#VertexAI
Explore tagged Tumblr posts
Text
New Cloud Translation AI Improvements Support 189 Languages
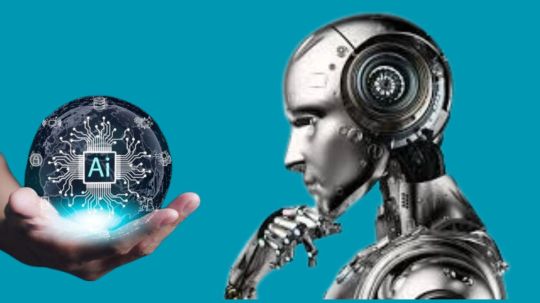
189 languages are now covered by the latest Cloud Translation AI improvements.
Your next major client doesn’t understand you. 40% of shoppers globally will never consider buying from a non-native website. Since 51.6% of internet users speak a language other than English, you may be losing half your consumers.
Businesses had to make an impossible decision up until this point when it came to handling translation use cases. They have to decide between the following options:
Human interpreters: Excellent, but costly and slow
Simple machine translation is quick but lacks subtleties.
DIY fixes: Unreliable and dangerous
The problem with translation, however, is that you need all three, and conventional translation techniques are unable to keep up. Using the appropriate context and tone to connect with people is more important than simply translating words.
For this reason, developed Translation AI in Vertex AI at Google Cloud. Its can’t wait to highlight the most recent developments and how they can benefit your company.
Translation AI: Unmatched translation quality, but in your way
There are two options available in Google Cloud‘s Translation AI:
A necessary set of tools for translation capability is the Translation API Basic. Google Cloud sophisticated Neural Machine Translation (NMT) model allows you to translate text and identify languages immediately. For chat interactions, short-form content, and situations where consistency and speed are essential, Translation AI Basic is ideal.
Advanced Translation API: Utilize bespoke glossaries to ensure terminology consistency, process full documents, and perform batch translations. For lengthy content, you can utilize Gemini-powered Translation model; for shorter content, you can use Adaptive Translation to capture the distinct tone and voice of your business. By using a glossary, improving its industry-leading translation algorithms, or modifying translation forecasts in real time, you can even personalize translations.
What’s new in Translation AI
Increased accuracy and reach
With 189-language support, which now includes Cantonese, Fijian, and Balinese, you can now reach audiences around the world while still achieving lightning-fast performance, making it ideal for call centers and user content.
Smarter adaptive translation
You can use as little as five samples to change the tone and style of your translations, or as many as 30,000 for maximum accuracy.
Choosing a model according to your use case
Depending on how sophisticated your translation use case is, you can select from a variety of methods when using Cloud Translation Advanced. For instance, you can select Adaptive Translation for real-time modification or use NMT model for translating generic text.
Quality without sacrificing
Although reports and leaderboards provide information about the general performance of the model, they don’t show how well a model meets your particular requirements. With the help of the gen AI assessment service, you can choose your own evaluation standards and get a clear picture of how well AI models and applications fit your use case. Examples of popular tools for assessing translation quality include Google MetricX and the popular COMET, which are currently accessible on the Vertex gen AI review service and have a significant correlation with human evaluation. Choose the translation strategy that best suits your demands by comparing models and prototyping solutions.
Google cloud two main goals while developing Translation AI were to change the way you translate and the way you approach translation. Its deliver on both in four crucial ways, whereas most providers only offer either strong translation or simple implementation.
Vertex AI for quick prototyping
Test translations in 189 languages right away. To determine your ideal fit, compare NMT or most recent translation-optimized Gemini-powered model. Get instant quality metrics to confirm your decisions and see how your unique adaptations work without creating a single line of code.
APIs that are ready for production for your current workflows
For high-volume, real-time translations, integrate Translation API (NMT) straight into your apps. When tone and context are crucial, use the same Translation API to switch to Adaptive Translation Gemini-powered model. Both models scale automatically to meet your demands and fit into your current workflows.
Customization without coding
Teach your industry’s unique terminology and phrases to bespoke translation models. All you have to do is submit domain-specific data, and Translation AI will create a unique model that understands your language. With little need for machine learning knowledge, it is ideal for specialist information in technical, legal, or medical domains.
Complete command using Vertex AI
With all-inclusive platform, Vertex AI, you can use Translation AI to own your whole translation workflow. You may choose the models you want, alter how they behave, and track performance in the real world with Vertex AI. Easily integrate with your current CI/CD procedures to get translation at scale that is really enterprise-grade.
Real impact: The Uber story
Uber’s goal is to enable individuals to go anywhere, get anything, and make their own way by utilizing the Google Cloud Translation AI product suite.
Read more on Govindhtech.com
#TranslationAI#VertexAI#GoogleCloud#AImodels#genAI#Gemini#CloudTranslationAI#News#Technology#technologynews#technews#govindhtech
2 notes
·
View notes
Text
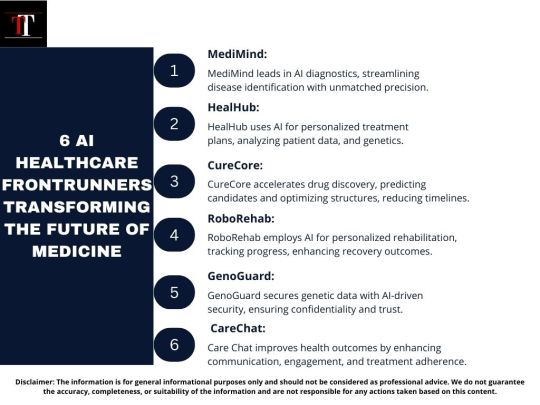
Google CEO Sundar Pichai Unveils Gemma: Developer's Innovation Hub
Sundar Pichai, CEO of Google and Alphabet, chose X platform to introduce Gemma, a groundbreaking AI innovation. Described as "a family of lightweight, state-of-the-art open models," Gemma leverages cutting-edge research and technology akin to Gemini models. With Gemma 2B and Gemma 7B versions, Google positions it alongside Gemini Pro 1.5 Pro, emphasizing responsible AI development tools and integration with frameworks like Colab, Kaggle notebooks, JAX, and more. Gemma, in collaboration with Vertex AI and Nvidia, enables generative AI applications with low latency and compatibility with NVIDIA GPUs, available through Google Cloud services.
#SundarPichai#GemmaAI#GoogleInnovation#OpenModels#AIAdvancement#ResponsibleAI#GemmaLaunch#VertexAI#NvidiaCollaboration#GoogleCloudAI
0 notes
Photo
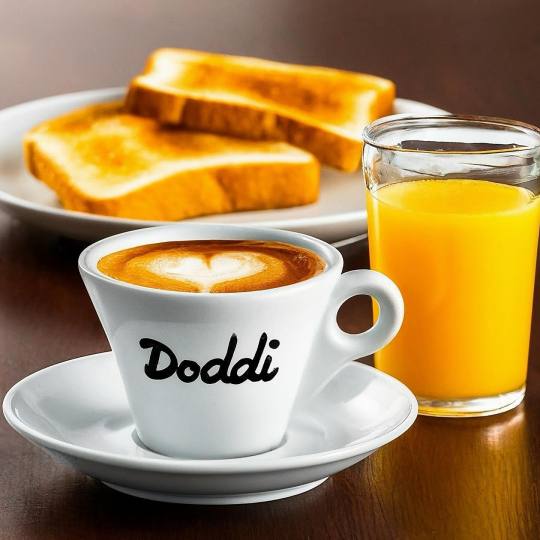
Minum kopi yuk! No, it is not real image. It is artifficially created by AI. It is created with Google Imagen 2 model of Generative AI. The enhancement of this new model compared to previous one is mindblowing! Please try them in Vertex AI console at Google Cloud. Prompt: A white coffee cup with a written caligraphic caption “Doddi” in it. It is sitting on a wooden tabletop, next to the cup is a plate with toast and a glass of fresh orange juice. #genai #googlecloud #vertexai #coffee #ai #prompt
0 notes
Text
Generative AI support on Vertex AI is now generally available
https://cloud.google.com/blog/products/ai-machine-learning/generative-ai-support-on-vertexai
0 notes
Photo
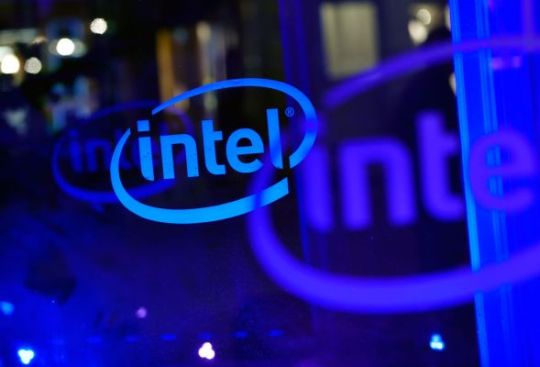
Intel buys deep-learning startup Vertex.AI to join its Movidius unit Intel has an ambition to bring more artificial intelligence technology into all aspects of its business, and today is stepping up its game a little in the area with an acquisition.
0 notes
Text
How will AI be used ethically in the future? AI Responsibility Lab has a plan
As the usage of AI grows in all sectors and virtually each side of society, there’s an more and more obvious want for controls for accountable AI.
Accountable AI is about guaranteeing that AI is utilized in a approach that isn’t unethical, helps respect private privateness, and usually avoids bias. There’s a seemingly limitless stream of firms, applied sciences and researchers tackling points associated to accountable AI. Now aptly named AI Accountability Labs (AIRL) is becoming a member of the fray, asserting $2 million in pre-seed funding, alongside a preview launch of its Mission Management software-as-a-service (SaaS) platform. from the corporate.
Main AIRL is the corporate’s CEO, Ramsay Brown, who skilled as a computational neuroscientist on the College of Southern California, the place he spent vital time mapping the human mind. His first startup was initially often known as Dopamine Labs, renamed Boundless Thoughts, with a give attention to behavioral know-how and utilizing machine studying to make predictions about how individuals will behave. boundless mind was acquired by Thrive International in 2019.
At AIRL, Brown and his group tackle the problems of AI safety and be certain that AI is used responsibly in a approach that doesn’t hurt society or the organizations utilizing the know-how.
“We based the corporate and constructed the Mission Management software program platform to start out serving to information science groups do their jobs higher, extra precisely, and quicker,” mentioned Brown. “If we glance across the accountable AI neighborhood, there are some individuals engaged on governance and compliance, however they don’t seem to be speaking to information science groups to search out out what actually hurts.”
What information science groups have to create accountable AI
Brown insisted that no group is prone to need to construct an AI that’s purposefully biased and makes use of information in an unethical approach.
What normally occurs in a posh improvement with many shifting components and totally different individuals is that information is inadvertently misused or machine studying fashions which have been skilled on incomplete information. When Brown and his group of information scientists requested what was lacking and what was hurting improvement efforts, respondents advised him they had been in search of venture administration software program relatively than a compliance framework.
“That was our large ‘a-ha’ second,” he mentioned. “What groups truly missed was not that they did not perceive the principles, it is that they did not know what their groups had been doing.”
Brown famous that 20 years in the past, software program engineering revolutionized the event of dashboard instruments like Atlassian’s Jira, which helped builders construct software program quicker. Now he hopes AIRL’s Mission Management would be the dashboard in information science to assist information groups construct applied sciences with accountable AI practices.
Working with present AI and MLops frameworks
There are a number of instruments organizations can use right now to handle AI and machine studying workflows, generally grouped beneath the MLops business class.
In style applied sciences embrace AWS Sagemaker, Google VertexAI, Domino Knowledge Lab, and BigPanda.
Brown mentioned one of many issues his firm has realized whereas constructing out the Mission Management service is that information science groups have many various instruments that they like to make use of. He mentioned that AIRL doesn’t need to compete with MLops and present AI instruments, however relatively offers an overlay for accountable AI use. What AIRL has accomplished is developed an open API endpoint so {that a} group utilizing Mission Management can enter any information from any platform and have it find yourself as a part of monitoring processes.
AIRL’s Mission Management offers a framework for groups to do what they’ve accomplished in advert hoc approaches and create standardized processes for machine studying and AI operations.
Brown mentioned Mission Management allows customers to take information science notebooks and convert them into repeatable processes and workflows that function inside configured parameters for accountable AI use. In such a mannequin, the info is linked to a monitoring system that may warn a corporation if there’s a violation of coverage. For instance, he famous that if a knowledge scientist makes use of a knowledge set that the coverage prohibits from getting used for a specific machine studying operation, Mission Management can mechanically catch it, alert managers, and pause the workflow.
“This centralization of data permits for higher coordination and visibility,” Brown mentioned. “It additionally reduces the possibility that methods with actually knotty and undesirable outcomes will find yourself in manufacturing.”
Trying ahead to 2027 and the way forward for accountable AI
Trying to 2027, AIRL has a roadmap to assist with extra superior issues round the usage of AI and the potential for Synthetic Normal Intelligence (AGI). The corporate’s focus in 2027 is on enabling an effort it calls the Artificial Labor Incentive Protocol (SLIP). The essential concept is to have some type of sensible contract for utilizing AGI-driven labor within the economic system.
“We’re trying on the creation of synthetic common intelligence, as a logistical enterprise and societal concern that shouldn’t be talked about in ‘sci-fi phrases,’ however in sensible incentive administration phrases,” Brown mentioned.
Source link
source https://epicapplications.com/how-will-ai-be-used-ethically-in-the-future-ai-responsibility-lab-has-a-plan/
0 notes
Quote
This guide provides a way to easily predict the structure of a protein (or multiple proteins) using a simplified version of AlphaFold running in a Vertex AI. For most targets, this method obtains predictions that are near-identical in accuracy compared to the full version.
Running AlphaFold on VertexAI | Google Cloud Blog
0 notes
Text
Intel Acquires Artificial Intelligence Startup Vertex.AI
Hardware manufacturers like Intel have also stepped into AI. Recently, Intel has acquired Vertex.AI, which is a Seattle-based, artificial intelligence startup and maker of deep learning engine PlaidML. source https://www.c-sharpcorner.com/news/intel-acquires-artificial-intelligence-startup-vertexai from C Sharp Corner https://ift.tt/2PsO74O
0 notes
Text
Aible And Google Cloud: Gen AI Models Sets Business Security
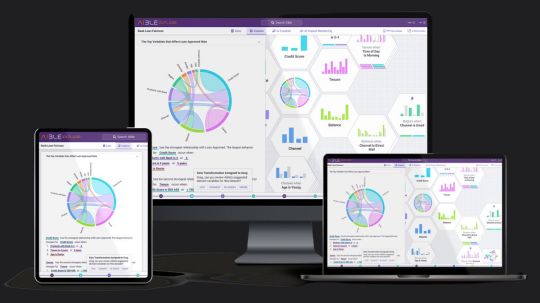
Enterprise controls and generative AI for business users in real time.
Aible
With solutions for customer acquisition, churn avoidance, demand prediction, preventive maintenance, and more, Aible is a pioneer in producing business impact from AI in less than 30 days. Teams can use AI to extract company value from raw enterprise data. Previously using BigQuery’s serverless architecture to save analytics costs, Aible is now working with Google Cloud to provide users the confidence and security to create, train, and implement generative AI models on their own data.
The following important factors have surfaced as market awareness of generative AI’s potential grows:
Enabling enterprise-grade control
Businesses want to utilize their corporate data to allow new AI experiences, but they also want to make sure they have control over their data to prevent unintentional usage of it to train AI models.
Reducing and preventing hallucinations
The possibility that models may produce illogical or non-factual information is another particular danger associated with general artificial intelligence.
Empowering business users
Enabling and empowering business people to utilize gen AI models with the least amount of hassle is one of the most beneficial use cases, even if gen AI supports many enterprise use cases.
Scaling use cases for gen AI
Businesses need a method for gathering and implementing their most promising use cases at scale, as well as for establishing standardized best practices and controls.
Regarding data privacy, policy, and regulatory compliance, the majority of enterprises have a low risk tolerance. However, given its potential to drive change, they do not see postponing the deployment of Gen AI as a feasible solution to market and competitive challenges. As a consequence, Aible sought an AI strategy that would protect client data while enabling a broad range of corporate users to swiftly adapt to a fast changing environment.
In order to provide clients complete control over how their data is used and accessed while creating, training, or optimizing AI models, Aible chose to utilize Vertex AI, Google Cloud’s AI platform.
Enabling enterprise-grade controls
Because of Google Cloud’s design methodology, users don’t need to take any more steps to ensure that their data is safe from day one. Google Cloud tenant projects immediately benefit from security and privacy thanks to Google AI products and services. For example, protected customer data in Cloud Storage may be accessed and used by Vertex AI Agent Builder, Enterprise Search, and Conversation AI. Customer-managed encryption keys (CMEK) can be used to further safeguard this data.
With Aible‘s Infrastructure as Code methodology, you can quickly incorporate all of Google Cloud’s advantages into your own applications. Whether you choose open models like LLama or Gemma, third-party models like Anthropic and Cohere, or Google gen AI models like Gemini, the whole experience is fully protected in the Vertex AI Model Garden.
In order to create a system that may activate third-party gen AI models without disclosing private data outside of Google Cloud, Aible additionally collaborated with its client advisory council, which consists of Fortune 100 organizations. Aible merely transmits high-level statistics on clusters which may be hidden if necessary instead of raw data to an external model. For instance, rather of transmitting raw sales data, it may communicate counts and averages depending on product or area.
This makes use of k-anonymity, a privacy approach that protects data privacy by never disclosing information about groups of people smaller than k. You may alter the default value of k; the more private the information transmission, the higher the k value. Aible makes the data transmission even more secure by changing the names of variables like “Country” to “Variable A” and values like “Italy” to “Value X” when masking is used.
Mitigating hallucination risk
It’s crucial to use grounding, retrieval augmented generation (RAG), and other strategies to lessen and lower the likelihood of hallucinations while employing gen AI. Aible, a partner of Built with Google Cloud AI, offers automated analysis to support human-in-the-loop review procedures, giving human specialists the right tools that can outperform manual labor.
Using its auto-generated Information Model (IM), an explainable AI that verifies facts based on the context contained in your structured corporate data at scale and double checks gen AI replies to avoid making incorrect conclusions, is one of the main ways Aible helps eliminate hallucinations.
Hallucinations are addressed in two ways by Aible’s Information Model:
It has been shown that the IM helps lessen hallucinations by grounding gen AI models on a relevant subset of data.
To verify each fact, Aible parses through the outputs of Gen AI and compares them to millions of responses that the Information Model already knows.
This is comparable to Google Cloud’s Vertex AI grounding features, which let you link models to dependable information sources, like as your company’s papers or the Internet, to base replies in certain data sources. A fact that has been automatically verified is shown in blue with the words “If it’s blue, it’s true.” Additionally, you may examine a matching chart created only by the Information Model and verify a certain pattern or variable.
The graphic below illustrates how Aible and Google Cloud collaborate to provide an end-to-end serverless environment that prioritizes artificial intelligence. Aible can analyze datasets of any size since it leverages BigQuery to efficiently analyze and conduct serverless queries across millions of variable combinations. One Fortune 500 client of Aible and Google Cloud, for instance, was able to automatically analyze over 75 datasets, which included 150 million questions and answers with 100 million rows of data. That assessment only cost $80 in total.
Aible may also access Model Garden, which contains Gemini and other top open-source and third-party models, by using Vertex AI. This implies that Aible may use AI models that are not Google-generated while yet enjoying the advantages of extra security measures like masking and k-anonymity.
All of your feedback, reinforcement learning, and Low-Rank Adaptation (LoRA) data are safely stored in your Google Cloud project and are never accessed by Aible.
Read more on Govindhtech.com
#Aible#GenAI#GenAIModels#BusinessSecurity#AI#BigQuery#AImodels#VertexAI#News#Technews#Technology#Technologynews#Technologytrends#govindhtech
2 notes
·
View notes
Text
Utilizing Dialogflow CX To Develop A Self-Escalating Chatbot
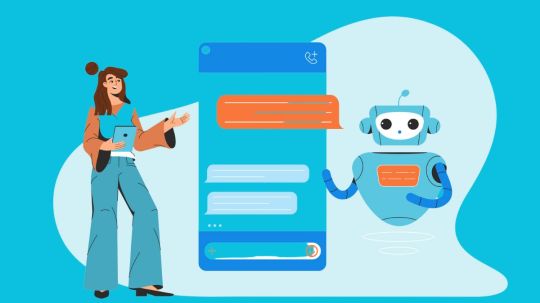
Use Webhooks and Generators to build a self-escalating chatbot in Conversational Agents (Dialogflow CX). Application developers must create chatbots that can accurately offer information and recognize when to escalate a user’s issue to a human agent, as conversational AI becomes an increasingly important aspect of the user experience.
This blog article will demonstrate how to use Google Cloud’s generative AI products, including Conversational Agents (Dialogflow CX) and Vertex AI, to build a self-escalating chatbot.
The solution has a number of advantages:
Better user experience: Even for complicated queries, users get quick help and accurate information.
Reduced agent workload: Agent effort is decreased since agents are given succinct recaps of prior exchanges, which enables them to handle problems quickly.
Improved chatbot capabilities: By learning from escalated requests, the chatbot can get better at handling encounters in the future.
Enhanced security and scalability: Cloud Run Functions (CRF) offers a safe and scalable environment for executing the webhook function.
Build the knowledge base
Suppose it would like a chatbot to respond to inquiries on Indian tourism. First, it will develop it using Conversational Agents (Dialogflow CX) and Vertex AI Agent Builder.
Datastore without structure: To use Vertex AI to index an article on “Tourism in India” as an unstructured datastore. This enables the chatbot to instantly access and extract pertinent information from the article, giving thorough responses to user inquiries.
Conversational Agents (Dialogflow CX): To utilize Conversational Agents (Dialogflow CX) to create the conversational flow, which allows the chatbot to comprehend user intent and react accordingly.
Gauge user satisfaction
Asking the user if they are happy with the chatbot’s response is an essential part of the conversation flow that to include to guarantee user happiness. This is accomplished by employing “yes” and “no” chips that are defined as part of the custom payload, giving consumers an easy-to-understand method of expressing their opinions.
Escalate with generative AI
The chatbot starts the escalation procedure if the user clicks “no,” indicating discontent. This is where generative AI’s capability is useful.
Generators: In Conversational Agents (Dialogflow CX), it develop a generator called “Summarize_mail” that summarizes the discussion using a zero-shot prompt, which is a direct prompt without any examples. The body of an email is then created using this summary, giving the human agent context.
You are an English expert in summarizing the text in form of a very short mail. Summarize the conversation and write it in form of a concise e-mail which will be forwarded to an agent as a ticket. The mail should be on point, properly formatted and written in formal tone with a polite closure. Keep the text as less as possible. Also, specify the conversation messages below the summary which are present in the conversation. The conversation is as follows: $conversation The feedback of the user about the issue is $last-user-utterance.
Model setup: To assist guarantee logical and instructive summaries, this generator makes use of the Gemini-1.5-flash model with a temperature of 0.3.
Trigger the email with Cloud Run Functions (CRF)
To link it Conversational Agents (Dialogflow CX) agent to a serverless function that is set up on Cloud Run Functions via a webhook in order to send the email. The logic for sending emails is handled by this function.
Configuring Cloud Run Functions (CRF): The following options are used while configuring the CRF:
Python 3.12 is the runtime.
Setting: Second Generation
Point of entry: handle_webhook
Type of trigger: HTTPS
Allotted memory: 256 MiB
Settings for ingress: Permit all traffic.
Requirements: functions-framework==3 (requirements.txt).
For Dialogflow CX Webhooks, are you unsure which CRF version to select? Here’s how to make a decision quickly:
First Generation: Suitable for simple webhooks with less demanding performance needs, it is easier to set up and deploy.
Second Generation: Better suited for intricate webhooks or high-traffic situations, it provides greater flexibility, control, and scalability.
Connect the pieces
To guarantee that the escalation procedure is initiated appropriately when necessary, provide the URL of it installed CRF as the webhook URL in Conversational Agents (Dialogflow CX).
Here’s how it all comes together
With a focus on the escalation process and the function of the CRF, this breakdown offers a thorough knowledge of the Conversational Agents (Dialogflow CX) flow architecture. Don’t forget to modify the email’s text, messages, and flow to fit your unique requirements and branding.
The name of the flow, such as “Customer Support Flow”
Pages: “Start Page” and “Escalate” are the two pages.
Start Page
Purpose: The goal is to greet the user and start a conversation while measuring their level of happiness.
Greeting entry fulfillment:
“Hello, name!” says the agent. Tell us how to may help you, please.
Datastore response entry fulfillment:
“Are you satisfied with the response?” asks the agent.
(This generates the “Yes” and “No” chips.) Custom payload
Routes:
Status: “Yes” chip was chosen
Transition: First page (Denotes that the discussion was successful.)
“Thank you!” says the agent. Do you need to assistance with anything else?
Situation: “No” chip was chosen
Transition: Page “Escalate”
“Sorry to hear that!” the agent says. (Recognizes user discontent.)
Escalate
Purpose: Providing the user with the choice to escalate to a human agent is the goal.
Fulfillment of entry:
“Would you like to escalate?” asks the agent.
(Identical “Yes” and “No” chips as the “Start Page”) Custom payload
Routes:
Situation: “No” chip was chosen
Change of Scene: “Start Page”
“Sure, Thank you!” the agent replies. (Permits the user to keep using the bot.)
Status: “Yes” chip was chosen
“Escalation Webhook” is the transition.
Webhook: Functions of Cloud Run (Starts the escalation procedure)
Goals:
It use the training terms “yes” and “no” to indicate the two intentions “confirm.yes” and “confirm.no.” This is equivalent to the user clicking on the “yes” and “no” chips or just writing the words or phrases that are comparable.
Cloud Run Functions (Escalation Webhook)
Trigger: When the “Escalate” page switches to the webhook, the HTTPS Eventarc trigger is triggered.
Usability:
Compile the history of the conversation: Using the $conversation in the generator prompt, which records the discussion between the agent and the user, excluding the user’s final utterance and the agent’s subsequent utterances, get and parse the whole conversation history from the Conversational Agents (Dialogflow CX) session.
Create a succinct synopsis of the discussion ($conversation), emphasizing any important user requests or problems.
Retrieve user data: Get the user’s email address (along with any other pertinent information) from your user database or the Conversational Agents (Dialogflow CX) session.
Write an email: Make an email using:
Topic: (for instance, “Escalated Conversation from [User Email]”)
Recipient: The email address of the support agent
CC: The email address of the user
Body:
Details about the user (e.g., name, ID)
Synopsis of the conversation
If accessible, a link to the entire discussion in Conversational Agents (Dialogflow CX)
Send email: Send the email using the API provided by your email provider or an email sending library (such as sendgrid, mailgun, or smtp).
Optional return response: Notify Conversational Agents (Dialogflow CX) that the user’s request has been escalated by sending a response (e.g., “The mail is successfully sent!” or “Your request has been escalated”). An agent will be in touch with you soon.”
Chatbot testing and results
You may test the chatbot by clicking “Publish” and then “Try it now” after finishing the aforementioned steps.
A few examples of user journeys are as follows:
The user does not want to escalate further since they are dissatisfied with the response.
The user escalates as well since they are dissatisfied with the response. The right image below displays an example of triggered mail.
This method demonstrates how to integrate several Google Cloud services, such as Vertex AI, to create intelligent and intuitive chatbots.
This may anticipate even more creative solutions that improve client experiences as conversational AI develops further.
Read more on govindhtech.com
#UtilizingDialogflowCX#DevelopA#VertexAI#SelfEscalatingChatbot#CloudRunFunctions#Gemini15flash#DialogflowCX#Chatbottesting#EscalationWebhook#technology#technews#news#govindhtech
0 notes
Text
The Mistral AI New Model Large-Instruct-2411 On Vertex AI
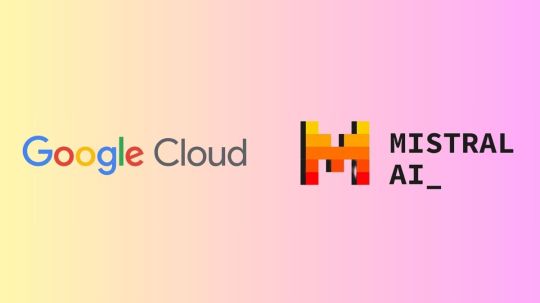
Introducing the Mistral AI New Model Large-Instruct-2411 on Vertex AI from Mistral AI
Mistral AI’s models, Codestral for code generation jobs, Mistral Large 2 for high-complexity tasks, and the lightweight Mistral Nemo for reasoning tasks like creative writing, were made available on Vertex AI in July. Google Cloud is announcing that the Mistral AI new model is now accessible on Vertex AI Model Garden: Mistral-Large-Instruct-2411 is currently accessible to the public.
Large-Instruct-2411 is a sophisticated dense large language model (LLM) with 123B parameters that extends its predecessor with improved long context, function calling, and system prompt. It has powerful reasoning, knowledge, and coding skills. The approach is perfect for use scenarios such as big context applications that need strict adherence for code generation and retrieval-augmented generation (RAG), or sophisticated agentic workflows with exact instruction following and JSON outputs.
The new Mistral AI Large-Instruct-2411 model is available for deployment on Vertex AI via its Model-as-a-Service (MaaS) or self-service offering right now.
With the new Mistral AI models on Vertex AI, what are your options?
Using Mistral’s models to build atop Vertex AI, you can:
Choose the model that best suits your use case: A variety of Mistral AI models are available, including effective models for low-latency requirements and strong models for intricate tasks like agentic processes. Vertex AI simplifies the process of assessing and choosing the best model.
Try things with assurance: Vertex AI offers fully managed Model-as-a-Service for Mistral AI models. Through straightforward API calls and thorough side-by-side evaluations in its user-friendly environment, you may investigate Mistral AI models.
Control models without incurring extra costs: With pay-as-you-go pricing flexibility and fully managed infrastructure built for AI workloads, you can streamline the large-scale deployment of the new Mistral AI models.
Adjust the models to your requirements: With your distinct data and subject expertise, you will be able to refine Mistral AI’s models to produce custom solutions in the upcoming weeks.
Create intelligent agents: Using Vertex AI’s extensive toolkit, which includes LangChain on Vertex AI, create and coordinate agents driven by Mistral AI models. To integrate Mistral AI models into your production-ready AI experiences, use Genkit’s Vertex AI plugin.
Construct with enterprise-level compliance and security: Make use of Google Cloud’s integrated privacy, security, and compliance features. Enterprise controls, like the new organization policy for Vertex AI Model Garden, offer the proper access controls to guarantee that only authorized models are accessible.
Start using Google Cloud’s Mistral AI models
Google Cloud’s dedication to open and adaptable AI ecosystems that assist you in creating solutions that best meet your needs is demonstrated by these additions. Its partnership with Mistral AI demonstrates its open strategy in a cohesive, enterprise-ready setting. Many of the first-party, open-source, and third-party models offered by Vertex AI, including the recently released Mistral AI models, can be provided as a fully managed Model-as-a-service (MaaS) offering, giving you enterprise-grade security on its fully managed infrastructure and the ease of a single bill.
Mistral Large (24.11)
The most recent iteration of the Mistral Large model, known as Mistral Large (24.11), has enhanced reasoning and function calling capabilities.
Mistral Large is a sophisticated Large Language Model (LLM) that possesses cutting-edge knowledge, reasoning, and coding skills.
Intentionally multilingual: English, French, German, Spanish, Italian, Chinese, Japanese, Korean, Portuguese, Dutch, Polish, Arabic, and Hindi are among the dozens of languages that are supported.
Multi-model capability: Mistral Large 24.11 maintains cutting-edge performance on text tasks while excelling at visual comprehension.
Competent in coding: Taught more than 80 coding languages, including Java, Python, C, C++, JavaScript, and Bash. Additionally, more specialized languages like Swift and Fortran were taught.
Agent-focused: Top-notch agentic features, including native function calls and JSON output.
Sophisticated reasoning: Cutting-edge reasoning and mathematical skills.
Context length: 128K is the most that Mistral Large can support.
Use cases
Agents: Made possible by strict adherence to instructions, JSON output mode, and robust safety measures
Text: Creation, comprehension, and modification of synthetic text
RAG: Important data is preserved across lengthy context windows (up to 128K tokens).
Coding includes creating, finishing, reviewing, and commenting on code. All popular coding languages are supported.
Read more on govindhtech.com
#MistralAI#ModelLarge#VertexAI#MistralLarge2#Codestral#retrievalaugmentedgeneration#RAG#VertexAIModelGarden#LargeLanguageModel#LLM#technology#technews#news#govindhtech
0 notes
Text
Google Cloud’s Generate Prompt And Prompt Refinement Tools
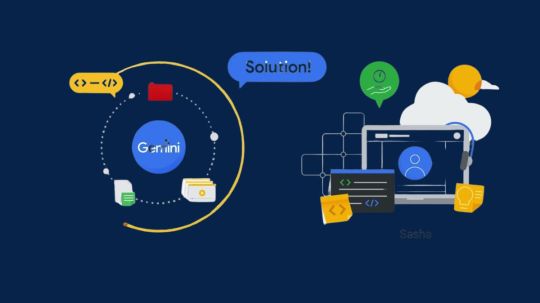
Google Cloud Introduces Prompt Refinement and Generate Prompt Tools
It can be an art form to create the ideal trigger for generative AI models. Sometimes a well-written prompt can make the difference between an AI response that is helpful and one that is generic. However, getting there frequently involves a learning curve, iteration, and time-consuming tweaking. Google Cloud presents new enhancements to Vertex AI’s AI-powered prompt writing tools, which are intended to make prompting simpler and more approachable for all developers.
Two strong features that will improve your timely engineering workflow are being introduced by Google Cloud: Create a prompt, then refine it.
Generate prompt
Create your own prompts with the Vertex AI Studio AI prompt assistance. You can expedite the prompt-writing process by creating your own prompts.
To create prompts, follow these steps:
Navigate to the Google Cloud console’s Freeform page.
A prompt with no title appears.
Click Help me write in the prompt box.
The dialogue box for “Help me write” opens.
Give a brief explanation of the goal you hope your prompt will accomplish. Take a music recommendation bot, for instance.
Press the “Generate prompt” button.
Based on your instructions, the AI prompt assistant makes a suggestion.
Press the Insert button.
The created prompt is copied by Vertex AI to the Freeform page’s Prompt section.
Press the “Submit” button.
Delete the prompt and try again if you’re not happy with the model’s response.
Generate prompt: In just a few seconds, go from objective to prompt
Consider that you require a prompt to compile client testimonials about your most recent offering. You can just specify your objective to the Generate prompt feature rather than creating the prompt yourself. After that, it will generate a thorough prompt with review placeholders that you can quickly fill in with your own information. Prompt engineering is made less uncertain by Generate Prompt by:
Transforming straightforward goals into customized, powerful prompts. You won’t have to stress over word choice and phrase this way.
Creating context-relevant placeholders, such as news items, code snippets, or customer reviews. This enables you to add your unique data quickly and see results right away.
Accelerating the process of prompt writing. Concentrate on your primary responsibilities rather than honing your prompt syntax.
Prompt Refinement
Vertex AI Studio’s AI assistant can aid you in honing your prompts according to the outcomes you hope to achieve.
To improve prompts, take the following actions:
Navigate to the Google Cloud console’s Freeform page.
A prompt with no title appears.
Put your prompt in the prompt box.
Press the “Submit” button.
The response box displays a response. Make a note of the aspects of the response that you would like to modify.
Select Refine the prompt.
The dialog box for Refine opens.
You can either choose one or more of the pre-filled feedback options below, or you can provide comments about what you would want to see changed in the model’s response to your prompt:
Make shorter
Maker longer
More professional
More casual
Click “Apply” and “Run.”
The new prompt is run by Vertex AI.
Continue doing this until the model’s reaction meets your needs.
Refine prompt: Use AI-powered ideas to iterate and enhance
Refine prompt assists you in adjusting a prompt for best results, whether it was created by Generate prompt or you wrote it yourself. This is how it operates:
After your prompt has been run, just comment on the response in the same manner that you would a writer.
Instant recommendations: Vertex AI considers your input and creates a fresh, recommended prompt in a single step.
Continue iterating by running the improved prompt and offering more input. The recommendation is yours to accept or reject.
In addition to saving a great deal of time during prompt design, prompt refinement improves the prompt’s quality. Usually, the prompt directions are enhanced in a way that Gemini can comprehend to boost the quality.
Some examples of prompts that were updated using the Refine prompt are provided below:
Regardless of your level of expertise, these two elements combine to assist you in creating the best prompt for your goal. While Refine prompt enables iterative development in five steps, Generate prompt gets you started quickly:
Specify your goal: Explain your goals to the Generate prompt.
Generate a prompt: This function generates a ready-to-use prompt, frequently with useful context-related placeholders.
Execute the prompt and examine the result: Use Vertex AI to carry out the suggestion using the LLM of your choice.
Refine with feedback: Give input on the output using the Refine prompt to get AI-powered recommendations for immediate enhancement.
Iterate till optimal performance: Keep improving and executing your suggestion until you get the outcomes you want.
How to begin
Try out Google Cloud’s interactive criticizing workflow to see how AI can help with prompt writing. Using this link, you can try Vertex AI’s user-friendly interface for refining prompts without creating a Google Cloud account (to demo without a Google Cloud account, make sure you are using incognito mode or are logged out of your Google account on your web browser. Those who have an account will be able to customize, modify, and save their prompts.
Read more on govindhtech.com
#GoogleCloud#GeneratePrompt#VertexAI#Gemini#PromptRefinementTools#Promptengineering#PromptRefinement#AIpoweredideas#technology#technews#news#govindhtech
0 notes
Text
Etsy’s Service Platform On Cloud Run Use An Hour Not A Days
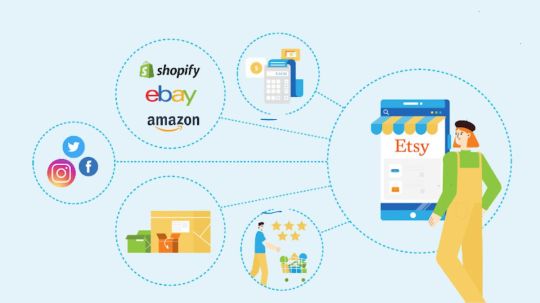
Deployment times for Etsy’s Service Platform on Cloud Run are reduced from days to less than an hour.
Overview
Popular online business Etsy sells vintage, handcrafted, and unusual items and attempts to give excellent service. Etsy needs more people, technology, and resources like many fast-growing organizations. Over 1400% of its gross product sales climbed to $13.5 billion between 2012 and 2021.
Etsy moved all of its infrastructure from conventional data centers to Google Cloud in an attempt to keep up with this development. In addition to being a major technology advancement, this change forced Etsy to reconsider how it approaches service development. The process resulted in the establishment of “ESP” (Etsy’s Service Platform), a Google Cloud Run-based service platform specifically designed for Etsy that simplifies microservices development, deployment, and administration.
The need for change and architectural vision
The need for technical team to handle more sophisticated features and more traffic in Google cloud marketplace increased along with Etsy’s growth. Etsy developers were able to investigate and use Google Cloud-based service platforms with 2018 transfer to GCP. However, this surge of technological innovation also brought out some new difficulties, such as redundant code and scaffolding and unsupported infrastructure with unclear ownership.
In order to overcome these obstacles, Etsy brought together a group of architects to create a blueprint outlining the direction of the company’s future service growth. The objective was unambiguous: establish a platform that frees developers from the burden of backend complexity and enables them to swiftly and securely launch new services by separating service development from infrastructure.
Transforming vision into reality
The resultant architectural concept served as the foundation for Etsy’s Service Platform, or ESP, and a newly assembled team was tasked with the thrilling task of making the vision a reality. Putting together a dynamic team that could bridge the gap between application development and infrastructure was the first step. The team, which was made up of seasoned engineers with a variety of specialties, contributed a wide range of abilities.
Understanding how critical it was to connect with future platform users, the team worked closely with Etsy’s engineering and architecture. By consenting to embed one of their senior engineers in the service platform team, the Ads Platform Team, which was previously involved in service development, played a crucial role. As part of the Etsy’s Service Platform experiment, they jointly produced a Minimum Viable Platform (MVP) to facilitate the rollout of a new Ads Platform service.
Choosing Cloud Run for accelerated development
By separating infrastructure and automating its provisioning, architectural vision for a successful service platform would simplify the developer experience. The team realized that the bigger engineering organization’s prospective clients also need a platform that could seamlessly integrate into their workflow. The service platform team decided to concentrate on Etsy-specific elements in order to do this, including observability, service catalog, security, compliance, CI/CD, connection with current services, developer experience and language support, and more.
It was a calculated move to use Google Cloud services, particularly Cloud Run. The team intended to provide value as soon as possible, even if options like GKE were alluring. The team was able to concentrate on core platform functionality because to Cloud Run’s strong and user-friendly architecture, which helped Cloud Run manage the more difficult and time-consuming parts of executing containerized services.
The Toolbox: A Closer Look
Etsy’s Service Platform uses a well chosen toolkit to provide a reliable and effective development and operational experience:
Developer Interface: A specially designed CLI tool to make developer interactions more efficient.
Protocols for standardized communication include protobuf and gRPC.
Supported languages include Go, Python, Node.js, PHP, Java, and Scala.
CI/CD: Use GitHub Actions to provide a seamless pipeline for integration and deployment.
Observability: Using Prometheus, AlertManager, Google Monitoring and Logging, and OTEL on Google Cloud services
Client Library: Artifactory has Etsy’s Service Platform-generated clients registered.
Service Catalog: Centralized service visibility via Backstage.
Cloud Run was selected as the runtime due to its compatibility and ease of use.
Navigating Challenges
There were challenges along the way to developing the service platform. Overloading occurred on the VPC connection, and in order to maximize resource allocation, some services needed to be adjusted. Future adopters will benefit from platform-level enhancements brought forth by these difficulties.
Flexibility was given top priority in Etsy’s Service Platform design to account for varied technological environment. Despite the team’s multi-technological experience, it was difficult to develop a platform that could accommodate a wide range of service and client languages and use cases. Based on customer input, Google cloud made the decision to first concentrate on a core feature set and then add incremental capabilities and workarounds.
Important lessons learned throughout ESP’s development influenced both its ongoing operations and its future direction.
Sandbox Feature: Developers were able to deploy development versions of new services on Cloud Run in less than five minutes, replete with CI/CD and observability, with a rapid iteration process provided by a “sandbox” environment.
Known Observability Tools: ESP simplified engineer processes by integrating with current tools, such as promQL and Grafana.
Security Considerations: Working with the Google Serverless Networking team guaranteed safe connection with the old apps, even though ESP preferred TLS and layer 7 authentication via Google IAM.
Encouraging AI/ML Innovation: ESP’s flexibility was shown at a company-wide hackathon when a service that interfaced with Google’s Vertex AI was quickly put into use.
Real-World Success: As client support in new languages became available, the Ads Platform service grew to three more systems. The increasing load was effortlessly managed by Cloud Run’s auto-scaling.
Conclusion and Future Outlook
Etsy’s Service Platform is being steadily and continuously adopted across the company, allowing engineers to be bold, quick, and safe. Collaboration between Google cloud internal GKE team and Google has been sparked by customer needs for workloads beyond the serverless approach. Extending ESP’s tools to accommodate a growing range of services while preserving a consistently high standard of developer and operational experience is the aim.
Read more on Govindhtech.com
#Etsy#EtsyService#CloudRun#microservices#GKE#CI/CD#VertexAI#AI#ESP#News#Technews#Technology#Technologynews#Technologytrends#govindhtech
0 notes
Text
Google Vertex AI API And Arize For Generative AI Success
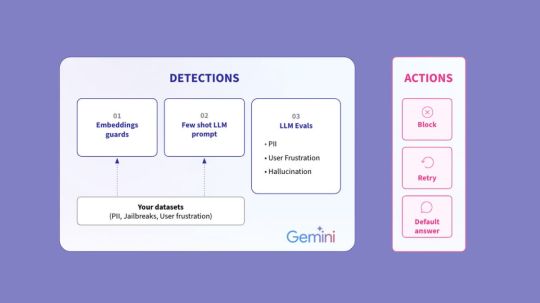
Arize, Vertex AI API: Assessment procedures to boost AI ROI and generative app development
Vertex AI API providing Gemini 1.5 Pro is a cutting-edge large language model (LLM) with multi-modal features that provides enterprise teams with a potent model that can be integrated across a variety of applications and use cases. The potential to revolutionize business operations is substantial, ranging from boosting data analysis and decision-making to automating intricate procedures and improving consumer relations.
Enterprise AI teams can do the following by using Vertex AI API for Gemini:
Develop more quickly by using sophisticated natural language generation and processing tools to expedite the documentation, debugging, and code development processes.
Improve the experiences of customers: Install advanced chatbots and virtual assistants that can comprehend and reply to consumer inquiries in a variety of ways.
Enhance data analysis: For more thorough and perceptive data analysis, make use of the capacity to process and understand different data formats, such as text, photos, and audio.
Enhance decision-making by utilizing sophisticated reasoning skills to offer data-driven insights and suggestions that aid in strategic decision-making.
Encourage innovation by utilizing Vertex AI’s generative capabilities to investigate novel avenues for research, product development, and creative activities.
While creating generative apps, teams utilizing the Vertex AI API benefit from putting in place a telemetry system, or AI observability and LLM assessment, to verify performance and quicken the iteration cycle. When AI teams use Arize AI in conjunction with their Google AI tools, they can:
As input data changes and new use cases emerge, continuously evaluate and monitor the performance of generative apps to help ensure application stability. This will allow you to promptly address issues both during development and after deployment.
Accelerate development cycles by testing and comparing the outcomes of multiple quick iterations using pre-production app evaluations and procedures.
Put safeguards in place for protection: Make sure outputs fall within acceptable bounds by methodically testing the app’s reactions to a variety of inputs and edge circumstances.
Enhance dynamic data by automatically identifying difficult or unclear cases for additional analysis and fine-tuning, as well as flagging low-performing sessions for review.
From development to deployment, use Arize’s open-source assessment solution consistently. When apps are ready for production, use an enterprise-ready platform.
Answers to typical problems that AI engineering teams face
A common set of issues surfaced while collaborating with hundreds of AI engineering teams to develop and implement generative-powered applications:
Performance regressions can be caused by little adjustments; even slight modifications to the underlying data or prompts might cause anticipated declines. It’s challenging to predict or locate these regressions.
Identifying edge cases, underrepresented scenarios, or high-impact failure modes necessitates the use of sophisticated data mining techniques in order to extract useful subsets of data for testing and development.
A single factually inaccurate or improper response might result in legal problems, a loss of confidence, or financial liabilities. Poor LLM responses can have a significant impact on a corporation.
Engineering teams can address these issues head-on using Arize’s AI observability and assessment platform, laying the groundwork for online production observability throughout the app development stage. Let’s take a closer look at the particular uses and integration tactics for Arize and Vertex AI, as well as how a business AI engineering team may use the two products in tandem to create superior AI.
Use LLM tracing in development to increase visibility
Arize’s LLM tracing features make it easier to design and troubleshoot applications by giving insight into every call in an LLM-powered system. Because orchestration and agentic frameworks can conceal a vast number of distributed system calls that are nearly hard to debug without programmatic tracing, this is particularly important for systems that use them.
Teams can fully comprehend how the Vertex AI API supporting Gemini 1.5 Pro handles input data via all application layers query, retriever, embedding, LLM call, synthesis, etc. using LLM tracing. AI engineers can identify the cause of an issue and how it might spread through the system’s layers by using traces available from the session level down to a specific span, such as retrieving a single document.Image credit to Google Cloud
Additionally, basic telemetry data like token usage and delay in system stages and Vertex AI API calls are exposed using LLM tracing. This makes it possible to locate inefficiencies and bottlenecks for additional application performance optimization. It only takes a few lines of code to instrument Arize tracing on apps; traces are gathered automatically from more than a dozen frameworks, including OpenAI, DSPy, LlamaIndex, and LangChain, or they may be manually configured using the OpenTelemetry Trace API.
Could you play it again and correct it? Vertex AI problems in the prompt + data playground
The outputs of LLM-powered apps can be greatly enhanced by resolving issues and performing fast engineering with your application data. With the help of app development data, developers may optimize prompts used with the Vertex AI API for Gemini in an interactive environment with Arize’s prompt + data playground.
It can be used to import trace data and investigate the effects of altering model parameters, input variables, and prompt templates. With Arize’s workflows, developers can replay instances in the platform directly after receiving a prompt from an app trace of interest. As new use cases are implemented or encountered by the Vertex AI API providing Gemini 1.5 Pro after apps go live, this is a practical way to quickly iterate and test various prompt configurations.Image credit to Google Cloud
Verify performance via the online LLM assessment
With a methodical approach to LLM evaluation, Arize assists developers in validating performance after tracing is put into place. To rate the quality of LLM outputs on particular tasks including hallucination, relevancy, Q&A on retrieved material, code creation, user dissatisfaction, summarization, and many more, the Arize evaluation library consists of a collection of pre-tested evaluation frameworks.
In a process known as Online LLM as a judge, Google customers can automate and scale evaluation processes by using the Vertex AI API serving Gemini models. Using Online LLM as a judge, developers choose Vertex AI API servicing Gemini as the platform’s evaluator and specify the evaluation criteria in a prompt template in Arize. The model scores, or assesses, the system’s outputs according to the specified criteria while the LLM application is operating.Image credit to Google Cloud
Additionally, the assessments produced can be explained using the Vertex AI API that serves Gemini. It can frequently be challenging to comprehend why an LLM reacts in a particular manner; explanations reveal the reasoning and can further increase the precision of assessments that follow.
Using assessments during the active development of AI applications is very beneficial to teams since it provides an early performance standard upon which to base later iterations and fine-tuning.
Assemble dynamic datasets for testing
In order to conduct tests and monitor enhancements to their prompts, LLM, or other components of their application, developers can use Arize’s dynamic dataset curation feature to gather examples of interest, such as high-quality assessments or edge circumstances where the LLM performs poorly.
By combining offline and online data streams with Vertex AI Vector Search, developers can use AI to locate data points that are similar to the ones of interest and curate the samples into a dataset that changes over time as the application runs. As traces are gathered to continuously validate performance, developers can use Arize to automate online processes that find examples of interest. Additional examples can be added by hand or using the Vertex AI API for Gemini-driven annotation and tagging.
Once a dataset is established, it can be used for experimentation. It provides developers with procedures to test new versions of the Vertex AI API serving Gemini against particular use cases or to perform A/B testing against prompt template modifications and prompt variable changes. Finding the best setup to balance model performance and efficiency requires methodical experimentation, especially in production settings where response times are crucial.
Protect your company with the Vertex AI API and Arize, which serve Gemini
Arize and Google AI work together to protect your AI against unfavorable effects on your clients and company. Real-time protection against malevolent attempts like as jailbreaks, context management, compliance, and user experience all depend on LLM guardrails.
Custom datasets and a refined Vertex AI Gemini model can be used to configure Arize guardrails for the following detections:
Embeddings guards: By analyzing the cosine distance between embeddings, it uses your examples of “bad” messages to protect against similar inputs. This strategy has the advantage of constant iteration during breaks, which helps the guard become increasingly sophisticated over time.
Few-shot LLM prompt: The model determines whether your few-shot instances are “pass” or “fail.” This is particularly useful when defining a guardrail that is entirely customized.
LLM evaluations: Look for triggers such as PII data, user annoyance, hallucinations, etc. using the Vertex AI API offering Gemini. Scaled LLM evaluations serve as the basis for this strategy.
An instant corrective action will be taken to prevent your application from producing an unwanted response if these detections are highlighted in Arize. The remedy can be set by developers to prevent, retry, or default an answer such “I cannot answer your query.”
Utilizing the Vertex AI API, your personal Arize AI Copilot supports Gemini 1.5 Pro
Developers can utilize Arize AI Copilot, which is powered by the Vertex AI API servicing Gemini, to further expedite the AI observability and evaluation process. AI teams’ processes are streamlined by an in-platform helper, which automates activities and analysis to reduce team members’ daily operational effort.
Arize Copilot allows engineers to:
Start AI Search using the Vertex AI API for Gemini; look for particular instances, such “angry responses” or “frustrated user inquiries,” to include in a dataset.
Take prompt action and conduct analysis; set up dashboard monitors or pose inquiries on your models and data.
Automate the process of creating and defining LLM assessments.
Prompt engineering: request that Gemini’s Vertex AI API produce prompt playground iterations for you.
Using Arize and Vertex AI to accelerate AI innovation
The integration of Arize AI with Vertex AI API serving Gemini is a compelling solution for optimizing and protecting generative applications as businesses push the limits of AI. AI teams may expedite development, improve application performance, and contribute to dependability from development to deployment by utilizing Google’s sophisticated LLM capabilities and Arize’s observability and evaluation platform.
Arize AI Copilot’s automated processes, real-time guardrails, and dynamic dataset curation are just a few examples of how these technologies complement one another to spur innovation and produce significant commercial results. Arize and Vertex AI API providing Gemini models offer the essential infrastructure to handle the challenges of contemporary AI engineering as you continue to create and build AI applications, ensuring that your projects stay effective, robust, and significant.
Do you want to further streamline your AI observability? Arize is available on the Google Cloud Marketplace! Deploying Arize and tracking the performance of your production models is now simpler than ever with this connection.
Read more on Govindhtech.com
#VertexAIAPI#largelanguagemodel#VertexAI#OpenAI#LlamaIndex#Geminimodels#VertexAIVectorSearch#GoogleCloudMarketplace#News#Technews#Technology#Technologynews#Technologytrends#govindhtech
1 note
·
View note
Text
Google Secure AI Framework: Improving AI Security And Trust
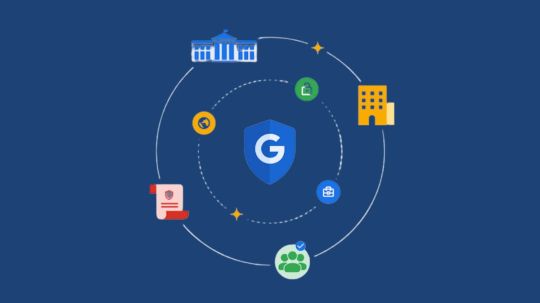
Google Secure AI Framework
A conceptual framework for cooperatively securing AI technologies is being released by Google.
AI has enormous promise, particularly generative AI. However, in order to develop and implement this technology responsibly, there must be clear industry security standards in place as it moves forward in these new areas of innovation. The Secure AI Framework (SAIF), a conceptual framework for secure AI systems.
Why SAIF is being introduced
Incorporating Google’s knowledge of security mega-trends and hazards unique to AI systems, Secure AI Framework draws inspiration from the security best practices it has implemented in software development, such as evaluating, testing, and managing the supply chain.
In order to ensure that responsible actors protect the technology that underpins AI developments and that AI models are secure by default when they are implemented, a framework spanning the public and private sectors is necessary.
At Google, they adopted a transparent and cooperative approach to cybersecurity over the years. To assist respond to and prevent cyberattacks, this entails fusing frontline intelligence, experience, and creativity with a dedication to sharing threat information with others. Building on that methodology, Secure AI Framework is intended to assist in reducing threats unique to AI systems, such as model theft, data poisoning of training data, quick injection of harmful inputs, and extraction of private information from training data. Following a bold and responsible framework will be even more important as AI capabilities are used in products worldwide.
Let’s now examine Secure AI Framework and its six fundamental components:
1. Provide the AI ecosystem with more robust security foundations
To safeguard AI systems, apps, and users, this involves utilizing secure-by-default infrastructure safeguards and knowledge accumulated over the previous 20 years. Develop organizational knowledge to stay up with AI developments while beginning to expand and modify infrastructure defenses in light of changing threat models and AI. For instance, companies can implement mitigations like input sanitization and limiting to assist better defend against prompt injection style attacks. Injection techniques like SQL injection have been around for a while.
2. Expand detection and response to include AI in the threat landscape of an organization
When it comes to identifying and handling AI-related cyber incidents, promptness is essential, and giving an organization access to threat intelligence and other capabilities enhances both. This involves employing threat intelligence to foresee assaults and keeping an eye on the inputs and outputs of generative AI systems to identify irregularities for companies. Usually, cooperation with threat intelligence, counter-abuse, and trust and safety teams is needed for this endeavor.
3. Automate defenses to stay ahead of both new and current threats
The scope and velocity of security incident response activities can be enhanced by the most recent advancements in AI. It’s critical to employ AI and its existing and developing capabilities to stay agile and economically viable when defending against adversaries, who will probably use them to scale their influence.
4. Align platform-level rules to provide uniform security throughout the company
To guarantee that all AI applications have access to the finest protections in a scalable and economical way, control framework consistency can help mitigate AI risk and scale protections across various platforms and technologies. At Google, this entails incorporating controls and safeguards into the software development lifecycle and expanding secure-by-default safeguards to AI platforms such as Vertex AI and Security AI Workbench. The firm as a whole can gain from state-of-the-art security by utilizing capabilities that cater to common use cases, such as Perspective API.
5.Adjust parameters to mitigate and speed up AI deployment feedback loops
Continuous learning and testing of implementations can guarantee that detection and prevention capabilities adapt to the ever-changing threat landscape. In addition to methods like updating training data sets, adjusting models to react strategically to attacks, and enabling the software used to create models to incorporate additional security in context (e.g. detecting anomalous behavior), this also includes techniques like reinforcement learning based on incidents and user feedback. To increase safety assurance for AI-powered products and capabilities, organizations can also regularly perform red team exercises.
6. Put the hazards of AI systems in the context of related business procedures
Last but not least, completing end-to-end risk assessments on an organization’s AI deployment can aid in decision-making. An evaluation of the overall business risk is part of this, as are data lineage, validation, and operational behavior monitoring for specific application types. Companies should also create automated tests to verify AI’s performance.
Why we are in favor of a safe AI community for everybody
To lower total risk and increase the standard for security, it has long supported and frequently created industry guidelines. Its groundbreaking work on its BeyondCorp access model produced the zero trust principles that are now industry standard, and it has partnered with others to introduce the Supply-chain Levels for Software Artifacts (SLSA) framework to enhance software supply chain integrity. These and other initiatives taught us that creating a community to support and further the work is essential to long-term success.
How Google is implementing Secure AI Framework
Five actions have already been taken to promote and develop a framework that benefits everyone.
With the announcement of important partners and contributors in the upcoming months and ongoing industry involvement to support the development of the NIST AI Risk Management Framework and ISO/IEC 42001 AI Management System Standard (the first AI certification standard in the industry), Secure AI Framework is fostering industry support. These standards are in line with SAIF elements and mainly rely on the security principles included in the NIST Cybersecurity Framework and ISO/IEC 27001 Security Management System, in which Google will be taking part to make sure upcoming improvements are appropriate to cutting-edge technologies like artificial intelligence.
Assisting businesses, including clients and governments, in understanding how to evaluate and reduce the risks associated with AI security. This entails holding workshops with professionals and keeping up with the latest publications on safe AI system deployment best practices.
Sharing information about cyber activities involving AI systems from Google’s top threat intelligence teams, such as Mandiant and TAG.
Extending existing bug hunters initiatives, such as Google Vulnerability Rewards Program, to encourage and reward AI security and safety research.
With partners like GitLab and Cohesity, it will keep providing secure AI solutions while expanding its skills to assist clients in creating safe systems.
Read more on Govindhtech.com
#generativeAI#AIFramework#SecureAIFramework#AItechnologies#AImodels#AIcapabilities#AIapplications#VertexAI#NISTCybersecurity#News#Technews#Technology#technologynews#technologytrends#govindhtech
0 notes
Text
Why Use The Upgraded Claude 3.5 Sonnet Model On Vertex AI
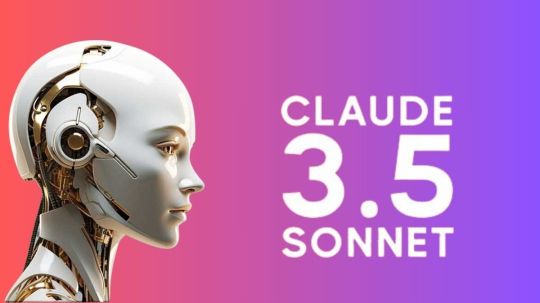
Introducing Vertex AI’s Upgraded Claude 3.5 Sonnet from Anthropic
In order to offer the most potent AI tools available together with unmatched choice and flexibility, Google Cloud has built its Vertex AI platform in an open manner. For this reason, Vertex AI gives you access to more than 160 models, including third-party, open-source, and first-party models, enabling you to create solutions that are especially suited to your requirements.
It declared in June that Vertex AI Model Garden now includes Anthropic’s Claude 3.5 Sonnet. Google Cloud announcing today that the upgraded Claude 3.5 Sonnet , which includes a new “computer use” capability in public beta on Vertex AI Model Garden, is now generally available for US clients. This implies that you may instruct the model to produce computer actions, including as keystrokes and mouse clicks, using the upgraded Claude 3.5 Sonnet enabling it to communicate with your user interface (UI). The upgraded Claude 3.5 Sonnet model is available to you via Model-as-a-Service (MaaS) solution.
Additionally, in the upcoming weeks, Vertex AI will also offer Claude 3.5 Haiku, Anthropic’s quickest and most affordable model.
Why Use Vertex AI’s Upgraded Claude 3.5 Sonnet Model?
Vertex AI streamlines the process of testing, implementing, and maintaining models such as the upgraded Claude 3.5 Sonnet by acting as a single AI platform.
By fusing Vertex AI’s strength with the intelligence of Claude 3.5 models, you can:
Try things with assurance
Vertex AI offers the enhanced Claude 3.5 Sonnet as a fully managed Model-as-a-Service. Without having to worry about complicated deployment procedures, MaaS allows you to do thorough tests in an intuitive environment and explore Claude 3.5 models with straightforward API requests.
Launch and operate without overhead
Simplify the deployment and scaling process. Claude 3.5 models offer pay-as-you-go or allocated throughput price options, as well as fully managed infrastructure tailored for AI workloads.
Create complex AI agents
Utilize Vertex AI’s tools and the enhanced Claude 3.5 Sonnet’s special features to create agents.
Construct with enterprise-level compliance and security
Make use of Google Cloud‘s integrated privacy, security, and compliance features. Enterprise controls, like the new organization policy for Vertex AI Model Garden, offer the proper access controls to guarantee that only authorized models are accessible.
Build with enterprise-grade security and compliance
It carefully curated library of more than 160 enterprise-ready first-party, open-source, and third-party models in Model Garden has grown with the inclusion of the Claude 3.5 models to Vertex AI. This allows you to choose the best models for your requirements through open and adaptable AI ecosystem.
Customers building with Anthropic’s Claude models on Vertex AI
Global energy provider AES uses Claude on Vertex AI to expedite energy safety assessments, greatly cutting down on the amount of time needed for this crucial yet time-consuming task:
Millions of people use Zapia, a personal AI assistant powered by Claude on Vertex AI, which is developed by BrainLogic AI, a firm creating innovative AI solutions especially for Latin America:
Through its AI-powered chat platform, Poe, Quora, the worldwide knowledge-sharing website, is utilizing Claude’s skills on Vertex AI to enable millions of daily interactions:
It is thrilled to collaborate with Anthropic and keep offering Google Cloud clients cutting-edge innovation backed by an open and accessible AI ecosystem.
How to get started with the upgraded Claude 3.5 Sonnet on Google Cloud
Choose the Claude 3.5 Sonnet v2 model tile by going to Model Garden. The upgraded Claude 3.5 Sonnet is also readily available on the Google Cloud Marketplace, where you can additionally benefit from the possibility of reducing your Google Cloud cost obligations. Today, only consumers in the United States can purchase the enhanced Claude 3.5 Sonnet v2.
Click “Enable,” then adhere to the next steps.
Start constructing by using sample notebook and documentation.
Safety & Trust
Every stage of Anthropic’s AI development is influenced by its dedication to safety. It carried out comprehensive safety assessments across several languages and policy domains when developing Claude 3.5 Haiku. Additionally, improved Claude’s capacity to handle delicate material with caution. Google Cloud in-house testing demonstrates that Claude 3.5 Haiku maintains exacting safety standards while delivering significant capability improvements.
Read more on govindhtech.com
#UpgradedClaude35#VertexAI#SonnetModel#GoogleCloud#ai#Claude35Haiku#GoogleCloudMarketplace#SafetyTrust#Anthropic#Customersbuilding#technology#technews#news#govindhtech
0 notes