#Data science internship
Explore tagged Tumblr posts
Text
Worried About Finding a Job in Data Science? What If You Had Job-Secured Training ?
With Futurix Academy’s job-secured programs, you’ll master the latest skills in AI, machine learning, and Python, while gaining access to career support and placement opportunities.
#artificial intelligence#data analytics#data science#deep learning#machinelearning#data science course in kerala#data science course in kochi#python programming#data science career#data science job#data science internship#data science training#data science certification
0 notes
Text
Data Science Internships: Kickstart Your Career in Analytics
In today's data-driven world, the demand for data science professionals is skyrocketing. Companies across various industries are leveraging data analytics to make informed decisions, optimize operations, and enhance customer experiences. For students and recent graduates, securing a data science internship can be a pivotal step toward a successful career in analytics. This article explores the significance of data science internships, the skills you can gain, and how platforms like Salarite can help you find the right opportunity.
The Growing Importance of Data Science
Data science has emerged as one of the most sought-after fields in recent years. With businesses generating vast amounts of data daily, the ability to analyze and interpret this information is crucial. Here are some key reasons why data science is essential:
Informed Decision-Making: Organizations rely on data analysis to guide their strategies and improve operational efficiency.
Competitive Advantage: Companies that harness data effectively can outperform their competitors by understanding market trends and customer preferences.
Diverse Applications: Data science is applicable across various sectors, including finance, healthcare, marketing, and technology.
Why Pursue a Data Science Internship?
Internships provide invaluable experience and exposure to real-world applications of data science concepts. Here are several reasons to consider pursuing a data science internship:
Hands-On Experience: Internships allow you to work on actual projects, applying theoretical knowledge to practical situations. This experience is crucial for building your resume.
Skill Development: You will have the opportunity to develop essential skills such as programming (Python, R), statistical analysis, machine learning, and data visualization tools (Tableau, Power BI).
Networking Opportunities: Internships enable you to connect with industry professionals, mentors, and peers who can provide guidance and support throughout your career.
Understanding Industry Standards: Working in a professional environment helps you grasp industry standards and best practices in data analytics.
Career Exploration: Internships allow you to explore different roles within data science, helping you identify your strengths and areas of interest.
Key Skills for Data Science Interns
To excel in a data science internship, focus on developing the following skills:
Statistical Analysis: A solid understanding of statistics is fundamental for analyzing data sets and drawing meaningful conclusions.
Programming Skills: Proficiency in programming languages such as Python or R is essential for manipulating data and building models.
Data Visualization: The ability to present data insights visually using tools like Tableau or Matplotlib helps communicate findings effectively.
Machine Learning: Familiarity with machine learning algorithms enables you to build predictive models that can drive business decisions.
Critical Thinking: Strong analytical skills and critical thinking are necessary for interpreting complex data sets and solving problems creatively.
Finding Data Science Internships
With the increasing demand for data science professionals, numerous platforms can help you find internships tailored to your interests. One such platform is Salarite, which connects aspiring interns with top startups looking for talent in analytics.
Tips for Securing an Internship
Build a Strong Resume: Highlight relevant coursework, projects, and any technical skills related to data science. Tailor your resume for each application.
Create a Portfolio: Showcase your projects on platforms like GitHub or personal websites. Include examples of your work with datasets or any analyses you've conducted.
Leverage Networking: Attend industry events, webinars, or workshops to meet professionals in the field. Networking can lead to internship opportunities that may not be publicly advertised.
Prepare for Interviews: Research common interview questions related to data science and practice articulating your thought process when solving problems.
Stay Updated on Industry Trends: Follow blogs, podcasts, or online courses related to data science to stay informed about new tools and methodologies.
The Future of Data Science Internships
As companies increasingly rely on data-driven insights, the demand for skilled professionals will continue to rise. This trend translates into more internship opportunities across various industries. Emerging fields such as artificial intelligence (AI) and big data analytics are also creating new roles that require specialized knowledge.
Internships will play a crucial role in preparing the next generation of data scientists by providing them with practical experience and exposure to cutting-edge technologies. By pursuing a data science internship now, you position yourself at the forefront of this exciting field.
Conclusion
Embarking on a career in analytics through a data science internship is an excellent way to kickstart your professional journey. With the right skills and determination, you can make significant contributions to organizations while gaining valuable experience that will serve you well throughout your career.
Platforms like Salarite simplify the process of finding internships by connecting you with startups eager for fresh talent in analytics. Embrace this opportunity to learn, grow, and build a successful future in the dynamic world of data science!
By investing time in developing relevant skills and seeking out internships in this thriving field, you set yourself up for success in an ever-evolving job market that values analytical expertise. Start your journey today!
0 notes
Text
Unlocking Opportunities in a Data-Driven World: Why You Should Master Data Science
In today’s fast-paced digital era, data science has emerged as a game-changing skill across industries. Organizations rely on data scientists to unravel complex datasets, generate actionable insights, and influence critical business decisions. Whether you’re starting from scratch or aiming to refine your expertise, diving into data science can unlock a wealth of career opportunities. Here’s how you can get started with top-notch courses designed for aspiring data scientists.
Why Pursue Data Science?
Data science is an interdisciplinary field that blends programming, statistics, and domain expertise to uncover patterns and insights hidden within data. As businesses generate massive amounts of data daily, professionals with data science expertise are more in demand than ever. Here’s why learning data science could be your next big career move:
High Earning Potential: Data science professionals command competitive salaries and excellent growth prospects.
Versatile Career Options: From data analysts and machine learning engineers to AI specialists and business intelligence experts, the possibilities are vast.
Meaningful Work: Contribute to solving real-world challenges, optimizing business processes, and enhancing customer experiences.
Future-Proof Your Career: With rapid technological advancements, data science remains a cornerstone across industries.
Explore Top Data Science Courses
1. Coursera - Data Science Specialization (Johns Hopkins University)
Overview: A 10-course program covering data cleaning, exploratory analysis, machine learning, and R programming.
Why Choose It: Comprehensive and beginner-friendly with practical projects to showcase your skills.
2. edX - MicroMasters in Data Science (University of California, San Diego)
Overview: Advanced training in Python, machine learning, and big data analytics.
Why Choose It: Ideal for professionals seeking in-depth technical skills and certification.
3. Udemy - Data Science A-Z™: Real-Life Data Science Exercises Included
Overview: A hands-on course emphasizing data preprocessing, visualization, and practical exercises.
Why Choose It: Affordable, flexible, and perfect for quick skill enhancement.
4. Google’s Data Analytics Professional Certificate (Coursera)
Overview: A beginner-friendly program covering tools like Excel, SQL, and Tableau for data analysis and visualization.
Why Choose It: Entry-level certification with industry recognition.
5. Kaggle Learn
Overview: Free, self-paced micro-courses on Python, Pandas, and machine learning.
Why Choose It: Focused on practical coding challenges with flexibility to fit any schedule.
Essential Skills to Master
By enrolling in data science courses, you’ll develop expertise in:
Programming: Learn Python, R, and SQL for effective data handling and analysis.
Statistical Analysis: Interpret data using advanced statistical methods.
Machine Learning: Build predictive models and understand key algorithms.
Data Visualization: Create impactful visuals using Tableau, Power BI, or Matplotlib.
Big Data Tools: Gain hands-on experience with Hadoop, Spark, and other big
#data science#data analytics#data science training#data security#data science course#data science internship
0 notes
Text
Data Science Course in Bhagalpur
Boost your career with the DataMites Data Science course in Bhagalpur. Gain hands-on skills through both online and offline classes, along with internship and job assistance. Earn globally recognized certifications from IABAC® and NASSCOM® to enhance your professional credentials.
0 notes
Text
Understanding Data Visualization for Beginners: A Complete Guide

1. What is Data Visualization?
At its core, data visualization is the process of converting complex data sets into visual formats such as charts, graphs, and maps. These visuals help transform raw numbers or abstract information into digestible formats, making it easier to spot patterns, trends, and insights that might not be obvious in a spreadsheet.
For example, looking at a table of stock prices may not immediately reveal whether the stock is on an upward or downward trend. However, when visualized in a line graph, the trajectory becomes clear at a glance.https://internshipgate.com
2. Why Data Visualization is Important
Data visualization is crucial because it makes data:
Easier to understand: It simplifies complex information.
Actionable: Insights from visualized data can inform decision-making.
Engaging: Visuals grab attention more effectively than plain numbers.
Communicative: They allow complex data to be presented to a broad audience, even those without a strong background in data analysis.
Whether you're presenting sales figures to a team, explaining scientific research, or studying trends in customer behavior, effective data visualization helps make your points clearer and more compelling.
3. Key Elements of Data Visualization
To create a successful data visualization, certain elements must come together effectively. These include:
Data: The raw information you're representing.
Visual Encoding: Using graphical elements (e.g., lines, bars, points) to represent different data points.
Labels and Legends: These explain the data and the relationships shown in the visualization.
Scale: Ensures accurate comparison across data points.
Context: The background information that helps users understand what the visualization is about.
4. Types of Data Visualizations
There are many types of visualizations, each with its own strengths depending on the data and the story you're trying to tell.
4.1 Bar Charts
Bar charts use rectangular bars to represent different data categories. They are best used for comparing quantities across categories, like showing sales for different products.
4.2 Line Graphs
A line graph displays data points connected by straight lines. It's commonly used to visualize trends over time, such as tracking monthly website visitors.
4.3 Pie Charts
Pie charts show proportions of a whole, divided into slices. Each slice represents a category's contribution to the overall dataset, like showing market share between different companies.
4.4 Scatter Plots
Scatter plots place points on a graph to display the relationship between two variables. For example, a scatter plot can show the correlation between years of education and income.
4.5 Heat Maps
Heat maps use color to show data density or intensity. A geographic heat map, for instance, might display the population density in various regions using varying shades of color.
5. Best Practices for Effective Data Visualization
Creating clear, effective data visualizations is an art and a science. Here are some best practices to ensure your visuals are both informative and easy to understand.
5.1 Choose the Right Type of Visualization
Every dataset tells a different story. A key part of data visualization is choosing the type of chart that best represents the data you're working with. For example, trends over time are often best shown using line graphs, while comparisons between categories might be better suited to bar charts.
5.2 Simplify the Design
The simpler the design, the easier it is for your audience to understand the data. Avoid overloading your charts with unnecessary elements like too many colors, gridlines, or decorations.
5.3 Use Color Intentionally
Color can be a powerful tool in data visualization if used correctly. Use color to highlight important data points or show differences between categories. However, too many colors can be overwhelming, so use them sparingly.
5.4 Label Your Visuals Clearly
Your audience needs to understand what the chart is showing. Make sure you include clear labels for axes, data points, and categories. Always provide a legend if multiple colors or symbols are used.
6. Common Mistakes in Data Visualization
Beginners often make some common errors when creating visualizations:
Choosing the wrong chart type: Not every chart suits every dataset.
Too much data in one chart: Overloading a chart with too many data points or variables can make it hard to interpret.
Ignoring data context: Visuals without proper context can be misleading. Always provide enough background for the data to be understood.
Improper scaling: Failing to maintain proportional scales can distort the data and mislead the viewer.
7. Tools to Get Started with Data Visualization
Many tools can help you create beautiful and insightful data visualizations, even if you're a beginner.
7.1 Microsoft Excel
Excel is a great starting point for basic data visualizations, such as bar charts, pie charts, and line graphs. It’s user-friendly and widely accessible.
7.2 Google Charts
Google Charts is a free, web-based tool that allows users to create interactive charts that can be easily shared online.
7.3 Tableau
Tableau is a popular data visualization tool for more advanced visualizations. It provides a wide range of customization options and is particularly useful for larger datasets.
7.4 Power BI
Microsoft's Power BI is another advanced tool that allows users to create interactive reports and dashboards, making it ideal for business use.
8. Case Study: A Beginner's Approach to Visualizing Data
Imagine you have sales data for five different products over the past year. A beginner might start by inputting this data into Excel and creating a simple bar chart to compare monthly sales between the products. This allows the user to quickly see which product performs best, and how sales change over time.
9. How to Interpret Data Visualizations
When looking at data visualizations, it's important to:
Understand the context of the data.
Look for trends, patterns, and outliers.
Be cautious of scale manipulation or visual elements that might distort the data.
For example, a bar chart that shows year-over-year growth may look impressive, but it's important to check if the scale starts at zero to avoid exaggerating small changes.
10. Data Visualization in Different Industries
Data visualization has applications across various industries:
Business: Track key performance indicators (KPIs), sales trends, and customer demographics.
Healthcare: Visualize patient data, medical trends, and research findings.
Education: Analyze student performance and teaching effectiveness.
Marketing: Measure campaign success, engagement rates, and audience behavior.
Science: Represent experimental results, population studies, and environmental data.
11. The Future of Data Visualization
The future of data visualization is exciting, with developments like:
AI-powered analytics: Automatically generating the best visual representations based on data.
Virtual reality (VR) and augmented reality (AR): Enabling immersive data exploration.
Interactive visualizations: Allowing users to interact with data in real time, exploring different scenarios and outcomes.
12. FAQs About Data Visualization
1. What is the best tool for beginners? For beginners, Microsoft Excel or Google Charts is a great place to start due to their simplicity and ease of use.
2. What types of data are best suited for visualizations? Any data can be visualized, but it's particularly useful for large datasets, time-series data, or comparative analysis.
3. How can I avoid making mistakes in my visualizations? Stick to best practices like choosing the right chart, simplifying your design, and clearly labeling all elements.
4. Can data visualizations be misleading? Yes, improper scaling, poor chart selection, or a lack of context can distort the data, leading to incorrect conclusions.https://internshipgate.com
#career#data analytics#data#internship#internshipgate#internship in india#virtualinternship#data science internship#job opportunities
1 note
·
View note
Text

#Data Science#Data Science Course#Data Science Internship#Data Analytics#Data Science Internship in Pune#Cgit
0 notes
Text
Opening Doors with a Program for Data Science Internships
Data is becoming the new oil in the rapidly changing world of technology, influencing choices across all sectors of the economy. The need for qualified data scientists is growing as businesses depend more and more on data to make decisions. A data science internship program provides a priceless chance for individuals wishing to enter into this exciting industry to obtain practical experience, develop necessary skills, and launch a bright future.

The Importance of a Data Science InternshipAn internship in data science helps close the knowledge gap between the classroom and practical implementation. The theoretical underpinning is provided by coursework, but internships give students the opportunity to work on real projects, interacting with real data sets and resolving real business issues. Understanding the intricacies of data analysis, processing, and visualization in a professional setting requires this hands-on experience. Participants in the internship get exposure to industry-standard tools and technologies like SQL, TensorFlow, R, and Python in addition to machine learning frameworks like Scikit-learn. Interns can hone their technical abilities and develop confidence in their capacity to take on data-driven difficulties by using these tools on actual assignments.
Principal Advantages of a Data Science Internship:
Practical Experience: Internships give students the opportunity to apply their theoretical knowledge to real-world problems through hands-on experience, which deepens their comprehension of data science methods. Development of Soft Skills: Soft skills, such as problem-solving, collaboration, and communication, are crucial for a successful career in data science and can be developed through internships in addition to technical ones. Opportunities for Networking: Internships provide an opportunity to meet people in the sector, which may lead to employment offers, mentorship opportunities, and insightful information about the industry. Resume Building: Completing a Data Science Internship shows employers that a candidate can use their skills in a real-world situation, which increases their attractiveness to employers.
What to Anticipate An internship in data science often lasts three to six months, during which time interns work in teams on a variety of projects involving data. These could involve activities like constructing models, data visualization, exploratory data analysis (EDA), and data collecting and cleaning. In addition, interns take part in team discussions, attend meetings, and sometimes even present their results to stakeholders. How to Begin Candidates should have a strong background in programming, statistics, and mathematics in addition to experience with data science tools in order to land a data science internship. Creating a portfolio of your work and connecting with industry experts will help you increase your chances of getting an internship.
The first step towards a prosperous career in this fascinating profession is an internship program in data science. It is a crucial first step for anyone wishing to enter the data science field since it offers the knowledge, abilities, and networks required to succeed in the field.
0 notes
Text
Uncover the World of Data Science in Pune
Discover the endless possibilities of data science through our immersive course in Pune. Gain expertise in analyzing data, extracting insights, and driving informed decisions. Equip yourself with essential skills for today's data-driven world with NeuAI Labs.
#data science training#data science course#data science online course#data scientist course#data science internship#data analysis certification#data analytics course#data analyst course#neuailabs#futureofai
0 notes
Text
Truck Manufacturing Company Hiring | UD TRUCKS | Senior Data Analyst - Quality Assurance role - apply now
Introduction Truck Manufacturing Company Hiring: UD Truck has Published notification for the vacancy of Data Analyst The educational qualification required to apply for this UD Truck is B.E ,B.Tech Engineers Interested and eligible candidates can apply for UD Truck. There is enough time to apply for any job. Read UD Truck date, last date to apply, full details of vacancies carefully. As per…

View On WordPress
#data analyst jobs for freshers#data analyst jobs in chennai#data entry jobs in coimbatore#data quality analyst#data quality manager#data science internship#data science jobs for freshers#data scientist jobs for freshers#truck company jobs#Truck Manufacturing Company Hiring | UD TRUCKS | Senior Data Analyst - Quality Assurance role - apply now
0 notes
Text
An online web development course is a virtual learning program designed to teach individuals the skills and knowledge necessary to build websites and web applications. These courses are typically delivered through an online platform or learning management system and can be taken at the learner's own pace.
#Java Developer Internship#Data Science Internship#Internship Machine Learning#Online Web Development Course
0 notes
Text
"From Passion to Profession: Steps to Enter the Tech Industry"
How to Break into the Tech World: Your Comprehensive Guide
In today’s fast-paced digital landscape, the tech industry is thriving and full of opportunities. Whether you’re a student, a career changer, or someone passionate about technology, you may be wondering, “How do I get into the tech world?” This guide will provide you with actionable steps, resources, and insights to help you successfully navigate your journey.
Understanding the Tech Landscape
Before you start, it's essential to understand the various sectors within the tech industry. Key areas include:
Software Development: Designing and building applications and systems.
Data Science: Analyzing data to support decision-making.
Cybersecurity: Safeguarding systems and networks from digital threats.
Product Management: Overseeing the development and delivery of tech products.
User Experience (UX) Design: Focusing on the usability and overall experience of tech products.
Identifying your interests will help you choose the right path.
Step 1: Assess Your Interests and Skills
Begin your journey by evaluating your interests and existing skills. Consider the following questions:
What areas of technology excite me the most?
Do I prefer coding, data analysis, design, or project management?
What transferable skills do I already possess?
This self-assessment will help clarify your direction in the tech field.
Step 2: Gain Relevant Education and Skills
Formal Education
While a degree isn’t always necessary, it can be beneficial, especially for roles in software engineering or data science. Options include:
Computer Science Degree: Provides a strong foundation in programming and system design.
Coding Bootcamps: Intensive programs that teach practical skills quickly.
Online Courses: Platforms like Coursera, edX, and Udacity offer courses in various tech fields.
Self-Learning and Online Resources
The tech industry evolves rapidly, making self-learning crucial. Explore resources like:
FreeCodeCamp: Offers free coding tutorials and projects.
Kaggle: A platform for data science practice and competitions.
YouTube: Channels dedicated to tutorials on coding, design, and more.
Certifications
Certifications can enhance your credentials. Consider options like:
AWS Certified Solutions Architect: Valuable for cloud computing roles.
Certified Information Systems Security Professional (CISSP): Great for cybersecurity.
Google Analytics Certification: Useful for data-driven positions.
Step 3: Build a Portfolio
A strong portfolio showcases your skills and projects. Here’s how to create one:
For Developers
GitHub: Share your code and contributions to open-source projects.
Personal Website: Create a site to display your projects, skills, and resume.
For Designers
Design Portfolio: Use platforms like Behance or Dribbble to showcase your work.
Case Studies: Document your design process and outcomes.
For Data Professionals
Data Projects: Analyze public datasets and share your findings.
Blogging: Write about your data analysis and insights on a personal blog.
Step 4: Network in the Tech Community
Networking is vital for success in tech. Here are some strategies:
Attend Meetups and Conferences
Search for local tech meetups or conferences. Websites like Meetup.com and Eventbrite can help you find relevant events, providing opportunities to meet professionals and learn from experts.
Join Online Communities
Engage in online forums and communities. Use platforms like:
LinkedIn: Connect with industry professionals and share insights.
Twitter: Follow tech influencers and participate in discussions.
Reddit: Subreddits like r/learnprogramming and r/datascience offer valuable advice and support.
Seek Mentorship
Finding a mentor can greatly benefit your journey. Reach out to experienced professionals in your field and ask for guidance.
Step 5: Gain Practical Experience
Hands-on experience is often more valuable than formal education. Here’s how to gain it:
Internships
Apply for internships, even if they are unpaid. They offer exposure to real-world projects and networking opportunities.
Freelancing
Consider freelancing to build your portfolio and gain experience. Platforms like Upwork and Fiverr can connect you with clients.
Contribute to Open Source
Engaging in open-source projects can enhance your skills and visibility. Many projects on GitHub are looking for contributors.
Step 6: Prepare for Job Applications
Crafting Your Resume
Tailor your resume to highlight relevant skills and experiences. Align it with the job description for each application.
Writing a Cover Letter
A compelling cover letter can set you apart. Highlight your passion for technology and what you can contribute.
Practice Interviewing
Prepare for technical interviews by practicing coding challenges on platforms like LeetCode or HackerRank. For non-technical roles, rehearse common behavioral questions.
Step 7: Stay Updated and Keep Learning
The tech world is ever-evolving, making it crucial to stay current. Subscribe to industry newsletters, follow tech blogs, and continue learning through online courses.
Follow Industry Trends
Stay informed about emerging technologies and trends in your field. Resources like TechCrunch, Wired, and industry-specific blogs can provide valuable insights.
Continuous Learning
Dedicate time each week for learning. Whether through new courses, reading, or personal projects, ongoing education is essential for long-term success.
Conclusion
Breaking into the tech world may seem daunting, but with the right approach and commitment, it’s entirely possible. By assessing your interests, acquiring relevant skills, building a portfolio, networking, gaining practical experience, preparing for job applications, and committing to lifelong learning, you’ll be well on your way to a rewarding career in technology.
Embrace the journey, stay curious, and connect with the tech community. The tech world is vast and filled with possibilities, and your adventure is just beginning. Take that first step today and unlock the doors to your future in technology!
contact Infoemation wensite: https://agileseen.com/how-to-get-to-tech-world/ Phone: 01722-326809 Email: [email protected]
#tech career#how to get into tech#technology jobs#software development#data science#cybersecurity#product management#UX design#tech education#networking in tech#internships#freelancing#open source contribution#tech skills#continuous learning#job application tips
8 notes
·
View notes
Text

Worried About Finding a Job in Data Science? What If You Had Job-Secured Training?
Futurix Academy provides hands-on, career-focused data science training that equips you with industry-ready skills in machine learning, AI, and Python. Their courses are designed for both beginners and professionals, offering flexible learning options and job-secured education to jumpstart your career
#artificial intelligence#data science#data analytics#deep learning#data science course in kerala#data science course in kochi#machinelearning#python programming#data science training#data science internship
0 notes
Text
Your Guide to B.Tech in Computer Science & Engineering Colleges
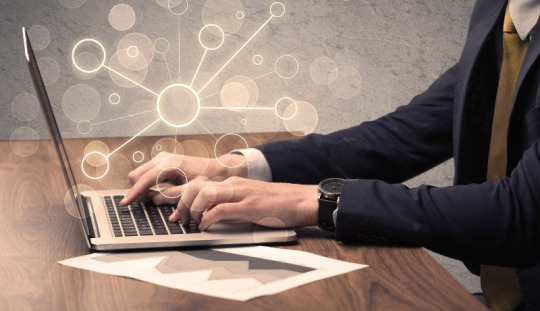
In today's technology-driven world, pursuing a B.Tech in Computer Science and Engineering (CSE) has become a popular choice among students aspiring for a bright future. The demand for skilled professionals in areas like Artificial Intelligence, Machine Learning, Data Science, and Cloud Computing has made computer science engineering colleges crucial in shaping tomorrow's innovators. Saraswati College of Engineering (SCOE), a leader in engineering education, provides students with a perfect platform to build a successful career in this evolving field.
Whether you're passionate about coding, software development, or the latest advancements in AI, pursuing a B.Tech in Computer Science and Engineering at SCOE can open doors to endless opportunities.
Why Choose B.Tech in Computer Science and Engineering?
Choosing a B.Tech in Computer Science and Engineering isn't just about learning to code; it's about mastering problem-solving, logical thinking, and the ability to work with cutting-edge technologies. The course offers a robust foundation that combines theoretical knowledge with practical skills, enabling students to excel in the tech industry.
At SCOE, the computer science engineering courses are designed to meet industry standards and keep up with the rapidly evolving tech landscape. With its AICTE Approved, NAAC Accredited With Grade-"A+" credentials, the college provides quality education in a nurturing environment. SCOE's curriculum goes beyond textbooks, focusing on hands-on learning through projects, labs, workshops, and internships. This approach ensures that students graduate not only with a degree but with the skills needed to thrive in their careers.
The Role of Computer Science Engineering Colleges in Career Development
The role of computer science engineering colleges like SCOE is not limited to classroom teaching. These institutions play a crucial role in shaping students' futures by providing the necessary infrastructure, faculty expertise, and placement opportunities. SCOE, established in 2004, is recognized as one of the top engineering colleges in Navi Mumbai. It boasts a strong placement record, with companies like Goldman Sachs, Cisco, and Microsoft offering lucrative job opportunities to its graduates.
The computer science engineering courses at SCOE are structured to provide a blend of technical and soft skills. From the basics of computer programming to advanced topics like Artificial Intelligence and Data Science, students at SCOE are trained to be industry-ready. The faculty at SCOE comprises experienced professionals who not only impart theoretical knowledge but also mentor students for real-world challenges.
Highlights of the B.Tech in Computer Science and Engineering Program at SCOE
Comprehensive Curriculum: The B.Tech in Computer Science and Engineering program at SCOE covers all major areas, including programming languages, algorithms, data structures, computer networks, operating systems, AI, and Machine Learning. This ensures that students receive a well-rounded education, preparing them for various roles in the tech industry.
Industry-Relevant Learning: SCOE’s focus is on creating professionals who can immediately contribute to the tech industry. The college regularly collaborates with industry leaders to update its curriculum, ensuring students learn the latest technologies and trends in computer science engineering.
State-of-the-Art Infrastructure: SCOE is equipped with modern laboratories, computer centers, and research facilities, providing students with the tools they need to gain practical experience. The institution’s infrastructure fosters innovation, helping students work on cutting-edge projects and ideas during their B.Tech in Computer Science and Engineering.
Practical Exposure: One of the key benefits of studying at SCOE is the emphasis on practical learning. Students participate in hands-on projects, internships, and industry visits, giving them real-world exposure to how technology is applied in various sectors.
Placement Support: SCOE has a dedicated placement cell that works tirelessly to ensure students secure internships and job offers from top companies. The B.Tech in Computer Science and Engineering program boasts a strong placement record, with top tech companies visiting the campus every year. The highest on-campus placement offer for the academic year 2022-23 was an impressive 22 LPA from Goldman Sachs, reflecting the college’s commitment to student success.
Personal Growth: Beyond academics, SCOE encourages students to participate in extracurricular activities, coding competitions, and tech fests. These activities enhance their learning experience, promote teamwork, and help students build a well-rounded personality that is essential in today’s competitive job market.
What Makes SCOE Stand Out?
With so many computer science engineering colleges to choose from, why should you consider SCOE for your B.Tech in Computer Science and Engineering? Here are a few factors that make SCOE a top choice for students:
Experienced Faculty: SCOE prides itself on having a team of highly qualified and experienced faculty members. The faculty’s approach to teaching is both theoretical and practical, ensuring students are equipped to tackle real-world challenges.
Strong Industry Connections: The college maintains strong relationships with leading tech companies, ensuring that students have access to internship opportunities and campus recruitment drives. This gives SCOE graduates a competitive edge in the job market.
Holistic Development: SCOE believes in the holistic development of students. In addition to academic learning, the college offers opportunities for personal growth through various student clubs, sports activities, and cultural events.
Supportive Learning Environment: SCOE provides a nurturing environment where students can focus on their academic and personal growth. The campus is equipped with modern facilities, including spacious classrooms, labs, a library, and a recreation center.
Career Opportunities After B.Tech in Computer Science and Engineering from SCOE
Graduates with a B.Tech in Computer Science and Engineering from SCOE are well-prepared to take on various roles in the tech industry. Some of the most common career paths for CSE graduates include:
Software Engineer: Developing software applications, web development, and mobile app development are some of the key responsibilities of software engineers. This role requires strong programming skills and a deep understanding of software design.
Data Scientist: With the rise of big data, data scientists are in high demand. CSE graduates with knowledge of data science can work on data analysis, machine learning models, and predictive analytics.
AI Engineer: Artificial Intelligence is revolutionizing various industries, and AI engineers are at the forefront of this change. SCOE’s curriculum includes AI and Machine Learning, preparing students for roles in this cutting-edge field.
System Administrator: Maintaining and managing computer systems and networks is a crucial role in any organization. CSE graduates can work as system administrators, ensuring the smooth functioning of IT infrastructure.
Cybersecurity Specialist: With the growing threat of cyberattacks, cybersecurity specialists are essential in protecting an organization’s digital assets. CSE graduates can pursue careers in cybersecurity, safeguarding sensitive information from hackers.
Conclusion: Why B.Tech in Computer Science and Engineering at SCOE is the Right Choice
Choosing the right college is crucial for a successful career in B.Tech in Computer Science and Engineering. Saraswati College of Engineering (SCOE) stands out as one of the best computer science engineering colleges in Navi Mumbai. With its industry-aligned curriculum, state-of-the-art infrastructure, and excellent placement record, SCOE offers students the perfect environment to build a successful career in computer science.
Whether you're interested in AI, data science, software development, or any other field in computer science, SCOE provides the knowledge, skills, and opportunities you need to succeed. With a strong focus on hands-on learning and personal growth, SCOE ensures that students graduate not only as engineers but as professionals ready to take on the challenges of the tech world.
If you're ready to embark on an exciting journey in the world of technology, consider pursuing your B.Tech in Computer Science and Engineering at SCOE—a college where your future takes shape.
#In today's technology-driven world#pursuing a B.Tech in Computer Science and Engineering (CSE) has become a popular choice among students aspiring for a bright future. The de#Machine Learning#Data Science#and Cloud Computing has made computer science engineering colleges crucial in shaping tomorrow's innovators. Saraswati College of Engineeri#a leader in engineering education#provides students with a perfect platform to build a successful career in this evolving field.#Whether you're passionate about coding#software development#or the latest advancements in AI#pursuing a B.Tech in Computer Science and Engineering at SCOE can open doors to endless opportunities.#Why Choose B.Tech in Computer Science and Engineering?#Choosing a B.Tech in Computer Science and Engineering isn't just about learning to code; it's about mastering problem-solving#logical thinking#and the ability to work with cutting-edge technologies. The course offers a robust foundation that combines theoretical knowledge with prac#enabling students to excel in the tech industry.#At SCOE#the computer science engineering courses are designed to meet industry standards and keep up with the rapidly evolving tech landscape. With#NAAC Accredited With Grade-“A+” credentials#the college provides quality education in a nurturing environment. SCOE's curriculum goes beyond textbooks#focusing on hands-on learning through projects#labs#workshops#and internships. This approach ensures that students graduate not only with a degree but with the skills needed to thrive in their careers.#The Role of Computer Science Engineering Colleges in Career Development#The role of computer science engineering colleges like SCOE is not limited to classroom teaching. These institutions play a crucial role in#faculty expertise#and placement opportunities. SCOE#established in 2004#is recognized as one of the top engineering colleges in Navi Mumbai. It boasts a strong placement record
2 notes
·
View notes
Text
me puttingon the filters "remote" and "data science major" on this job site from my university and just scrolling thru and applying to a ton that don't need cover letter without reading the qualifications/requirements 😀
#data science major is enough LMAO i'm slightly qualified at least right#if they get back to me i can read/reconsider LOL#bitch has to write a goddamn cover letter at some point tho lmfao#tmrw i'm getting together w my friend who also needs to apply for jobs i'll surely write a cover letter then right for shore#bro there's this unpaid internship that is like pretty fitting for me#but it's fucking unpaid which is so annoying lmfao#didn't realize it was unpaid until today (i saw it like yesterday lmao)#anyway looked at my email and reminded that i have to reply to an email abt a job i actualy have rn lmao 😭#and just didn't do this semester#but i want/need to get back to it#but i've spent the last week rotting and doing absolutely nothing#idk where the motivation to do things is gonna come from LOL but we gotta do it#i gotta put work time like scheduled out in my gcal or smth to make myself actually do it :\#and even then it probably won't rly happen lol#ig i spent the last while lying here clicking apply -> submit resume to all these jobs that don't need anything else LOL#so i'm not completely doing nothing 😀#i also have done a bunch of club stuff 😀 for the club that i am no longer director for but ya know#i need to wash my hairrrrrrrrrr lmao ;-;#jeanne talks
2 notes
·
View notes
Text
Data Science Course in Karnal
Boost your career with the DataMites Data Science course in Karnal. Gain hands-on skills through both online and offline classes, along with internship and job assistance. Earn globally recognized certifications from IABAC® and NASSCOM® to enhance your professional credentials.
#data science course#data science certification#data science internship#data science classes in pune
0 notes
Text
Data Science Best Practices for Internship Projects

projects offer an excellent opportunity for aspiring data scientists to gain practical experience, but maximizing this learning experience requires a strategic approach. Below, we will explore best practices to ensure that your data science internship project is successful and provides sInternship ubstantial learning outcomes.rstand the Problem
Before diving into coding or data analysis, it's crucial to thoroughly understand the problem you're working on. Take time to discuss the project with your mentor or supervisor, clarifying the business objectives, and ensuring you comprehend the problem's scope.
Key Questions to Ask:
What are the expected outcomes?
What is the purpose of the data analysis or model?
Who will be using the results?
By framing the problem clearly, you will be able to focus your efforts on producing relevant results.
2. Data Collection and Cleaning
Data science projects heavily depend on the quality of the data. Data collection and cleaning can take up to 80% of your time, and for good reason. Poor quality data can lead to misleading results.
Best Practices for Data Collection:
Make sure the data is from a reliable source.
Check for missing or inconsistent data points.
Format the data consistently.
For cleaning, automate repetitive tasks as much as possible (e.g., missing data imputation, handling outliers) using tools like Python’s pandas or dplyr in R.
3. Exploratory Data Analysis (EDA)
Once you have clean data, perform Exploratory Data Analysis (EDA). EDA helps you understand the data’s underlying patterns, detect outliers, and discover relationships between variables.
Techniques for EDA:
Use summary statistics (mean, median, mode, variance).
Visualize distributions using histograms or box plots.
Plot pairwise relationships between variables with scatterplots or heatmaps.
EDA often reveals unexpected insights, helping refine your hypotheses and approach.
4. Choose the Right Model
Choosing the correct machine learning model is crucial. The right choice depends on your project’s goals, the size of your dataset, and the nature of the data.
Best Practices for Model Selection:
Start with simple models like linear regression or decision trees.
Experiment with complex models such as Random Forest, XGBoost, or deep learning if necessary.
Use cross-validation to ensure the model’s performance generalizes well to new data.
Always evaluate multiple models before finalizing your choice. Performance metrics such as accuracy, precision, recall, and F1-score can help guide your selection.
5. Model Evaluation
Model evaluation is a critical part of the project. Ensure that you're not just selecting a model based on its performance on training data but also how well it performs on unseen data.
Metrics to Consider:
Accuracy (for classification problems).
Mean Squared Error or R-squared (for regression problems).
Use confusion matrices to get detailed insights into classification model performance.
Don’t forget to check for overfitting (when a model performs well on training data but poorly on test data).
6. Document Your Work
Good documentation is key to any data science project, especially for internships where your project might be handed over to someone else. Document every step, including the data preprocessing, modeling techniques, and final decisions.
Best Practices:
Maintain a well-organized notebook (e.g., Jupyter Notebooks).
Use comments in your code to explain what each section does.
Provide justifications for your choices (model, hyperparameters, etc.).
This not only makes your project easy to follow but also shows professionalism and attention to detail.
7. Effective Communication and Visualization
It's essential to communicate your findings effectively. Visualizations are powerful tools to make complex data more digestible. Use tools like Matplotlib, Seaborn, or Plotly for high-quality plots and dashboards.
Key Visualizations:
Use bar charts and line graphs to track trends.
Pie charts or stacked bar charts for composition insights.
Heatmaps for correlation matrices.
Keep in mind who your audience is—your technical audience may appreciate complex metrics, while business stakeholders might prefer clear, actionable insights.
8. Collaboration and Code Sharing
Data science projects often involve collaboration. To make your code easy to share and review, follow best coding practices.
Tools to Consider:
Use Git and GitHub for version control.
Structure your code in modular functions.
Ensure your code is readable and reusable.
You can also make use of tools like Docker for environment replication to ensure that your code runs smoothly in any setting.
9. Managing Time and Expectations
Internship projects are often short-term, making time management critical. Be realistic about what can be accomplished within the available timeframe.
Time Management Tips:
Break the project down into smaller tasks.
Set clear milestones and goals.
Regularly communicate with your mentor or supervisor to stay on track.
10. Final Thoughts
Data science internships are an opportunity to apply theoretical knowledge to real-world problems. By following these best practices, you can deliver a successful project that not only benefits your employer but also enhances your learning experience.
#career#internship#virtualinternship#internshipgate#internship in india#data science#data science internship#data scientist
0 notes