#Azure SQL Data warehouse
Explore tagged Tumblr posts
Text
Connection to Azure SQL Database from C#
Prerequisites Before we begin, ensure that you have the following: An Azure Subscription: If you don’t have one, you can create a free account. An Azure SQL Database: Set up a database in Azure and note down your server name (like yourserver.database.windows.net), database name, and the credentials (username and password) used for accessing the database. SQL Server Management Studio (SSMS):…
View On WordPress
0 notes
Text
Power of Data Visualization: A Deep Dive into Microsoft Power BI Services
In today’s data-driven world, the ability to transform raw data into actionable insights is a crucial asset for businesses. As organizations accumulate vast amounts of data from various sources, the challenge lies not just in storing and managing this data but in making sense of it. This is where Microsoft Power BI Services comes into play—a powerful tool designed to bring data to life through intuitive and dynamic visualizations.
What is Microsoft Power BI?
Microsoft Power BI is a suite of business analytics tools that enables organizations to analyze data and share insights. It provides interactive visualizations and business intelligence capabilities with a simple interface, making it accessible to both technical and non-technical users. Whether you are analyzing sales performance, tracking customer behavior, or monitoring operational efficiency, Power BI empowers you to create dashboards and reports that highlight the key metrics driving your business.
Key Features of Microsoft Power BI Services
User-Friendly Interface: One of the standout features of Power BI is its user-friendly interface. Even those with minimal technical expertise can quickly learn to create reports and dashboards. The drag-and-drop functionality allows users to effortlessly build visualizations, while pre-built templates and AI-powered insights help accelerate the decision-making process.
Data Connectivity: Power BI supports a wide range of data sources, including Excel, SQL Server, cloud-based data warehouses, and even social media platforms. This extensive connectivity ensures that users can pull in data from various systems and consolidate it into a single, coherent view. The ability to connect to both on-premises and cloud-based data sources provides flexibility and scalability as your data needs evolve.
Real-Time Analytics: In today’s fast-paced business environment, real-time data is critical. Power BI’s real-time analytics capabilities allow users to monitor data as it’s collected, providing up-to-the-minute insights. Whether tracking website traffic, monitoring social media engagement, or analyzing sales figures, Power BI ensures that you are always equipped with the latest information.
Custom Visualizations: While Power BI comes with a robust library of standard visualizations, it also supports custom visuals. Organizations can create unique visualizations that cater to specific business needs, ensuring that the data is presented in the most effective way possible. These custom visuals can be developed in-house or sourced from the Power BI community, offering endless possibilities for data representation.
Collaboration and Sharing: Collaboration is key to making data-driven decisions. Power BI makes it easy to share insights with colleagues, whether through interactive reports or shared dashboards. Reports can be published to the Power BI service, embedded in websites, or shared via email, ensuring that stakeholders have access to the information they need, when they need it.
Integration with Microsoft Ecosystem: As part of the Microsoft ecosystem, Power BI seamlessly integrates with other Microsoft products like Excel, Azure, and SharePoint. This integration enhances productivity by allowing users to leverage familiar tools and workflows. For example, users can import Excel data directly into Power BI, or embed Power BI reports in SharePoint for easy access.
The Benefits of Microsoft Power BI Services for Businesses
The adoption of Microsoft Power BI Services offers numerous benefits for businesses looking to harness the power of their data:
Enhanced Decision-Making: By providing real-time, data-driven insights, Power BI enables businesses to make informed decisions faster. The ability to visualize data through dashboards and reports ensures that critical information is easily accessible, allowing decision-makers to respond to trends and challenges with agility.
Cost-Effective Solution: Power BI offers a cost-effective solution for businesses of all sizes. With a range of pricing options, including a free version, Power BI is accessible to small businesses and large enterprises alike. The cloud-based service model also reduces the need for expensive hardware and IT infrastructure, making it a scalable option as your business grows.
Improved Data Governance: Data governance is a growing concern for many organizations. Power BI helps address this by providing centralized control over data access and usage. Administrators can set permissions and define data access policies, ensuring that sensitive information is protected and that users only have access to the data they need.
Scalability and Flexibility: As businesses grow and their data needs evolve, Power BI scales effortlessly to accommodate new data sources, users, and reporting requirements. Whether expanding to new markets, launching new products, or adapting to regulatory changes, Power BI provides the flexibility to adapt and thrive in a dynamic business environment.
Streamlined Reporting: Traditional reporting processes can be time-consuming and prone to errors. Power BI automates many of these processes, reducing the time spent on report creation and ensuring accuracy. With Power BI, reports are not only generated faster but are also more insightful, helping businesses to stay ahead of the competition.
Empowering Non-Technical Users: One of Power BI’s greatest strengths is its accessibility. Non-technical users can easily create and share reports without relying on IT departments. This democratization of data empowers teams across the organization to take ownership of their data and contribute to data-driven decision-making.
Use Cases of Microsoft Power BI Services
Power BI’s versatility makes it suitable for a wide range of industries and use cases:
Retail: Retailers use Power BI to analyze sales data, track inventory levels, and understand customer behavior. Real-time dashboards help retail managers make quick decisions on pricing, promotions, and stock replenishment.
Finance: Financial institutions rely on Power BI to monitor key performance indicators (KPIs), analyze risk, and ensure compliance with regulatory requirements. Power BI’s robust data security features make it an ideal choice for handling sensitive financial data.
Healthcare: In healthcare, Power BI is used to track patient outcomes, monitor resource utilization, and analyze population health trends. The ability to visualize complex data sets helps healthcare providers deliver better care and improve operational efficiency.
Manufacturing: Manufacturers leverage Power BI to monitor production processes, optimize supply chains, and manage quality control. Real-time analytics enable manufacturers to identify bottlenecks and make data-driven adjustments on the fly.
Conclusion
In an era where data is a key driver of business success, Microsoft Power BI Services offers a powerful, flexible, and cost-effective solution for transforming raw data into actionable insights. Its user-friendly interface, extensive data connectivity, and real-time analytics capabilities make it an invaluable tool for organizations across industries. By adopting Power BI, businesses can unlock the full potential of their data, making informed decisions that drive growth, efficiency, and innovation.
5 notes
·
View notes
Text
The demand for SAP FICO vs. SAP HANA in India depends on industry trends, company requirements, and evolving SAP technologies. Here’s a breakdown:
1. SAP FICO Demand in India
SAP FICO (Finance & Controlling) has been a core SAP module for years, used in almost every company that runs SAP ERP. It includes:
Financial Accounting (FI) – General Ledger, Accounts Payable, Accounts Receivable, Asset Accounting, etc.
Controlling (CO) – Cost Center Accounting, Internal Orders, Profitability Analysis, etc.
Why is SAP FICO in demand? ✅ Essential for businesses – Every company needs finance & accounting. ✅ High job availability – Many Indian companies still run SAP ECC, where FICO is critical. ✅ Migration to S/4HANA – Companies moving from SAP ECC to SAP S/4HANA still require finance professionals. ✅ Stable career growth – Finance roles are evergreen.
Challenges:
As companies move to S/4HANA, traditional FICO skills alone are not enough.
Need to upskill in SAP S/4HANA Finance (Simple Finance) and integration with SAP HANA.
2. SAP HANA Demand in India
SAP HANA is an in-memory database and computing platform that powers SAP S/4HANA. Key areas include:
SAP HANA Database (DBA roles)
SAP HANA Modeling (for reporting & analytics)
SAP S/4HANA Functional & Technical roles
SAP BW/4HANA (Business Warehouse on HANA)
Why is SAP HANA in demand? ✅ Future of SAP – SAP S/4HANA is replacing SAP ECC, and all new implementations are on HANA. ✅ High-paying jobs – Technical consultants with SAP HANA expertise earn more. ✅ Cloud adoption – Companies prefer SAP on AWS, Azure, and GCP, requiring HANA skills. ✅ Data & Analytics – Business intelligence and real-time analytics run on HANA.
Challenges:
More technical compared to SAP FICO.
Requires skills in SQL, HANA Modeling, CDS Views, and ABAP on HANA.
Companies still transitioning from ECC, meaning FICO is not obsolete yet.
Mail us on [email protected]
Website: Anubhav Online Trainings | UI5, Fiori, S/4HANA Trainings
0 notes
Text
Best Snowflake Online Course Hyderabad | Snowflake Course
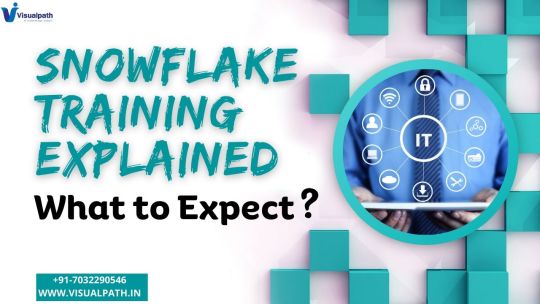
Snowflake Training Explained: What to Expect
Snowflake Online Course Hyderabad has gained immense popularity as a cloud-based data platform. Businesses and professionals are eager to master it for better data management, analytics, and performance. If you're considering Snowflake training, you might be wondering what to expect. This article provides a comprehensive overview of the learning process, key topics covered, and benefits of training.
Snowflake is a cloud-native data warehouse that supports structured and semi-structured data. It runs on major cloud providers like AWS, Azure, and Google Cloud. Learning Snowflake can enhance your skills in data engineering, analytics, and business intelligence. Snowflake Course
Who Should Enrol in Snowflake Training?
Snowflake training is ideal for various professionals. Whether you are a beginner or an experienced data expert, the training can be tailored to your needs. Here are some of the key roles that benefit from Snowflake training:
Data Engineers – Learn how to build and manage data pipelines.
Data Analysts – Gain insights using SQL and analytics tools.
Database Administrators – Optimize database performance and security.
Cloud Architects – Understand Snowflake's architecture and integration.
Business Intelligence Professionals – Enhance reporting and decision-making.
Even if you have basic SQL knowledge, you can start learning Snowflake. Many courses cater to beginners, covering fundamental concepts before diving into advanced topics.
Key Topics Covered in Snowflake Training
A well-structured Snowflake Online Course program covers a range of topics. Here are the core areas you can expect:
1. Introduction to Snowflake Architecture
Understanding Snowflake’s multi-cluster shared data architecture is crucial. Training explains how Snowflake separates compute, storage, and services, offering scalability and performance benefits.
2. Working with Databases, Schemas, and Tables
You’ll learn how to create and manage databases, schemas, and tables. The training covers structured and semi-structured data handling, including JSON, Parquet, and Avro file formats.
3. Writing and Optimizing SQL Queries
SQL is the backbone of Snowflake Course covers SQL queries, joins, aggregations, and analytical functions. You’ll also learn query optimization techniques to improve efficiency.
4. Snowflake Data Sharing and Security
Snowflake allows secure data sharing across different organizations. The training explains role-based access control (RBAC), encryption, and compliance best practices.
5. Performance Tuning and Cost Optimization
Understanding how to optimize performance and manage costs is essential. You’ll learn about virtual warehouses, caching, and auto-scaling to enhance efficiency.
6. Integration with BI and ETL Tools
Snowflake integrates with tools like Tableau, Power BI, and ETL platforms such as Apache Airflow and Matillion. Training explores these integrations to streamline workflows.
7. Data Loading and Unloading Techniques
You'll learn how to load data into Snowflake using COPY commands, Snow pipe, and bulk load methods. The training also covers exporting data in different formats.
Learning Modes: Online vs. Instructor-Led Training
When choosing a Snowflake training program, you have two main options: online self-paced courses or instructor-led training. Each has its pros and cons.
Online Self-Paced Training
Flexible learning schedule.
Affordable compared to instructor-led courses.
Access to recorded sessions and study materials.
Suitable for individuals who prefer self-learning.
Instructor-Led Training
Live interaction with experienced trainers.
Opportunity to ask questions and clarify doubts.
Hands-on projects and real-time exercises.
Structured curriculum with guided learning.
Your choice depends on your learning style, budget, and time availability. Some platforms offer a combination of both for a balanced experience.
Benefits of Snowflake Training
Investing in Snowflake training offers numerous advantages. Here’s why it’s worth considering:
1. High Demand for Snowflake Professionals
Organizations worldwide are adopting Snowflake, creating a demand for skilled professionals. Earning Snowflake expertise can open new career opportunities.
2. Better Data Management Skills
Snowflake training equips you with best practices for handling large-scale data. You’ll learn how to manage structured and semi-structured data efficiently.
3. Improved Job Prospects and Salary Growth
Certified Snowflake professionals often receive higher salaries. Many organizations prefer candidates with Snowflake expertise over traditional database management skills.
4. Real-World Project Experience
Most training programs include hands-on projects that simulate real-world scenarios. This experience helps in applying theoretical knowledge to practical use cases.
5. Enhanced Business Intelligence Capabilities
With Snowflake training, you can improve data analytics and reporting skills. This enables businesses to make data-driven decisions more effectively.
How to Choose the Right Snowflake Training Program
With various training providers available, choosing the right one is crucial. Here are some factors to consider:
Accreditation and Reviews – Look for well-reviewed courses from reputable providers.
Hands-on Labs and Projects – Practical exercises help reinforce learning.
Certification Preparation – If you're aiming for Snowflake certification, choose a course that aligns with exam objectives.
Support and Community – A strong community and mentor support can enhance learning.
Conclusion
Snowflake training is an excellent investment for data professionals. Whether you're a beginner or an experienced database expert, learning Snowflake can boost your career prospects. The training covers essential concepts such as architecture, SQL queries, performance tuning, security, and integrations.
Choosing the right training format—self-paced or instructor-led—depends on your learning preferences. With growing demand for Snowflake professionals, gaining expertise in this platform can lead to better job opportunities and higher salaries. If you’re considering a career in data engineering, analytics, or cloud computing, Snowflake training is a smart choice.
Start your Snowflake learning journey today with Visualpath and take your data skills to the next level!
Visualpath is the Leading and Best Institute for learning in Hyderabad. We provide Snowflake Online Training. You will get the best course at an affordable cost.
For more Details Contact +91 7032290546
Visit: https://www.visualpath.in/snowflake-training.html
#Snowflake Training#Snowflake Online Training#Snowflake Training in Hyderabad#Snowflake Training in Ameerpet#Snowflake Course#Snowflake Training Institute in Hyderabad#Snowflake Online Course Hyderabad#Snowflake Online Training Course#Snowflake Training in Chennai#Snowflake Training in Bangalore#Snowflake Training in India#Snowflake Course in Ameerpet
0 notes
Text
Azure Data Engineer Course In Bangalore | Azure Data
PolyBase in Azure SQL Data Warehouse: A Comprehensive Guide
Introduction to PolyBase
PolyBase is a technology in Microsoft SQL Server and Azure Synapse Analytics (formerly Azure SQL Data Warehouse) that enables querying data stored in external sources using T-SQL. It eliminates the need for complex ETL processes by allowing seamless data integration between relational databases and big data sources such as Hadoop, Azure Blob Storage, and external databases.
PolyBase is particularly useful in Azure SQL Data Warehouse as it enables high-performance data virtualization, allowing users to query and import large datasets efficiently without moving data manually. This makes it an essential tool for organizations dealing with vast amounts of structured and unstructured data. Microsoft Azure Data Engineer
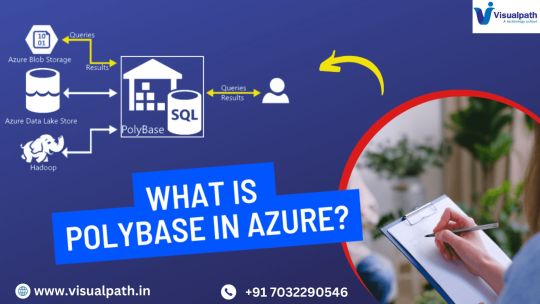
How PolyBase Works
PolyBase operates by creating external tables that act as a bridge between Azure SQL Data Warehouse and external storage. When a query is executed on an external table, PolyBase translates it into the necessary format and fetches the required data in real-time, significantly reducing data movement and enhancing query performance.
The key components of PolyBase include:
External Data Sources – Define the external system, such as Azure Blob Storage or another database.
File Format Objects – Specify the format of external data, such as CSV, Parquet, or ORC.
External Tables – Act as an interface between Azure SQL Data Warehouse and external data sources.
Data Movement Service (DMS) – Responsible for efficient data transfer during query execution. Azure Data Engineer Course
Benefits of PolyBase in Azure SQL Data Warehouse
Seamless Integration with Big Data – PolyBase enables querying data stored in Hadoop, Azure Data Lake, and Blob Storage without additional transformation.
High-Performance Data Loading – It supports parallel data ingestion, making it faster than traditional ETL pipelines.
Cost Efficiency – By reducing data movement, PolyBase minimizes the need for additional storage and processing costs.
Simplified Data Architecture – Users can analyze external data alongside structured warehouse data using a single SQL query.
Enhanced Analytics – Supports machine learning and AI-driven analytics by integrating with external data sources for a holistic view.
Using PolyBase in Azure SQL Data Warehouse
To use PolyBase effectively, follow these key steps:
Enable PolyBase – Ensure that PolyBase is activated in Azure SQL Data Warehouse, which is typically enabled by default in Azure Synapse Analytics.
Define an External Data Source – Specify the connection details for the external system, such as Azure Blob Storage or another database.
Specify the File Format – Define the format of the external data, such as CSV or Parquet, to ensure compatibility.
Create an External Table – Establish a connection between Azure SQL Data Warehouse and the external data source by defining an external table.
Query the External Table – Data can be queried seamlessly without requiring complex ETL processes once the external table is set up. Azure Data Engineer Training
Common Use Cases of PolyBase
Data Lake Integration: Enables organizations to query raw data stored in Azure Data Lake without additional data transformation.
Hybrid Data Solutions: Facilitates seamless data integration between on-premises and cloud-based storage systems.
ETL Offloading: Reduces reliance on traditional ETL tools by allowing direct data loading into Azure SQL Data Warehouse.
IoT Data Processing: Helps analyze large volumes of sensor-generated data stored in cloud storage.
Limitations of PolyBase
Despite its advantages, PolyBase has some limitations:
It does not support direct updates or deletions on external tables.
Certain data formats, such as JSON, require additional handling.
Performance may depend on network speed and the capabilities of the external data source. Azure Data Engineering Certification
Conclusion
PolyBase is a powerful Azure SQL Data Warehouse feature that simplifies data integration, reduces data movement, and enhances query performance. By enabling direct querying of external data sources, PolyBase helps organizations optimize their big data analytics workflows without costly and complex ETL processes. For businesses leveraging Azure Synapse Analytics, mastering PolyBase can lead to better data-driven decision-making and operational efficiency.
Implementing PolyBase effectively requires understanding its components, best practices, and limitations, making it a valuable tool for modern cloud-based data engineering and analytics solutions.
For More Information about Azure Data Engineer Online Training
Contact Call/WhatsApp: +91 7032290546
Visit: https://www.visualpath.in/online-azure-data-engineer-course.html
#Azure Data Engineer Course#Azure Data Engineering Certification#Azure Data Engineer Training In Hyderabad#Azure Data Engineer Training#Azure Data Engineer Training Online#Azure Data Engineer Course Online#Azure Data Engineer Online Training#Microsoft Azure Data Engineer#Azure Data Engineer Course In Bangalore#Azure Data Engineer Course In Chennai#Azure Data Engineer Training In Bangalore#Azure Data Engineer Course In Ameerpet
0 notes
Text
Building Data Pipelines with Snowflake and Apache Airflow
1. Introduction to Snowflake
Snowflake is a cloud-native data platform designed for scalability and ease of use, providing data warehousing, data lakes, and data sharing capabilities. Unlike traditional databases, Snowflake’s architecture separates compute, storage, and services, making it highly scalable and cost-effective. Some key features to highlight:
Zero-Copy Cloning: Allows you to clone data without duplicating it, making testing and experimentation more cost-effective.
Multi-Cloud Support: Snowflake works across major cloud providers like AWS, Azure, and Google Cloud, offering flexibility in deployment.
Semi-Structured Data Handling: Snowflake can handle JSON, Parquet, XML, and other formats natively, making it versatile for various data types.
Automatic Scaling: Automatically scales compute resources based on workload demands without manual intervention, optimizing cost.
2. Introduction to Apache Airflow
Apache Airflow is an open-source platform used for orchestrating complex workflows and data pipelines. It’s widely used for batch processing and ETL (Extract, Transform, Load) tasks. You can define workflows as Directed Acyclic Graphs (DAGs), making it easy to manage dependencies and scheduling. Some of its features include:
Dynamic Pipeline Generation: You can write Python code to dynamically generate and execute tasks, making workflows highly customizable.
Scheduler and Executor: Airflow includes a scheduler to trigger tasks at specified intervals, and different types of executors (e.g., Celery, Kubernetes) help manage task execution in distributed environments.
Airflow UI: The intuitive web-based interface lets you monitor pipeline execution, visualize DAGs, and track task progress.
3. Snowflake and Airflow Integration
The integration of Snowflake with Apache Airflow is typically achieved using the SnowflakeOperator, a task operator that enables interaction between Airflow and Snowflake. Airflow can trigger SQL queries, execute stored procedures, and manage Snowflake tasks as part of your DAGs.
SnowflakeOperator: This operator allows you to run SQL queries in Snowflake, which is useful for performing actions like data loading, transformation, or even calling Snowflake procedures.
Connecting Airflow to Snowflake: To set this up, you need to configure a Snowflake connection within Airflow. Typically, this includes adding credentials (username, password, account, warehouse, and database) in Airflow’s connection settings.
Example code for setting up the Snowflake connection and executing a query:pythonfrom airflow.providers.snowflake.operators.snowflake import SnowflakeOperator from airflow import DAG from datetime import datetimedefault_args = { 'owner': 'airflow', 'start_date': datetime(2025, 2, 17), }with DAG('snowflake_pipeline', default_args=default_args, schedule_interval=None) as dag: run_query = SnowflakeOperator( task_id='run_snowflake_query', sql="SELECT * FROM my_table;", snowflake_conn_id='snowflake_default', # The connection ID in Airflow warehouse='MY_WAREHOUSE', database='MY_DATABASE', schema='MY_SCHEMA' )
4. Building a Simple Data Pipeline
Here, you could provide a practical example of an ETL pipeline. For instance, let’s create a pipeline that:
Extracts data from a source (e.g., a CSV file in an S3 bucket),
Loads the data into a Snowflake staging table,
Performs transformations (e.g., cleaning or aggregating data),
Loads the transformed data into a production table.
Example DAG structure:pythonfrom airflow.providers.snowflake.operators.snowflake import SnowflakeOperator from airflow.providers.amazon.aws.transfers.s3_to_snowflake import S3ToSnowflakeOperator from airflow import DAG from datetime import datetimewith DAG('etl_pipeline', start_date=datetime(2025, 2, 17), schedule_interval='@daily') as dag: # Extract data from S3 to Snowflake staging table extract_task = S3ToSnowflakeOperator( task_id='extract_from_s3', schema='MY_SCHEMA', table='staging_table', s3_keys=['s3://my-bucket/my-file.csv'], snowflake_conn_id='snowflake_default' ) # Load data into Snowflake and run transformation transform_task = SnowflakeOperator( task_id='transform_data', sql='''INSERT INTO production_table SELECT * FROM staging_table WHERE conditions;''', snowflake_conn_id='snowflake_default' ) extract_task >> transform_task # Define task dependencies
5. Error Handling and Monitoring
Airflow provides several mechanisms for error handling:
Retries: You can set the retries argument in tasks to automatically retry failed tasks a specified number of times.
Notifications: You can use the email_on_failure or custom callback functions to notify the team when something goes wrong.
Airflow UI: Monitoring is easy with the UI, where you can view logs, task statuses, and task retries.
Example of setting retries and notifications:pythonwith DAG('data_pipeline_with_error_handling', start_date=datetime(2025, 2, 17)) as dag: task = SnowflakeOperator( task_id='load_data_to_snowflake', sql="SELECT * FROM my_table;", snowflake_conn_id='snowflake_default', retries=3, email_on_failure=True, on_failure_callback=my_failure_callback # Custom failure function )
6. Scaling and Optimization
Snowflake’s Automatic Scaling: Snowflake can automatically scale compute resources based on the workload. This ensures that data pipelines can handle varying loads efficiently.
Parallel Execution in Airflow: You can split your tasks into multiple parallel branches to improve throughput. The task_concurrency argument in Airflow helps manage this.
Task Dependencies: By optimizing task dependencies and using Airflow’s ability to run tasks in parallel, you can reduce the overall runtime of your pipelines.
Resource Management: Snowflake supports automatic suspension and resumption of compute resources, which helps keep costs low when there is no processing required.1. Introduction to Snowflake
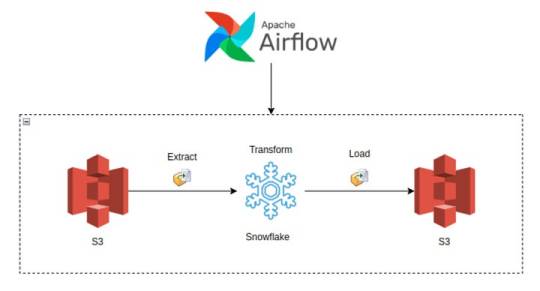
0 notes
Text
[Fabric] Fast Copy con Dataflows gen2
Cuando pensamos en integración de datos con Fabric está claro que se nos vienen dos herramientas a la mente al instante. Por un lado pipelines y por otro dataflows. Mientras existía Azure Data Factory y PowerBi Dataflows la diferencia era muy clara en audiencia y licencias para elegir una u otra. Ahora que tenemos ambas en Fabric la delimitación de una u otra pasaba por otra parte.
Por buen tiempo, el mercado separó las herramientas como dataflows la simple para transformaciones y pipelines la veloz para mover datos. Este artículo nos cuenta de una nueva característica en Dataflows que podría cambiar esta tendencia.
La distinción principal que separa estas herramientas estaba basado en la experiencia del usuario. Por un lado, expertos en ingeniería de datos preferían utilizar pipelines con actividades de transformaciones robustas d datos puesto que, para movimiento de datos y ejecución de código personalizado, es más veloz. Por otro lado, usuarios varios pueden sentir mucha mayor comodidad con Dataflows puesto que la experiencia de conectarse a datos y transformarlos es muy sencilla y cómoda. Así mismo, Power Query, lenguaje detrás de dataflows, ha probado tener la mayor variedad de conexiones a datos que el mercado ha visto.
Cierto es que cuando el proyecto de datos es complejo o hay cierto volumen de datos involucrado. La tendencia es usar data pipelines. La velocidad es crucial con los datos y los dataflows con sus transformaciones podían ser simples de usar, pero mucho más lentos. Esto hacía simple la decisión de evitarlos. ¿Y si esto cambiara? Si dataflows fuera veloz... ¿la elección sería la misma?
Veamos el contexto de definición de Microsoft:
Con la Fast Copy, puede ingerir terabytes de datos con la experiencia sencilla de flujos de datos (dataflows), pero con el back-end escalable de un copy activity que utiliza pipelines.
Como leemos de su documentación la nueva característica de dataflow podría fortalecer el movimiento de datos que antes frenaba la decisión de utilizarlos. Todo parece muy hermoso aun que siempre hay frenos o limitaciones. Veamos algunas consideraciones.
Origenes de datos permitidos
Fast Copy soporta los siguientes conectores
ADLS Gen2
Blob storage
Azure SQL DB
On-Premises SQL Server
Oracle
Fabric Lakehouse
Fabric Warehouse
PostgreSQL
Snowflake
Requisitos previos
Comencemos con lo que debemos tener para poder utilizar la característica
Debe tener una capacidad de Fabric.
En el caso de los datos de archivos, los archivos están en formato .csv o parquet de al menos 100 MB y se almacenan en una cuenta de Azure Data Lake Storage (ADLS) Gen2 o de Blob Storage.
En el caso de las bases de datos, incluida la de Azure SQL y PostgreSQL, 5 millones de filas de datos o más en el origen de datos.
En configuración de destino, actualmente, solo se admite lakehouse. Si desea usar otro destino de salida, podemos almacenar provisionalmente la consulta (staging) y hacer referencia a ella más adelante. Más info.
Prueba
Bajo estas consideraciones construimos la siguiente prueba. Para cumplir con las condiciones antes mencionadas, disponemos de un Azure Data Lake Storage Gen2 con una tabla con información de vuelos que pesa 1,8Gb y esta constituida por 10 archivos parquet. Creamos una capacidad de Fabric F2 y la asignaciones a un área de trabajo. Creamos un Lakehouse. Para corroborar el funcionamiento creamos dos Dataflows Gen2.
Un dataflow convencional sin FastCopy se vería así:
Podemos reconocer en dos modos la falta de fast copy. Primero porque en el menú de tabla no tenemos la posibilidad de requerir fast copy (debajo de Entable staging) y segundo porque vemos en rojo los "Applied steps" como cuando no tenemos query folding andando. Allí nos avisaría si estamos en presencia de fast copy o intenta hacer query folding:
Cuando hace query folding menciona "... evaluated by the datasource."
Activar fast copy
Para activarlo, podemos presenciar el apartado de opciones dentro de la pestaña "Home".
Allí podemos encontrarlo en la opción de escalar o scale:
Mientras esa opción esté encendida. El motor intentará utilizar fast copy siempre y cuando la tabla cumpla con las condiciones antes mencionadas. En caso que no las cumpla, por ejemplo la tabla pese menos de 100mb, el fast copy no será efectivo y funcionaría igual que un dataflow convencional.
Aquí tenemos un problema, puesto que la diferencia de tiempos entre una tabla que usa fast copy y una que no puede ser muy grande. Por esta razón, algunos preferiríamos que el dataflow falle si no puede utilizar fast copy en lugar que cambie automaticamente a no usarlo y demorar muchos minutos más. Para exigirle a la tabla que debe usarlo, veremos una opción en click derecho:
Si forzamos requerir fast copy, entonces la tabla devolverá un error en caso que no pueda utilizarlo porque rompa con las condiciones antes mencionadas a temprana etapa de la actualización.
En el apartado derecho de la imagen tambien podemos comprobar que ya no está rojo. Si arceramos el mouse nos aclarará que esta aceptado el fast copy. "Si bien tengo otro detalle que resolver ahi, nos concentremos en el mensaje aclarando que esta correcto. Normalmente reflejaría algo como "...step supports fast copy."
Resultados
Hemos seleccionado exactamente los mismos archivos y ejecutado las mismas exactas transformaciones con dataflows. Veamos resultados.
Ejecución de dataflow sin fast copy:
Ejecución de dataflow con fast copy:
Para validar que tablas de nuestra ejecución usan fast copy. Podemos ingresar a la corrida
En el primer menú podremos ver que en lugar de "Tablas" aparece "Actividades". Ahi el primer síntoma. El segundo es al seleccionar una actividad buscamos en motor y encontramos "CopyActivity". Así validamos que funcionó la característica sobre la tabla.
Como pueden apreciar en este ejemplo, la respuesta de fast copy fue 4 veces más rápida. El incremento de velocidad es notable y la forma de comprobar que se ejecute la característica nos revela que utiliza una actividad de pipeline como el servicio propiamente dicho.
Conclusión
Seguramente esta característica tiene mucho para dar e ir mejorando. No solamente con respecto a los orígenes sino tambien a sus modos. No podemos descargar que también lo probamos contra pipelines y aqui esta la respuesta:
En este ejemplo los Data Pipelines siguen siendo superiores en velocidad puesto que demoró 4 minutos en correr la primera vez y menos la segunda. Aún tiene mucho para darnos y podemos decir que ya está lista para ser productiva con los origenes de datos antes mencionados en las condiciones apropiadas. Antes de terminar existen unas limitaciones a tener en cuenta:
Limitaciones
Se necesita una versión 3000.214.2 o más reciente de un gateway de datos local para soportar Fast Copy.
El gateway VNet no está soportado.
No se admite escribir datos en una tabla existente en Lakehouse.
No se admite un fixed schema.
#fabric#microsoft fabric#fabric training#fabric tips#fabric tutorial#data engineering#dataflows#fabric dataflows#fabric data factory#ladataweb
0 notes
Text
The demand for SAP FICO vs. SAP HANA in India depends on industry trends, company requirements, and evolving SAP technologies. Here’s a breakdown:
1. SAP FICO Demand in India
SAP FICO (Finance & Controlling) has been a core SAP module for years, used in almost every company that runs SAP ERP. It includes:
Financial Accounting (FI) – General Ledger, Accounts Payable, Accounts Receivable, Asset Accounting, etc.
Controlling (CO) – Cost Center Accounting, Internal Orders, Profitability Analysis, etc.
Why is SAP FICO in demand? ✅ Essential for businesses – Every company needs finance & accounting. ✅ High job availability – Many Indian companies still run SAP ECC, where FICO is critical. ✅ Migration to S/4HANA – Companies moving from SAP ECC to SAP S/4HANA still require finance professionals. ✅ Stable career growth – Finance roles are evergreen.
Challenges:
As companies move to S/4HANA, traditional FICO skills alone are not enough.
Need to upskill in SAP S/4HANA Finance (Simple Finance) and integration with SAP HANA.
2. SAP HANA Demand in India
SAP HANA is an in-memory database and computing platform that powers SAP S/4HANA. Key areas include:
SAP HANA Database (DBA roles)
SAP HANA Modeling (for reporting & analytics)
SAP S/4HANA Functional & Technical roles
SAP BW/4HANA (Business Warehouse on HANA)
Why is SAP HANA in demand? ✅ Future of SAP – SAP S/4HANA is replacing SAP ECC, and all new implementations are on HANA. ✅ High-paying jobs – Technical consultants with SAP HANA expertise earn more. ✅ Cloud adoption – Companies prefer SAP on AWS, Azure, and GCP, requiring HANA skills. ✅ Data & Analytics – Business intelligence and real-time analytics run on HANA.
Challenges:
More technical compared to SAP FICO.
Requires skills in SQL, HANA Modeling, CDS Views, and ABAP on HANA.
Companies still transitioning from ECC, meaning FICO is not obsolete yet.
Mail us on [email protected]
Website: Anubhav Online Trainings | UI5, Fiori, S/4HANA Trainings
0 notes
Text
Snowflake Online Training | Snowflake Online Course Hyderabad
How to Become a Snowflake Expert: Courses & Tips
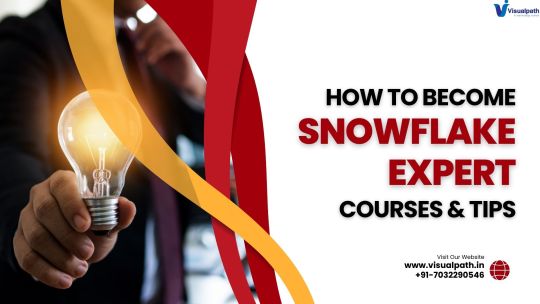
Snowflake Online Training is a leading cloud data platform used for data warehousing, analytics, and data sharing. Many businesses rely on it for handling large-scale data operations efficiently. If you want to become a Snowflake expert, you need the right knowledge, training, and practice. This article will guide you through the steps to master Snowflake, including recommended courses and essential tips.
Why Learn Snowflake?
Snowflake Online Course Hyderabad is a highly scalable and flexible cloud-based data platform. It supports structured and semi-structured data and is widely used in various industries. Here are some key reasons to learn Snowflake:
High demand for Snowflake professionals in the job market.
Simplified data management with cloud-based storage and computing.
Compatibility with multiple cloud providers like AWS, Azure, and Google Cloud.
Pay-as-you-go pricing model, making it cost-effective for businesses.
Strong community and extensive documentation for learning support.
Ability to handle large datasets efficiently with minimal administrative effort.
Support for advanced analytics and data sharing features.
Best Courses to Learn Snowflake
To become an expert, enrolling in structured training programs is essential. Here are some of the best Snowflake courses:
1. Snowflake Official Training
Snowflake offers official training programs, including:
Snowflake Fundamentals
SnowPro Core Certification Preparation
Snowflake Advanced Architecting
Snowflake Data Engineering & Administration
These courses provide in-depth knowledge about platform functionalities and best practices. The official training is highly recommended for those aiming for certification.
2. Visualpath Snowflake Courses
Visualpath offers multiple courses on Snowflake for different skill levels. Some popular ones include:
Snowflake Masterclass: The Complete Guide
Hands-on Snowflake Training for Beginners
Snowflake Online Training for Data Engineers and Analysts
These courses include practical examples, real-world use cases, and hands-on exercises, making them ideal for learners looking for step-by-step guidance.
3. Coursera & LinkedIn Learning
Coursera and LinkedIn Learning have structured courses on Snowflake and cloud data warehousing. These platforms are great for obtaining industry-recognized certifications and expanding professional skills with expert-led instruction.
4. YouTube Tutorials
Many YouTube channels provide free Snowflake tutorials. Some well-known educators offer step-by-step guidance on Snowflake concepts and hands-on exercises. These tutorials are great for beginners and experienced professionals who want to learn at their own pace.
5. Pluralsight and A Cloud Guru
Pluralsight and A Cloud Guru offer high-quality Snowflake courses focusing on real-world applications, performance optimization, and integration with other cloud technologies. These platforms are useful for IT professionals and data engineers.
Essential Tips to Master Snowflake
1. Get Hands-On Experience
Practice is the key to mastering Snowflake. Create a free Snowflake trial account and experiment with different features like:
Creating databases and schemas.
Writing SQL queries for data analysis.
Understanding virtual warehouses and performance tuning.
Managing and optimizing storage and compute resources.
2. Learn SQL and Data Warehousing Concepts
Since Snowflake is an SQL-based platform, having strong SQL skills is necessary. Learn:
Basic and advanced SQL queries.
Data modeling and schema design.
ETL (Extract, Transform, Load) processes.
Query optimization techniques for better performance.
3. Study Snowflake Documentation
Snowflake Online Course Hyderabad provides comprehensive documentation covering all its features. Regularly reading the official documentation will help you stay updated with new functionalities and best practices.
4. Join Snowflake Community and Forums
Engaging with the Snowflake community can be beneficial. Join platforms like:
Snowflake Community Forum
LinkedIn Snowflake Groups
Reddit & Stack Overflow discussions
Snowflake User Groups and Meetups
These platforms help you connect with experts, ask questions, and learn from others. Attending Snowflake webinars and industry events is another great way to enhance knowledge.
5. Get Snowflake Certified
Earning a Snowflake certification enhances your credibility. The most recognized certifications include:
SnowPro Core Certification – For foundational knowledge.
SnowPro Advanced Certifications – Specializations in architecture, data engineering, and administration.
Snowflake Data Warehouse Specialist – Focuses on data storage and management best practices.
Certification boosts career opportunities, validates expertise, and increases your chances of securing high-paying roles.
Career Opportunities with Snowflake Expertise
Once you become proficient in Snowflake, various career paths open up, such as:
Snowflake Developer – Responsible for designing and implementing Snowflake solutions.
Data Engineer – Works on data pipelines and ETL processes in Snowflake.
Cloud Data Architect – Designs large-scale Snowflake data solutions and ensures optimal performance.
BI & Analytics Specialist – Uses Snowflake for data analysis and reporting.
Data Scientist – Leverages Snowflake’s analytical capabilities to derive insights from large datasets.
Companies worldwide are adopting Snowflake, increasing the demand for skilled professionals. With Snowflake expertise, you can work in various industries, including finance, healthcare, e-commerce, and technology.
Advanced Snowflake Skills to Learn
Once you master the basics, focus on advanced Snowflake skills such as:
Performance Tuning – Understanding query optimization and storage management.
Data Sharing and Security – Learning how to securely share data across organizations.
Integration with BI Tools – Connecting Snowflake with Tableau, Power BI, and Looker.
Automating Workflows – Using Snowflake with tools like Apache Airflow for data pipeline automation.
Multi-Cloud Deployments – Understanding how to implement Snowflake across different cloud platforms.
Conclusion
Becoming a Snowflake expert requires continuous learning and practice. Start with structured courses, get hands-on experience, and engage with the community. Certification adds value to your profile and boosts your career opportunities. With the right approach, you can master Snowflake and become a sought-after professional in the cloud data industry.
The demand for Snowflake professionals is rising, making it an excellent career choice. Stay updated with the latest trends, keep refining your skills, and leverage networking opportunities to grow in this field. By dedicating time and effort, you can establish yourself as a Snowflake expert and take advantage of the numerous job opportunities available.
Visualpath is the Leading and Best Institute for learning in Hyderabad. We provide Snowflake Online Training. You will get the best course at an affordable cost.
For more Details Contact +91 7032290546
Visit: https://www.visualpath.in/snowflake-training.html
#Snowflake Training#Snowflake Online Training#Snowflake Training in Hyderabad#Snowflake Training in Ameerpet#Snowflake Course#Snowflake Training Institute in Hyderabad#Snowflake Online Course Hyderabad#Snowflake Online Training Course#Snowflake Training in Chennai#Snowflake Training in Bangalore#Snowflake Training in India#Snowflake Course in Ameerpet
0 notes
Text
Price: [price_with_discount] (as of [price_update_date] - Details) [ad_1] Leverage the power of Microsoft Azure Data Factory v2 to build hybrid data solutions Key Features Combine the power of Azure Data Factory v2 and SQL Server Integration Services Design and enhance performance and scalability of a modern ETL hybrid solution Interact with the loaded data in data warehouse and data lake using Power BI Book Description ETL is one of the essential techniques in data processing. Given data is everywhere, ETL will always be the vital process to handle data from different sources. Hands-On Data Warehousing with Azure Data Factory starts with the basic concepts of data warehousing and ETL process. You will learn how Azure Data Factory and SSIS can be used to understand the key components of an ETL solution. You will go through different services offered by Azure that can be used by ADF and SSIS, such as Azure Data Lake Analytics, Machine Learning and Databrick’s Spark with the help of practical examples. You will explore how to design and implement ETL hybrid solutions using different integration services with a step-by-step approach. Once you get to grips with all this, you will use Power BI to interact with data coming from different sources in order to reveal valuable insights. By the end of this book, you will not only learn how to build your own ETL solutions but also address the key challenges that are faced while building them. What you will learn Understand the key components of an ETL solution using Azure Data Factory and Integration Services Design the architecture of a modern ETL hybrid solution Implement ETL solutions for both on-premises and Azure data Improve the performance and scalability of your ETL solution Gain thorough knowledge of new capabilities and features added to Azure Data Factory and Integration Services Who this book is for This book is for you if you are a software professional who develops and implements ETL solutions using Microsoft SQL Server or Azure cloud. It will be an added advantage if you are a software engineer, DW/ETL architect, or ETL developer, and know how to create a new ETL implementation or enhance an existing one with ADF or SSIS. Table of Contents Azure Data Factory Getting Started with Our First Data Factory ADF and SSIS in PaaS Azure Data Lake Machine Learning on the Cloud Sparks with Databrick Power BI reports ASIN : B07DGJSPYK Publisher : Packt Publishing; 1st edition (31 May 2018) Language : English File size : 32536 KB Text-to-Speech : Enabled Screen Reader : Supported Enhanced typesetting : Enabled X-Ray : Not Enabled Word Wise : Not Enabled Print length : 371 pages [ad_2]
0 notes
Text
Use cases like integrating sales data from multiple sources.
Retailers often collect sales data from various sources, including point-of-sale (POS) systems, e-commerce platforms, third-party marketplaces, and ERP systems.
Azure Data Factory (ADF) enables seamless integration of this data for unified reporting, analytics, and business insights. Below are key use cases where ADF plays a crucial role:
1. Omnichannel Sales Data Integration
Scenario: A retailer operates physical stores, an online website, and sells on third-party marketplaces (Amazon, eBay, Shopify). Data from these sources need to be unified for accurate sales reporting.
✅ ADF Solution:
Extracts sales data from POS systems, e-commerce APIs, and ERP databases.
Loads data into a centralized data warehouse (Azure Synapse Analytics).
Enables real-time updates for tracking product performance across all channels.
🔹 Business Impact: Unified sales tracking across online and offline channels for better decision-making.
2. Real-Time Sales Analytics for Demand Forecasting
Scenario: A supermarket chain wants to predict demand by analyzing real-time sales trends across different locations.
✅ ADF Solution:
Uses Event-Based Triggers to process real-time sales transactions.
Connects to Azure Stream Analytics to generate demand forecasts.
Feeds insights into Power BI for managers to adjust inventory accordingly.
🔹 Business Impact: Reduced stockouts and overstocking, improving revenue and operational efficiency.
3. Sales Performance Analysis Across Regions
Scenario: A multinational retailer needs to compare sales performance across different countries and regions.
✅ ADF Solution:
Extracts regional sales data from distributed SQL databases.
Standardizes currency, tax, and pricing variations using Mapping Data Flows.
Aggregates data in Azure Data Lake for advanced reporting.
🔹 Business Impact: Enables regional managers to compare performance and optimize sales strategies.
4. Personalized Customer Insights for Marketing
Scenario: A fashion retailer wants to personalize promotions based on customer purchase behavior.
✅ ADF Solution:
Merges purchase history from CRM, website, and loyalty programs.
Applies AI/ML models in Azure Machine Learning to segment customers.
Sends targeted promotions via Azure Logic Apps and Email Services.
🔹 Business Impact: Higher customer engagement and improved sales conversion rates.
5. Fraud Detection in Sales Transactions
Scenario: A financial services retailer wants to detect fraudulent transactions based on unusual sales patterns.
✅ ADF Solution:
Ingests transaction data from multiple sources (credit card, mobile wallets, POS).
Applies anomaly detection models using Azure Synapse + ML algorithms.
Alerts security teams in real-time via Azure Functions.
🔹 Business Impact: Prevents fraudulent activities and financial losses.
6. Supplier Sales Reconciliation & Returns Management
Scenario: A retailer needs to reconcile sales data with supplier shipments and manage product returns efficiently.
✅ ADF Solution:
Integrates sales, purchase orders, and supplier shipment data.
Uses Data Flows to match sales records with supplier invoices.
Automates refund and restocking workflows using Azure Logic Apps.
🔹 Business Impact: Improves supplier relationships and streamlines return processes.
Conclusion
Azure Data Factory enables retailers to integrate, clean, and process sales data from multiple sources, driving insights and automation. Whether it’s demand forecasting, fraud detection, or customer personalization, ADF helps retailers make data-driven decisions and enhance efficiency.
WEBSITE: https://www.ficusoft.in/azure-data-factory-training-in-chennai/
0 notes
Text
Best Azure Data Engineer | Azure Data Engineer Course Online
Azure Data Factory vs SSIS: Understanding the Key Differences
Azure Data Factory (ADF) is a modern, cloud-based data integration service that enables organizations to efficiently manage, transform, and move data across various systems. In contrast, SQL Server Integration Services (SSIS) is a traditional on-premises ETL tool designed for batch processing and data migration. Both are powerful data integration tools offered by Microsoft, but they serve different purposes, environments, and capabilities. In this article, we’ll delve into the key differences between Azure Data Factory and SSIS, helping you understand when and why to choose one over the other. Microsoft Azure Data Engineer
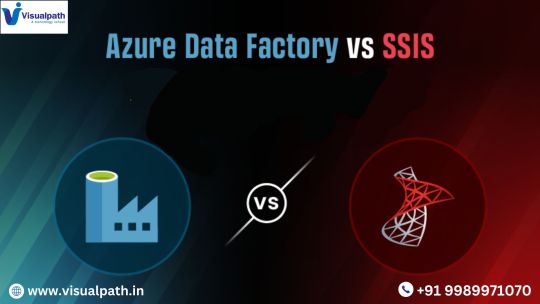
1. Overview
SQL Server Integration Services (SSIS)
SSIS is a traditional on-premises ETL (Extract, Transform, Load) tool that is part of Microsoft SQL Server. It allows users to create workflows for data integration, transformation, and migration between various systems. SSIS is ideal for batch processing and is widely used for enterprise-scale data warehouse operations.
Azure Data Factory (ADF)
ADF is a cloud-based data integration service that enables orchestration and automation of data workflows. It supports modern cloud-first architectures and integrates seamlessly with other Azure services. ADF is designed for handling big data, real-time data processing, and hybrid environments.
2. Deployment Environment
SSIS: Runs on-premises or in virtual machines. While you can host SSIS in the Azure cloud using Azure-SSIS Integration Runtime, it remains fundamentally tied to its on-premises roots.
ADF: Fully cloud-native and designed for Azure. It leverages the scalability, reliability, and flexibility of cloud infrastructure, making it ideal for modern, cloud-first architectures. Azure Data Engineering Certification
3. Data Integration Capabilities
SSIS: Focuses on traditional ETL processes with strong support for structured data sources like SQL Server, Oracle, and flat files. It offers various built-in transformations and control flow activities. However, its integration with modern cloud and big data platforms is limited.
ADF: Provides a broader range of connectors, supporting over 90 on-premises and cloud-based data sources, including Azure Blob Storage, Data Lake, Amazon S3, and Google Big Query. ADF also supports ELT (Extract, Load, Transform), enabling transformations within data warehouses like Azure Synapse Analytics.
4. Scalability and Performance
SSIS: While scalable in an on-premises environment, SSIS’s scalability is limited by your on-site hardware and infrastructure. Scaling up often involves significant costs and complexity.
ADF: Being cloud-native, ADF offers elastic scalability. It can handle vast amounts of data and scale resources dynamically based on workload, providing cost-effective processing for both small and large datasets.
5. Monitoring and Management
SSIS: Includes monitoring tools like SSISDB and SQL Server Agent, which allow you to schedule and monitor package execution. However, managing SSIS in distributed environments can be complex.
ADF: Provides a centralized, user-friendly interface within the Azure portal for monitoring and managing data pipelines. It also offers advanced logging and integration with Azure Monitor, making it easier to track performance and troubleshoot issues. Azure Data Engineer Course
6. Cost and Licensing
SSIS: Requires SQL Server licensing, which can be cost-prohibitive for organizations with limited budgets. Running SSIS in Azure adds additional infrastructure costs for virtual machines and storage.
ADF: Operates on a pay-as-you-go model, allowing you to pay only for the resources you consume. This makes ADF a more cost-effective option for organizations looking to minimize upfront investment.
7. Flexibility and Modern Features
SSIS: Best suited for organizations with existing SQL Server infrastructure and a need for traditional ETL workflows. However, it lacks features like real-time streaming and big data processing.
ADF: Supports real-time and batch processing, big data workloads, and integration with machine learning models and IoT data streams. ADF is built to handle modern, hybrid, and cloud-native data scenarios.
8. Use Cases
SSIS: Azure Data Engineer Training
On-premises data integration and transformation.
Migrating and consolidating data between SQL Server and other relational databases.
Batch processing and traditional ETL workflows.
ADF:
Building modern data pipelines in cloud or hybrid environments.
Handling large-scale big data workloads.
Real-time data integration and IoT data processing.
Cloud-to-cloud or cloud-to-on-premises data workflows.
Conclusion
While both Azure Data Factory and SSIS are powerful tools for data integration, they cater to different needs. SSIS is ideal for traditional, on-premises data environments with SQL Server infrastructure, whereas Azure Data Factory is the go-to solution for modern, scalable, and cloud-based data pipelines. The choice ultimately depends on your organization’s infrastructure, workload requirements, and long-term data strategy.
By leveraging the right tool for the right use case, businesses can ensure efficient data management, enabling them to make informed decisions and gain a competitive edge.
Visualpath is the Best Software Online Training Institute in Hyderabad. Avail complete Azure Data Engineering worldwide. You will get the best course at an affordable cost.
Attend Free Demo
Call on - +91-9989971070.
Visit: https://www.visualpath.in/online-azure-data-engineer-course.html
WhatsApp: https://www.whatsapp.com/catalog/919989971070/
Visit Blog: https://azuredataengineering2.blogspot.com/
#Azure Data Engineer Course#Azure Data Engineering Certification#Azure Data Engineer Training In Hyderabad#Azure Data Engineer Training#Azure Data Engineer Training Online#Azure Data Engineer Course Online#Azure Data Engineer Online Training#Microsoft Azure Data Engineer
0 notes
Text
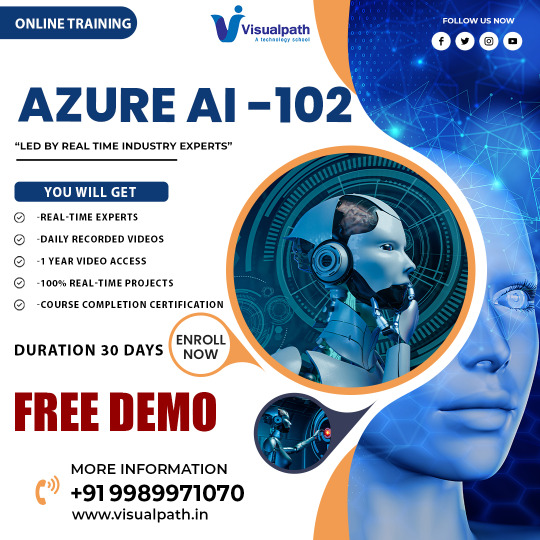
#VisualPath offers Azure AI Engineer Online Training in Hyderabad, designed to help you achieve your #AI-102 Microsoft Azure AI Training certification. Master AI technologies with hands-on expertise in Matillion, Snowflake, ETL, Informatica, SQL, and more. Gain deep knowledge in Data Warehouse, Power BI, Databricks, Oracle, SAP, and Amazon Redshift. Enjoy flexible schedules, recorded sessions, and global access for self-paced learning. Learn from industry experts and advance your career in AI and data. Call +91-9989971070 for a free demo today!
WhatsApp: https://www.whatsapp.com/catalog/919989971070/
Visit Blog: https://visualpathblogs.com/category/aws-data-engineering-with-data-analytics/
Visit: https://www.visualpath.in/online-ai-102-certification.html
#visualpathedu#testing#automation#selenium#git#github#JavaScript#Azure#CICD#AzureDevOps#playwright#handonlearning#education#SoftwareDevelopment#onlinelearning#newtechnology#software#ITskills#training#trendingcourses#careers#students#typescript
0 notes
Text
Azure Data Engineering Certification Course
Azure Data Engineering Training: What Is Azure Data Engineering?
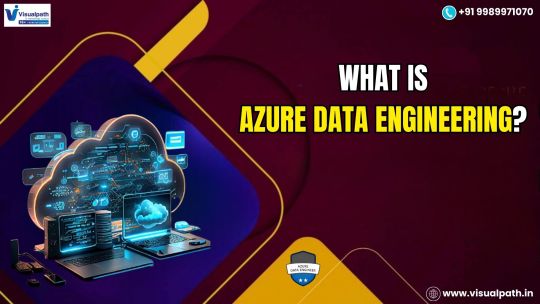
Introduction:
Azure Data Engineering Training has emerged as a critical skill set for professionals working with cloud-based data solutions. As organizations increasingly rely on cloud technologies for data management, an Azure Data Engineer becomes a key player in managing, transforming, and integrating data to drive decision-making and business intelligence. Azure Data Engineering refers to the process of designing and managing data systems on Microsoft’s Azure cloud platform, using a wide range of tools and services provided by Microsoft. This includes building, managing, and optimizing data pipelines, data storage solutions, and real-time analytics. For professionals aspiring to excel in this field, an Azure Data Engineer Course offers comprehensive knowledge and skills, paving the way for an Azure Data Engineering Certification.
What Does an Azure Data Engineer Do?
An Azure Data Engineer works with various data management and analytics tools to design, implement, and maintain data solutions. They are responsible for ensuring that data is accurate, accessible, and scalable. Their work typically includes:
Building Data Pipelines: Azure Data Engineers design and implement data pipelines using Azure tools like Azure Data Factory, which automate the movement and transformation of data from various sources into data storage or data warehouses.
Data Storage Management: Azure provides scalable storage solutions such as Azure Data Lake, Azure Blob Storage, and Azure SQL Database. An Azure Data Engineer ensures the proper storage architecture is in place, optimizing for performance, security, and compliance.
Data Transformation: Azure Data Engineers use tools like Azure Data bricks, Azure Synapse Analytics, and SQL to transform raw data into meaningful, actionable insights. This process includes cleaning, enriching, and aggregating data to create datasets that can be analysed for reporting or predictive analytics.
Integration with Data Solutions: They integrate various data sources, including on-premises databases, cloud-based data stores, and real-time streaming data, into a unified platform for data processing and analytics.
Automation and Monitoring: Data engineers automate repetitive tasks, such as data loading and processing, and implement monitoring solutions to ensure the pipelines are running smoothly.
Data Security and Compliance: Ensuring that data is securely stored, accessed, and processed is a major responsibility for an Azure Data Engineer. Azure offers various security features like Azure Active Directory, encryption, and role-based access controls, all of which data engineers configure and manage.
Tools and Technologies in Azure Data Engineering
A Microsoft Azure Data Engineer uses a variety of tools provided by Azure to complete their tasks. Some key technologies in Azure Data Engineering include:
Azure Data Factory: A cloud-based data integration service that allows you to create, schedule, and orchestrate data pipelines. Azure Data Factory connects to various data sources, integrates them, and moves data seamlessly across systems.
Azure Data bricks: A collaborative platform for data engineers, data scientists, and analysts to work together on big data analytics and machine learning. It integrates with Apache Spark and provides a unified environment for data engineering and data science tasks.
Azure Synapse Analytics: This is a cloud-based analytical data warehouse solution that brings together big data and data warehousing. It allows Azure Data Engineers to integrate data from various sources, run complex queries, and gain insights into their data.
Azure Blob Storage & Azure Data Lake Storage: These are scalable storage solutions for unstructured data like images, videos, and logs. Data engineers use these storage solutions to manage large volumes of data, ensuring that it is secure and easily accessible for processing.
Azure SQL Database: A relational database service that is highly scalable and provides tools for managing and querying structured data. Azure Data Engineers often use this service to store and manage transactional data.
Azure Stream Analytics: A real-time data stream processing service that allows data engineers to analyse and process real-time data streams and integrate them with Azure analytics tools.
Why Choose an Azure Data Engineering Career?
The demand for skilled Azure Data Engineers has skyrocketed in recent years as organizations have realized the importance of leveraging data for business intelligence, decision-making, and competitive advantage. Professionals who earn an Azure Data Engineering Certification demonstrate their expertise in designing and managing complex data solutions on Azure, a skill set that is highly valued across industries such as finance, healthcare, e-commerce, and technology.
The growth of data and the increasing reliance on cloud computing means that Azure Data Engineers are needed more than ever. As businesses continue to migrate to the cloud, Microsoft Azure Data Engineer roles are becoming essential to the success of data-driven enterprises. These professionals help organizations streamline their data processes, reduce costs, and unlock the full potential of their data.
Benefits of Azure Data Engineering Certification
Industry Recognition: Earning an Azure Data Engineering Certification from Microsoft provides global recognition of your skills and expertise in managing data on the Azure platform. This certification is recognized by companies worldwide and can help you stand out in a competitive job market.
Increased Job Opportunities: With businesses continuing to shift their data infrastructure to the cloud, certified Azure Data Engineers are in high demand. This certification opens up a wide range of job opportunities, from entry-level positions to advanced engineering roles.
Improved Job Performance: Completing an Azure Data Engineer Course not only teaches you the theoretical aspects of Azure Data Engineering but also gives you hands-on experience with the tools and technologies you will be using daily. This makes you more effective and efficient on the job.
Higher Salary Potential: As a certified Microsoft Azure Data Engineer, you can expect higher earning potential. Data engineers with Azure expertise often command competitive salaries, reflecting the importance of their role in driving data innovation.
Staying Current with Technology: Microsoft Azure is continually evolving, with new features and tools being introduced regularly. The certification process ensures that you are up-to-date with the latest developments in Azure Data Engineering.
Azure Data Engineer Training Path
To start a career as an Azure Data Engineer, professionals typically begin by enrolling in an Azure Data Engineer Training program. These training courses are designed to provide both theoretical and practical knowledge of Azure data services. The Azure Data Engineer Course usually covers topics such as:
Core data concepts and analytics
Data storage and management in Azure
Data processing using Azure Data bricks and Azure Synapse Analytics
Building and deploying data pipelines with Azure Data Factory
Monitoring and managing data solutions on Azure
Security and compliance practices in Azure Data Engineering
Once you complete the training, you can pursue the Azure Data Engineering Certification by taking the Microsoft certification exam, which tests your skills in designing and implementing data solutions on Azure.
Advanced Skills for Azure Data Engineers
To excel as an Azure Data Engineer, professionals must cultivate advanced technical and problem-solving skills. These skills not only make them proficient in their day-to-day roles but also enable them to handle complex projects and large-scale data systems.
Conclusion
The role of an Azure Data Engineer is pivotal in today’s data-driven world. With the increasing reliance on cloud computing and the massive growth in data, organizations need skilled professionals who can design, implement, and manage data systems on Azure. By enrolling in an Azure Data Engineer Course and earning the Azure Data Engineering Certification, professionals can gain the expertise needed to build scalable and efficient data solutions on Microsoft’s cloud platform.
The demand for Microsoft Azure Data Engineer professionals is growing rapidly, offering a wealth of job opportunities and competitive salaries. With hands-on experience in the Azure ecosystem, data engineers are equipped to address the challenges of modern data management and analytics. Whether you’re just starting your career or looking to advance your skills, Azure Data Engineer Training provides the foundation and expertise needed to succeed in this exciting field.
Visualpath is the Best Software Online Training Institute in Hyderabad. Avail complete Azure Data Engineering worldwide. You will get the best course at an affordable cost.
Attend Free Demo
Call on - +91-9989971070.
WhatsApp: https://www.whatsapp.com/catalog/919989971070/
Visit Blog: https://visualpathblogs.com/
Visit: https://www.visualpath.in/online-azure-data-engineer-course.html
#Azure Data Engineer Course#Azure Data Engineering Certification#Azure Data Engineer Training In Hyderabad#Azure Data Engineer Training#Azure Data Engineer Training Online#Azure Data Engineer Course Online#Azure Data Engineer Online Training#Microsoft Azure Data Engineer
0 notes
Text
IBM Db2 AI Updates: Smarter, Faster, Better Database Tools
IBM Db2
Designed to handle mission-critical workloads worldwide.
What is IBM Db2?
IBM Db2 is a cloud-native database designed to support AI applications at scale, real-time analytics, and low-latency transactions. It offers database managers, corporate architects, and developers a single engine that is based on decades of innovation in data security, governance, scalability, and availability.
- Advertisement -
When moving to hybrid deployments, create the next generation of mission-critical apps that are available 24/7 and have no downtime across all clouds.
Support for all contemporary data formats, workloads, and programming languages will streamline development.
Support for open formats, including Apache Iceberg, allows teams to safely communicate data and information, facilitating quicker decision-making.
Utilize IBM Watsonx integration for generative artificial intelligence (AI) and integrated machine learning (ML) capabilities to implement AI at scale.
Use cases
Power next-gen AI assistants
Provide scalable, safe, and accessible data so that developers may create AI-powered assistants and apps.
Build new cloud-native apps for your business
Create cloud-native applications with low latency transactions, flexible scalability, high concurrency, and security that work on any cloud. Amazon Relational Database Service (RDS) now offers it.
Modernize mission-critical web and mobile apps
Utilize Db2 like-for-like compatibility in the cloud to modernize your vital apps for hybrid cloud deployments. Currently accessible via Amazon RDS.
Power real-time operational analytics and insights
Run in-memory processing, in-database analytics, business intelligence, and dashboards in real-time while continuously ingesting data.
Data sharing
With support for Apache Iceberg open table format, governance, and lineage, you can share and access all AI data from a single point of entry.
In-database machine learning
With SQL, Python, and R, you can create, train, assess, and implement machine learning models from inside the database engine without ever transferring your data.
Built for all your workloads
IBM Db2 Database
Db2 is the database designed to handle transactions of any size or complexity. Currently accessible via Amazon RDS.
IBM Db2 Warehouse
You can safely and economically conduct mission-critical analytical workloads on all kinds of data with IBM Db2 Warehouse. Watsonx.data integration allows you to grow AI workloads anywhere.
IBM Db2 Big SQL
IBM Db2 Big SQL is a high-performance, massively parallel SQL engine with sophisticated multimodal and multicloud features that lets you query data across Hadoop and cloud data lakes.
Deployment options
You require an on-premises, hybrid, or cloud database. Use Db2 to create a centralized business data platform that operates anywhere.
Cloud-managed service
Install Db2 on Amazon Web Services (AWS) and IBM Cloud as a fully managed service with SLA support, including RDS. Benefit from the cloud’s consumption-based charging, on-demand scalability, and ongoing improvements.
Cloud-managed container
Launch Db2 as a cloud container:integrated Db2 into your cloud solution and managed Red Hat OpenShift or Kubernetes services on AWS and Microsoft Azure.
Self-managed infrastructure or IaaS
Take control of your Db2 deployment by installing it as a conventional configuration on top of cloud-based infrastructure-as-a-service or on-premises infrastructure.
IBM Db2 Updates With AI-Powered Database Helper
Enterprise data is developing at an astonishing rate, and companies are having to deal with ever-more complicated data environments. Their database systems are under more strain than ever as a result of this. Version 12.1 of IBM’s renowned Db2 database, which is scheduled for general availability this week, attempts to address these demands. The latest version redefines database administration by embracing AI capabilities and building on Db2’s lengthy heritage.
The difficulties encountered by database administrators who must maintain performance, security, and uptime while managing massive (and quickly expanding) data quantities are covered in Db2 12.1. A crucial component of their strategy is IBM Watsonx’s generative AI-driven Database Assistant, which offers real-time monitoring, intelligent troubleshooting, and immediate replies.
Introducing The AI-Powered Database Assistant
By fixing problems instantly and averting interruptions, the new Database Assistant is intended to minimize downtime. Even for complicated queries, DBAs may communicate with the system in normal language to get prompt responses without consulting manuals.
The Database Assistant serves as a virtual coach in addition to its troubleshooting skills, speeding up DBA onboarding by offering solutions customized for each Db2 instance. This lowers training expenses and time. By enabling DBAs to address problems promptly and proactively, the database assistant should free them up to concentrate on strategic initiatives that improve the productivity and competitiveness of the company.
IBM Db2 Community Edition
Now available
Db2 12.1
No costs. No adware or credit card. Simply download a single, fully functional Db2 Community License, which you are free to use for as long as you wish.
What you can do when you download Db2
Install on a desktop or laptop and use almost anywhere. Join an active user community to discover events, code samples, and education, and test prototypes in a real-world setting by deploying them in a data center.
Limits of the Community License
Community license restrictions include an 8 GB memory limit and a 4 core constraint.
Read more on govindhtech.com
#IBMDb2AIUpdates#BetterDatabaseTools#IBMDb2#ApacheIceberg#AmazonRelationalDatabaseService#RDS#machinelearning#IBMDb2Database#IBMDb2BigSQL#AmazonWebServices#AWS#MicrosoftAzure#IBMWatsonx#Db2instance#technology#technews#news#govindhtech
0 notes
Text
Data is everywhere! Consider any industry, be it healthcare, finance, or education; there is a lot of information to be stored. Storing data can be done efficiently in the cloud using data storage services like Azure Blob store, Azure SQL Database, etc., or you can prefer keeping it on-premises. Whatever may be the case, a considerable amount of unstructured data is stored every day. Also, some enterprises will ingest data across both cloud & on-premises where there might be a need to combine data from both these sources to perform better analytics. What is Azure Data Factory? In the above situations, it becomes more important to transform and move data across different datastores, and this is when Azure Data Factory comes into play! Data Factory is a cloud-based ETL (Extract-Transform-load) and data integration service that allows you to automate data movement between various data stores and perform data transforming by creating pipelines. Where can I use it? Data Factory helps in the same way as any other traditional ETL tool, which helps extract raw data from one/multiple sources to transform & load them to any destination like a Data warehouse. But Data Factory differs from other ETL tools by performing these tasks without any code to be written. Now, don’t you agree that it is a solution that can perfectly fit in if you are looking to transform all your unstructured data into a structured one? Before getting into the concepts, here is a quick recap of Data Factory’s history The version we are using has improved and developed in numerous ways compared to the first version made generally available in 2015. Back then, you have to build a workflow only in Visual Studio. But the version 2 (public preview in 2017) was released to overshadow all the challenges in v1. With Data Factory v2, build code-free ETL processes where you can also leverage 90+ built-in connectors to acquire data from any data store of your choice. Top-level Concepts Now imagine, if you are moving a CSV file from a Blob Storage to a customer table in SQL database, then all the below-mentioned concepts will get involved, Here are the six essential components that you must know, Pipeline A pipeline is a logical grouping of activities that performs a unit of work. For example, a pipeline performs a series of tasks like ingesting data from Blob Storage, transforming it into meaningful data, and then writing it into the SQL Database. It involves mapping the activities in a sequential. So, you can automate the ETL process by creating any number of pipelines for a particular Data Factory. Activities These are the actions that get performed on the data in a pipeline. It includes three activities – Data Movement (Copy), Data transformation & control flow activities. But copying & transforming are the two core activities of Azure Data Factory. So here, copy data activity will get the CSV file from a Blob and loads it into the database, during which you can also convert the file formats. And transformation is mainly done with the help of a capability called Data Flows, which allows developing data transformation logic without using code. Datasets The datasets show what kind of data you are pulling from the data stores. So, it simply points to the data used in the activity as an input or output. Linked Services Linked Services helps to connect other resources with Data Factory, acting like connection strings. When considering the above example, Linked Service will serve as a definition of connectivity to the Blob storage & performs authorization. Similarly, the target (SQL Database) will also have a separate Linked Service. Triggers Triggers are to execute your pipelines without any manual intervention (i.e.,) it determines when a pipeline should get completed. There are three essential triggers in Data Factory, Schedule Trigger: A trigger that invokes a pipeline on a set schedule. You can specify both the date and time on which the trigger should initiate the pipeline run.
Tumbling Window Trigger: A trigger that operates on a periodic interval. Event-based Trigger: This trigger executes whenever an event occurs. For instance, when a file is uploaded or deleted in Blob Storage, the trigger will respond to that event. The triggers and pipelines will have a many-to-many relationship where multiple Triggers can kick off a single pipeline, or a single Trigger can also kick off various pipelines. But as an exception, Tumbling Window Triggers alone will have one-to-many relation with the pipelines. Integration Runtime Integration Runtime is the compute infrastructure used by the Data Factory to provide the following data integration capabilities (Data Flow, Data Movement, Activity dispatch, and SSIS package execution) across different networks. It has three types: Azure integration runtime: This is preferred when you want to copy and transform data between data stores in the cloud. Self-hosted integration runtime: Utilize this when you want to execute using On-premises data stores. Azure-SSIS integration runtime: It helps to execute SSIS packages through Data Factory. I hope that you have understood what a Data Factory is and how it works. We are now moving into its monitoring and managing aspects. Monitoring Azure Data Factory The Azure Monitor supports monitoring your pipeline runs, trigger runs, integration runtimes, and other various metrics. It has an interactive Dashboard where you will view the statistics of all the runs involved in your Data Factory. In addition, you get to create alerts on the metrics to get notified whenever something goes wrong in the Data Factory. Azure Monitor does offer all the necessities for monitoring and alerting, but the real-time business demands much more than that! Also, with the Azure Portal, it becomes difficult to manage when there are Data Factories with different pipelines, data sources across various Subscriptions, Regions, and Tenants. So, here is a third-party tool, Serverless360 that can help your operations and support team manage and monitor the Data Factory much more efficiently. Capabilities of Serverless360 Serverless360 will serve as a complete support tool for managing and monitoring your Azure resources. It helps to achieve application-level grouping and extensive monitoring features that are not available in the Azure portal. A glimpse of what Serverless360 can offer: An interactive and vivid dashboard for visualizing complex data metrics. Group all the siloed Azure resources using business application feature to achieve application-level monitoring. Get one consolidated monitoring report to know the status of all your Azure resources. Monitor the health status and get a report at regular intervals, say every 2 hours. Configure Threshold monitoring rules and get alerted whenever your resource is not in the expected state, and it can automatically correct it and bring it back to the active state. Monitor the resources on various metrics like canceled activity runs, failed pipeline runs, succeeded trigger runs, etc., without any additional cost. Conclusion In this blog, I gave an overview of one of the essential ETL Tools (Azure Data Factory) and its core features that you should be aware of if you plan to use it for your business. Along with that, I have also mentioned a third-party Azure support tool capable of reducing the pressure in managing and monitoring your resources. I hope you had a great time reading this article!
0 notes