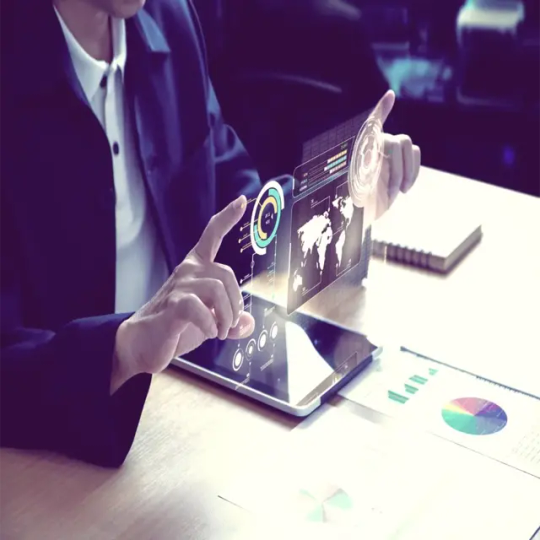
Visualizing data using Tableau is a straightforward process, thanks to its user-friendly interface and powerful visualization capabilities. Here's a step-by-step guide on how to visualize data using Tableau:
Connect to Your Data Source
Launch Tableau Desktop.
Click on "Connect to Data" to select your data source. Tableau supports various data sources, including databases, spreadsheets, cloud services, and more.
Choose the data source type and provide the necessary connection details.
Import or Load Data
After connecting to your data source, you can either import the data into Tableau as an extract or use a live connection, depending on your preference and performance requirements.
Select the specific tables or sheets you want to work with and load the data into Tableau.
Create a New Worksheet
Once your data is loaded, you'll be directed to a new worksheet in Tableau.
Choose the Visualization Type
In Tableau, you can create various types of visualizations, such as bar charts, line charts, scatter plots, maps, and more.
To choose a visualization type, drag and drop a dimension and a measure onto the Columns and Rows shelves.
Tableau will automatically recommend visualization options based on your data, or you can select a specific visualization type from the "Show Me" menu.
Customize Your Visualization
After selecting a visualization type, you can customize it using the Marks card on the left side of the screen.
Adjust colors, labels, formatting, and other settings to tailor the visualization to your needs.
Add Filters and Parameters
To enhance interactivity, you can add filters and parameters to your visualization. Drag dimensions to the Filters shelf to create filter controls that allow users to interactively refine the data displayed.
Parameters provide dynamic control over aspects of the visualization, such as selecting a specific measure or date range.
Create Calculations
Tableau allows you to create calculated fields to perform custom calculations on your data. Use the calculation editor to define expressions and create new fields.
Build Dashboards
Combine multiple visualizations into interactive dashboards. Click on the "Dashboard" tab to create a new dashboard, and then drag and drop sheets onto the dashboard canvas.
Arrange and format elements to create a cohesive and informative dashboard.
0 notes
Text
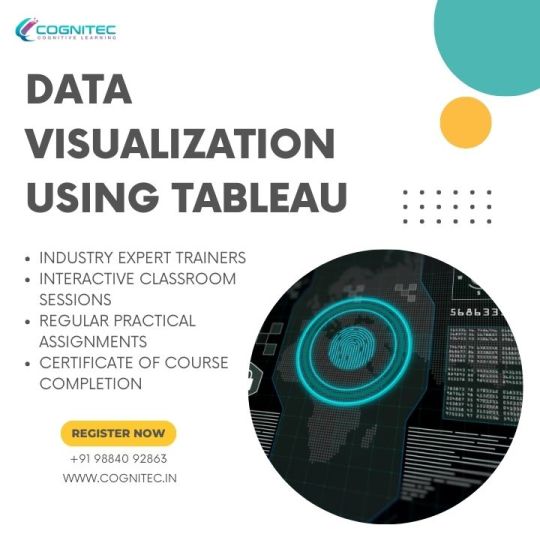
Data Visualization Using Tableau,Using Tableau To Visualize Data,Visualization Using Tableau,Tableau For Beginners Data Visualisation,How To Visualize Data Using Tableau,Data Visualization Using Tableau Tutorial,Tableau Visualisation,Data Visualisation With Tableau
#Data Visualization Using Tableau#Using Tableau To Visualize Data#Visualization Using Tableau#Tableau For Beginners Data Visualisation#How To Visualize Data Using Tableau#Data Visualization Using Tableau Tutorial#Tableau Visualisation#Data Visualisation With Tableau
0 notes
Text
youtube
Discover how the world’s top companies are leveraging Business Intelligence (BI) to stay ahead of the competition! In this video, we break down the strategies and tools used by giants like Google, Amazon, Apple, and more to optimize operations, enhance customer experience, and drive innovation. From real-time data analysis to predictive analytics, these companies are transforming the way business is done.
Whether you’re a business owner, a data enthusiast, or just curious about how big brands like Netflix and Tesla use BI to gain a competitive edge, this video is a must-watch. Learn how Business Intelligence tools like Tableau, Microsoft Power BI, and SAP BusinessObjects are being used to make smarter decisions, predict customer behavior, and streamline operations.
Visit Our Webiste: https://vuelitics.com/
#businessintelligence#data analytics#businessstrategy#data strategy#data visualization#business analytics#advance data solution#howcompanyusebi#datainsights#business analysis techniques#top artificial intelligence companies#Business Intelligence#BI tools#predictive analytics#top companies using BI#Google BI strategy#Amazon BI tools#Microsoft Power BI#SAP BusinessObjects#Tableau#Netflix data analytics#how companies use BI#business intelligence strategies#real-time data analysis#supply chain optimization#customer experience enhancement#data-driven decision making.#business analyst#microsoft 365#microsoft power bi
0 notes
Text
grow with me
flow with me
tableau with me
grow with me
flow with me
adapt with me
#it’s a dataviz joke#dataviz#data visualization#tableau#I���d rather use ggplot2#ggplot2#or excel#excel#microsoft excel#Microsoft excel is underrated but it’s actually very powerful#poetry#poem#the original was supposed to be about love#love#love is an action#love poem#prose#it rhymed ok?#rhyming#words#look i’m trying here#i’m gonna make multiple drafts on here#if someone said this to me I would consider it flirting#flirting#romance#this is how you romance me
8 notes
·
View notes
Text
lol just gonna vent about work for a second:
i'm realizing why (aside from the bullshit accommodation situation) i have been feeling so demoralized at work lately. our newest team member is about 8 months in now, so he is taking on more and more responsibilities, which includes data visualization bc he knows tableau. blah blah blah, i won't go into the details of what's gone on the last two months but i had a very frustrating experience with a project i was working with him on.
anyway, what's bugging me is this: this huge initiative that we compile/analyze/report the data for has been central for my entire time in this role; when i got here, we had hardly any data. i was central to compiling basically all of it, providing descriptive analytics and some basic visualizations (so. many. excel. charts.) there's not many people on my team, so truly, i think it's fair to say i have the most thorough understanding of this data, not just in terms of what it represents for this initiative, but also what it takes to compile it.
so it frustrates me for someone to come in who has significant experience with data analysis tools but less experience (seemingly) with like, being in the trenches with data. i don't know how else to explain it, but like, we're talking merging, compiling, analyzing and visualizing data all with excel! versus running code on a dataset that you were just given & not actually spending a lot of time in the data. (this is how a bunch of errors almost ended up in a pretty big presentation!)
also, on a related note, i am frustrated with my position because i do have to spend so much time mired in data, i don't have a whole lot of time to learn and implement new skills, but i have all of this analytic understanding courtesy of my two soc degrees that i never get to use! it's not about not liking what i do, it's just feeling like i'm slightly being pushed out of things i was central to building and simultaneously feeling like i'm lowest on the totem pole.
and i'm also like, slightly jaded in this weird backwards way because i don't understand why i was promoted in the context of all this lmao. it sucks to feel like i need more education to be able to advance in my field because the only skills i'm developing rn are with antiquated tools.
9 notes
·
View notes
Text
What Are the Qualifications for a Data Scientist?
In today's data-driven world, the role of a data scientist has become one of the most coveted career paths. With businesses relying on data for decision-making, understanding customer behavior, and improving products, the demand for skilled professionals who can analyze, interpret, and extract value from data is at an all-time high. If you're wondering what qualifications are needed to become a successful data scientist, how DataCouncil can help you get there, and why a data science course in Pune is a great option, this blog has the answers.
The Key Qualifications for a Data Scientist
To succeed as a data scientist, a mix of technical skills, education, and hands-on experience is essential. Here are the core qualifications required:
1. Educational Background
A strong foundation in mathematics, statistics, or computer science is typically expected. Most data scientists hold at least a bachelor’s degree in one of these fields, with many pursuing higher education such as a master's or a Ph.D. A data science course in Pune with DataCouncil can bridge this gap, offering the academic and practical knowledge required for a strong start in the industry.
2. Proficiency in Programming Languages
Programming is at the heart of data science. You need to be comfortable with languages like Python, R, and SQL, which are widely used for data analysis, machine learning, and database management. A comprehensive data science course in Pune will teach these programming skills from scratch, ensuring you become proficient in coding for data science tasks.
3. Understanding of Machine Learning
Data scientists must have a solid grasp of machine learning techniques and algorithms such as regression, clustering, and decision trees. By enrolling in a DataCouncil course, you'll learn how to implement machine learning models to analyze data and make predictions, an essential qualification for landing a data science job.
4. Data Wrangling Skills
Raw data is often messy and unstructured, and a good data scientist needs to be adept at cleaning and processing data before it can be analyzed. DataCouncil's data science course in Pune includes practical training in tools like Pandas and Numpy for effective data wrangling, helping you develop a strong skill set in this critical area.
5. Statistical Knowledge
Statistical analysis forms the backbone of data science. Knowledge of probability, hypothesis testing, and statistical modeling allows data scientists to draw meaningful insights from data. A structured data science course in Pune offers the theoretical and practical aspects of statistics required to excel.
6. Communication and Data Visualization Skills
Being able to explain your findings in a clear and concise manner is crucial. Data scientists often need to communicate with non-technical stakeholders, making tools like Tableau, Power BI, and Matplotlib essential for creating insightful visualizations. DataCouncil’s data science course in Pune includes modules on data visualization, which can help you present data in a way that’s easy to understand.
7. Domain Knowledge
Apart from technical skills, understanding the industry you work in is a major asset. Whether it’s healthcare, finance, or e-commerce, knowing how data applies within your industry will set you apart from the competition. DataCouncil's data science course in Pune is designed to offer case studies from multiple industries, helping students gain domain-specific insights.
Why Choose DataCouncil for a Data Science Course in Pune?
If you're looking to build a successful career as a data scientist, enrolling in a data science course in Pune with DataCouncil can be your first step toward reaching your goals. Here’s why DataCouncil is the ideal choice:
Comprehensive Curriculum: The course covers everything from the basics of data science to advanced machine learning techniques.
Hands-On Projects: You'll work on real-world projects that mimic the challenges faced by data scientists in various industries.
Experienced Faculty: Learn from industry professionals who have years of experience in data science and analytics.
100% Placement Support: DataCouncil provides job assistance to help you land a data science job in Pune or anywhere else, making it a great investment in your future.
Flexible Learning Options: With both weekday and weekend batches, DataCouncil ensures that you can learn at your own pace without compromising your current commitments.
Conclusion
Becoming a data scientist requires a combination of technical expertise, analytical skills, and industry knowledge. By enrolling in a data science course in Pune with DataCouncil, you can gain all the qualifications you need to thrive in this exciting field. Whether you're a fresher looking to start your career or a professional wanting to upskill, this course will equip you with the knowledge, skills, and practical experience to succeed as a data scientist.
Explore DataCouncil’s offerings today and take the first step toward unlocking a rewarding career in data science! Looking for the best data science course in Pune? DataCouncil offers comprehensive data science classes in Pune, designed to equip you with the skills to excel in this booming field. Our data science course in Pune covers everything from data analysis to machine learning, with competitive data science course fees in Pune. We provide job-oriented programs, making us the best institute for data science in Pune with placement support. Explore online data science training in Pune and take your career to new heights!
#In today's data-driven world#the role of a data scientist has become one of the most coveted career paths. With businesses relying on data for decision-making#understanding customer behavior#and improving products#the demand for skilled professionals who can analyze#interpret#and extract value from data is at an all-time high. If you're wondering what qualifications are needed to become a successful data scientis#how DataCouncil can help you get there#and why a data science course in Pune is a great option#this blog has the answers.#The Key Qualifications for a Data Scientist#To succeed as a data scientist#a mix of technical skills#education#and hands-on experience is essential. Here are the core qualifications required:#1. Educational Background#A strong foundation in mathematics#statistics#or computer science is typically expected. Most data scientists hold at least a bachelor’s degree in one of these fields#with many pursuing higher education such as a master's or a Ph.D. A data science course in Pune with DataCouncil can bridge this gap#offering the academic and practical knowledge required for a strong start in the industry.#2. Proficiency in Programming Languages#Programming is at the heart of data science. You need to be comfortable with languages like Python#R#and SQL#which are widely used for data analysis#machine learning#and database management. A comprehensive data science course in Pune will teach these programming skills from scratch#ensuring you become proficient in coding for data science tasks.#3. Understanding of Machine Learning
3 notes
·
View notes
Text
The Skills I Acquired on My Path to Becoming a Data Scientist
Data science has emerged as one of the most sought-after fields in recent years, and my journey into this exciting discipline has been nothing short of transformative. As someone with a deep curiosity for extracting insights from data, I was naturally drawn to the world of data science. In this blog post, I will share the skills I acquired on my path to becoming a data scientist, highlighting the importance of a diverse skill set in this field.
The Foundation — Mathematics and Statistics
At the core of data science lies a strong foundation in mathematics and statistics. Concepts such as probability, linear algebra, and statistical inference form the building blocks of data analysis and modeling. Understanding these principles is crucial for making informed decisions and drawing meaningful conclusions from data. Throughout my learning journey, I immersed myself in these mathematical concepts, applying them to real-world problems and honing my analytical skills.
Programming Proficiency
Proficiency in programming languages like Python or R is indispensable for a data scientist. These languages provide the tools and frameworks necessary for data manipulation, analysis, and modeling. I embarked on a journey to learn these languages, starting with the basics and gradually advancing to more complex concepts. Writing efficient and elegant code became second nature to me, enabling me to tackle large datasets and build sophisticated models.
Data Handling and Preprocessing
Working with real-world data is often messy and requires careful handling and preprocessing. This involves techniques such as data cleaning, transformation, and feature engineering. I gained valuable experience in navigating the intricacies of data preprocessing, learning how to deal with missing values, outliers, and inconsistent data formats. These skills allowed me to extract valuable insights from raw data and lay the groundwork for subsequent analysis.
Data Visualization and Communication
Data visualization plays a pivotal role in conveying insights to stakeholders and decision-makers. I realized the power of effective visualizations in telling compelling stories and making complex information accessible. I explored various tools and libraries, such as Matplotlib and Tableau, to create visually appealing and informative visualizations. Sharing these visualizations with others enhanced my ability to communicate data-driven insights effectively.
Machine Learning and Predictive Modeling
Machine learning is a cornerstone of data science, enabling us to build predictive models and make data-driven predictions. I delved into the realm of supervised and unsupervised learning, exploring algorithms such as linear regression, decision trees, and clustering techniques. Through hands-on projects, I gained practical experience in building models, fine-tuning their parameters, and evaluating their performance.
Database Management and SQL
Data science often involves working with large datasets stored in databases. Understanding database management and SQL (Structured Query Language) is essential for extracting valuable information from these repositories. I embarked on a journey to learn SQL, mastering the art of querying databases, joining tables, and aggregating data. These skills allowed me to harness the power of databases and efficiently retrieve the data required for analysis.
Domain Knowledge and Specialization
While technical skills are crucial, domain knowledge adds a unique dimension to data science projects. By specializing in specific industries or domains, data scientists can better understand the context and nuances of the problems they are solving. I explored various domains and acquired specialized knowledge, whether it be healthcare, finance, or marketing. This expertise complemented my technical skills, enabling me to provide insights that were not only data-driven but also tailored to the specific industry.
Soft Skills — Communication and Problem-Solving
In addition to technical skills, soft skills play a vital role in the success of a data scientist. Effective communication allows us to articulate complex ideas and findings to non-technical stakeholders, bridging the gap between data science and business. Problem-solving skills help us navigate challenges and find innovative solutions in a rapidly evolving field. Throughout my journey, I honed these skills, collaborating with teams, presenting findings, and adapting my approach to different audiences.
Continuous Learning and Adaptation
Data science is a field that is constantly evolving, with new tools, technologies, and trends emerging regularly. To stay at the forefront of this ever-changing landscape, continuous learning is essential. I dedicated myself to staying updated by following industry blogs, attending conferences, and participating in courses. This commitment to lifelong learning allowed me to adapt to new challenges, acquire new skills, and remain competitive in the field.
In conclusion, the journey to becoming a data scientist is an exciting and dynamic one, requiring a diverse set of skills. From mathematics and programming to data handling and communication, each skill plays a crucial role in unlocking the potential of data. Aspiring data scientists should embrace this multidimensional nature of the field and embark on their own learning journey. If you want to learn more about Data science, I highly recommend that you contact ACTE Technologies because they offer Data Science courses and job placement opportunities. Experienced teachers can help you learn better. You can find these services both online and offline. Take things step by step and consider enrolling in a course if you’re interested. By acquiring these skills and continuously adapting to new developments, they can make a meaningful impact in the world of data science.
#data science#data visualization#education#information#technology#machine learning#database#sql#predictive analytics#r programming#python#big data#statistics
14 notes
·
View notes
Text
How to write a paper with ai
mbrace the Future of Research: The Advantages of Using AI Websites for Writing Academic Papers
The landscape of academic writing is evolving with the incorporation of artificial intelligence (AI). AI-powered websites have become a valuable asset in the arsenal of students, researchers, and academics. I highlight the benefits of using AI websites for writing papers and provide recommendations for those looking to optimize their writing process.
Why Use AI Websites for Academic Writing?
1. Efficiency in Research: Tools like Google Scholar and arXiv provide AI-enhanced search functionalities enabling you to quickly find relevant and credible academic sources, thereby accelerating the research process.
2. Streamlined Writing Process: AI writing assistants, such as Jasper AI, offer to help you compose text based on provided prompts or outlines. They can assist in creating drafts more rapidly than traditional methods.
3. Enhanced Organization: Note-taking and outlining AI tools like Evernote or Notion AI can categorize your research, create sophisticated outlines, and keep all your ideas and references neatly organized.
4. High-Quality Drafts: AI websites such as WriteGo.ai generate comprehensive essay drafts, including complex financial analysis and data interpretation, which can significantly improve the initial quality of your paper.
5. Advanced Editing Assistance: Editing platforms like Grammarly use AI to detect grammatical errors, suggest style improvements, and ensure your paper reads naturally and adheres to professional writing standards.
6. Plagiarism Detection: AI-based tools like Turnitin and Copyscape scan your document against a vast database to check for originality and prevent any instances of plagiarism.
7. Data Analysis and Visualization: AI-driven data tools like Tableau can sift through and visualize large datasets, which is particularly beneficial for data-intensive disciplines like finance and sciences.
Recommendations for AI Websites:
Here are some of the top AI websites I recommend for writing academic papers:
Jasper AI for generating written content.
Evernote or Notion AI for organizing your research and notes.
Grammarly or ProWritingAid for editing and refining drafts.
Google Scholar for conducting an AI-enhanced literature search.
Turnitin for plagiarism checks.
Conclusion:
Using AI to assist in writing academic papers is an innovative approach that combines cutting-edge technology with scholarly rigor. The fusion of AI with your own analytical skills can vastly improve the quality of your work, making the process more efficient and leading to higher caliber research outputs.
Whether you are writing a comprehensive review, an empirical paper, or a thesis, AI websites have the potential to complement your intellect and to push the boundaries of what you can achieve in the academic realm. As we move further into the digital era, embracing these tools can help maintain a competitive edge and ensure your academic writing is as impactful and effective as possible.
writego
2 notes
·
View notes
Text
Cracking the Code: A Beginner's Roadmap to Mastering Data Science
Embarking on the journey into data science as a complete novice is an exciting venture. While the world of data science may seem daunting at first, breaking down the learning process into manageable steps can make the endeavor both enjoyable and rewarding. Choosing the best Data Science Institute can further accelerate your journey into this thriving industry.
In this comprehensive guide, we'll outline a roadmap for beginners to get started with data science, from understanding the basics to building a portfolio of projects.
1. Understanding the Basics: Laying the Foundation
The journey begins with a solid understanding of the fundamentals of data science. Start by familiarizing yourself with key concepts such as data types, variables, and basic statistics. Platforms like Khan Academy, Coursera, and edX offer introductory courses in statistics and data science, providing a solid foundation for your learning journey.
2. Learn Programming Languages: The Language of Data Science
Programming is a crucial skill in data science, and Python is one of the most widely used languages in the field. Platforms like Codecademy, DataCamp, and freeCodeCamp offer interactive lessons and projects to help beginners get hands-on experience with Python. Additionally, learning R, another popular language in data science, can broaden your skill set.
3. Explore Data Visualization: Bringing Data to Life
Data visualization is a powerful tool for understanding and communicating data. Explore tools like Tableau for creating interactive visualizations or dive into Python libraries like Matplotlib and Seaborn. Understanding how to present data visually enhances your ability to derive insights and convey information effectively.
4. Master Data Manipulation: Unlocking Data's Potential
Data manipulation is a fundamental aspect of data science. Learn how to manipulate and analyze data using libraries like Pandas in Python. The official Pandas website provides tutorials and documentation to guide you through the basics of data manipulation, a skill that is essential for any data scientist.
5. Delve into Machine Learning Basics: The Heart of Data Science
Machine learning is a core component of data science. Start exploring the fundamentals of machine learning on platforms like Kaggle, which offers beginner-friendly datasets and competitions. Participating in Kaggle competitions allows you to apply your knowledge, learn from others, and gain practical experience in machine learning.
6. Take Online Courses: Structured Learning Paths
Enroll in online courses that provide structured learning paths in data science. Platforms like Coursera (e.g., "Data Science and Machine Learning Bootcamp with R" or "Applied Data Science with Python") and edX (e.g., "Harvard's Data Science Professional Certificate") offer comprehensive courses taught by experts in the field.
7. Read Books and Blogs: Supplementing Your Knowledge
Books and blogs can provide additional insights and practical tips. "Python for Data Analysis" by Wes McKinney is a highly recommended book, and blogs like Towards Data Science on Medium offer a wealth of articles covering various data science topics. These resources can deepen your understanding and offer different perspectives on the subject.
8. Join Online Communities: Learning Through Connection
Engage with the data science community by joining online platforms like Stack Overflow, Reddit (e.g., r/datascience), and LinkedIn. Participate in discussions, ask questions, and learn from the experiences of others. Being part of a community provides valuable support and insights.
9. Work on Real Projects: Applying Your Skills
Apply your skills by working on real-world projects. Identify a problem or area of interest, find a dataset, and start working on analysis and predictions. Whether it's predicting housing prices, analyzing social media sentiment, or exploring healthcare data, hands-on projects are crucial for developing practical skills.
10. Attend Webinars and Conferences: Staying Updated
Stay updated on the latest trends and advancements in data science by attending webinars and conferences. Platforms like Data Science Central and conferences like the Data Science Conference provide opportunities to learn from experts, discover new technologies, and connect with the wider data science community.
11. Build a Portfolio: Showcasing Your Journey
Create a portfolio showcasing your projects and skills. This can be a GitHub repository or a personal website where you document and present your work. A portfolio is a powerful tool for demonstrating your capabilities to potential employers and collaborators.
12. Practice Regularly: The Path to Mastery
Consistent practice is key to mastering data science. Dedicate regular time to coding, explore new datasets, and challenge yourself with increasingly complex projects. As you progress, you'll find that your skills evolve, and you become more confident in tackling advanced data science challenges.
Embarking on the path of data science as a beginner may seem like a formidable task, but with the right resources and a structured approach, it becomes an exciting and achievable endeavor. From understanding the basics to building a portfolio of real-world projects, each step contributes to your growth as a data scientist. Embrace the learning process, stay curious, and celebrate the milestones along the way. The world of data science is vast and dynamic, and your journey is just beginning. Choosing the best Data Science courses in Chennai is a crucial step in acquiring the necessary expertise for a successful career in the evolving landscape of data science.
3 notes
·
View notes
Text
Data Engineering Concepts, Tools, and Projects
All the associations in the world have large amounts of data. If not worked upon and anatomized, this data does not amount to anything. Data masterminds are the ones. who make this data pure for consideration. Data Engineering can nominate the process of developing, operating, and maintaining software systems that collect, dissect, and store the association’s data. In modern data analytics, data masterminds produce data channels, which are the structure armature.
How to become a data engineer:
While there is no specific degree requirement for data engineering, a bachelor's or master's degree in computer science, software engineering, information systems, or a related field can provide a solid foundation. Courses in databases, programming, data structures, algorithms, and statistics are particularly beneficial. Data engineers should have strong programming skills. Focus on languages commonly used in data engineering, such as Python, SQL, and Scala. Learn the basics of data manipulation, scripting, and querying databases.
Familiarize yourself with various database systems like MySQL, PostgreSQL, and NoSQL databases such as MongoDB or Apache Cassandra.Knowledge of data warehousing concepts, including schema design, indexing, and optimization techniques.
Data engineering tools recommendations:
Data Engineering makes sure to use a variety of languages and tools to negotiate its objects. These tools allow data masterminds to apply tasks like creating channels and algorithms in a much easier as well as effective manner.
1. Amazon Redshift: A widely used cloud data warehouse built by Amazon, Redshift is the go-to choice for many teams and businesses. It is a comprehensive tool that enables the setup and scaling of data warehouses, making it incredibly easy to use.
One of the most popular tools used for businesses purpose is Amazon Redshift, which provides a powerful platform for managing large amounts of data. It allows users to quickly analyze complex datasets, build models that can be used for predictive analytics, and create visualizations that make it easier to interpret results. With its scalability and flexibility, Amazon Redshift has become one of the go-to solutions when it comes to data engineering tasks.
2. Big Query: Just like Redshift, Big Query is a cloud data warehouse fully managed by Google. It's especially favored by companies that have experience with the Google Cloud Platform. BigQuery not only can scale but also has robust machine learning features that make data analysis much easier. 3. Tableau: A powerful BI tool, Tableau is the second most popular one from our survey. It helps extract and gather data stored in multiple locations and comes with an intuitive drag-and-drop interface. Tableau makes data across departments readily available for data engineers and managers to create useful dashboards. 4. Looker: An essential BI software, Looker helps visualize data more effectively. Unlike traditional BI tools, Looker has developed a LookML layer, which is a language for explaining data, aggregates, calculations, and relationships in a SQL database. A spectacle is a newly-released tool that assists in deploying the LookML layer, ensuring non-technical personnel have a much simpler time when utilizing company data.
5. Apache Spark: An open-source unified analytics engine, Apache Spark is excellent for processing large data sets. It also offers great distribution and runs easily alongside other distributed computing programs, making it essential for data mining and machine learning. 6. Airflow: With Airflow, programming, and scheduling can be done quickly and accurately, and users can keep an eye on it through the built-in UI. It is the most used workflow solution, as 25% of data teams reported using it. 7. Apache Hive: Another data warehouse project on Apache Hadoop, Hive simplifies data queries and analysis with its SQL-like interface. This language enables MapReduce tasks to be executed on Hadoop and is mainly used for data summarization, analysis, and query. 8. Segment: An efficient and comprehensive tool, Segment assists in collecting and using data from digital properties. It transforms, sends, and archives customer data, and also makes the entire process much more manageable. 9. Snowflake: This cloud data warehouse has become very popular lately due to its capabilities in storing and computing data. Snowflake’s unique shared data architecture allows for a wide range of applications, making it an ideal choice for large-scale data storage, data engineering, and data science. 10. DBT: A command-line tool that uses SQL to transform data, DBT is the perfect choice for data engineers and analysts. DBT streamlines the entire transformation process and is highly praised by many data engineers.
Data Engineering Projects:
Data engineering is an important process for businesses to understand and utilize to gain insights from their data. It involves designing, constructing, maintaining, and troubleshooting databases to ensure they are running optimally. There are many tools available for data engineers to use in their work such as My SQL, SQL server, oracle RDBMS, Open Refine, TRIFACTA, Data Ladder, Keras, Watson, TensorFlow, etc. Each tool has its strengths and weaknesses so it’s important to research each one thoroughly before making recommendations about which ones should be used for specific tasks or projects.
Smart IoT Infrastructure:
As the IoT continues to develop, the measure of data consumed with high haste is growing at an intimidating rate. It creates challenges for companies regarding storehouses, analysis, and visualization.
Data Ingestion:
Data ingestion is moving data from one or further sources to a target point for further preparation and analysis. This target point is generally a data storehouse, a unique database designed for effective reporting.
Data Quality and Testing:
Understand the importance of data quality and testing in data engineering projects. Learn about techniques and tools to ensure data accuracy and consistency.
Streaming Data:
Familiarize yourself with real-time data processing and streaming frameworks like Apache Kafka and Apache Flink. Develop your problem-solving skills through practical exercises and challenges.
Conclusion:
Data engineers are using these tools for building data systems. My SQL, SQL server and Oracle RDBMS involve collecting, storing, managing, transforming, and analyzing large amounts of data to gain insights. Data engineers are responsible for designing efficient solutions that can handle high volumes of data while ensuring accuracy and reliability. They use a variety of technologies including databases, programming languages, machine learning algorithms, and more to create powerful applications that help businesses make better decisions based on their collected data.
2 notes
·
View notes
Text
Top Skills Data Analytics Recruiters Seek in Resumes
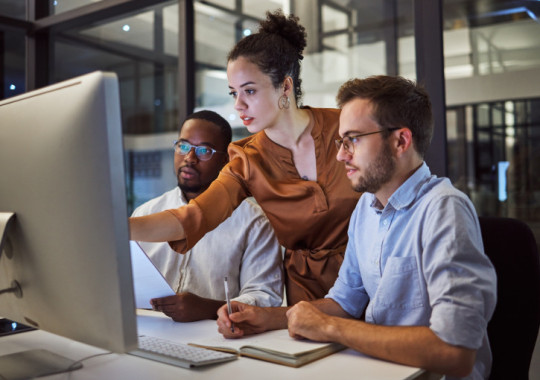
In today's data-driven world, data analytics has become an essential function for organizations across various industries. As companies increasingly rely on data to drive decision-making and gain a competitive edge, the demand for skilled data analysts continues to grow. For those seeking roles in this field, presenting the right skills on your resume is critical. Data analytics recruitment consultants in Dubai often look for a combination of technical proficiency, analytical thinking, and communication abilities that align with a company's strategic goals.
Proficiency in Data Analysis Tools and Software: One of the most sought-after skills in data analytics is proficiency in industry-standard tools and software. Recruiters look for candidates with hands-on experience in platforms like Excel, Tableau, Power BI, and Google Analytics. Knowledge of SQL, R, Python, and SAS is also highly desirable for more advanced roles. These tools are the foundation of data collection, analysis, and visualization. Having a solid understanding of these tools showcases your ability to transform raw data into meaningful insights.
Statistical Analysis and Mathematical Modeling: Data analysts must be able to apply statistical techniques and mathematical models to draw accurate conclusions from data. Skills in hypothesis testing, regression analysis, and probability are critical. Recruiters seek candidates who can apply these techniques to solve real-world problems, whether it's identifying trends, forecasting, or performing risk analysis. A strong grasp of these concepts demonstrates that you can handle complex datasets and extract actionable insights.
Data Visualization: The ability to present data visually is a key component of a data analyst's skill set. Recruiters look for candidates who can translate complex datasets into easy-to-understand charts, graphs, and dashboards. Effective data visualization helps organizations make data-driven decisions by making insights accessible to non-technical stakeholders. Familiarity with visualization tools such as Power BI, Tableau, and Looker can greatly enhance a resume and show that you can communicate findings clearly and effectively.
Critical Thinking and Problem-Solving: Beyond technical skills, recruiters value critical thinking and problem-solving abilities. Data analysts must not only analyze numbers but also interpret them within the context of the business or industry they're working in. Recruiters seek candidates who can ask the right questions, understand the nuances of the data, and provide innovative solutions to complex problems. This skill demonstrates your ability to think analytically and strategically, which is essential for making informed decisions. For those seeking data analyst jobs in Dubai, showcasing strong problem-solving skills can be a key differentiator in a highly competitive job market.
Knowledge of Data Management and Databases: Understanding how to manage and work with large datasets is critical for a career in data analytics. Recruiters often look for candidates with experience in data cleaning and data wrangling, which are crucial steps in preparing data for analysis. Knowledge of database management systems such as MySQL, PostgreSQL, or Oracle is also important. This expertise shows that you can work with structured and unstructured data, ensuring that the datasets used for analysis are accurate and reliable.
Communication Skills: One of the most underestimated but essential skills for data analysts is the ability to communicate findings effectively. Recruiters are looking for individuals who can present complex data and technical analysis in a way that is accessible to non-experts. Strong written and verbal communication skills are crucial for producing reports, presenting findings, and collaborating with other teams. The ability to explain data insights in clear, concise language can set you apart from other candidates.
Business Acumen: Finally, having a solid understanding of the business landscape is essential for any data analyst. Recruiters seek professionals who not only have technical skills but can also align their analysis with a company's business objectives. Understanding industry-specific challenges, KPIs, and the strategic goals of an organization allows data analysts to provide relevant insights that contribute to decision-making and growth.
In the fast-paced world of data analytics, recruiters look for a mix of technical expertise, analytical thinking, and communication skills. A well-rounded resume that showcases proficiency in tools and software, statistical analysis, data visualization, and business understanding is crucial for standing out in the competitive job market. For those seeking guidance, data analytics recruitment consultants in Dubai can provide invaluable insights and connect candidates with top organizations, ensuring that your resume highlights the skills that recruiters are most eager to find. Huxley, with its specialized expertise in data analytics recruitment, can help professionals navigate this landscape and secure the right opportunities. By partnering with Huxley, candidates can receive tailored career advice and access to top positions, ensuring a successful and fulfilling career path in the growing data analytics field.
0 notes
Text
Discover the Best Data Science ML & AI Course in Kerala: Your Key to a Future-Ready Career
In today’s fast-paced digital era, data has become the cornerstone of innovation and growth. From automating processes to providing actionable insights, data science, machine learning (ML), and artificial intelligence (AI) are transforming industries worldwide. If you’re looking to unlock career opportunities in this thriving field, enrolling in the best data science course in Kerala is your first step toward a successful future.
This blog will explore the benefits of pursuing data science training in Kerala, why Kerala is a prime destination for tech education, and how the best Machine Learning and AI courses can set you apart in this competitive field.
Why Opt for a Data Science Course in Kerala?
Kerala has emerged as a significant player in India’s tech ecosystem, with a growing demand for skilled professionals in data science, ML, and AI. Here’s why data science training in Kerala is an ideal choice:
1. Access to Top Training Institutes
Kerala is home to institutions like Quest Innovative Solutions, offering the best Data Science, ML & AI course in Kerala, tailored to meet global industry standards.
2. Growing IT Industry
Kerala’s tech hubs in cities like Kochi, Trivandrum, Calicut, and Kannur are thriving, providing ample job opportunities for data science graduates.
3. Affordable Education with Quality
Compared to other IT hubs, Kerala offers high-quality training at a fraction of the cost, making it a preferred destination for learners across India.
What Makes Data Science Courses in Kerala Unique?
The best data science course in Kerala blends theory and practical application to equip students with in-demand skills. Here’s what sets these courses apart:
Comprehensive Curriculum: Covering Python programming, statistical modeling, machine learning, artificial intelligence, and data visualization.
Hands-On Projects: Real-world projects using datasets from industries like healthcare, finance, and e-commerce.
Placement Support: Institutes like Quest Innovative Solutions provide dedicated placement services, helping students secure roles in top companies.
Experienced Trainers: Courses are led by industry experts with practical knowledge of data science, ML, and AI.
Why Data Science, ML & AI Are the Careers of Tomorrow
The need for data-driven decision-making is reshaping industries, making data science, ML, and AI some of the most sought-after skills globally. Here’s how they’re changing the game:
Enhanced Decision-Making: Data science helps businesses identify trends, forecast outcomes, and make strategic decisions.
Automation and Efficiency: Machine learning and AI automate repetitive tasks, increasing productivity.
Industry Applications: From predictive analytics in healthcare to fraud detection in banking, the possibilities are endless.
What Will You Learn in a Data Science Course in Kerala?
Enrolling in a data science course in Kerala equips you with the knowledge and skills needed to excel in this dynamic field. Key learning outcomes include:
Programming ProficiencyMaster programming languages like Python and R for data manipulation and analysis.
Machine Learning ExpertiseLearn to build predictive models and deploy machine learning algorithms for practical applications.
Data VisualizationUse tools like Tableau and Power BI to create compelling data stories.
Artificial Intelligence FrameworksGain hands-on experience with AI technologies like TensorFlow and Keras.
Real-World ApplicationWork on industry-specific datasets to solve real-world challenges.
Best Machine Learning Training in Kerala: Why It Matters
Machine learning is at the core of modern AI systems, making it a crucial component of any data science course. The best machine learning training in Kerala ensures you gain:
A strong foundation in ML algorithms.
Practical experience in building and testing predictive models.
Exposure to advanced concepts like deep learning and natural language processing (NLP).
Benefits of Data Science ML & AI Training in Kerala
1. High Demand for Data Science Professionals
With industries increasingly relying on data, the demand for data scientists, ML engineers, and AI specialists is skyrocketing.
2. Lucrative Career Opportunities
A career in data science promises high earning potential, with salaries often surpassing those in traditional IT roles.
3. Global Career Prospects
The skills gained from a data science course in Kerala are universally recognized, opening doors to opportunities worldwide.
Why Choose Quest Innovative Solutions for Data Science Training in Kerala?
When it comes to pursuing the best data science course in Kerala, Quest Innovative Solutions stands out as a leader. Here’s why:
1. Industry-Focused Curriculum
Our courses are designed to align with industry needs, ensuring you gain relevant skills.
2. Expert Trainers
Learn from experienced professionals who bring real-world insights into the classroom.
3. Hands-On Learning
We emphasize practical training with projects and live datasets.
4. Placement Assistance
Our dedicated placement team connects students with top companies, offering internships and full-time roles.
5. Flexible Learning Options
Choose between online and in-person classes at our centers in Kochi, Calicut, Trivandrum, and Kannur.
Success Stories from Our Data Science Course in Kerala
Many of our graduates have secured positions at leading companies thanks to our comprehensive training. Here are a few success stories:
Career Switch: A mechanical engineer transitioned to a high-paying role as a data analyst.
Fresh Graduate Success: A fresher with no prior experience landed a job as an AI specialist in a multinational company.
Rapid Career Growth: A working professional advanced to a senior ML engineer role within months of completing our course.
How to Get Started?
Enrolling in the best Data Science ML & AI course in Kerala is easy. Follow these steps:
Research and Choose the Right InstituteQuest Innovative Solutions is a trusted choice for comprehensive data science training.
Set Clear GoalsDecide your career objectives and focus on building skills to achieve them.
Commit to Hands-On LearningEngage in projects and internships to gain real-world experience.
Leverage Placement ServicesUse the institute’s placement support to connect with top companies.
Conclusion
In an era dominated by data, a career in data science, ML, and AI is your gateway to endless opportunities. The data science course in Kerala offered by Quest Innovative Solutions is designed to provide you with industry-relevant skills, hands-on experience, and unmatched placement support.
Whether you’re a fresher, a working professional, or someone looking to switch careers, our training programs cater to your needs. Don’t wait—take the first step toward a future-proof career in data science, ML, and AI today.
Admissions are now open for the new batch. Enroll in the best data science course in Kerala and secure your place in the tech-driven future!
#Best Data Science ML& AI course in kerala#data science course in kerala#data science training#Best Machine Learning Training in Kerala#Data science ML & AI training in kerala
0 notes
Text
Emerging Technologies Every Digital Marketing Company Should Use

The digital marketing landscape is evolving rapidly, and staying ahead requires more than traditional strategies. Emerging technologies are revolutionizing the way digital marketing companies operate, enabling them to deliver smarter, faster, and more personalized experiences for their clients. Here's a closer look at the top technologies reshaping the industry and why every digital marketing company should leverage them.
Digital Marketing Company in Coimbatore
1. Artificial Intelligence (AI) and Machine Learning (ML)
AI and ML are no longer futuristic concepts; they are integral to modern digital marketing. These technologies help companies analyse consumer behaviour, predict trends, and automate tasks.
Applications:
Chatbots for customer service.
Predictive analytics to anticipate customer needs.
Content personalization based on user preferences.
Why It Matters: AI streamlines operations, reduces costs, and enhances user engagement.
2. Marketing Automation Tools
Automation tools like HubSpot, Marketo, and ActiveCampaign have become indispensable. They allow marketers to schedule campaigns, manage leads, and track performance seamlessly.
Applications:
Automating email marketing workflows.
Scheduling social media posts.
Managing customer relationship management (CRM) systems.
Why It Matters: Saves time and ensures consistent communication with audiences.
3. Voice Search Optimization
With the rise of smart speakers and voice assistants like Alexa, Siri, and Google Assistant, voice search is a game-changer. Digital marketing companies must adapt their strategies to accommodate voice queries.
Applications:
Optimizing for long-tail keywords.
Creating FAQ-style content.
Ensuring mobile-friendly website designs.
Why It Matters: Voice search queries are conversational and intent-driven, providing valuable opportunities for engagement.
Digital Marketing Company in Coimbatore
4. Augmented Reality (AR) and Virtual Reality (VR)
AR and VR offer immersive experiences that captivate audiences. Brands use these technologies to showcase products and enhance customer interactions.
Applications:
AR filters on social media platforms.
Virtual showrooms for e-commerce.
Interactive ad campaigns.
Why It Matters: Creates memorable, interactive experiences that drive customer engagement and loyalty.
5. Blockchain Technology
Blockchain ensures transparency and security in digital marketing. From verifying ad metrics to protecting consumer data, its applications are vast.
Applications:
Preventing ad fraud through transparent transactions.
Building trust with secure data handling.
Creating decentralized marketing platforms.
Why It Matters: Builds consumer trust and ensures ethical marketing practices.
6. Advanced Analytics and Data Visualization
Data is the backbone of digital marketing, and advanced analytics tools like Google Analytics 4, Tableau, and Power BI provide actionable insights.
Applications:
Tracking campaign performance.
Understanding customer journeys.
Identifying high-performing content.
Why It Matters: Helps companies make data-driven decisions and optimize strategies.
Digital Marketing Agency in Coimbatore
7. Programmatic Advertising
Programmatic advertising leverages AI to automate the buying and selling of ads, ensuring they are delivered to the right audience at the optimal time.
Applications:
Real-time bidding for ad placements.
Targeting niche audiences with precision.
Measuring ad performance accurately.
Why It Matters: Improves ROI by delivering highly targeted and efficient ad campaigns.
8. 5G Technology
The rollout of 5G is revolutionizing how consumers access content online. Faster internet speeds mean higher-quality video streaming and quicker website loading times.
Applications:
Enhanced mobile marketing campaigns.
Immersive AR/VR experiences.
Instant delivery of high-quality content.
Why It Matters: Creates opportunities for innovative content formats and seamless user experiences.
9. Social Commerce Tools
Platforms like Instagram, Facebook, and TikTok are merging social media with e-commerce, enabling direct product purchases.
Applications:
Shoppable posts and stories.
Influencer marketing with direct sales links.
Integrating e-commerce platforms with social profiles.
Why It Matters: Streamlines the buyer journey and boosts conversions.
Digital Marketing Agency in Coimbatore
Conclusion
Adopting emerging technologies is not just about staying competitive—it’s about leading the way in delivering innovative, impactful solutions for clients. By leveraging AI, automation, voice search, AR/VR, blockchain, and more, digital marketing companies can transform their strategies, improve efficiency, and deliver exceptional results.
The future of digital marketing is here—embrace it or risk being left behind.
#digital marketing company in Coimbatore#digital marketing agency in Coimbatore#digital marketing services in Coimbatore#best digital marketing company in Coimbatore
0 notes
Text
Data Visualization in Action: The Science of Visual Insights
In the era of big data, the ability to communicate complex information effectively has never been more critical. Data visualization serves as a bridge between raw data and meaningful understanding, transforming numbers and statistics into clear, compelling visual narratives. It is both an art and a science, enabling data to tell stories that drive decisions, spark innovation, and inspire action.
The Role of Data Visualization
Data visualization simplifies complex datasets by presenting information in graphical formats such as charts, graphs, heat maps, and dashboards. These visual tools make patterns, trends, and anomalies instantly recognizable, allowing audiences to process information faster than with text or raw numbers.
For example, a line graph can depict sales trends over a year, highlighting seasonal peaks and dips at a glance. Similarly, heat maps can identify geographic regions with high customer engagement, guiding targeted marketing strategies.
The Science Behind Visual Insights
The effectiveness of data visualization lies in its foundation in cognitive science. Visual elements like color, size, and spatial relationships are processed by the human brain more intuitively than numerical data. When well-designed, visualizations enhance comprehension, retention, and decision-making.
Key principles for effective data visualization include:
Clarity: Visuals should prioritize simplicity, avoiding clutter and unnecessary elements.
Relevance: Visualizations should align with the audience's needs, focusing on insights that drive action.
Consistency: Standardized formats and labels help maintain clarity and professionalism.
Tools and Technologies
Modern tools like Tableau, Power BI, and D3.js empower professionals to create dynamic, interactive visualizations. These platforms allow users to manipulate data in real-time, exploring different perspectives to uncover hidden insights.
Applications Across Industries
Business: Companies use dashboards to monitor KPIs, track performance, and optimize operations.
Healthcare: Visualizing patient data aids in diagnostics, treatment planning, and resource allocation.
Education: Data visualization enhances learning by making abstract concepts more accessible.
Government: Visual tools support policy-making by presenting demographic or economic data clearly.
The Future of Data Visualization
As artificial intelligence and machine learning evolve, data visualization is becoming even more powerful. Predictive visual analytics, augmented reality dashboards, and automated storytelling are transforming how we interpret and act on data.
Conclusion
Data visualization is more than a technical skill; it’s a critical communication tool in a world where data drives every decision. By mastering the science of visual insights, individuals and organizations can unlock the full potential of their data, turning complexity into clarity and action.
In today’s data-rich landscape, the ability to visualize effectively isn't just an advantage—it's a necessity.
0 notes
Text
5 Tools Everyone in the Data Science Full Course Industry Should Be Using
The data science field thrives on the use of cutting-edge tools that make data analysis, modeling, and visualization seamless. Whether you're just starting a data science full course or working in the industry, knowing the right tools can supercharge your efficiency and impact. In this blog, we’ll highlight 5 essential tools every data science professional and learner should incorporate into their workflow.
1. Python
The Versatile Programming Powerhouse Python has become synonymous with data science because of its simplicity and extensive library ecosystem.
Key Features:
Libraries like Pandas for data manipulation, Matplotlib for visualization, and Scikit-learn for machine learning.
Wide adoption in both academia and industry.
Why It’s Essential: Python is beginner-friendly yet robust enough for advanced tasks, making it the backbone of most data science projects.
Example Use: Analyze a large dataset to uncover trends or train a predictive machine learning model.
2. R
The Statistician’s Best Friend R is a programming language designed for statistical analysis and visualization.
Key Features:
Advanced statistical modeling capabilities.
Libraries like ggplot2 for stunning data visualizations.
Why It’s Essential: R excels in statistical tasks, making it invaluable for data scientists focused on analytics.
Pro Tip: Use R for projects requiring detailed statistical testing or visualization.
3. SQL
The Database Querying Expert Data is often stored in relational databases, and SQL (Structured Query Language) is the universal tool for accessing it.
Key Features:
Efficient querying of massive datasets.
Integration with data visualization and analysis tools.
Why It’s Essential: Almost every data science job requires SQL proficiency to retrieve and prepare data.
Example Use: Write a query to extract sales data for a specific product category over the last year.
4. Jupyter Notebook
The Interactive Workspace for Coding and Sharing Jupyter Notebook allows you to combine live code, visualizations, and text explanations in one interface.
Key Features:
Interactive Python environment for coding.
Markdown support for creating rich documentation.
Why It’s Essential: Perfect for documenting workflows, creating tutorials, and presenting analysis.
Pro Tip: Use Jupyter to create sharable notebooks that showcase your work in a professional format.
5. Tableau
The Data Visualization Pro When it comes to presenting insights, Tableau is a game-changer.
Key Features:
Drag-and-drop functionality for creating dashboards.
Ability to connect to various data sources.
Why It’s Essential: Tableau makes it easy to turn raw data into visually compelling stories that stakeholders can understand.
Example Use: Build a dashboard showing real-time sales performance across multiple regions.
Conclusion
These five tools—Python, R, SQL, Jupyter Notebook, and Tableau—are the cornerstones of success in data science. By mastering them, you'll not only excel in your data science full course but also set a solid foundation for a successful career.
Want to see these tools in action? Check out our YouTube Live session to learn how to harness their full potential in your data science journey!
0 notes