#Big data analytics in research
Explore tagged Tumblr posts
Text
The Role of Technology in Unveiling Knowledge Horizons
Introduction In today’s rapidly evolving world, technology plays a pivotal role in reshaping the horizons of knowledge. The unprecedented pace at which technology advances enables us to access, analyze, and disseminate information like never before. This article delves into how technology is unveiling new knowledge horizons, transforming education, research, communication, and societal…
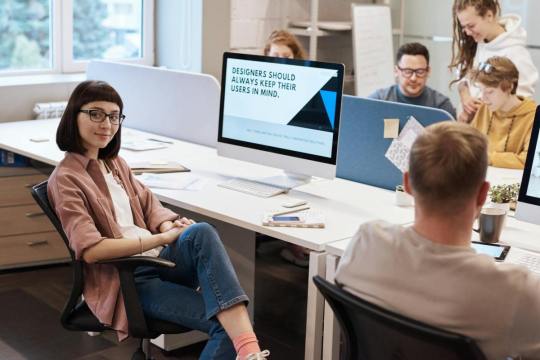
View On WordPress
#Artificial intelligence in education#Augmented reality learning#Big data analytics in research#Blogs and online publications#Bridging the knowledge gap#Collaborative research platforms#Digital Libraries#Digital literacy campaigns#E-learning platforms#Global collaboration in research#Knowledge dissemination#Online education#Open access journals#Podcasts and webinars#Remote learning programs#Social media and knowledge sharing#Technology and education#Telemedicine and healthcare#Virtual reality in education
0 notes
Text
प्रचंड उपलब्ध माहितीच्या विश्लेषणासाठी तज्ज्ञांची मोठी गरज
शिवाजी विद्यापीठात आंतरराष्ट्रीय संख्याशास्त्र परिषदेत सूर; यशस्वी सांगता कोल्हापूर : एकविसाव्या शतकात डेटा प्रचंड प्रमाणात उपलब्ध आहे, तितक्याच मोठ्या प्रमाणात त्याच्या गतिमान संख्याशास्त्रीय विश्लेषणाचीही आवश्यकता आहे. समाजाच्या समस्याही मोठ्या आहेत. त्या सोडविण्यासाठी या क्षेत्रामध्ये सामाजिक जाणिवेच्या भावनेतून, आधुनिक ज्ञानसाधनांच्या आधारे काम करणाऱ्या संशोधक, विश्लेषकांची मोठी गरज आहे.…
#2025 events#२०२५ परिषद#academic conference#big data#data analytics#data interpretation#data science#data scientists#data-driven research#Digambar Shirke#expert analysts#higher education#international conference#Kolhapur#modern research tools#official statistics#Pramod Patil#research collaboration#research innovation#Shivaji University#statistical analysis#statistical education#statistical expertise#statistical methods#statistics conference#university news#अभ्यास संमेलन#आंतरराष्ट्रीय परिषद#आधुनिक संशोधन#उच्चशिक्षण
0 notes
Text

#Big Data Analytics in Retail Market#Big Data Analytics in Retail Market Share#Big Data Analytics in Retail Market Size#Big Data Analytics in Retail Market Research#Big Data Analytics in Retail Industry#What is Big Data Analytics in Retail?
0 notes
Text
The Illusion of Influence: An Examination of the Media, Security Agencies, and Technological Power in Shaping Public Perception
Introduction In today’s digital age, the boundary between reality and illusion has blurred significantly. This essay explores how the perception of magical influence, akin to saying “hocus pocus” and seeing changes unfold, mirrors the intricate interplay between journalism, security agencies, state agencies, and information specialists in contemporary society. By examining these mechanisms and…
#Advanced Analytics#augmented reality#behavioral analysis#Behavioral Insights#Big Data#control mechanisms#data analysis#data collection#Data Management#data mining#Data Privacy#data security#Design#digital age#digital culture#Digital Design#Digital Dynamics#Digital Dynamics Research#digital identity#Digital Impact#Digital Influence#Digital Influence Factors#Digital Information#Digital Innovations#Digital Marketing#digital media#Digital Media Influence#Digital Perception#digital surveillance#digital technology
0 notes
Text
Kerala establishes seven advanced research centers of excellence.
Thiruvananthapuram: The Kerala government has approved the establishment of seven Centers of Excellence, which would operate as independent institutions and concentrate on various fields of advanced research and training. These will be manned by elite teachers, researchers, and students, and furnished with cutting-edge amenities.
ALSO READ MORE-https://apacnewsnetwork.com/2024/07/kerala-establishes-seven-advanced-research-centers-of-excellence/
#big data analytics#Calicut University#Cochin University of Science and Technology#Kerala Government#nanotechnology#seven advanced research centers#seven advanced research centers of excellence#sustainable fuels#systems biology#waste management
0 notes
Text
Desktop Trace Drug Detector
Labtron Desktop Trace Drug Detector offers rapid, accurate detection of trace amounts of narcotics with a sensitivity limit of 100 ng for TNT and an 8 second analysis time. Features include an audio and visual alert system and advanced ion mobility spectrometry technology, providing real-time results, and ensuring reliable identification of a wide range of drugs.
1 note
·
View note
Text
How Big Data Analytics is Changing Scientific Discoveries
Introduction
In the contemporary world of the prevailing sciences and technologies, big data analytics becomes a powerful agent in such a way that scientific discoveries are being orchestrated. At Techtovio, we explore this renewed approach to reshaping research methodologies for better data interpretation and new insights into its hastening process. Read to continue
#CategoriesScience Explained#Tagsastronomy data analytics#big data analytics#big data automation#big data challenges#big data in healthcare#big data in science#big data privacy#climate data analysis#computational data processing#data analysis in research#data-driven science#environmental research#genomics big data#personalized medicine#predictive modeling in research#real-time scientific insights#scientific data integration#scientific discoveries#Technology#Science#business tech#Adobe cloud#Trends#Nvidia Drive#Analysis#Tech news#Science updates#Digital advancements#Tech trends
1 note
·
View note
Link
#market research future#healthcare big data analytics#healthcare big data market#healthcare big data industry
0 notes
Text
The vast amount of data available for retailers today is helping them drive a better, enhanced tailored segmentation for customers different needs and preferences.
Contact Information:
Address: PO Box: 127239, Business Bay, Dubai, UAE
Ph: +971 (04)4431578
email: [email protected]
Website: www.marketwaysarabia.com
#Machine Learning Consultancy uae#Big Data Analytics Consultancy uae#Artificial Intelligence research Consultancy uae#Data Mining & Analytics Consultancy dubai uae
0 notes
Text
Big Data Analysis Company in Kolkata
Introduction
In the dynamic landscape of technology, big data has emerged as a game-changer for businesses worldwide. As organizations in Kolkata increasingly recognize the importance of harnessing data for strategic decision-making, the role of big data analysis companies has become pivotal.

The Rise of Big Data in Kolkata
Kolkata, known for its rich cultural heritage, is also witnessing remarkable growth in the realm of big data. Over the years, the city has transitioned from traditional methods to advanced data analytics, keeping pace with global trends.
Key Players in Kolkata’s Big Data Scene
Prominent among the contributors to this transformation are the leading big data analysis companies in Kolkata. Companies like DataSolve and AnalytixPro have carved a niche for themselves, offering cutting-edge solutions to businesses across various sectors.
Services Offered by Big Data Companies
These companies provide a range of services, including data analytics solutions, machine learning applications, and customized big data solutions tailored to meet the unique needs of their clients.
Impact on Business Decision-Making
The impact of big data on business decision-making cannot be overstated. By analyzing vast datasets, companies can gain valuable insights that inform strategic decisions, leading to increased efficiency and competitiveness.
Challenges and Solutions
However, the journey toward effective big data implementation is not without challenges. Big data companies in Kolkata face issues like data security and integration complexities. Innovative solutions, such as advanced encryption algorithms and seamless integration platforms, are being developed to address these challenges.
Future Prospects
Looking ahead, the future of big data in Kolkata appears promising. The integration of artificial intelligence and the Internet of Things is expected to open new avenues for data analysis, presenting exciting possibilities for businesses in the city.
Case Study: Successful Big Data Implementation
A closer look at a successful big data implementation in Kolkata reveals how a major e-commerce player utilized data analytics to enhance customer experience and optimize supply chain management.
Training and Skill Development
To keep up with the evolving landscape, there is a growing emphasis on training and skill development in the big data industry. Institutes in Kolkata offer comprehensive programs to equip professionals with the necessary skills.
Big Data and Small Businesses
Contrary to popular belief, big data is not exclusive to large enterprises. Big data companies in Kolkata are tailoring their services to suit the needs of small businesses, making data analytics accessible and affordable.
Ethical Considerations in Big Data
As the volume of data being processed increases, ethical considerations become paramount. Big data companies in Kolkata are taking steps to ensure data privacy and uphold ethical standards in their practices.
Expert Insights
Leading experts in the big data industry in Kolkata share their insights on current trends and future developments. Their perspectives shed light on the evolving nature of the industry.
Success Stories
Success stories from businesses in Kolkata highlight the transformative power of big data. From healthcare to finance, these stories underscore the positive impact that data analysis can have on diverse sectors.
Tips for Choosing a Big Data Analysis Company
For businesses considering a partnership with a big data company, careful consideration of factors such as experience, scalability, and data security is crucial. Avoiding common pitfalls in the selection process is key to a successful partnership.
Conclusion
In conclusion, the journey of big data analysis company in Kolkata reflects a broader global trend. As businesses increasingly recognize the value of data, the role of big data analysis companies becomes indispensable. The future promises even greater advancements, making it an exciting time for both businesses and big data professionals in Kolkata.
Know more:
Oil and Gas Software Development Company in kolkata, Oil and Gas Software Development Services in kolkata
banking software development company, banking software development services, bank software development, banking financial software development
opentable mobile app, restaurant mobile app, best restaurant apps, restaurant app ordering system, restaurant ordering system using mobile application
Best recruitment portal in Kolkata, job portal development services, job portal development company, online job portal development, job portal website development, recruitment portal development
mobile app development company, mobile application development, app development company, mobile app development services, android app development company
hr management software, human resource management system software, human resource management information system, best hr management software, cloud based hr software, best hrms software company in Kolkata
Agriculture software Development company in Kolkata, Agricultural Statistics Database Management in kolkata, Agricultural Application Development in kolkata
#data analysis#big data analytics#statistical analysis#descriptive statistics and inferential statistics#business data analyst#statistical analysis in research#data analytics companies#financial data analytics#statistics and data analysis
0 notes
Text
AI’s Life-Changing, Measurable Impact on Cancer
New Post has been published on https://thedigitalinsider.com/ais-life-changing-measurable-impact-on-cancer/
AI’s Life-Changing, Measurable Impact on Cancer
Leveraging Big Data to Enhance AI in Cancer Detection and Treatment
Integrating AI into the healthcare decision making process is helping to revolutionize the field and lead to more accurate and consistent treatment decisions due to its virtually limitless ability to identify patterns too complex for humans to see.
The field of oncology generates enormous data sets, from unstructured clinical histories to imaging and genomic sequencing data, at various stages of the patient journey. AI can “intelligently” analyze large-scale data batches at faster speeds than traditional methods, which is critical for training the machine learning algorithms that are foundational for advanced cancer testing and monitoring tools. AI also has tremendous inherent pattern recognition capabilities for efficiently modeling data set complexities. This is important because it enables deeper, multi-layered understandings of the impact of nuanced molecular signatures in cancer genomics and tumor microenvironments. Discovering a pattern between genes only found in a certain subset of cancer cases or cancer progression patterns can lead to a more tailored, patient-specific approach to treatment.
What is the ultimate goal? AI-powered cancer tests that support clinical decision-making for doctors and their patients at every step of the cancer journey – from screening and detection, to identifying the right treatment, and for monitoring patients’ response to interventions and predicting recurrence.
Data Quality and Quantity: The Key to AI Success
Ultimately, an AI algorithm will only be as good as the quality of data that trains it. Poor, incomplete or improperly labeled data can hamstring AI’s ability to find the best patterns (garbage in, garbage out). This is especially true for cancer care, where predictive modeling relies on impeccable precision – one gene modification out of thousands, for example, could signal tumor development and inform early detection. Ensuring that high level of quality is time-consuming and costly but leads to better data, which results in optimal testing accuracy. However, developing a useful goldmine of data comes with significant challenges. For one, collecting large-scale genomic and molecular data, which can involve millions of data points, is a complex task. It begins with having the highest quality assays that measure these characteristics of cancer with impeccable precision and resolution. The molecular data collected must also be as diverse in geography and patient representation as possible to expand the predictive capacity of the training models. It also benefits from building long-term multi-disciplinary collaborations and partnerships that can help gather and process raw data for analysis. Finally, codifying strict ethics standards in data handling is of paramount importance when it comes to healthcare information and adhering to strict patient privacy regulations, which can sometimes present a challenge in data collection.
An abundance of accurate, detailed data will not only result in testing capabilities that can find patterns quickly and empower physicians with the best opportunity to address the unmet needs for their patients but will also improve and advance every aspect of clinical research, especially the urgent search for better medicines and biomarkers for cancer.
AI Is Already Showing Promise in Cancer Care and Treatment
More effective ways to train AI are already being implemented. My colleagues and I are training algorithms from a comprehensive array of data, including imaging results, biopsy tissue data, multiple forms of genomic sequencing, and protein biomarkers, among other analyses – all of which add up to massive quantities of training data. Our ability to generate data on the scale of quadrillions rather than billions has allowed us to build some of the first truly accurate predictive analytics in clinical use, such as tumor identification for advanced cancers of unknown primary origin or predictive chemotherapy treatment pathways involving subtle genetic variations.
At Caris Life Sciences, we’ve proven that extensive validation and testing of algorithms are necessary, with comparisons to real-world evidence playing a key role. For example, our algorithms trained to detect specific cancers benefit from validation against laboratory histology data, while AI predictions for treatment regimens can be cross compared with real-world clinical survival outcomes.
Given the rapid advancements in cancer research, experience suggests that continuous learning and algorithm refinement is an integral part of a successful AI strategy. As new treatments are developed and our understanding of the biological pathways driving cancer evolves, updating models with the most up-to-date information offers deeper insights and enhances detection sensitivity.
This ongoing learning process highlights the importance of broad collaboration between AI developers and the clinical and research communities. We’ve found that developing new tools to analyze data more rapidly and with greater sensitivity, coupled with feedback from oncologists, is essential. Bottom-line: the true measure of an AI algorithm’s success is how accurately it equips oncologists with reliable, predictive insights they need and how adaptable the AI strategy is to ever-changing treatment paradigms.
Real-World Applications of AI Are Already Increasing Survival Rates and Improving Cancer Management
Advances in data scale and quality have already had measurable impacts by expanding the physician decision-making toolkit, which has had real-world positive results on patient care and survival outcomes. The first clinically validated AI tool for navigating chemotherapy treatment choices for a difficult-to-treat metastatic cancer can potentially extend patient survival by 17.5 months, compared to standard treatment decisions made without predictive algorithms1. A different AI tool can predict with over 94% accuracy the tumor of origin for dozens of metastatic cancers2 – which is critical to creating an effective treatment plan. AI algorithms are also predicting how well a tumor will respond to immunotherapy based on each person’s unique tumor genetics. In each of these cases, AI toolkits empower clinical decision-making that improves patient outcomes compared with current standards of care.
Expect An AI Revolution in Cancer
AI is already changing how early we can detect cancer and how we treat it along the way. Cancer management will soon have physicians working side-by-side with integrated AI in real time to treat and monitor patients and stay one step ahead of cancer’s attempts to outwit medicines with mutations. In addition to ever-improving predictive models for detecting cancer earlier and providing more effective personalized treatment paradigms, physicians, researchers, and biotech companies are hard at work today to leverage data and AI analyses to drive new therapeutic discoveries and molecular biomarkers for tomorrow.
In the not-too-distant future, these once-impossible advances in AI will reach far beyond cancer care to all disease states, ending an era of uncertainty and making medicine more accurate, more personalized, and more effective.
#ADD#ai#AI in healthcare#AI strategy#AI-powered#algorithm#Algorithms#analyses#Analysis#Analytics#applications#approach#Big Data#biomarkers#biopsy#biotech#Building#Cancer#Cancer Care#cancers#challenge#chemotherapy#clinical#clinical research#Collaboration#Companies#comprehensive#continuous#data#data collection
0 notes
Text

#Big Data Analytics Market#Big Data Analytics Market Share#Big Data Analytics Market Size#Big Data Analytics Market Research#Big Data Analytics Industry#What is Big Data Analytics?
0 notes
Text
Mercury in the Houses
paid readings | Masterlist
1st house When Mercury resides here, your very essence speaks. Your intellect shapes your persona; you are a naturally curious individual, expressing yourself directly and often with remarkable openness. This placement signifies a mind that is constantly engaged with learning, readily absorbing information from your surroundings and projecting your thoughts outward. You possess a distinct verbal presence, making your initial impressions impactful.
2nd house Mercury in this house means your mind focuses on resources and values. You think practically about money, possessions, and security. Communication skills are often tied to earning or acquiring. You might articulate well about finances, business ventures, or what you deem worthwhile. This placement suggests a cleverness regarding material matters, and perhaps a talent for verbalizing your worth.
3rd house This is Mercury's natural domain, intensifying its qualities. Your intellect is incredibly agile, absorbing vast amounts of data from your immediate environment. You are a natural communicator, excelling in everyday conversations, writing, and short journeys. Curiosity drives you to learn continuously, making you adept at various forms of expression and connecting with siblings or local communities.
4th house Mercury in this position��indicates a mind deeply connected to home, family, and personal roots. Your thoughts often revolve around domestic matters, security, and your heritage. Communication within the family unit is crucial, and you may enjoy intellectual discussions at home. This placement suggests a reflective intellect, often seeking inner peace through understanding your foundational experiences.
5th house Mercury here indicates that you have a mind that's has a natural disposition towards the arts and creativity. You communicate with zest, finding joy in self-expression and intellectual challenges. This position suggests a talent for entertainment, teaching, or any activity where you can blend wit with imaginative flair.
6th house Mercury in this house means your intellect is directed towards practical concerns, work, and well-being. You possess an analytical mind, excelling at organization, problem-solving, and managing details. Communication is precise and efficient, often focused on daily routines, service to others, or health matters. This placement highlights a knack for methodical thinking and a desire for order.
7th house Mercury here emphasizes intellect in partnerships and relationships. You seek mental stimulation from others, thriving on discussions and exchanges of viewpoints. Communication is key to your one-on-one interactions, and you often prefer fair, balanced dialogue. This position suggests a person who learns through relating to others and may be drawn to intellectual alliances.
8th house This placement points to a profound and investigative mind. You delve into mysteries, hidden truths, and complex subjects like psychology, shared resources, or transformation. Your communication is often intense and probing, seeking deeper understanding beneath the surface. You may be drawn to research, occult studies, or uncovering secrets.
9th house Mercury in this house means your intellect expands into higher learning, philosophy, and foreign cultures. You possess a broad perspective, eager to explore different belief systems and distant lands. Communication is often philosophical, inspiring, and focused on big ideas. This position suggests a natural teacher, traveler, or someone deeply interested in global thought.
10th house Mercury in this house places your intellect and communication firmly in your career and public image. You express yourself professionally, often through writing, speaking, or strategic planning within your vocation. Your mind is geared towards achieving success and establishing authority. This placement indicates a person whose reputation is shaped by their articulate nature and intellectual contributions.
11th house Here, Mercury's influence extends to your social groups, aspirations, and humanitarian ideals. Your intellect thrives in collective settings, engaging in discussions about future possibilities and shared objectives. You communicate effectively within teams, contributing innovative ideas and fostering connections based on mutual interests. This position suggests a mind focused on progress and community.
12th house When Mercury is in this house, your intellect operates in subtle, often hidden ways. You possess a highly intuitive and introspective mind, processing information through dreams, intuition, and unspoken cues. Communication may be less direct, leaning towards creative expression, spiritual contemplation, or working behind the scenes. This placement suggests a mind that finds peace in solitude and deep reflection.
DISCLAIMER: This post is a generalisation and may not resonate. I recommend you get a reading from an astrologer (me). If you want a reading from me check out my sales page.
@astrofaeology private services 2025 all rights reserved
577 notes
·
View notes
Text
The Future of Market Research: Unveiling the Top 10 Emerging Trends
The landscape of market research is undergoing a transformative shift, driven by the convergence of technology, consumer behavior, and data-driven insights. Embracing these six emerging trends empowers businesses to connect with their target audiences on a deeper level, adapt to changing market dynamics, and make informed decisions that drive success
#Artificial intelligence (AI)#Augmented reality (AR) and virtual reality (VR)#Automation#Big data#Blockchain technology#Consumer behavior#Customer experience (CX)#Data analytics#Digital transformation#Emerging trends#Ethnographic research#Future of market research#Internet of Things (IoT)#Machine learning#market research#market xcel#Mobile market research#Personalization#Predictive analytics#Social media analytics#Voice of the customer (VoC)
0 notes
Text
Since Lando is involved, there's of course a particular narrative that has popped up around his Monster release and which other drivers deserve it more, so I'm going to get on my professional soap box once again because oh my god shut the fuck up already.
My creds: dual BS in Business Analytics and Marketing. MA in Strategic Communication (thesis on sports PR in the social media age). MBA with a sports economics coursework emphasis. Consultant working in corporate partnerships in a variety of sports, including motorsport.
Based on what I've seen today, people have no idea how much work goes into securing personal sponsors. In order to get a personal sponsorship deal, you and your team have to pitch the sponsor and demonstrate the value that it will bring to their business through things like DATA and RESEARCH. Engagement metrics, impressions, reach, products sold, brand recognition, return on investment, etc.
If a McLaren sponsor does a personal sponsorship of Lando as well, it's because his team pitched and demonstrated that the metrics bear out that it would be worth their money. It's not like oh let's throw money at this kid bc VIBES. Or bc Zak Brown says we had to. I keep seeing people implying that they just picked him on a whim, when things like this take ages to decide, with a ton of data, a ton of research, and a ton of really smart people analyzing it before making the call.
You have to show a sponsor the reasons that they should work with you and why it's worth their money. Lando and Quadrant have done that. And it's a fuckton of work to not only get them, but to deliver the results to retain them. Some of those results are in the form of social media engagement that they've gotten from Lando and his brands likely before the drink was even contracted.
Identifying sponsors, pitching and securing their money, etc. is a multi-billion dollar industry that requires a ton of work, data analysis, content testing, focus group testing, etc. The people saying "it should have been _____" clearly either have a personal bias or don't understand the level of personal brand you have to have to get this sort of a deal.
Lando has larger reach, more engagement, recognizable brands with very passionate followings, etc. when compared to some of the other drivers people are bringing up here. He's selling out merch collection after merch collection after merch collection, and that is not true of 90% of the other drivers on the grid. He sold so many tickets to Landostand that they quite literally BUILT ANOTHER GRANDSTAND. And sold that one out, too! These are things that come with a ton of value to sponsors. Sponsors are seeking out his audience based on demographics. It's not some sort of conspiracy, it's business.
Right now, there are a couple of drivers that are LEAGUES above the others when it comes to the effort they've put into developing their personal brand, ability to drive product, and relevancy within high disposable income and retail-spending fan demographics (Lando over indexes with four key demos: young women, highly educated women, queer men, families with children). Averaged across these "big spender demos," Lando is in the top 2. I can say that based on the data I have at my fingertips.
tl;dr - Monster is looking to sell product. Data says Lando Norris sells product because people actually like him very much.
#lando norris#lando x monster#please do not make me have to get on my soapbox again#normally i charge people a billable hourly rate for this shit
161 notes
·
View notes
Text
Snezka and SCP-049 beginning (lore)
1. Dr. Snezhana Lewandowski lore (Snezka)
(Events before the containment breach).
In the corridors of the SCP Foundation, where secrets and anomalies are hidden from the public eye, Dr. Snezhana Lewandowski worked. Her life was full of mystery, and this is what drew her to the world of anomalies.
- - - - - - - - - - - - - - - - - - - - - - - - - -
Name: Snezhana Lewandowski
Age: ██
Date of Birth: 27/08/ ████
Clearance Level: Third
Personnel Category: B
Specialty: IT Specialist. Psychologist.
Place of work: Zone 19
Foundation experience: 5 years
- - - - - - - - - - - - - - - - - - - - - - - - - - -
Education
First: IT-Specialist.
Snezhana enrolled in the Faculty of Information Technology at a state university. During her studies, she specialized in cybersecurity and data analytics.
After graduating with a Bachelor's degree, then a Master's degree, she continued her education in graduate school, where she focused on research in the areas of:
1. Information Security.
2. Big data analytics.
She defended her doctoral dissertation on "Anomaly detection methods for system and big data", which attracted the attention of the SCP Foundation.
Second: Psychologist.
After achieving the success she needed in technical education, she decided to fulfill her life's main dream of working in the field of psychology. Due to some unpleasant events in her childhood, she did not have the opportunity to study in this field, so she had to temporarily change her orientation in life.
From an early age, Snezhana has shown an interest in psychology and human behavior. The motivation for this comes from a tough youth and personal traumatic experiences. Her goal was to help those who couldn't make it on their own, as she once couldn't. After enough time had passed, she managed to apply to the academy to study psychology. Upon graduating from the academy, Snezhana specialized in the areas of:
1. Clinical psychology.
2. Experimental psychology.
3. Counseling psychology.
After she got a job in a psychoneurological dispensary, gaining valuable experience and practice, a broader understanding of the inner world of people.
Invitation to the Foundation
Finding contacts and personal information about Snezhana was not difficult for the Foundation. Having noticed her abilities, the Foundation ordered to send a recruiter - ████ ██████ - into her social circle to get more information about the candidate. As a result, the recruiter ████ and Snezhana became close friends.
After some time of observation and communication of the recruiter with her, having received all the necessary information, the Board of the Foundation came to the decision to give her the invitation to work.
Realizing the risks and danger, Snezhana accepted the invitation, having resigned from her previous job after working for about 2 years.
After testing, interviewing and training, she became an employee of the Foundation with a level 2 security clearance.
Career at the Foundation
Snezhana started her career as a junior specialist in cybersecurity, a little later gaining a junior position in psychology.
Her specialization is a unique combination of programming and psychology, allowing her to look at anomalies from two perspectives: both as complex systems that require analysis, and as entities with their own consciousness and motivation.
Incident
While analyzing data, Dr. Lewandowski came across strange signals on the network. These were not just hacking attempts - they were coming from an entity known as SCP-███ She and others in the programming field developed a comprehensive defense strategy. In the process, they faced many challenges: the SCP-███ system was constantly adapting, and they had to find new solutions. Under conditions of stress and strain, Dr. Lewandowski managed to implement their real-time protection algorithm.
After that, for some reason, she was assigned more work in the field of psychology. Also, her success in the incident gave her a promotion to level 3 clearance.
In psychology
Dr. Lewandowski researches and analyzes object psychology. She develops methods of interacting with abnormal entities and helps in understanding their behavior.
Real security is achieved not only through physical barriers, but also through the emotional stability of employees. She took time to help her colleagues through seminars and psychology trainings for the staff of the Facility, trying to create an atmosphere of trust and mutual support among the employees.
For about 2 years, Dr. Lewandowski worked only with Safe Class facilities, and after being promoted to Senior Specialist in Psychology, she was ordered to work with Euclid Class facilities.
This promotion led her to meet one subject that changed her life, and in the future, his too... SCP-049 - Plague Doctor.
2. Lewandowski and SCP-049
(Events on the eve of the containment breach).
Experiment
The first days of Dr. Lewandowski and the SCP-049 subject were formal, protocol-driven. Lewandowski conducted standard interview sessions in an attempt to understand the motives and nature of his subject. The subject was cool and detached.
She knew SCP-049 was dangerous, but she saw him not only as an anomaly, but as a complex, multifaceted individual. His words were full of sadness and loneliness, imbued with longing and melancholy. She understood his logic, his pain, his desire to “help,” even if the methods were questionable, but perhaps all is not what it seems at first glance.
And yet she too had thought similar things to what SCP-049 had said, that the world was sick, humanity needed to be saved. Ever since she was a child, she had had thoughts like his in her mind.
She had a theory that there were no bad people in the world, but wounded people who had not been healed. She believed that a person becomes wounded, after being treated unfairly or cruelly, by similarly wounded people. If a person does not work on their wound, it will not heal, because there are many wounded people in the world that will scratch that wound, making it bigger. And this pain and resentment, like a virus or rot, infects his soul completely, making the person the same as the one who once inflicted the wound. Later that person carries this pain and resentment further, taking it out on others, making more infected. It is an endless cycle that cannot be eradicated completely, but can be minimized. Some can handle it, and some need help. You have to want help, you have to understand the situation and try to solve it too, because no one can help you better than you can help yourself, and that's what Snezka had to go through.
She thought these thoughts were strange, but after so many years, there was a creature that literally reflected her thoughts, with whom you can share this theory, and perhaps get understanding.
She saw herself in him, only a better version of herself, but with the same mental problems.
— How can that be!? He's just an abnormal object, why can't I stop thinking about him!? I can't stop thinking about him, his words. Maybe it's my soft nature, too compassionate, empathic, or maybe it's his anomalous action?
Dr. Lewandowski felt a kindred spirit in him and wanted to have more sessions with him, and most importantly, she wanted to get away from formal conversation, and share her thoughts with him about their common problem.
She decided to send a request to the management above about the idea of having experimental conversations with SCP-049, so that he would see Dr. Lewandowski as genuinely understanding, get comfortable with her, and tell her more about his treatment, himself, and the fever. Approval was granted, but it was a long wait. Finally she could be sincere with him.
Trust
New conversations with SCP-049, but not as formal as before, it took several such conversations before SCP-049 settled down with Dr. Lewandowski.
He told her more about the fever, about the treatment, they began to have deep dialogues about life and death, about philosophy, about society, SCP-049 even began to tell stories from his own life. Lewandowski also told him about her life, but she liked to listen to him more.
She found comfort in his monologues about the world, about death, about eternity, his stories about medicine. Her mind resisted, but her heart could no longer deny it: she felt something more than professional interest in him. It was a dangerous game, bordering on insanity. She knew it, but she couldn't stop. Lewandowski realized that her feelings were illogical, unacceptable, but they were there. Her greatest fear was that the Foundation would suspect something and restrict her access to him, which unfortunately happened...
Another conversation between SCP-049 and Lewandowski, the subject had already started addressing her by her favorite version of her name, Snezka, while she addressed him as Doctor. Suddenly the object fell silent.
— Doctor? Is everything all right?
— ...Yes. Snezka, let me demonstrate something to you, you should know....
The subject held out his hand, as far as it was possible being handcuffed for safety's sake, and nodded, offering to touch her. Sensing that this venture might not end well, Lewandowski extended her palm, and SCP-049 touched it.
— Wait, what...? Oh... My thoughts about your abilities were valid. - Was the last thing Snezka said before the cell door opened and she was summoned to leave.
Reprimand
— Dr. Lewandowski, your experimental conversations with the subject have come to an end. We have all the information we need, you will no longer have to work with SCP-049. Let's be honest, it seems you've begun to abuse your access to the facility, and the Foundation board has some questions about that.
— ...It was part of an experiment, you see.... It gave you a lot of new information, before such experiments such informative results were not observed. - said Lewandowski in her defense.
— Lucky for you that it didn't end in your death! Objects like 049 are dangerous, you can't trust them or reach out to them. Or have you forgotten what he did to Dr. Hamm? And everything was fine at first, too. This object is unpredictable! You're lucky you're a valued member of our staff, and this is the first time you've had an incident like this, otherwise we'd be forced to take action.
Separation
More work with other facilities has now fallen on her. One day, she dared to inquire about SCP-049. But she didn't get a clear answer.
Meanwhile, SCP-049 was asking the same question to the staff - when would Dr. Lewandowski visit?
— We're sorry, but Dr. Lewandowski has declined to work with you, SCP-049.
— ...That can't be true, you're lying! She couldn't have done it, I can feel it!
Subject SCP-049 became hostile to the Foundation, uncooperative and drove all attempts at contact away.
All or Nothing
— "Is the dream and freedom worth risking the lives and safety of others?" — Pondered by Dr. Lewandowski . — "Risking everything, insanity, selfishness(?). But if it's for a great purpose, for the good of all mankind..."
INCIDENT ██-████
(Latest walkie-talkie recordings)
(Static noise and warning signal sound)
Operator One: (Confused) This is operator one. Security protocol has failed! Repeat, security protocol has failed! All subjects... all subjects are at large!
Agent █: Reporting a security breach in Sector 3. Doors unresponsive, cameras disabled. Looks like SCP-███ is out of control.
Commander ███████: be careful! Confirm the presence of the intruder and take action to apprehend him.
(Noise, sound of gunshots)
Agent ██: (panic) This is crazy! Reporting SCP-███ is not alone. There are several other objects present. Very aggressive, we need help, repeat ████████████████ (interference).
Commander ████████: Come in, Agent ██, come in!!! (communication lost).
Commander ████████: Fuck...
Dr. █████: It could be SCP-███! He could have hacked into the security system and released the other facilities.
Commander ████: █████, are you sure? How?!
Dr. █████: I can't say for sure, but... (struggle noise) HOW ARE YOU!? STOP, DOCTOR... (interference).
Commander ████: All personnel to the assault! Stop the targets at all costs! What the hell's going on! Where's all the security personnel!?
(explosions, shouting)
Agent █████: They're all over the place
Agent ██: Where's the ██ squad?
Agent █████: they're holding back SCP-682
Agent ██: he's the last fucking thing we need!
SB-2: SB-1, this is SB-2! We've got-- we've got SCP-███breaking through! We can't stop him!
SB-1: Hold him off at all costs! Support is on the way.
(Siren and explosions)
Operator Three: SB-1, this is Operator Three! The ██ camera signal is gone! SCP-█████... he's out!
SB-1: All SB personnel to camera ██! Isolate SCP-█████! Repeat, isolate SCP-█████! (radio interference)
End of Calm
There has been a massive failure of the entire Foundation security system in Area 19, as well as a power failure. Security protocol, alarms, and warnings went off with a delay with most of the dangerous objects wreaking havoc in the Foundation. The alleged culprit of the SCP-“”" incident. Likely had accomplices, an investigation is underway.
During the incident, a large number of soldiers and staff died. There was a leak of several objects to freedom. The lists of dead and missing were equal. Dr. Snezana Lewandowski and SCP-049 were also listed as missing, among dozens of personnel and facilities.
The SCP Foundation, having suffered loss and damage, is doing its best to restore order after the chaos, but the world is no longer safe as before.
3. Conversations between Lewandowski and SCP-049
Interviewer: Dr. Snezhana Lewandowski, Area 19
Interviewee: SCP-049
[START RECORDING]
Dr. Lewandowski: Good afternoon, SCP-049. I am your psychologist, Dr. Snezhana Lewandowski. It's a pleasure to meet you!
SCP-049: Greetings, doctor, I hope we can work together.
Dr. Lewandowski: I think so! So, how are you feeling today?
SCP-049: (pause) I feel... A burden. A burden that is hard to describe in words. Every day I see suffering, and it depresses me.
Dr. Lewandowski: Are you talking about human suffering? About those whom you refer to as sick people?
SCP-049: Yes. The weathering is not just a physical condition. This world, this wretched piece of the universe, is forever doomed to suffer. (Irritated) Mortals, powerless over their own weaknesses, forever seek salvation in false doctrines and vain hopes. They build cities out of flimsy material, create weapons that can destroy themselves, and cling to life without realizing its true nature. I see their pain, their despair, feel their fear of the inevitable. And I know I can help them. But they don't understand. They fear me! They reject my mercy! They call me a monster, a monstrosity! I see them trying to stop me, lock me up, impose their rules. But can you limit what is beyond their comprehension? Can one defeat that which is eternal? Their attempts are futile. I will always exist. Let them resist, let them fear. It only confirms their blindness.
(A moment's silence)
Dr. Lewandowski: (Sigh)...Have you ever thought about why this “fever” bothers you so much? What exactly causes you to feel so strongly about it?
SCP-049: (sighs) I have witnessed a lot of suffering. I have seen people lose hope, I have seen them fall victim to their weakness. This makes me want to help, but sometimes I feel that my efforts are futile.
Dr. Lewandowski: Do you feel that your help is really effective? Or is there any doubt about your methods?
SCP-049: (with some irritation) My methods are the only way to free people from their suffering! I cannot afford to doubt my vocation. But sometimes... It catches up with me.
Dr. Lewandowski: It is normal to have doubts. Many people face similar feelings. What do you do to deal with these emotions?
SCP-049: I continue my work. I focus on my goals.
Dr. Lewandowski: Maybe you should take time to reflect on yourself and your feelings. It may help you better understand your motivation and calm your mind.
SCP-049: I don't think so, but... You may be right. I worry that if I stop, the darkness around me will consume everything.
Dr. Lewandowski: (Pause)...How I understand that. Sometimes you have to take a step back to see the big picture. It doesn't mean you stop fighting the disease. It can only make you stronger.
SCP-049: Perhaps...
Dr. Lewandowski: This is an important step. And remember, you are not alone in your experience. I'm here to help you make sense of it.
SCP-049: Thank you... I'll try to remember that.
[END RECORDING]
- - - - - - - - - - - - - - - - - - - - - - - - - - - - - -
Interviewer: Dr. Snezhana Lewandowski, Area 19
Interviewee: SCP-049
[START RECORDING]
Dr. Lewandowski: Good evening Doctor, how are you feeling?
SCP-049: It would be better if I were allowed to return to my work.
Dr. Lewandowski: Don't worry Doctor, you will definitely be given that opportunity...
SCP-049: Really? Really!? I'm finally going back to work, I'm glad to hear that! When do I get a patient?
Dr. Lewandowski: ...Unfortunately, I don't have that information.
SCP-049: Maybe you could influence this situation? Explain my urgent need to progress with my treatment!
Dr. Lewandowski: I don't think I can... Doctor, you need to rest, you've been working hard. As a doctor, you should know that rest is very important for high productivity.
SCP-049: (Sighs) I wish I had something to do in these four walls...
Dr. Lewandowski: I have a suggestion. Do you like to read? I can bring you some books if the Foundation leadership says so.
SCP-049: That's a very marvelous idea.
Dr. Lewandowski: What kind of literature do you prefer?
SCP-049: I was interested in modern books about medicine, it is interesting to study what is being written about it now. It would be interesting to read something from philosophy.
Dr. Lewandowski: Excellent choice Doctor, we have the same taste in literature. Would you mind if I brought a couple more books on my recommendation for you?
SCP-049: Sounds good, I agree.
Dr. Lewandowski: See you, Doctor.
SCP-049: See you, thank you for such an interesting proposal, Dr. Lewandowski.
[END OF RECORDING]
- - - - - - - - - - - - - - - - - - - - - - - - - - - - - -
Interviewer: Dr. Snezhana Lewandowski, Area 19
Interviewee: SCP-049
[START RECORDING]
Dr. Lewandowski: Good evening, Doctor.
SCP-049: Good evening, Dr. Lewandowski.
Dr. Lewandowski: I want to understand how you feel when you look at the world through the prism of your...your uniqueness.
SCP-049: (hesitantly) Uniqueness?
Dr. Lewandowski: I'm talking about wisdom. You have lived for centuries, watching the birth and decline of civilizations. What have you learned in that time?
(Minute's silence)
SCP-049: I've seen nations rise and fall, empires born of dust and turned to stone, ideas ignite people's hearts and turn to ash. (Pause). I realized that existence is a perpetual cycle of birth and death, a constant struggle for survival. People are so hungry for meaning, but it eludes them.
Dr. Lewandowski: Isn't this struggle, this longing for meaning, the very essence of being?
SCP-049: (With sadness in his voice) Meaning... (Pause) It wanders like a ghost through the labyrinths of our consciousness. We search for it in religion, in science, in art, but it eludes us, leaving only emptiness.
Dr. Lewandowski: But don't you see, Doctor? You create your own meaning by helping people to get rid of the disease. You believe in what you're doing, Doctor. You believe it's necessary.
SCP-049: Faith... (Pause) This is another one of those ghosts that haunt us. I see things that others do not see, and it pains me. It pains me to see the suffering that is inevitable for all living things.
Dr. Lewandowski: And you're trying to help, Doctor...
SCP-049: (Interrupting) We are but pawns in the hands of fate. Our actions are insignificant in comparison to eternity.
Dr. Lewandowski: Don't say that, Doctor. You are not a pawn. You are the creator of your own destiny. And I believe you will find your path, your meaning.
SCP-049: (Heavy sigh) But not in this place, not here, not now.
Dr. Lewandowski: (Pause) You know Doctor... I'd like to share with you a thought that has been on my mind since I was a child.
SCP-049: What is it that's bothering you?
[recording interrupted for 5 minutes]
Dr. Lewandowski: I almost forgot, the Foundation gave me permission to bring you some books.
(Took several books out of her bag and placed a stack on the table next to the subject)
SCP-049: (With joy in her voice) Glad to hear that! Immensely grateful to the Foundation and of course to you, Dr. Lewandowski.
Dr. Lewandowski: (Smiling) I'm glad it lifted your spirits. See you later, we'll be sure to discuss what we read next time.
SCP-049: I look forward to seeing you.
[END RECORDING]
- - - - - - - - - - - - - - - - - - - - - - - - - - - - - -
Interviewer: Dr. Snezhana Lewandowski, Zone 19
Interviewee: SCP-049
[START RECORDING]
Dr. Lewandowski: Hello, Doctor! How are you today?
SCP-049: Good afternoon, Dr. Lewandowski. I'm feeling... quite well. I've been studying some of the books you brought me at the last meeting.
Dr. Lewandowski: Excellent! Which books did you enjoy the most?
SCP-049: I was impressed with the works on human nature. Their authors convey emotions and experiences in a very subtle way.
Dr. Lewandowski: Yes, literature can indeed be a great way to understand people. Is there anything that surprised you about these books?
SCP-049: I was surprised by how diverse human feelings are. Everyone experiences love, fear, and hope in their own way.
Dr. Lewandowski: Yes, humans are amazing creatures! What about you? Have you ever experienced something similar to love?
SCP-049: (Pause) I'm not sure. My life has been devoted to fighting the windfall, and I haven't given much thought to such feelings. But... sometimes I feel warm when I talk to you. (turns away)
Dr. Lewandowski: I'm glad to see your condition has improved markedly. I feel warm when we talk, too. (Pause) You are an unusually interesting conversationalist.
SCP-049: (surprised) Interesting? I've always thought of myself as rather... odd. But it's nice to hear you say that. You don't hear that very often in this place, in general... I've never heard it from you.
Dr. Lewandowski: Weird people can be very interesting! We all have our unique qualities. For example, your view of the world is something special, not strange. By the way, have you read that novel?
SCP-049: The Master and Margarita? Yes, an interesting work about the power of love and the struggle against darkness.
Dr. Lewandowski: I think there are so many layers and philosophical themes there. You know, you sound like the Master (Laughs).
SCP-049: Hmm... There's something, maybe, and you remind me of Marguerite.
Dr. Lewandowski: (Laughs) That's probably true. Unfortunately, I have to go. Don't forget, you deserve to be understood. And I'm here to help you do just that.
SCP-049: (With a slight sadness in his voice) Your support gives me strength.
Dr. Lewandowski: We all need each other's support.
SCP-049: I look forward to our future conversations.
[END OF RECORDING]
- - - - - - - - - - - - - - - - - - - - - - - - - - - - - -
Interviewer: Dr. Snezhana Lewandowski, Area 19
Interviewee: SCP-049
[START RECORDING]
Dr. Lewandowski: Good afternoon Doctor, I would like to know what your thoughts have been occupied with lately?
SCP-049: Greetings. (Pause) Relatively recently, I've been thinking about the nature of human cruelty.
Dr. Lewandowski: Cruelty... (Pause) Unfortunately, it permeates every facet of existence, like a shadow that follows the light.
SCP-049: Yes. (Sighs) I watch people, and it makes me sad how capable they are of terrible things. There is a darkness lurking in their hearts that sometimes overshadows even the brightest impulses.
Dr. Lewandowski: As it happens, humanity is a paradox. On the one hand, it creates beautiful works of art, science and culture, and on the other hand, it is capable of ruthless acts of violence and hatred.
SCP-049: Exactly. I see how people can be kind and compassionate, but at the same time they easily cross the line into becoming instruments of destruction. It causes me deep sadness and bewilderment.
Dr. Lewandowski: I share your feelings and support your thoughts, Doctor.
SCP-049: It is most gratifying to know that, Dr. Lewandowski. My mission is to rid mankind of disease. At times I am at a loss as to what to do about this inner cruelty? How do you heal a society that is tearing itself apart?
Dr. Lewandowski: You see, many of these atrocities come from fear, ignorance and hatred. Or perhaps they were mentally wounded once, and that wound, without proper treatment, began to fester, infecting the whole mind and soul.
SCP-049: (Interested) I like the way you think. Abuse is not just physical violence. It is also words that hurt, betrayals and indifference to the suffering of others. In each of these acts I see a reflection of the disease.
Dr. Lewandowski: (Sigh) Sadly, dreams of a world where humanity can overcome its cruelty and learn to love are utopian.
SCP-049: I'm not so sure about that. It's certainly not an easy task, the whole world and one me, the road to healing is long and thorny. But the more I work at it, the closer that peace comes.
Dr. Lewandowski: (Pause) Perhaps someday people will realize that cruelty is not part of their true nature. Perhaps they will learn to see in each other a reflection of themselves and learn to care for those who suffer. Perhaps... (Pause) But sometimes you get the idea that some people are so rotten in their souls that treatment will be useless, and the only thing left to do is to rid the world of that person so they don't hurt others. This is not humane, but in such a case it is possible to make sure that this person stays alive but is not capable of hurting others.
SCP-049: (Surprised) Your thoughts make sense. We've had a rather pleasant dialog. I've never had such an in-depth discussion with anyone else. I feel much better.
Dr. Lewandowski: (Smiling) That's wonderful, Doctor, I confess I don't often have someone to talk to about this either.
[END RECORDING]
- - - - - - - - - - - - - - - - - - - - - - - - - - - - - -
Interviewer: Dr. Snezhana Lewandowski, Area 19
Interviewee: SCP-049
[START RECORDING]
Dr. Lewandowski: Good afternoon, Doctor, how is your mood?
SCP-049: Greetings. My mood is a little down, without my work I'm having a hard time mentally.
Dr. Lewandowski: I'm sorry to hear that, Doctor. (Pause) Let me ask you something.
SCP-049: I'm listening to you.
Dr. Lewandowski: Do you have any pleasant memory from your life?
SCP-049: (Sighs) Unfortunately, there aren't many... (Pause) I do recall one. Yes, it was a time when I was out in the wild, searching for rare herbs and plants in the forest. No people and just the sounds of nature. And then I came to a field, a field of lavender. (Sighs) It's... It was a magical feeling. The field was filled with bright colors and the air was full of a light, slightly spicy aroma. I felt like I was in another world, away from my worries. I walked slowly among the flowers, watching the bees as they worked to gather nectar. The bees are not aware of the suffering that humans are experiencing, they are just doing their job, benefiting the world around them. It made me think: maybe that's what humanity needs - the simple pursuit of the good. (Pause) The whole day I was there. It was wonderful. I dream of being in that moment again one day.
Dr. Lewandowski: That's... It's a wonderful memory, very beautiful.
SCP-049: Yes... It's beautiful. You know, I feel a little better.
Dr. Lewandowski: (Smiling) That's wonderful to hear. Try to remember something else, good thoughts can smooth out your condition within these walls.
SCP-049: I'll try. Thank you for encouraging me to remember this.
[END RECORDING]
- - - - - - - - - - - - - - - - - - - - - - - - - - - - - -
Interviewer: Dr. Snezhana Lewandowski, Area 19
Interviewee: SCP-049
[START RECORDING]
Dr. Lewandowski: Good afternoon Doctor, how is your mood?
SCP-049: Good afternoon Snezka. I guess my mood is somewhere between average and good. Good to see you, how are you feeling?
Dr. Lewandowski: I'm not bad. I have something I'd like to give you. I had a hard time getting permission for it from the foundation, but....
(Took out of her bag a small pillow with a lavender flower print pillowcase and placed it on the table in front of the subject).
This is for you. I remember that you don't need sleep, but that's not what it's for. Rather it is for coziness, and your favorable mood. This pillow is stuffed with lavender.
SCP-049: (Surprised) It's for me?! What a wonderful gift, lavender really calms me down.
Dr. Lewandowski: I'm glad the gift is useful to you. I would have brought you something else, but unfortunately the Foundation has only authorized this one so far.
SCP-049: I am honored by such attention, thank you, Dr..... Snezka. (Pause) ...How did I not notice that... Your last name is...
Dr. Lewandowski: (Interested) Hmm?
SCP-049: Lewandowski, that last name comes from the Old Polish word levanda, which means lavender. What a wonderful coincidence, my favorite plant.
Dr. Lewandowski: (Smiles) Yes, that's right, my favorite too by the way. I am amazed at your knowledge, Doctor!
SCP-049: It is a pleasure to be appreciated and understood by you. I must admit, you are not out of my mind.
Dr. Lewandowski: (Embarrassed) Oh... You know, it's mutual. Doctors aren't supposed to have favorites, but I have to admit...(Pause) You're my favorite patient.
(momentary silence)
SCP-049: I wish we could meet more often...
Dr. Lewandowski: I'd like that too, Doctor...
[END OF RECORDING]
Supplement
https://www.tumblr.com/snezka-049/766312832505626624/snezka-and-doctor-reference-and-lore-scp-049?source=share
#artists on tumblr#art#scp#aesthetic#scp foundation#scp 049#scp fandom#scp plague doctor#scp containment breach#scp fanart#scp shitposting#scp sl#scp secret laboratory#scp sedition#scp stuff#scp doctors#scp 049 × snezka#scp fragmented minds#scp oc#selfship ocxcanon#selfship community#oc x canon shipping#scp lore#scp character#scp community#scp art#scp alagadda#alagadda#plaguecore#plague doctor
109 notes
·
View notes