#CO2Emissions
Explore tagged Tumblr posts
Text

Why many scientists are now saying climate change is an all-out ‘emergency’
Escalating rhetoric comes as new study shows there’s just six years left to keep global warming to 1.5 degrees Celsius at current CO2 emissions rate.
37 notes
·
View notes
Link
#Ahmedabad#AsphaltManufacturing#AsphaltProduction#CircularEconomy#CleanTechnology#CO2Emissions#ConstructionInnovation#DustControl#EcoFriendly#EmissionControl#EnergyEfficiency#EnvironmentalImpact#GajjarEquipmentsPrivateLimited’s#GajjarEquipments#GreenConstruction#Gujarat#India#InfrastructureDevelopment#LowTemperatureAsphalt#NoiseReduction#RAP#ReclaimedAsphaltPavement#RenewableResources#SUSTAINABLEASPHALTPLANTMANUFACTURING#SustainableAsphalt#SustainableDevelopment#SustainableInfrastructure#WasteReduction
0 notes
Video
youtube
Will the carbon offset markets be enough to cover 50 Billion Tons of CO2...
#youtube#CarbonOffset#ClimateChange#CarbonMarket#CO2Emissions#EnvironmentalImpact#Sustainability#ClimateSolutions#CarbonNeutral#GreenTechnology#RenewableEnergy#CarbonTrading
0 notes
Text
Early Signs of Spring Becoming New Norm and Citizen Scientists Are Needed to Help Monitor Situation Say Woodland Trust

Woodland Trust warns earlier signs of spring becoming the new norm and is asking volunteers to become citizen scientists to help record data. Read more on Hillingdon Today. #SpringShift #CitizenScience #Citizen #ClimateChange #WoodlandTrust #NatureMonitoring #EcologicalShifts #EarlySpring #VolunteerMonitoring #EnvironmentalAction #ClimateAction #SpringTrends Read the full article
#Citizenscience#Climateaction#Climatechangeimpact#Climateresilience#CO2emissions#Earlysignsofspring#Ecologicalshifts#Environmentalaction#Naturemonitoring#Springindex#Springphenology#Springtrends#Volunteeropportunities#Wildlifemonitoring#WoodlandTrust
0 notes
Text
Attention Israel! Prepare yourselves for an electrifying event as @Make My Day takes the stage at the prestigious Eilat-Eilot Renewable Energy 2024 conference!

📍Dan Hotel, Eilat, February 28-29, 2024
The Eilat-Eilot Conference on Renewable Energy aims to continue to empower the clean energy economy as leverage for regional rehabilitation and development in Israel, the Middle East, and around the world.
@Cnaan Aviv, our CTO & Cofounder, has been recognized as an EV expert and invited to participate in an EV charging panel discussion. He will be addressing the challenges of EV Charging Optimization and showcasing how Make My Day, with its cutting-edge technology in the EV Charging sector, can effectively tackle these issues. Come to hear him on February 29, at 3:30 pm!
Contact us to schedule a meeting: https://lnkd.in/dNNP7UBR Purchase your ticket: https://lnkd.in/d2Xms4aw Event agenda link: https://lnkd.in/dumfGJ8H
#renewableenergy#climatetech#climatecrisis#EnergyConferenceIsrael#Israel#Energy#energy#climatechange#EnergyConference#EilatEilotConference#Eilat#EVChargingOptimization#EV#electricvehicle#co2reduction#co2emissions#FleetEfficiency#CarbonReduction#fleets#Fualmanagement#electricvehicles
0 notes
Photo

Yet the #co2emissions keep being accelerated! :)))) https://www.instagram.com/p/CqahQi0SlbN/?igshid=NGJjMDIxMWI=
0 notes
Text
Mobilità sostenibile

Se vogliamo parlare di mobilità sostenibile diciamo subito che il miglior viaggio e quello evitato. Circa il 25% delle emissioni italiane di gas serra arriva dai trasporti (1.700 kg per persona all’anno). E’ facile produrli, basta bruciare un paio di litri di gasolio (ogni litro genera 2,6 kg di CO2). Se una autovettura fa circa 20 km con un litro di carburante fossile emette circa 130 gr di CO2 al chilometro.
Una valida soluzione di trasporto, per abbassare il nostro impatto ambientale, potrebbe essere l’auto elettrica, solo se verrà caricata con energie rinnovabili e una rigida filiera di riciclo delle batterie esauste.
Poi ci sono i consueti mezzi pubblici: treni, tram, metropolitane, bus. Sono sempre convenienti in termini di basse emissioni per passeggero e per chilometro percorso, anche se qualche volta i prezzi e il livello di servizio non ne incoraggiano l’uso. Ma i migliori mezzi di locomozione sono i cari vecchi piedi e la bicicletta (se volete quella elettrica a pedalata assistita).
Illustrazione di Andy Singer
#auto elettrica#combustibili fossili#decrescita#trasporti#co2emission#mobilità sostenibile#mobilità elettrica#tramvay#gas serra#ambiente#sostenibilità#bicicletta
2 notes
·
View notes
Text
Exploring Global Longevity: Analyzing Life Expectancy and Urbanization Trends Across Nations
I would like to know more about the relation between climate change and urbanization and how this affects people’s lives all around the globe. For this reason, I selected the database from the Gapminder codebook {Gapminder codebook (.pdf)}
Specifically, my Research Question is: Does life expectancy associated with urban rate per country?
So, I decided that I am most interested in exploring environmental factors of urban rate, in this case CO2 emissions and residential electricity consumption, that affect life expectancy dependence.
Sub-research Question: Do environmental factors like CO2 emissions and residential electricity consumption impact life expectancy in urban areas?
The variables of the research questions derived from the Gapminder codebook: co2emissions, lifeexpectancy, relectricperperson, urbanrate. (You can see the image in the end that I created an Excel shit with only these variables).
I have two hypothesis based on the results I found:
1. That the more people are gathered in urban centers, the higher the technological development and the higher the industrialization rates, and this ultimately increases pollution that affects life expectancy in urban areas.
2. A positive relationship between CO2 emissions and life expectancy in West Africa. CO2 emissions may indirectly contribute to improved life expectancy through mechanisms such as enhanced healthcare infrastructure and increased access to medical services facilitated by economic activities associated with CO2 emissions, notably industrialization.
My hypothesis is based on the following literature review:
Elevated CO2 emissions in urban areas are expected to negatively impact life expectancy due to increased pollution. Prolonged exposure to high CO2 levels can result in respiratory and cardiovascular health problems, thereby reducing life expectancy. Additionally, electricity rates may indirectly affect urban CO2 emissions by shaping energy consumption behaviors.https://www.sciencedirect.com/science/article/pii/S2352550921001950
Reducing exposure to ambient fine-particulate air pollution led to notable and measurable enhancements in life expectancy in the United States.https://www.nejm.org/doi/full/10.1056/NEJMsa0805646
The detrimental impact of CO2 emissions on agricultural output, they might indirectly contribute positively to life expectancy in West Africa. Possible explanations for this unexpected relationship include enhancements in healthcare infrastructure and accessibility to medical services driven by economic activities linked to CO2 emissions.https://ojs.jssr.org.pk/index.php/jssr/article/view/115
The study highlights that CO2 emissions negatively impact life expectancy in both Asian and African countries, potentially due to increased urban pollution and deteriorating air quality. Economic progression has a mixed impact on life expectancy, with a negative overall effect but a positive influence observed in the highest economic quantile. This suggests that while economic growth may enhance life expectancy under certain conditions, it can also lead to negative health outcomes in urban areas due to pollution and lifestyle changes.https://ojs.jssr.org.pk/index.php/jssr/article/view/115
2 notes
·
View notes
Text
Descripción de mis datos
Sample
The collect data was taken from GapMonder which is a site that collects data from a handful of sources (Institute for Health Metrics and Evaluation, the US Census Bureau’s International Database, the United Nations Statistics Division, and the World Bank). The sample was of 213 countries with their respective population information data, product per capita, co2 emissions, i.e.
Measures
the characteristics of the variables measured are:
incomeperperson: 2010 Gross Domestic Product per capita in constant 2000 US$. The inflation but not the differences in the cost of living between countries has been taken into account.
alcconsumption: 2008 Alcohol consumption per adult (age 15+), litres Recorded and estimated average alcohol consumption, adult (15+) per capita consumption in litres pure alcohol
armedforcesrate: Armed forces personnel (% of the total labour force)
breastcancerper100TH: 2002 breast cancer new cases per 100,000 females. Number of new cases of breast cancer in 100,000 female residents during a certain year.
co2emissions: 2006 cumulative CO2 emission (metric tons), Total amount of CO2 emission in metric tons since 1751.
femaleemployrate: 2007 female employees age 15+ (% of the population). Percentage of female population, age above 15, that has been employed during the given year.
HIVrate: 2009 estimated HIV Prevalence % - (Ages 15-49). Estimated number of people living with HIV per 100 population of age group 15-49.
Internetuserate: 2010 Internet users (per 100 people). Internet users are people with access to the worldwide network.
lifeexpectancy: 2011 life expectancy at birth (years). The average number of years a newborn child would live if current mortality patterns were to stay the same.
oilperperson: 2010 oil Consumption per capita (tonnes per year and person).
polityscore: 2009 Democracy score (Polity).Overall polity score from the Polity IV dataset, calculated by subtracting an autocracy score from a democracy score. The summary measure of a country's democratic and free nature. -10 is the lowest value, 10 the highest.
relectricperperson 2008 residential electricity consumption, per person (kWh). The amount of residential electricity consumption per person during the given year, counted in kilowatt-hours (kWh).
suicideper100TH: Suicide, age-adjusted, per 100,000 Mortality due to self-inflicted injury, per 100,000 standard population, age-adjusted.
employrate: 2007 total employees age 15+ (% of the population). Percentage of the total p population, age above 15, that has been employed during the given year.
urbanrate: Urban population (% of total) Urban population refers to people living in urban areas as defined by national statistical offices (calculated using World Bank population estimates and urban ratios from the United Nations World Urbanization Prospects)
0 notes
Text
Analysis of some Gapminder data (part4)
In this post I will continue analyzing the chosen variables using at first univariate graph in order to characterize them singularly and in a second time using combined graph (bivariate) in order to evaluate the eventual correlation.
CODE
#import necessary libraries
import pandas as pd import numpy as np import seaborn as sb import matplotlib.pyplot as plt
#import data set
data = pd.read_csv('gapminder.csv', low_memory=False)
#convert necessary variables to numeric values
data['urbanrate']=pd.to_numeric(data['urbanrate'],'coerce') data['co2emissions']=pd.to_numeric(data['co2emissions'],'coerce') data['lifeexpectancy']=pd.to_numeric(data['lifeexpectancy'],'coerce')
#plot univariate variables
sb.displot(data["urbanrate"].dropna(), kde=False) plt.xlabel('Urban rate') plt.ylabel('Urban rate distribution')
#calculate center and spread
print('Urban rate has center =', data['urbanrate'].mean(), 'and variance(spread) =', data['urbanrate'].std() ) print('Life expectancy rate has center =', data['lifeexpectancy'].mean(), 'and variance(spread) =', data['lifeexpectancy'].std() ) print('CO2 has center =', data['co2emissions'].mean(), 'and variance(spread) =', data['co2emissions'].std() )
#co2emissions has a very high range of values,
#therefore it's useful to use its log values instead
data1 = data.copy() data1['co2emissions'] = np.log10(data1['co2emissions']) print('Log(CO2) has center =', data1['co2emissions'].mean(), 'and variance(spread) =', data1['co2emissions'].std() )
sb.displot(data1["co2emissions"].dropna(), kde=False) plt.xlabel('CO2 Emissions') plt.ylabel('CO2 Emission ditribution')
sb.displot(data["lifeexpectancy"].dropna(), kde=False) plt.xlabel('Life expectancy') plt.ylabel('Life expectancy ditribution')
#create graph showing the association between your explanatory
#and response variables (bivariate graph)
sb.relplot(x='urbanrate', y='co2emissions', data=data1) plt.title('CO2 emissions vs co2 emissions')
sb.relplot(x='urbanrate', y='lifeexpectancy', data=data) plt.title('Urban rate vs life expectancy')
sb.relplot(x='co2emissions', y='lifeexpectancy', data=data1) plt.title('CO2 emissions vs life expectancy')
OUTPUT
Urban rate has center = 56.76935960591131 and variance(spread) = 23.844932647298503 Life expectancy rate has center = 69.75352356020943 and variance(spread) = 9.708620536096552 CO2 has center = 5033261621.666663 and variance(spread) = 25738118429.892727 Log(CO2) has center = 8.344214041062171 and variance(spread) = 1.1797927281121965
SUMMARY
Urban rate has an almost symmetrical graph with a very high variance.
Co2 emissions has very skewed values and therefore difficult in interpretation with a graph, therefore I decided to use its logarithm.
Its new graph show a perfectly centered unimodal variable with relative small spread.
Life expectancy has a bimodal distribution.
From the bivariate graphs we can recognize some associations between explanatory and response variables:
We can see that urban rate has an influence on both life expectation and co2 emissions, both increasing with increasing urban rate
In the graph "life expectation vs co2 emissions" we can also see that increasing co2 emission has not a negative influence on life expectation, as it could be expected.
0 notes
Text
Building-integrated Photovoltaics Market 2024 : Industry Analysis, Trends, Segmentation, Regional Overview And Forecast 2033
The building-integrated photovoltaics global market report 2024 from The Business Research Company provides comprehensive market statistics, including global market size, regional shares, competitor market share, detailed segments, trends, and opportunities. This report offers an in-depth analysis of current and future industry scenarios, delivering a complete perspective for thriving in the industrial automation software market.
Building-integrated Photovoltaics Market, 2024 report by The Business Research Company offers comprehensive insights into the current state of the market and highlights future growth opportunities.

Market Size - The building-integrated photovoltaics market size has grown exponentially in recent years. It will grow from $16.65 billion in 2023 to $20.81 billion in 2024 at a compound annual growth rate (CAGR) of 25.0%. The growth in the historic period can be attributed to increased environmental awareness and demand for sustainable building solutions, stricter building energy codes and green building certifications, urbanization and the need for space-efficient renewable energy generation, energy price volatility, and growing investment in research and development of bipv products.
The building-integrated photovoltaics market size is expected to see exponential growth in the next few years. It will grow to $48.44 billion in 2028 at a compound annual growth rate (CAGR) of 23.5%. The growth in the forecast period can be attributed to rising electricity prices and grid resilience considerations, expansion of bipv applications beyond rooftops, growing demand for aesthetically pleasing and customizable bipv solutions, support from architectural and construction industries, and increasing environmental concerns. Major trends in the forecast period include integration of bipv into smart building and iot systems, advances in solar technology, partnerships between solar manufacturers, architects, and construction firms, net-zero energy and carbon-neutral building trends, and technological innovations in bipv materials and designs..
Order your report now for swift delivery @ https://www.thebusinessresearchcompany.com/report/building-integrated-photovoltaics-global-market-report
The Business Research Company's reports encompass a wide range of information, including:
1. Market Size (Historic and Forecast): Analysis of the market's historical performance and projections for future growth.
2. Drivers: Examination of the key factors propelling market growth.
3. Trends: Identification of emerging trends and patterns shaping the market landscape.
4. Key Segments: Breakdown of the market into its primary segments and their respective performance.
5. Focus Regions and Geographies: Insight into the most critical regions and geographical areas influencing the market.
6. Macro Economic Factors: Assessment of broader economic elements impacting the market.
Market Drivers - The increasing demand for zero-energy buildings is expected to fuel the growth of the building-integrated photovoltaics market going forward. Zero-energy buildings combine energy efficiency and renewable energy generation to consume only the amount of energy that can be produced onsite using renewable resources over a certain period of time. The global concern regarding the carbon emissions from buildings and buildings construction is the rising demand for zero-energy buildings. Building-integrated photovoltaics materials provide a reasonable option for building skins and help in achieving zero energy levels in buildings. For instance, in November 2022, according to United Nations Environment Programme, investments in building energy efficiency rose by 16% to reach USD 237 billion, but the expansion of floor space outstripped the progress in energy efficiency measures globally. The sector’s 2021 operational energy-related CO2emissions were up 5 per cent over 2020 and 2 per cent over the pre-pandemic peak in 2019. Therefore, the growing demand for zero-energy buildings will contribute to the growth of the building-integrated photovoltaics market.
The building-integrated photovoltaics market covered in this report is segmented –
1) By Product Type: Polyscrystaline, Thin-Film, Other Product Types 2) By Application: Roof, Facade, Glass, Other Applications 3) By End-User: Residential, Commercial, Industrial
Get an inside scoop of the building-integrated photovoltaics market, Request now for Sample Report @ https://www.thebusinessresearchcompany.com/sample.aspx?id=5922&type=smp
Regional Insights - Asia-Pacific was the largest region in the building-integrated photovoltaics market in 2023. The regions covered in the building-integrated photovoltaics market report include Asia-Pacific, Western Europe, Eastern Europe, North America, South America, Middle East and Africa.
Key Companies - Major companies operating in the building-integrated photovoltaics market include First Solar Inc., Sharp Corporation, Yingli Solar, Solar Frontier, SunPower Corporation, Hanwha Solar, Renewable Energy Corporation, Panasonic Corporation, Kyocera Document Solutions Company, Canadian Solar Inc., Suntech Power Holdings Co. Ltd., Harsha Abakus Solar Private Limited, Ascent Solar Technologies Inc., Greatcell Energy Limited, Global Solar Energy Inc., Hanergy Holding Group Ltd., Onyx Solar Energy S.L., ISSOL SA, Solarcentury Holdings Limited, Trina Solar Limited, Hanwha Q CELLS Co. Ltd., GAF Energy LLC, RGS Energy, NanoFlex Power Corporation, Tesla Inc., Jinko Solar Holding Co. Ltd., LG Electronics Inc., SolarWorld Americas Inc., Sanyo Electric Co. Ltd., Mitsubishi Electric Corporation, SolarCity Corporation, REC Solar Holdings AS, Enphase Energy Inc., SolarEdge Technologies Inc., SMA Solar Technology AG, ABB Ltd., Schneider Electric SE, Siemens AG, General Electric Company
Table of Contents 1. Executive Summary 2. Building-integrated Photovoltaics Market Report Structure 3. Building-integrated Photovoltaics Market Trends And Strategies 4. Building-integrated Photovoltaics Market – Macro Economic Scenario 5. Building-integrated Photovoltaics Market Size And Growth ….. 27. Building-integrated Photovoltaics Market Competitor Landscape And Company Profiles 28. Key Mergers And Acquisitions 29. Future Outlook and Potential Analysis 30. Appendix
Contact Us:
The Business Research Company
Europe: +44 207 1930 708
Asia: +91 88972 63534
Americas: +1 315 623 0293
Email: [email protected]
Follow Us On:
LinkedIn: https://in.linkedin.com/company/the-business-research-company
Twitter: https://twitter.com/tbrc_info
Facebook: https://www.facebook.com/TheBusinessResearchCompany
YouTube: https://www.youtube.com/channel/UC24_fI0rV8cR5DxlCpgmyFQ
Blog: https://blog.tbrc.info/
Healthcare Blog: https://healthcareresearchreports.com/
Global Market Model: https://www.thebusinessresearchcompany.com/global-market-model
0 notes
Text
🌱Sustainable Living: Practical Tips for Adopting Eco-Friendly Habits🚴
🌍 Introduction
Sustainable living isn't just a trend; it's a necessity in our fast-paced, resource-consuming world. With climate change becoming a pressing issue, adopting eco-friendly habits has never been more crucial. This article provides practical tips for embracing sustainable living, backed by the latest data, historical context, and actionable solutions to overcome challenges. Let’s dive into how we can make a positive impact on the planet.
#SustainableLiving #EcoFriendly #ClimateAction #GoGreen #Sustainability
🛠️ 1. Understanding the Need for Sustainable Living
Historical Context: The concept of sustainability dates back to the early 20th century, but it gained momentum during the environmental movements of the 1960s and 70s. The 1987 Brundtland Report defined sustainable development as meeting the needs of the present without compromising the ability of future generations to meet their own needs.
Current Situation: In 2023, global CO2 emissions hit a record high of 40.6 billion tonnes. The Earth Overshoot Day, the date when humanity's demand for ecological resources exceeds what the Earth can regenerate in that year, occurred on July 28, 2023. This highlights the urgent need for sustainable living practices.
#ClimateChange #GlobalWarming #CO2Emissions
🔍 2. Key Factors Driving Sustainable Living
Environmental Degradation: Deforestation, pollution, and loss of biodiversity are critical issues.
Resource Depletion: Overuse of natural resources like water, fossil fuels, and minerals is unsustainable.
Climate Change: Increasing global temperatures and extreme weather events are direct results of unsustainable practices.
Health Concerns: Pollution and environmental toxins are linked to various health problems, driving people towards greener lifestyles.
#Biodiversity #Deforestation #ResourceDepletion #HealthAndEnvironment
📊 3. Challenges in Adopting Sustainable Habits
Awareness Gap: Many people lack knowledge about sustainable practices.
Economic Barriers: Eco-friendly products often come with higher upfront costs.
Habitual Resistance: Breaking old, unsustainable habits can be difficult.
Infrastructure Limitations: Lack of access to sustainable options like public transportation or recycling facilities.
Data Insight: According to a 2023 study by the World Resources Institute, 65% of consumers in developed countries are willing to adopt sustainable habits, but only 26% actually do so, primarily due to economic and infrastructural barriers.
#SustainabilityChallenges #EcoAwareness #GreenEconomy
💡 4. Practical Tips for Sustainable Living
Reduce, Reuse, Recycle: Start by reducing waste. Reuse what you can and recycle properly. Simple steps like avoiding single-use plastics can make a big difference.
Energy Efficiency: Switch to energy-efficient appliances and LED bulbs. Consider solar panels for renewable energy.
Sustainable Transportation: Use public transportation, carpool, or opt for biking and walking. Electric vehicles (EVs) are also a greener alternative.
Conscious Consumption: Buy local, organic, and seasonal food. Reduce meat consumption, as the meat industry is a significant contributor to greenhouse gas emissions.
Water Conservation: Install water-saving fixtures and practice mindful water usage, like turning off the tap while brushing your teeth.
#GreenLiving #EnergyEfficiency #ZeroWaste #ConsciousConsumption #WaterConservation
🔄 5. Overcoming Challenges: Solutions for Sustainable Living
Education and Awareness: Governments and NGOs should focus on educating the public about the importance and methods of sustainable living.
Incentives for Green Choices: Providing financial incentives for using renewable energy or purchasing eco-friendly products can encourage more people to go green.
Community Initiatives: Local communities can promote sustainability by organizing events like clean-up drives and eco-workshops.
Policy Support: Governments need to enforce regulations that promote sustainability, such as carbon taxes and bans on single-use plastics.
Case Study: Sweden has implemented a carbon tax since 1991, leading to a significant reduction in carbon emissions. As a result, the country is on track to achieve net-zero emissions by 2045.
#EcoEducation #GreenIncentives #CommunitySustainability #PolicyForChange
🚀 6. The Way Forward: Building a Sustainable Future
To build a sustainable future, it's essential to take collective action. Individuals, communities, businesses, and governments must work together to adopt and promote eco-friendly practices. The shift towards sustainability is not just a choice but a responsibility we owe to future generations.
Action Steps:
Support Green Businesses: Choose products from companies that prioritize sustainability.
Advocate for Policy Changes: Participate in campaigns and vote for leaders committed to environmental policies.
Lead by Example: Adopt sustainable habits in your own life and inspire others to follow.
#FutureOfSustainability #CollectiveAction #GreenLeadership
📚 References and Sources
World Resources Institute (2023) - Sustainable Living Study
UN Environment Programme (2023) - Global CO2 Emissions Report
Swedish Carbon Tax Success Story - Government of Sweden
Earth Overshoot Day (2023) - Global Footprint Network
🌟 Conclusion
Sustainable living is about making conscious choices that benefit both the planet and our well-being. By understanding the importance, overcoming challenges, and adopting practical tips, we can all contribute to a greener, healthier world. Start small, stay consistent, and watch the impact grow!
#SustainableFuture #EcoFriendlyHabits #ClimateActionNow #SustainabilityJourney
Disclaimer: This content is accurate and true to the best of the author’s knowledge and is not meant to substitute for formal and individualized advice from a qualified professional.
© 2024 ANUJ VIJLANI
1 note
·
View note
Text
Sir Keir Starmer promises 'all we can' to save Port Talbot jobs
Sir Keir Starmer promises 'all we can' to save Port Talbot jobs #aviationsector #CO2emissions #electricarcfurnaces
#aviation sector#CO2 emissions#electric arc furnaces#GB Energy#government funding#green agenda#job losses#Keir Starmer#London City Airport#Port Talbot#redundancies#South Wales#steelworks#Tata Steel#wind farm
0 notes
Text
Univariate and bivariate graphs
The code is the following:
import pandas as pd
import numpy as np
import seaborn as sns
import matplotlib.pyplot as plt
#Import data
data = pd.read_csv('gapminder_pds_2.csv', low_memory = False)
# Tranform data to numerical
data['incomeperperson'] = pd.to_numeric(data['incomeperperson'], errors='coerce')
data['breastcancerper100th'] = pd.to_numeric(data['breastcancerper100th'], errors='coerce')
data['lifeexpectancy'] = pd.to_numeric(data['lifeexpectancy'], errors='coerce')
data['co2emissions'] = pd.to_numeric(data['co2emissions'], errors='coerce')
data['relectricperperson'] = pd.to_numeric(data['relectricperperson'], errors = 'coerce')
# ----- Describe for income per person ------
d_inc = data['incomeperperson'].describe()
#print(d_inc)
data['income_quart'] = pd.qcut(data["incomeperperson"], 4, labels=["1=25% ~ 748.24","2=50% ~ 2553.49","3=75% ~ 9379.89","4=100% ~ 105147.4377"])
q1 = data['income_quart'].value_counts(sort=False,dropna=True)
#print(q1)
plt.figure(figsize=(8, 6))
sns.histplot(data['incomeperperson'].dropna(), kde=False, bins = 20); # --- Histogram --
plt.xlabel('Income per person')
plt.title('Income per person among the Countries')
# ----- Describe for breastcancerper100th ------
d_brcanc = data['breastcancerper100th'].describe()
#print(d_brcanc)
plt.figure(figsize=(8, 6))
sns.histplot(data['breastcancerper100th'].dropna(), kde=False, bins = 20); # --- Histogram --
plt.xlabel('Breast cancer per 100th')
plt.title('Breast cancer per 100th among the Countries')
# ----- Describe for co2emissions ------
d_co2 = data['co2emissions'].describe()
#print(d_brcanc)
plt.figure(figsize=(8, 6))
sns.histplot(data['co2emissions'].dropna(), kde=False, bins = 10); # --- Histogram --
plt.xlabel('Co2 Emissions')
plt.title('Co2 emissions among the Countries')
# ----- Describe for lifeexpectancy ------
d_life = data['lifeexpectancy'].describe()
#print(d_brcanc)
#plt.figure(figsize=(8, 6))
#sns.histplot(data['lifeexpectancy'].dropna(), kde=False, bins = 10); # --- Histogram --
#plt.xlabel('Life Expectancy')
#plt.title('Life expectancy among the Countries')
# ----- Describe for relectricperperson ------
d_elect = data['relectricperperson'].describe()
#print(d_brcanc)
plt.figure(figsize=(8, 6))
sns.histplot(data['relectricperperson'].dropna(), kde=False, bins = 15); # --- Histogram --
plt.xlabel('relectric per person')
plt.title('relectric per person among the Countries')
# ----- First scatter plots co2 emission & income ------
plt.figure(figsize=(8, 6))
scat_co2_income = sns.regplot(x="co2emissions",y="incomeperperson", data=data)
plt.xlabel('co2emissions')
plt.ylabel('income per person')
data['C02GRP4'] = pd.cut(data.co2emissions,4, labels=["1=25th","2=50th","3=75th","4=100th"])
c10 = data['C02GRP4'].value_counts(sort=False, dropna=True)
#print(c10)
sns.catplot(x='C02GRP4', y='incomeperperson', data=data, kind="bar", ci=None)
plt.xlabel('co2 emissions group')
plt.ylabel('mean income per person')
# ----- Second scatter plots co2 emission & breast cancer ------
plt.figure(figsize=(8, 6))
scat_co2_breastcancer = sns.regplot(x="co2emissions",y="breastcancerper100th", data=data)
plt.xlabel('co2emissions')
plt.ylabel('breast cancer')
sns.catplot(x='C02GRP4', y='breastcancerper100th', data=data, kind="bar", ci=None)
plt.xlabel('co2 emissions group')
plt.ylabel('mean breast cancer per 100th')
# ----- Third scatter plots co2 emission & life expectancy ------
plt.figure(figsize=(8, 6))
scat_co2_income = sns.regplot(x="co2emissions",y="lifeexpectancy", data=data)
plt.xlabel('co2emissions')
plt.ylabel('lifeexpectancy')
sns.catplot(x='C02GRP4', y='lifeexpectancy', data=data, kind="bar", ci=None)
plt.xlabel('co2 emissions group')
plt.ylabel('mean life expectancy')
# ----- Fourth scatter plots co2 emission & relectricperperson ------
plt.figure(figsize=(8, 6))
scat_co2_income = sns.regplot(x="co2emissions",y="relectricperperson", data=data)
plt.xlabel('co2emissions')
plt.ylabel('relectricperperson')
sns.catplot(x='C02GRP4', y='relectricperperson', data=data, kind="bar", ci=None)
plt.xlabel('co2 emissions group')
plt.ylabel('mean electric per person')
For every scatter plot that has been created, the dots were gathered on the one side of the graph. For this reason, and in order to have a better sense whether the variables have a relationship, categorization has been implemented. Finally it appeared that there is a relationship between the variables as follows:
Countries with high income have high CO2 emissions.
High breast cancer is seen in countries with high CO2 emissions.
High life expectancy is seen in countries with high CO2 emissions. But the differences are not great between countries.
Electricity consumption is higher in countries with high CO2 emissions.
0 notes
Text
Peer-graded Assignment: Making Data Management Decisions
#Import necessary libraries
import pandas as pd import numpy as np
#Load the dataset from a CSV file
data = pd.read_csv("gapminder.csv")
#Convert specific columns to numeric type to ensure they can be analyzed correctly
columns_to_convert = [ 'incomeperperson', 'alcconsumption', 'armedforcesrate', 'breastcancerper100th', 'co2emissions', 'femaleemployrate', 'hivrate', 'internetuserate', 'lifeexpectancy', 'oilperperson', 'polityscore', 'relectricperperson', 'suicideper100th', 'employrate', 'urbanrate' ]
for column in columns_to_convert: data[column] = pd.to_numeric(data[column], errors='coerce')
#Handle missing values: fill with the mean of the column (alternative strategies could be used)
data.fillna(data.mean(), inplace=True)
#Function to print frequency distributions for selected columns
def print_frequency_distributions(columns): for column in columns: value_counts = data[column].value_counts(sort=False) normalized_counts = data[column].value_counts(sort=False, normalize=True) print(f"Frequency distribution for {column}:") print(value_counts) print(f"Normalized frequency distribution for {column}:") print(normalized_counts) print("\n")
#Generate and print frequency distributions for chosen variables
chosen_columns = [ 'incomeperperson', 'alcconsumption', 'armedforcesrate', 'breastcancerper100th', 'co2emissions', 'femaleemployrate', 'hivrate', 'internetuserate', 'lifeexpectancy', 'oilperperson', 'polityscore', 'relectricperperson', 'suicideper100th', 'employrate', 'urbanrate' ]
print_frequency_distributions(chosen_columns)
#Select specific columns and rows for further analysis
#Here, we'll select columns related to economic and health metrics for countries with high income per person
selected_columns = [ 'country', 'incomeperperson', 'lifeexpectancy', 'co2emissions', 'alcconsumption' ]
#Define a threshold for high income per person (e.g., income greater than 40,000)
high_income_threshold = 40000 selected_rows = data[data['incomeperperson'] > high_income_threshold]
#Display the selected data
selected_data = selected_rows[selected_columns] print("Selected data for further analysis (countries with high income per person):") print(selected_data)
0 notes
Text
Make My Day is thrilled to announce our recent elevation to the #Standard tier partnership in the GEOTAB #Marketplace!
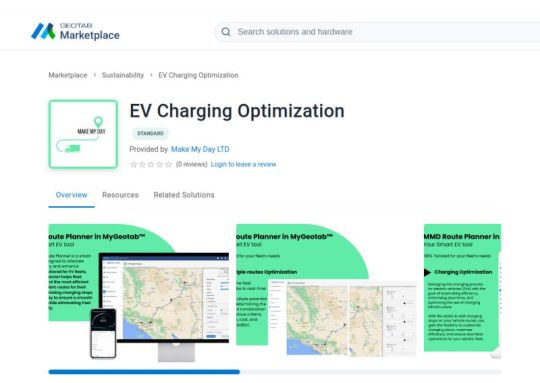
As a Standard Marketplace partner, we have diligently crafted tailor-made and customized integrations exclusively for GEOTAB resellers, enriching the core GEOTAB experience. Rest assured, our solutions adhere to the highest Technical, Legal, and Security industry standards. We invite you to order today our smart #EVChargingOptimization solution that is now available in the GEOTAB marketplace: https://lnkd.in/dMYQK57F
#Decarbonization#ElectricVehicles#Charging#Sustainability#GreenFleet#GEOTAB#FuelManagement#Fleet#Fleets#Carbonfootprint#co2reduction#co2emissions#FleetEfficiency#CarbonReduction#GeotabMarketplace#fleets#EV#Fualmanagement#electricvehicles#geotab#CO2
0 notes