#Biglake
Explore tagged Tumblr posts
Text
BigQuery Studio From Google Cloud Accelerates AI operations

Google Cloud is well positioned to provide enterprises with a unified, intelligent, open, and secure data and AI cloud. Dataproc, Dataflow, BigQuery, BigLake, and Vertex AI are used by thousands of clients in many industries across the globe for data-to-AI operations. From data intake and preparation to analysis, exploration, and visualization to ML training and inference, it presents BigQuery Studio, a unified, collaborative workspace for Google Cloud’s data analytics suite that speeds up data to AI workflows. It enables data professionals to:
Utilize BigQuery’s built-in SQL, Python, Spark, or natural language capabilities to leverage code assets across Vertex AI and other products for specific workflows.
Improve cooperation by applying best practices for software development, like CI/CD, version history, and source control, to data assets.
Enforce security standards consistently and obtain governance insights within BigQuery by using data lineage, profiling, and quality.
The following features of BigQuery Studio assist you in finding, examining, and drawing conclusions from data in BigQuery:
Code completion, query validation, and byte processing estimation are all features of this powerful SQL editor.
Colab Enterprise-built embedded Python notebooks. Notebooks come with built-in support for BigQuery DataFrames and one-click Python development runtimes.
You can create stored Python procedures for Apache Spark using this PySpark editor.
Dataform-based asset management and version history for code assets, including notebooks and stored queries.
Gemini generative AI (Preview)-based assistive code creation in notebooks and the SQL editor.
Dataplex includes for data profiling, data quality checks, and data discovery.
The option to view work history by project or by user.
The capability of exporting stored query results for use in other programs and analyzing them by linking to other tools like Looker and Google Sheets.
Follow the guidelines under Enable BigQuery Studio for Asset Management to get started with BigQuery Studio. The following APIs are made possible by this process:
To use Python functions in your project, you must have access to the Compute Engine API.
Code assets, such as notebook files, must be stored via the Dataform API.
In order to run Colab Enterprise Python notebooks in BigQuery, the Vertex AI API is necessary.
Single interface for all data teams
Analytics experts must use various connectors for data intake, switch between coding languages, and transfer data assets between systems due to disparate technologies, which results in inconsistent experiences. The time-to-value of an organization’s data and AI initiatives is greatly impacted by this.
By providing an end-to-end analytics experience on a single, specially designed platform, BigQuery Studio tackles these issues. Data engineers, data analysts, and data scientists can complete end-to-end tasks like data ingestion, pipeline creation, and predictive analytics using the coding language of their choice with its integrated workspace, which consists of a notebook interface and SQL (powered by Colab Enterprise, which is in preview right now).
For instance, data scientists and other analytics users can now analyze and explore data at the petabyte scale using Python within BigQuery in the well-known Colab notebook environment. The notebook environment of BigQuery Studio facilitates data querying and transformation, autocompletion of datasets and columns, and browsing of datasets and schema. Additionally, Vertex AI offers access to the same Colab Enterprise notebook for machine learning operations including MLOps, deployment, and model training and customisation.
Additionally, BigQuery Studio offers a single pane of glass for working with structured, semi-structured, and unstructured data of all types across cloud environments like Google Cloud, AWS, and Azure by utilizing BigLake, which has built-in support for Apache Parquet, Delta Lake, and Apache Iceberg.
One of the top platforms for commerce, Shopify, has been investigating how BigQuery Studio may enhance its current BigQuery environment.
Maximize productivity and collaboration
By extending software development best practices like CI/CD, version history, and source control to analytics assets like SQL scripts, Python scripts, notebooks, and SQL pipelines, BigQuery Studio enhances cooperation among data practitioners. To ensure that their code is always up to date, users will also have the ability to safely link to their preferred external code repositories.
BigQuery Studio not only facilitates human collaborations but also offers an AI-powered collaborator for coding help and contextual discussion. BigQuery’s Duet AI can automatically recommend functions and code blocks for Python and SQL based on the context of each user and their data. The new chat interface eliminates the need for trial and error and document searching by allowing data practitioners to receive specialized real-time help on specific tasks using natural language.
Unified security and governance
By assisting users in comprehending data, recognizing quality concerns, and diagnosing difficulties, BigQuery Studio enables enterprises to extract reliable insights from reliable data. To assist guarantee that data is accurate, dependable, and of high quality, data practitioners can profile data, manage data lineage, and implement data-quality constraints. BigQuery Studio will reveal tailored metadata insights later this year, such as dataset summaries or suggestions for further investigation.
Additionally, by eliminating the need to copy, move, or exchange data outside of BigQuery for sophisticated workflows, BigQuery Studio enables administrators to consistently enforce security standards for data assets. Policies are enforced for fine-grained security with unified credential management across BigQuery and Vertex AI, eliminating the need to handle extra external connections or service accounts. For instance, Vertex AI’s core models for image, video, text, and language translations may now be used by data analysts for tasks like sentiment analysis and entity discovery over BigQuery data using straightforward SQL in BigQuery, eliminating the need to share data with outside services.
Read more on Govindhtech.com
#BigQueryStudio#BigLake#AIcloud#VertexAI#BigQueryDataFrames#generativeAI#ApacheSpark#MLOps#news#technews#technology#technologynews#technologytrends#govindhtech
0 notes
Text
Big Lake RV Camping: Safety Tips and Emergency Preparedness

Image Credit : https://litsupervs.best/
Big Lake, with its serene waters and picturesque surroundings, is a dream destination for RV camping enthusiasts. As you gear up for your next adventure at Big Lake, it's crucial to prioritize safety and be prepared for any emergencies that may arise. Whether you're a seasoned camper or a novice explorer, these safety tips and emergency preparedness guidelines will ensure a smooth and enjoyable experience.
1. Planning Your Trip
Before hitting the road to Big Lake, thorough planning is the key. Research the area's climate, terrain, and potential hazards to anticipate any challenges you might encounter. Check weather forecasts regularly and be prepared for sudden changes in conditions, especially during winter months when snowstorms are common. Additionally, familiarize yourself with the layout of the campground and locate the nearest emergency services.
2. Equipment and Gear
Having the right equipment and gear can make all the difference in ensuring your safety during RV camping. Ensure your RV is well-maintained and equipped with essentials such as first aid kits, fire extinguishers, and emergency contact information. Pack appropriate clothing and footwear for the prevailing weather conditions, including rain gear and layers for cold temperatures. Don't forget to bring along a reliable GPS device or map of the area to navigate unfamiliar terrain.
3. Water Safety
Big Lake offers ample opportunities for water-based activities, including boating and fishing. If you plan to venture out on the lake, prioritize water safety measures. Ensure all passengers wear properly fitting life jackets, regardless of their swimming abilities. Familiarize yourself with local boating regulations and adhere to speed limits and navigational rules. Before setting sail, inspect your boat for any signs of damage and carry essential safety equipment such as flares and a can be thrown flotation device.
4. Emergency Communication
Effective communication is essential in any emergency situation. Before embarking on your RV camping trip, establish a communication plan with your travel companions and designate meeting points in case you get separated. Ensure your mobile phone is fully charged and consider bringing along a portable charger or solar-powered device for extended trips. In areas with limited cell service, invest in a two-way radio or satellite phone for reliable communication with emergency services.
5. Fire Safety
Campfires are a quintessential part of the RV camping experience, but they also pose a potential fire hazard if not managed properly. When building a campfire, choose a designated fire pit away from overhanging branches and flammable materials. Keep a bucket of water or fire extinguisher nearby for extinguishing embers, and never leave the fire unattended.
6. Emergency Preparedness
Despite your best efforts to stay safe, emergencies can still occur during RV camping trips. Be prepared to handle common emergencies such as medical incidents, vehicle breakdowns, or inclement weather events. Pack a comprehensive first aid kit and familiarize yourself with basic first aid procedures. Consider taking a wilderness first aid course to enhance your emergency response skills.
Conclusion
The Big Lake RV camping is offering unparalleled opportunities for adventure and relaxation amid the beauty of nature. By prioritizing safety and emergency preparedness, you can enjoy a worry-free experience and create lasting memories with your loved ones. Remember to plan ahead, equip yourself with the necessary gear, and stay vigilant to potential hazards. With these tips in mind, you're ready to embark on an unforgettable journey at Big Lake.
You may also read :
0 notes
Text

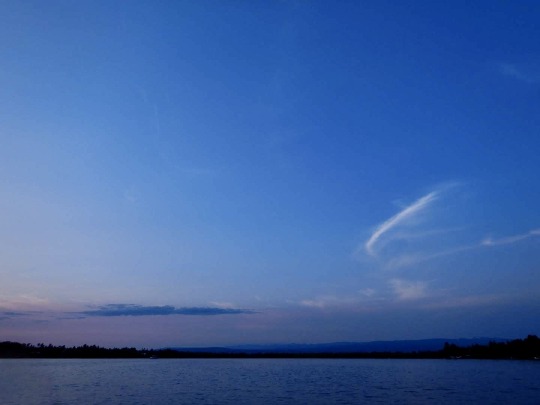
Late night sunsets are so nostalgic to me.
Horseshoe Lake, Alaska
July 6th, 2018 @ 11:02pm.
0 notes
Link
Integrating BigQuery and Apache Iceberg Tables provides several benefits, including lower cost and more efficient data management, helping to improve the performance of data queries, easier data versioning & tracking, and improving scalability and flexibility. It is very good news for BigQuery users.
0 notes
Photo

„Loch Ness” Fine Art Giclée Print • 63x43cm (passend geschnitten für 70x50 Rahmen) • Unikat • Zertifikat • nummeriert • handsigniert • Papier: Hahnemühle German Etching 310g • www.glezfineart.com . . . . . #fineart #gicleeprint #kunstdruck #unikat #einzelstück #kunst #wandbild #wallart #itsakaiser #oneofakind #schwarzweiss #blackandwhite #loch #ness #scottishhighlands #hahnemühle #hahnemuhlepaper #Schottland #biglake #scotland #passion_in_bnw #lochness #minimalismus #minimalism #bwlove (at Loch Ness, Scotland) https://www.instagram.com/p/Ck5QcoLIQtj/?igshid=NGJjMDIxMWI=
#fineart#gicleeprint#kunstdruck#unikat#einzelstück#kunst#wandbild#wallart#itsakaiser#oneofakind#schwarzweiss#blackandwhite#loch#ness#scottishhighlands#hahnemühle#hahnemuhlepaper#schottland#biglake#scotland#passion_in_bnw#lochness#minimalismus#minimalism#bwlove
0 notes
Link
#BigData#DataLake#архитектура#Большиеданные#облака#обработкаданных#Цифроваятрансформация#цифровизация
0 notes
Text
BigLake Tables: Future of Unified Data Storage And Analytics

Introduction BigLake external tables
This article introduces BigLake and assumes database tables and IAM knowledge. To query data in supported data storage, build BigLake tables and query them using GoogleSQL:
Create Cloud Storage BigLake tables and query.
Create BigLake tables in Amazon S3 and query.
Create Azure Blob Storage BigLake tables and query.
BigLake tables provide structured data queries in external data storage with delegation. Access delegation separates BigLake table and data storage access. Data store connections are made via service account external connections. Users only need access to the BigLake table since the service account retrieves data from the data store. This allows fine-grained table-level row- and column-level security. Dynamic data masking works for Cloud Storage-based BigLake tables. BigQuery Omni explains multi-cloud analytic methods integrating BigLake tables with Amazon S3 or Blob Storage data.
Support for temporary tables
BigLake Cloud Storage tables might be temporary or permanent.
Amazon S3/Blob Storage BigLake tables must last.
Source files multiple
Multiple external data sources with the same schema may be used to generate a BigLake table.
Cross-cloud connects
Query across Google Cloud and BigQuery Omni using cross-cloud joins. Google SQL JOIN can examine data from AWS, Azure, public datasets, and other Google Cloud services. Cross-cloud joins prevent data copying before queries.
BigLake table may be used in SELECT statements like any other BigQuery table, including in DML and DDL operations that employ subqueries to obtain data. BigQuery and BigLake tables from various clouds may be used in the same query. BigQuery tables must share a region.
Cross-cloud join needs permissions
Ask your administrator to give you the BigQuery Data Editor (roles/bigquery.dataEditor) IAM role on the project where the cross-cloud connect is done. See Manage project, folder, and organization access for role granting.
Cross-cloud connect fees
BigQuery splits cross-cloud join queries into local and remote portions. BigQuery treats the local component as a regular query. The remote portion constructs a temporary BigQuery table by performing a CREATE TABLE AS SELECT (CTAS) action on the BigLake table in the BigQuery Omni region. This temporary table is used for your cross-cloud join by BigQuery, which deletes it after eight hours.
Data transmission expenses apply to BigLake tables. BigQuery reduces these expenses by only sending the BigLake table columns and rows referenced in the query. Google Cloud propose a thin column filter to save transfer expenses. In your work history, the CTAS task shows the quantity of bytes sent. Successful transfers cost even if the primary query fails.
One transfer is from an employees table (with a level filter) and one from an active workers table. BigQuery performs the join after the transfer. The successful transfer incurs data transfer costs even if the other fails.
Limits on cross-cloud join
The BigQuery free tier and sandbox don’t enable cross-cloud joins.
A query using JOIN statements may not push aggregates to BigQuery Omni regions.
Even if the identical cross-cloud query is repeated, each temporary table is utilized once.
Transfers cannot exceed 60 GB. Filtering a BigLake table and loading the result must be under 60 GB. You may request a greater quota. No restriction on scanned bytes.
Cross-cloud join queries have an internal rate limit. If query rates surpass the quota, you may get an All our servers are busy processing data sent between regions error. Retrying the query usually works. Request an internal quota increase from support to handle more inquiries.
Cross-cloud joins are only supported in colocated BigQuery regions, BigQuery Omni regions, and US and EU multi-regions. Cross-cloud connects in US or EU multi-regions can only access BigQuery Omni data.
Cross-cloud join queries with 10+ BigQuery Omni datasets may encounter the error “Dataset was not found in location “. When doing a cross-cloud join with more than 10 datasets, provide a location to prevent this problem. If you specifically select a BigQuery region and your query only includes BigLake tables, it runs as a cross-cloud query and incurs data transfer fees.
Can’t query _FILE_NAME pseudo-column with cross-cloud joins.
WHERE clauses cannot utilize INTERVAL or RANGE literals for BigLake table columns.
Cross-cloud join operations don’t disclose bytes processed and transmitted from other clouds. Child CTAS tasks produced during cross-cloud query execution have this information.
Only BigQuery Omni regions support permitted views and procedures referencing BigQuery Omni tables or views.
No pushdowns are performed to remote subqueries in cross-cloud queries that use STRUCT or JSON columns. Create a BigQuery Omni view that filters STRUCT and JSON columns and provides just the essential information as columns to enhance speed.
Inter-cloud joins don’t allow time travel queries.
Connectors
BigQuery connections let you access Cloud Storage-based BigLake tables from other data processing tools. BigLake tables may be accessed using Apache Spark, Hive, TensorFlow, Trino, or Presto. The BigQuery Storage API enforces row- and column-level governance on all BigLake table data access, including connectors.
In the diagram below, the BigQuery Storage API allows Apache Spark users to access approved data:Image Credit To Google Cloud
The BigLake tables on object storage
BigLake allows data lake managers to specify user access limits on tables rather than files, giving them better control.
Google Cloud propose utilizing BigLake tables to construct and manage links to external object stores because they simplify access control.
External tables may be used for ad hoc data discovery and modification without governance.
Limitations
BigLake tables have all external table constraints.
BigQuery and BigLake tables on object storage have the same constraints.
BigLake does not allow Dataproc Personal Cluster Authentication downscoped credentials. For Personal Cluster Authentication, utilize an empty Credential Access Boundary with the “echo -n “{}” option to inject credentials.
Example: This command begins a credential propagation session in myproject for mycluster:
gcloud dataproc clusters enable-personal-auth-session \ --region=us \ --project=myproject \ --access-boundary=<(echo -n "{}") \ mycluster
The BigLake tables are read-only. BigLake tables cannot be modified using DML or other ways.
These formats are supported by BigLake tables:
Avro
CSV
Delta Lake
Iceberg
JSON
ORC
Parquet
BigQuery requires Apache Iceberg’s manifest file information, hence BigLake external tables for Apache Iceberg can’t use cached metadata.
AWS and Azure don’t have BigQuery Storage API.
The following limits apply to cached metadata:
Only BigLake tables that utilize Avro, ORC, Parquet, JSON, and CSV may use cached metadata.
Amazon S3 queries do not provide new data until the metadata cache refreshes after creating, updating, or deleting files. This may provide surprising outcomes. After deleting and writing a file, your query results may exclude both the old and new files depending on when cached information was last updated.
BigLake tables containing Amazon S3 or Blob Storage data cannot use CMEK with cached metadata.
Secure model
Managing and utilizing BigLake tables often involves several organizational roles:
Managers of data lakes. Typically, these administrators administer Cloud Storage bucket and object IAM policies.
Data warehouse managers. Administrators usually edit, remove, and create tables.
A data analyst. Usually, analysts read and query data.
Administrators of data lakes create and share links with data warehouse administrators. Data warehouse administrators construct tables, configure restricted access, and share them with analysts.
Performance metadata caching
Cacheable information improves BigLake table query efficiency. Metadata caching helps when dealing with several files or hive partitioned data. BigLake tables that cache metadata include:
Amazon S3 BigLake tables
BigLake cloud storage
Row numbers, file names, and partitioning information are included. You may activate or disable table metadata caching. Metadata caching works well for Hive partition filters and huge file queries.
Without metadata caching, table queries must access the external data source for object information. Listing millions of files from the external data source might take minutes, increasing query latency. Metadata caching lets queries split and trim files faster without listing external data source files.
Two properties govern this feature:
Cache information is used when maximum staleness is reached.
Metadata cache mode controls metadata collection.
You set the maximum metadata staleness for table operations when metadata caching is enabled. If the interval is 1 hour, actions against the table utilize cached information if it was updated within an hour. If cached metadata is older than that, Amazon S3 or Cloud Storage metadata is retrieved instead. Staleness intervals range from 30 minutes to 7 days.
Cache refresh may be done manually or automatically:
Automatic cache refreshes occur at a system-defined period, generally 30–60 minutes. If datastore files are added, destroyed, or updated randomly, automatically refreshing the cache is a good idea. Manual refresh lets you customize refresh time, such as at the conclusion of an extract-transform-load process.
Use BQ.REFRESH_EXTERNAL_METADATA_CACHE to manually refresh the metadata cache on a timetable that matches your needs. You may selectively update BigLake table information using subdirectories of the table data directory. You may prevent superfluous metadata processing. If datastore files are added, destroyed, or updated at predetermined intervals, such as pipeline output, manually refreshing the cache is a good idea.
Dual manual refreshes will only work once.
The metadata cache expires after 7 days without refreshment.
Manual and automated cache refreshes prioritize INTERACTIVE queries.
To utilize automatic refreshes, establish a reservation and an assignment with a BACKGROUND job type for the project that executes metadata cache refresh tasks. This avoids refresh operations from competing with user requests for resources and failing if there aren’t enough.
Before setting staleness interval and metadata caching mode, examine their interaction. Consider these instances:
To utilize cached metadata in table operations, you must call BQ.REFRESH_EXTERNAL_METADATA_CACHE every 2 days or less if you manually refresh the metadata cache and set the staleness interval to 2 days.
If you automatically refresh the metadata cache for a table and set the staleness interval to 30 minutes, some operations against the table may read from the datastore if the refresh takes longer than 30 to 60 minutes.
Tables with materialized views and cache
When querying structured data in Cloud Storage or Amazon S3, materialized views over BigLake metadata cache-enabled tables increase speed and efficiency. Automatic refresh and adaptive tweaking are available with these materialized views over BigQuery-managed storage tables.
Integrations
BigLake tables are available via other BigQuery features and gcloud CLI services, including the following.
Hub for Analytics
Analytics Hub supports BigLake tables. BigLake table datasets may be listed on Analytics Hub. These postings provide Analytics Hub customers a read-only linked dataset for their project. Subscribers may query all connected dataset tables, including BigLake.
BigQuery ML
BigQuery ML trains and runs models on BigLake in Cloud Storage.
Safeguard sensitive data
BigLake Sensitive Data Protection classifies sensitive data from your tables. Sensitive Data Protection de-identification transformations may conceal, remove, or obscure sensitive data.
Read more on Govindhtech.com
#BigLaketable#DataStorage#BigQueryOmni#AmazonS3#BigQuery#Crosscloud#ApacheSpark#CloudStoragebucket#news#technews#technology#technologynews#technologytrends#govindhtech
0 notes
Photo

Piloting
#amanwithvanishingideas#original Photographers#originalphotography#swedish photographer#sweden#Vänern#biglake
87 notes
·
View notes
Photo
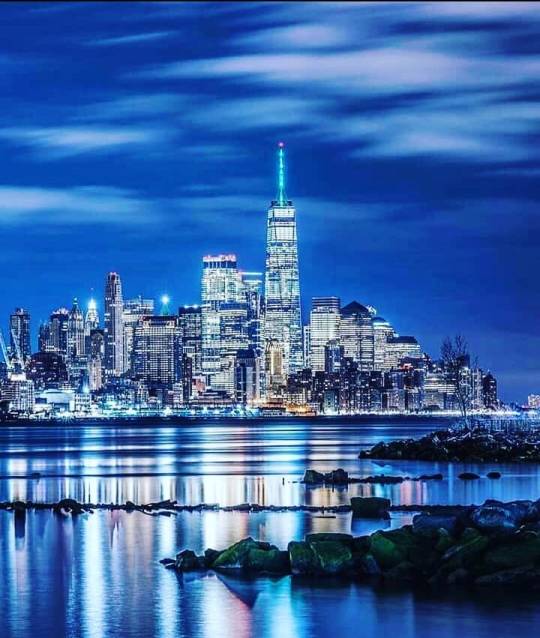
#chicago #biglake #landscape #cities #places #cities #travel #photooftheday #photography https://www.instagram.com/p/CR_1pPmHs9Z/?utm_medium=tumblr
2 notes
·
View notes
Photo

This artwork is about my mum, Mirdidingkingathi Juwarnda. She was taken away from her country but she never lost her creative roots.
Her art is bright and light, just like the land she was born in, Mirdidingki, on the south side of Bentinck Island.
This is her Country, where the Big Lake is.
MIART Amanda Gabori ~ My Mother’s Country No 671-20 Acrylic on unstretched Belgian Linen Size: 198w x 102h cms
Available at: https://artloversaustralia.com.au/product/amanda-gabori-my-mothers-country-no-671-20/
#art#artloversaustralia#bright#light#artwork#colour#country#island#biglake#acrylic#mother#artlovers#artlife#colourful#roundup
1 note
·
View note
Photo

Urungach lake by EugeneD
21 notes
·
View notes
Text
Come to see Annecy in France! https://youtu.be/hiWXWNozeLs
youtube

#road trip#travel#vacation#annecy#savoie#citylife#lakelife#lake#biglake#youtube#youtube video#Jbproduction74#Annecy4k#annecy 2020#viral videos
1 note
·
View note
Photo

Such a beautiful day here in Coeur d’Alene, Idaho. We are very excited to test drive the 2020 Hyundai #Palisade tomorrow. Stay tuned for lots of pictures and beautiful scenery. #histurnherturn #biglake #biglife #bigambitions (at Coeur d'Alene, Idaho) https://www.instagram.com/p/By1PFVeBjYb/?igshid=y8zy9ejo1r4p
1 note
·
View note
Video
instagram
Love the fresh air and deep blue color of Lake Superior in the springtime! 💙💙💙 . . . . . #nature #exploremn #sunset #photography #love #outdoors #michiganphotographer #naturephotography #biglake #freshwater #northshore #travel #wisconsin #greatlakes #upnorth #hiking #summer #explore #fall #minnesota #canada #nature #lakelife #adventure #lakesuperior #photooftheday #wanderlust #optoutside #onlyinmn #lake (at Stoney Point) https://www.instagram.com/p/BxkqhbBlRbj/?igshid=1cavrogt6ji34
#nature#exploremn#sunset#photography#love#outdoors#michiganphotographer#naturephotography#biglake#freshwater#northshore#travel#wisconsin#greatlakes#upnorth#hiking#summer#explore#fall#minnesota#canada#lakelife#adventure#lakesuperior#photooftheday#wanderlust#optoutside#onlyinmn#lake
1 note
·
View note
Photo

It's good to be home after a roadtrip. Thanks to @mr.l_art and @mr.pataconi for having me at the Wausau East CAFE night. My family got home and went plein air painting at the lake. It was super windy and the gouache dries really fast but I was able to knock this out in 20 mins. #studiotou #touher #gouache #sketchbook #pleinair #biglake (at Big Lake, Minnesota) https://www.instagram.com/p/BxiTBlDljEl/?igshid=1nl8caaaekdaj
1 note
·
View note