#AI-driven solutions
Explore tagged Tumblr posts
Text
HUMAN FORM (EXERCISE 3 DRAFT 3)
Imagining what the Human Form would be 80 years in the future
Food
Food production will integrate AI-driven vertical farms within cities, enabling year-round cultivation of algae, lab-grown proteins, and tailored micronutrient blends. Personalized meal pods will 3D-print food using real-time health data to match nutritional requirements. Urban food forests and micro-gardens will provide fresh produce in every neighborhood, significantly reducing transport emissions and waste.
Water
District-wide closed-loop water systems will collect, purify, and recycle water seamlessly, minimizing wastage. Innovations like fog-harvesting nets and water-from-air technology will turn humidity into clean water in arid regions. Smart water analyzers will empower citizens to track usage and quality, optimizing conservation practices.
Housing
Homes will be constructed with self-healing materials that repair cracks and extend the building’s lifespan. Biodiverse rooftops will serve as both insulation and habitats for pollinators, while modular interiors will adapt to changing family needs. Shared community spaces will prioritize functionality and mental well-being, fostering collaboration and connection.
Education
Education will blend virtual reality classrooms with real-world problem-solving projects, ensuring a balance of theory and application. AI tutors will provide tailored support, while multi-sensory immersive experiences will replace traditional rote learning. Empathy and cross-cultural understanding will be core subjects, creating globally connected citizens.
Healthcare
AI-powered micro-implants will continuously monitor bodily functions, providing predictive diagnostics and preventive care. Tele-health hubs within homes will deliver instant consultations and treatments, eliminating wait times. Advanced regenerative medicine will repair damaged organs, and mental health solutions will include virtual therapy spaces with AI emotional support companions.
Social Equity
Equity will be enhanced through decentralized systems that allocate resources efficiently, addressing gaps in real time. Universal access to essential services will be supported by AI equity monitors that detect bias or exclusion in policies and take corrective action. Community hubs will provide tools for skills development, entrepreneurship, and social mobility.
Gender Equality
Gender-sensitive algorithms will analyze decision-making processes across industries, ensuring inclusivity and equity. All public and private institutions will adopt universal gender-neutral facilities and policies. Representation in leadership and media will reflect the diversity of society, erasing traditional gender biases and fostering equality.
Work & Income
Work will be redefined, focusing on creativity, innovation, and societal impact. People will engage in passion-driven projects, supported by universal basic income and flexible work structures. Collaborative hubs will allow freelancers and teams to work together dynamically, while automation will handle repetitive, low-value tasks.
Energy / Electricity
Clean energy will dominate, with wireless energy transfer systems powered by fusion reactors and solar satellites. Buildings will operate as mini power plants, generating and storing their energy through solar tiles, wind walls, and kinetic technologies. AI will forecast energy demands, ensuring sustainable usage and zero wastage.
Peace & Justice
AI mediation will revolutionize conflict resolution, detecting underlying tensions and resolving them proactively. Community-driven justice models will prioritize rehabilitation and dialogue over punishment. Citizens will have access to real-time updates on laws and policies, fostering accountability and transparency in governance.
Transportation
Seamless, multi-modal transportation systems will integrate maglev trains, shared autonomous pods, and aerial drones. Roads will be replaced with green pedestrian corridors, while self-driving vehicles will reduce accidents and traffic. Efficient, low-cost public transit will make private car ownership obsolete.
Political Voice
Blockchain technology will power secure, transparent voting, enabling real-time decision-making by citizens on policies and budgets. Governments will adopt participatory governance models where community-led councils actively shape policies. AI-driven tools will eliminate lobbying influence, ensuring fair representation.
Air Pollution
Air pollution will be managed by smart bioremediation systems, such as genetically modified plants and moss walls in urban areas. Autonomous filtration drones will purify air at hotspots, and green technologies will eliminate pollution sources, making cities carbon-neutral and breathable.
Noise Pollution
Cities will incorporate soundscaping technology that redirects or neutralizes excess noise. Acoustic zoning will ensure quieter residential areas, while sound-absorbing materials in buildings and transit systems will maintain peace. Noise-free transportation and natural sound corridors will offer tranquil public spaces.
Non-Human Life
Urban planning will integrate wildlife bridges, aquatic corridors, and microhabitats for insects and small animals. AI-driven biodiversity monitors will balance urban growth with ecosystem preservation. Coexistence with non-human life will be a fundamental principle of development.
Chemical Pollution
Sustainable production methods will eliminate toxic chemicals from supply chains, while industrial processes will incorporate "green chemistry" principles. Waste treatment plants will convert pollutants into reusable resources, ensuring a circular approach to waste management.
Water Bodies & Supply
Advanced desalination technologies, powered by renewable energy, will make seawater a primary source of potable water. Floating wetlands will cleanse waterways and restore biodiversity, while AI systems will monitor water ecosystems to maintain balance and purity.
Waste Management
Circular economies will eliminate waste entirely, with AI directing materials to recycling or composting streams. Smart appliances will optimize waste segregation at the household level, and community repair hubs will extend product lifespans. Organic waste will fuel bioenergy systems, closing the loop sustainably.
Land Use, Streets & Public Spaces
Urban design will prioritize vibrant, multi-functional public spaces that double as community hubs. Streets will serve as extensions of green spaces, accommodating pedestrians, cyclists, and small autonomous vehicles. Public art installations and cultural activities will enhance urban vibrancy.
Ocean Pollution
Self-sustaining robotic fleets will continuously clean oceans, removing pollutants and plastics. AI-powered monitoring systems will prevent future pollution by regulating shipping and fishing practices. Marine reserves will expand, protecting biodiversity and replenishing aquatic ecosystems.
Effects of Climate Change
Resilient urban designs will combat rising temperatures, sea levels, and extreme weather. Reforestation, carbon capture, and soil restoration projects will help reverse environmental damage. Predictive AI will provide climate adaptation strategies, safeguarding communities worldwide.
Urban Agriculture & Greenification
Vertical gardens and urban forests will be integral to cityscapes, providing food, shade, and improved air quality. Smart sensors will optimize agricultural practices, reducing water use and maximizing yields. Public spaces will feature edible landscapes, promoting food sovereignty and community engagement.
Gender & Sexuality
Comprehensive inclusion policies will enable individuals to freely express their identities. Media, education, and workplaces will embrace and celebrate diverse perspectives, creating a culture of acceptance and belonging. Healthcare will address gender-specific needs with sensitivity and precision.
Diversity & Inclusion
AI-driven policies will eliminate biases in recruitment, education, and governance, ensuring diverse representation at all levels. Cultural festivals and public forums will celebrate differences, fostering a shared sense of humanity and respect for all.
Accessibility
Cities will adopt universal design principles, ensuring accessibility for people of all abilities. AI-powered assistance systems will enable seamless navigation, while sensory-friendly environments will accommodate diverse needs. Inclusivity will be ingrained in all public and private infrastructure.
Sustainability
Regenerative practices will redefine industries, with circular economies prioritizing zero waste and zero emissions. Collaboration between nations will ensure sustainable development goals are met, balancing progress with planetary health.
#Future of Humanity#Imagining the Future#Sustainable Living#Futuristic Society#Green Innovation#Inclusive Cities#AI-Driven Solutions#Urban Transformation
0 notes
Text
The Future of AI: A Symphony of Progress for Humanity
AI transforms healthcare, education, and the environment. Discover how humans and technology collaborate for a smarter, more sustainable future.
What’s On My Mind Today? As the sun rises on a new era of technological advancement, the concept of What’s On My Mind Today? AI is no longer just an idea from science fiction; it’s shaping our world in ways that were unimaginable just a few decades ago. From medicine to education, environmental conservation to creative expression, the possibilities seem endless. But this isn’t just about…
#AI and environment#AI collaboration#AI in healthcare#AI technology trends#AI-driven solutions#creative AI#Ethical AI#future of AI#human-AI partnership#personalized education#sustainable AI
0 notes
Text
What is the role of AI in fraud prevention?
AI fraud detection isn’t just a theory; companies worldwide are using it. Fraudulent activities have grown into a complex threat, extending far beyond financial losses-they can erode customer trust, damage brand reputation, and result in costly regulatory penalties.
Fraud scams and bank fraud schemes resulted in $485.6 billion in losses globally last year, according to Nasdaq’s 2024 Global Financial Crime Report.
From safeguarding online transactions to protecting sensitive data, staying ahead of sophisticated fraudsters has never been more critical. Fortunately, artificial intelligence (AI) has emerged as a powerful technology.
By leveraging advanced machine learning algorithms and predictive analytics, AI identifies and mitigates threats with unprecedented speed and accuracy to continuously adapt new fraud tactics. This has positioned AI as an indispensable tool, safeguarding financial transactions and instilling confidence in businesses and consumers. Let’s explore the role of AI in making online transactions safer.
The rising threat of payment fraud to be aware of
Digital transactions are revolutionizing the financial landscape, and the menace of payment fraud is rapidly increasing. This presenting formidable challenges to enterprises, financial institutions, and individuals. As the proliferation of online transactions increases, so does the ingenuity of fraudsters, who relentlessly innovate to exploit the evolving intricacies of payment systems. The factors driving this growing threat are:
Expansion of digital payment platforms
Increased transaction volume
Use of AI by hackers
Regulatory challenges
Synthetic identity fraud
Impact on businesses and consumers
Fraud not only results in direct financial losses but also has broader implications:
Financial losses
Reputational damage
Operational disruptions
Increased costs
Let’s explore the types of fraudulent activities and expected loss:
Fraud Type: Global online payment fraud losses
Expected Loss: $91 billion
Date: 2028
Fraud Type: US eCommerce fraud totals
Expected Loss: $48 billion
Date: 2023
Fraud Type: Global eCommerce payment fraud losses
Expected Loss: $343 billion
Date: 2023 -2027
Fraud Type: Global AI fraud loss (conservative scenario)
Expected Loss: $1 trillion
Date: 2030
Fraud Type: Synthetic Identity fraud
Expected Loss: $23 billion
Date: 2030
Fraud Type: Credit card losses
Expected Loss: $43 billion
Date: 2026
Fraud Type: Total amount of regulatory fines against institutions for KYC failures (including money laundering)
Expected Loss: $5 billion
Date: 2022
3 key use cases of generative AI for the financial industry
The adoption of generative AI in financial sector makes a substantial impact in detecting financial fraud. It analyzes vast amount of data in real time, enhance security measures, and increase customer support and security.
Read more
The role of AI in fraud detection for increased precision and efficiency
Real-time transaction monitoring
AI offers significant advantages in fraud detection by analyzing vast amounts of transactional data in real time. Unlike traditional systems that rely on static rules and respond slowly to new threats, AI uses machine learning algorithms to identify patterns and anomalies, quickly recognizing suspicious activities. This capability for real-time monitoring allows for immediate intervention, preventing fraudulent transactions before they inflict damage.
Predictive analytics and pattern recognition
AI excels at identifying patterns within complex datasets, making it highly effective at predicting and preventing fraud. By analyzing historical data, AI models uncover subtle correlations and trends that might indicate fraudulent behavior. These predictive analytics capabilities allow AI to forecast potential fraud scenarios and implement proactive measures, thereby reducing the risk of fraud before it occurs.
Enhanced accuracy and reduction of false positives
A common challenge in fraud detection is false positives, where legitimate transactions are flagged as fraudulent. AI improves accuracy by analyzing a wider range of data points and context, allowing it to better distinguish between genuine and fraudulent transactions. This precision enhances security and reduces unnecessary disruptions, improving the user experience.
Behavioral biometrics
AI-powered behavioral biometrics is a cutting-edge fraud detection method that analyzes unique user behaviors like typing speed, mouse movements, and interaction patterns. By creating a behavioral profile for each user, AI can detect deviations that may indicate fraud, adding a layer of security that is hard for fraudsters to replicate and effective against account takeovers and identity theft.
How artificial intelligence in investment management will give you an edge
The key enterprises applications of AI in investment management are to scrape smartphone reviews from various websites and extract themes to highlight essential topic and trends.
How fraud detection using AI is making banking and financial transactions safer
Identifying fake accounts: AI detects synthetic identities or fake accounts created using a mix of original and fabricated information. It analyzes account behavior and background data, allowing banks to identify and prevent these fraudulent accounts from causing harm.
Preventing money laundering: Artificial intelligence analyzes transaction patterns to spot potential money laundering activities. It compares transactions against known laundering techniques and flags suspicious patterns for further investigation.
Phishing attacks: AI helps detect phishing attempts by analyzing email patterns and content to identify fraudulent messages that attempt to steal sensitive information. AI system filters the message and alerts the users to suspicious communications, reducing the risk of successful phishing attacks.
Credit card theft: The technology identifies patterns consistent with credit card theft, such as unusual spending or transactions from locations not previously associated with the cardholder. This real-time analysis allows banks to flag or block potentially fraudulent transactions.
Document forgery: AI tools can analyze documents for signs of forgery by comparing them with known genuine documents and identifying discrepancies. This helps to detect and prevent the use of forged documents for fraudulent purposes, such as opening fake accounts or applying for loans.
Fraudulent loan applications: AI analyzes loan applications for inconsistencies or red flags that might indicate fraud. By evaluating the application data against historical patterns and known fraud indicators, AI helps prevent fraudulent loan disbursements.
Unusual transaction patterns: AI can detect unusual transaction patterns that deviate from a user’s historical behavior, such as sudden large transactions or transactions in unusual locations. These anomalies are flagged for further review or automatic action.
The future of AI in fraud prevention
1. Enhanced detection capabilities
AI algorithms are becoming more advanced in analyzing vast amounts of data to detect anomalies and suspicious patterns. Machine learning models, particularly those utilizing deep learning, can recognize subtle deviations from normal behavior that traditional systems might miss. The ability to process and analyze data in real time enhances the accuracy of fraud detection and reduces the chances of false positives.
2. Adaptive learning
One of AI’s most significant advantages is its ability to learn and adapt. Fraud tactics are continuously evolving, and static fraud prevention systems can quickly become obsolete. AI-powered systems, however, use adaptive learning to stay ahead of emerging threats. By continuously training on new data and adjusting algorithms accordingly, AI can evolve alongside the fraudsters’ tactics, improving its effectiveness over time.
3. Behavioral analytics
AI in financial operations enhances fraud prevention by analyzing user behavior patterns. By establishing a baseline of normal behavior for each user, AI systems can detect unusual activities that deviate from the norm. For example, if a user who typically logs in from a specific location suddenly accesses their account from a different country, AI can flag this as a potential fraud risk and trigger additional verification steps.
Real-life use cases: AI preventing fraud enhances payment strategies
JP Morgan
The global financial leader, JP Morgan, has implemented an AI system known as DocLLM to enhance their fraud detection capabilities. This system leverages advanced natural language processing and machine learning techniques to swiftly analyze vast amounts of legal documents. In just a few seconds, DocLLM can sift these documents to identify inconsistencies, anomalies, and warning signs that might indicate fraudulent activities.
This proactive approach helps the bank mitigate risks, protect its assets, and maintain trust with its clients by preventing fraudulent transactions before they can cause significant harm.
Mastercard
Mastercard’s AI-based platform, Decision Intelligence, plays a critical role in fraud prevention. It uses generative AI to analyze cardholder spending patterns in real time, evaluating the likelihood of fraud for each transaction as it occurs.
The integration of AI technology has greatly enhanced the security of Mastercard’s payment network. The platform’s efficiency is evident, as it has already helped Mastercard “score and safely approve 143 billion transactions a year,” providing a robust layer of protection for cardholders and the company itself.
Capital One
Capital One has integrated AI into its customer service strategy through Eno, a virtual assistant launched in 2017. It is designed to interact with users via mobile apps, text messages, email, and desktop. It allows answering customer questions, sending fraud alerts, and handling routine tasks like paying credit card bills, tracking account balances, and checking transactions.
What sets Eno apart is its ability to communicate in a natural, human-like manner, even incorporating emojis into its responses. This makes interactions with the AI feel more personal and engaging, enhancing the overall customer experience while ensuring that essential banking tasks are handled efficiently and securely.
Autonomous AI Agents for Finance: The future is now
The future of finance is autonomous as AI agents help finance leaders focus on strategic tasks, drive innovation by identifying new opportunities, influence change, align goals, and drive value.
Read more
Leverage Softweb’s AI-driven solutions for fraud detection and prevention
In 2024 and beyond, banks and financial institutions are set to enhance their fraud prevention strategies by investing in advanced analytics and harnessing the transformative potential of AI. This approach will boost efficiency and effectiveness in detecting fraud.
Adopting a risk-based methodology, managing alerts, evaluating various scenarios, and responding quickly to emerging threats can create a secure environment for digital transactions. Softweb’s AI consulting services offer end-to-end solutions such as:
Strategic development to deployment and maintenance
Analysis of market trends
Risk management and compliance
Fraud detection and prevention strategy
Customer segment and personalization
Contact our AI consultants to discuss your use case.
Originally published at softwebsolutions on August 30, 2024.
#AI-driven solutions#ai consulting#AI in fraud prevention#AI in fraud detection#AI in financial industry#AI consulting services
0 notes
Text
Transform Customer Service with Deep Brain AI Avatars!
Welcome to our deep dive into DeepBrain AI, a groundbreaking player in the generative AI landscape. In a world where artificial intelligence is rapidly evolving, DeepBrain AI stands out by harnessing the power of advanced algorithms to create realistic and engaging content. This innovative tool is not just a technological marvel; it’s reshaping how we think about content creation, communication, and even personal branding.
As tech enthusiasts, understanding tools like DeepBrain AI is crucial for both personal and professional growth. Whether you're a content creator, marketer, or simply someone curious about the future of technology, grasping the capabilities of AI can open up new avenues for creativity and efficiency.
In this video, we’ll explore how DeepBrain AI works, its applications across various industries, and why it’s essential to stay informed about such advancements. By the end, you’ll not only appreciate the significance of DeepBrain AI but also feel empowered to leverage its potential in your own projects. So, let’s embark on this exciting journey into the world of generative AI and discover how it can transform our lives!
Target Audience:
The primary audience for DeepBrain AI encompasses a diverse range of individuals and organizations, including content creators, marketers, and businesses eager to harness the power of artificial intelligence. Content creators, such as bloggers, video producers, and social media influencers, can utilize DeepBrain AI to streamline their workflow, generate engaging content, and enhance their creative output.
Marketers, on the other hand, can leverage this tool to craft personalized campaigns, analyze consumer behavior, and optimize their strategies for better engagement. Businesses of all sizes are also part of this audience, as they seek innovative solutions to improve efficiency, reduce costs, and stay competitive in a rapidly changing market.
Within this audience, there are varying levels of expertise, ranging from beginners who are just starting to explore AI tools to advanced users who are already familiar with generative AI technologies. DeepBrain AI caters to all these segments by offering user-friendly interfaces and robust features that can be tailored to different skill levels. For beginners, it provides an accessible entry point into AI, while advanced users can take advantage of its sophisticated capabilities to push the boundaries of their projects. Ultimately, DeepBrain AI empowers each segment to unlock new possibilities and drive success in their respective fields.
List of Features:
DeepBrain AI boasts a range of impactful features that set it apart in the generative AI landscape. First and foremost is its advanced natural language processing (NLP) capability, which allows the tool to understand and generate human-like text. This feature can be utilized in real-world applications such as chatbots for customer service, where it can provide instant responses to inquiries, enhancing user experience.
Next is its robust content generation capability, enabling users to create articles, social media posts, and marketing copy with minimal effort. For instance, a marketer can input key themes and receive a fully developed campaign draft in seconds, saving time and resources.
Another standout feature is its ability to analyze and summarize large volumes of data, making it invaluable for businesses looking to extract insights from reports or customer feedback. This unique selling point differentiates DeepBrain AI from other generative AI products, as it combines content creation with data analysis in a seamless manner.
Additionally, DeepBrain AI offers customizable templates tailored to various industries, allowing users to maintain brand consistency while leveraging AI-generated content. These features collectively empower users to enhance productivity, creativity, and decision-making in their professional endeavors.
Conclusion:
In summary, DeepBrain AI represents a significant advancement in the generative AI landscape, offering powerful features that cater to a diverse audience, including content creators, marketers, and businesses. Its advanced natural language processing and content generation capabilities enable users to produce high-quality material efficiently, while its data analysis features provide valuable insights that can drive strategic decisions.
Key takeaways from this video include the importance of understanding how DeepBrain AI can enhance productivity and creativity, regardless of your level of expertise. Whether you’re just starting out or are an advanced user, this tool has something to offer that can elevate your projects and initiatives.
We hope you found this exploration of DeepBrain AI informative and engaging. If you enjoyed the content, please consider subscribing to our channel, liking this video, and sharing it with others who might benefit from learning about AI tools. Don’t forget to check out our related content for more insights into the world of artificial intelligence and how it can transform your personal and professional life. Thank you for watching, and we look forward to seeing you in our next video!
#DeepBrain AI#generative AI#hyperrealistic avatars#virtual humans#AI platform#deep learning techniques#lifelike digital representations#real-time interaction#customer service AI#virtual assistance#entertainment technology#education AI#personalized interactions#speech synthesis#natural language processing#emotion recognition#user experience enhancement#content creation tools#innovative AI solutions#digital avatars#AI technology#virtual interactions#advanced AI features#business applications#digital representation#interactive avatars#AI-driven solutions#virtual human technology#engaging content#AI in education
1 note
·
View note
Text
Transform manufacturing with generative AI: streamline processes, enhance quality, predict maintenance, and accelerate innovation.
#AI Integration In Manufacturing#AI-Driven Design Tools#AI-Driven Solutions#Generative AI In Manufacturing#Generative AI Models#Product Design And Development#Saas Professionals In The USA#Saas Providers
0 notes
Text
Transform manufacturing with generative AI: streamline processes, enhance quality, predict maintenance, and accelerate innovation.
#AI Integration In Manufacturing#AI-Driven Design Tools#AI-Driven Solutions#Generative AI In Manufacturing#Generative AI Models#Product Design And Development#Saas Professionals In The USA#Saas Providers
0 notes
Text
Transform manufacturing with generative AI: streamline processes, enhance quality, predict maintenance, and accelerate innovation.
#AI Integration In Manufacturing#AI-Driven Design Tools#AI-Driven Solutions#Generative AI In Manufacturing#Generative AI Models#Product Design And Development#Saas Professionals In The USA#Saas Providers
0 notes
Text
Lenovo Premier Support Plus is now available in the Philippines
Lenovo Premier Support provides proactive IT solutions, ensuring smooth operations and minimizing downtime. Experience top-tier support and stay ahead in today's fast-paced digital landscape. #ProductivityBoost #LenovoPremierSupport @LenovoPhils
The new global study reveals that a majority of 12,000 employees surveyed (91%) believe they would be more productive if their IT issues at work were resolved quickly and effectively. Another 74% say poor IT support has decreased their motivation at work. Results show an efficient and effective IT support system needs to be in place to power today’s hybrid workforce. Lenovo Premier Support Plus…
View On WordPress
#AI-driven solutions#digital intelligence#efficient IT support#employee experience#employee motivation#global coverage#hybrid workforce#IT issues resolution#IT support service#Lenovo Premier Support#productivity enhancement
0 notes
Text
Top 5 DeepSeek AI Features Powering Industry Innovation
Table of Contents1. The Problem: Why Legacy Tools Can’t Keep Up2. What Makes DeepSeek AI Unique?3. 5 Game-Changing DeepSeek AI Features (with Real Stories)3.1 Adaptive Learning Engine3.2 Real-Time Anomaly Detection3.3 Natural Language Reports3.4 Multi-Cloud Sync3.5 Ethical AI Auditor4. How These Features Solve Everyday Challenges5. Step-by-Step: Getting Started with DeepSeek AI6. FAQs: Your…
#affordable AI solutions#AI automation#AI for educators#AI for entrepreneurs#AI for non-techies#AI for small business#AI in manufacturing#AI innovation 2024#AI time management#business growth tools#data-driven decisions#DeepSeek AI Features#ethical AI solutions#healthcare AI tools#no-code AI tools#Predictive Analytics#real-time analytics#remote work AI#retail AI features#startup AI tech
2 notes
·
View notes
Text
AI EXPRESS - ETHICAL CONSIDERATIONS IN AI.
Rubem Didini Filho

The increasing integration of AI technologies into various aspects of society raises crucial ethical considerations that require careful attention. The sources, along with our previous discussions, highlight the importance of addressing these concerns to ensure AI's responsible and beneficial development and deployment. One major ethical concern is algorithmic bias, where AI systems can inadvertently inherit and perpetuate biases present in the training data. This can result in unfair or discriminatory outcomes, especially in sensitive areas like hiring, loan applications, or criminal justice. For example, a facial recognition system trained primarily on images of a particular demographic group may be less accurate at recognizing individuals from other groups, leading to biased outcomes.
Data privacy and security are also paramount ethical considerations. As AI systems often rely on massive datasets, including personal information, protecting this data from misuse is essential. Ethical data handling practices include obtaining informed consent for data collection, anonymizing data when possible, and implementing robust security measures to prevent unauthorized access or breaches. The sources emphasize the need to balance the benefits of AI with the potential risks to individual privacy.
The sources, particularly our conversation about transparency, also emphasize the importance of transparency and explainability in AI systems. Complex AI models, especially deep learning algorithms, can operate as "black boxes," making it difficult to understand how they reach their decisions. This lack of transparency raises concerns about accountability and potential biases. Ethical AI development emphasizes creating explainable AI (XAI), where the reasoning behind AI's decisions can be understood and audited. This transparency fosters trust and allows for the identification and mitigation of potential biases or errors.
Ethical Considerations in AI: The sources emphasize the importance of ethics in AI. As AI systems become more sophisticated and integrated into various aspects of our lives, it is crucial to ensure they are developed and used responsibly and ethically. Several key ethical considerations arise from the sources:
Algorithmic Bias: AI algorithms can inherit and perpetuate biases present in the training data. This can lead to discriminatory outcomes, especially in sensitive areas like hiring, loan applications, or criminal justice. For example, if a facial recognition system is trained primarily on images of light-skinned individuals, it may be less accurate at recognizing people with darker skin tones, potentially leading to unfair or biased outcomes.
Data Privacy and Security: AI systems often rely on vast amounts of data, including personal information. Ensuring the privacy and security of this data is paramount. Ethical considerations include obtaining informed consent for data collection, anonymizing data when possible, and implementing robust security measures to prevent unauthorized access or misuse.
Transparency and Explainability: The decision-making processes of complex AI systems, particularly deep learning models, can be opaque and difficult to understand. This lack of transparency raises concerns about accountability. Ethical AI development emphasizes creating explainable AI (XAI), where the reasoning behind an AI's decision can be understood and audited, fostering trust and allowing for the identification and mitigation of potential biases.
Job Displacement and Economic Impact: The increasing automation capabilities of AI raise concerns about job displacement across various sectors. As AI systems become capable of performing tasks previously done by humans, ethical considerations involve addressing potential job losses, providing retraining opportunities, and ensuring a fair transition for workers affected by automation.
Responsibility and Accountability: Determining responsibility when an AI system makes an error or causes harm is a complex ethical issue. Establishing clear lines of accountability for AI developers, deployers, and users is essential. Ethical frameworks must address questions of liability and potential legal repercussions in cases where AI systems cause unintended consequences.
The sources highlight the need for ongoing dialogue and the development of ethical guidelines and regulations to address these concerns. As AI continues to evolve, ethical considerations must be at the forefront to ensure that these technologies are used for the benefit of humanity, promoting fairness, justice, and well-being.
The increasing integration of AI technologies into various aspects of society raises crucial ethical considerations that require careful attention. The sources, along with our previous discussions, highlight the importance of addressing these concerns to ensure AI's responsible and beneficial development and deployment. One major ethical concern is algorithmic bias, where AI systems can inadvertently inherit and perpetuate biases present in the training data. This can result in unfair or discriminatory outcomes, especially in sensitive areas like hiring, loan applications, or criminal justice. For example, a facial recognition system trained primarily on images of a particular demographic group may be less accurate at recognizing individuals from other groups, leading to biased outcomes.
Data privacy and security are also paramount ethical considerations. As AI systems often rely on massive datasets, including personal information, protecting this data from misuse is essential. Ethical data handling practices include obtaining informed consent for data collection, anonymizing data when possible, and implementing robust security measures to prevent unauthorized access or breaches. The sources emphasize the need to balance the benefits of AI with the potential risks to individual privacy.
The sources, particularly our conversation about transparency, also emphasize the importance of transparency and explainability in AI systems. Complex AI models, especially deep learning algorithms, can operate as "black boxes," making it difficult to understand how they reach their decisions. This lack of transparency raises concerns about accountability and potential biases. Ethical AI development emphasizes creating explainable AI (XAI), where the reasoning behind AI's decisions can be understood and audited. This transparency fosters trust and allows for the identification and mitigation of potential biases or errors.
Ethical Considerations in AI: The sources emphasize the importance of ethics in AI. As AI systems become more sophisticated and integrated into various aspects of our lives, it is crucial to ensure they are developed and used responsibly and ethically. Several key ethical considerations arise from the sources:
Algorithmic Bias: AI algorithms can inherit and perpetuate biases present in the training data. This can lead to discriminatory outcomes, especially in sensitive areas like hiring, loan applications, or criminal justice. For example, if a facial recognition system is trained primarily on images of light-skinned individuals, it may be less accurate at recognizing people with darker skin tones, potentially leading to unfair or biased outcomes.
Data Privacy and Security: AI systems often rely on vast amounts of data, including personal information. Ensuring the privacy and security of this data is paramount. Ethical considerations include obtaining informed consent for data collection, anonymizing data when possible, and implementing robust security measures to prevent unauthorized access or misuse.
Transparency and Explainability: The decision-making processes of complex AI systems, particularly deep learning models, can be opaque and difficult to understand. This lack of transparency raises concerns about accountability. Ethical AI development emphasizes creating explainable AI (XAI), where the reasoning behind an AI's decision can be understood and audited, fostering trust and allowing for the identification and mitigation of potential biases.
Job Displacement and Economic Impact: The increasing automation capabilities of AI raise concerns about job displacement across various sectors. As AI systems become capable of performing tasks previously done by humans, ethical considerations involve addressing potential job losses, providing retraining opportunities, and ensuring a fair transition for workers affected by automation.
Responsibility and Accountability: Determining responsibility when an AI system makes an error or causes harm is a complex ethical issue. Establishing clear lines of accountability for AI developers, deployers, and users is essential. Ethical frameworks must address questions of liability and potential legal repercussions in cases where AI systems cause unintended consequences.
The sources highlight the need for ongoing dialogue and the development of ethical guidelines and regulations to address these concerns. As AI continues to evolve, ethical considerations must be at the forefront to ensure that these technologies are used for the benefit of humanity, promoting fairness, justice, and well-being.
2 notes
·
View notes
Text
Boost E-commerce in Saudi Arabia with ML-Powered Apps
In today's digital era, the e-commerce industry in Saudi Arabia is rapidly expanding, fueled by increasing internet penetration and a tech-savvy population. To stay competitive, businesses are turning to advanced technologies, particularly Machine Learning (ML), to enhance user experiences, optimize operations, and drive growth. This article explores how ML is transforming the e-commerce landscape in Saudi Arabia and how businesses can leverage this technology to boost their success.
The Current E-commerce Landscape in Saudi Arabia
The e-commerce market in Saudi Arabia has seen exponential growth over the past few years. With a young population, widespread smartphone usage, and supportive government policies, the Kingdom is poised to become a leading e-commerce hub in the Middle East. Key players like Noon, Souq, and Jarir have set the stage, but the market is ripe for innovation, especially with the integration of Machine Learning.
The Role of Machine Learning in E-commerce
Machine Learning, a subset of Artificial Intelligence (AI), involves the use of algorithms to analyze data, learn from it, and make informed decisions. In e-commerce, ML enhances various aspects, from personalization to fraud detection. Machine Learning’s ability to analyze large datasets and identify trends is crucial for businesses aiming to stay ahead in a competitive market.
Personalized Shopping Experiences
Personalization is crucial in today’s e-commerce environment. ML algorithms analyze user data, such as browsing history and purchase behavior, to recommend products that align with individual preferences. This not only elevates the customer experience but also drives higher conversion rates. For example, platforms that leverage ML for personalization have seen significant boosts in sales, as users are more likely to purchase items that resonate with their interests.
Optimizing Inventory Management
Effective inventory management is critical for e-commerce success. ML-driven predictive analytics can forecast demand with high accuracy, helping businesses maintain optimal inventory levels. This minimizes the chances of overstocking or running out of products, ensuring timely availability for customers. E-commerce giants like Amazon have successfully implemented ML to streamline their inventory management processes, setting a benchmark for others to follow.
Dynamic Pricing Strategies
Price is a major factor influencing consumer decisions. Machine Learning enables real-time dynamic pricing by assessing market trends, competitor rates, and customer demand. This allows businesses to adjust their prices to maximize revenue while remaining competitive. Dynamic pricing, powered by ML, has proven effective in attracting price-sensitive customers and increasing overall profitability.
Enhanced Customer Support
Customer support is another area where ML shines. AI-powered chatbots and virtual assistants can handle a large volume of customer inquiries, providing instant responses and resolving issues efficiently. This not only improves customer satisfaction but also reduces the operational costs associated with maintaining a large support team. E-commerce businesses in Saudi Arabia can greatly benefit from incorporating ML into their customer service strategies.
Fraud Detection and Security
With the rise of online transactions, ensuring the security of customer data and payments is paramount. ML algorithms can detect fraudulent activities by analyzing transaction patterns and identifying anomalies. By implementing ML-driven security measures, e-commerce businesses can protect their customers and build trust, which is essential for long-term success.
Improving Marketing Campaigns
Effective marketing is key to driving e-commerce success. ML can analyze customer data to create targeted marketing campaigns that resonate with specific audiences. It enhances the impact of marketing efforts, leading to improved customer engagement and higher conversion rates. Successful e-commerce platforms use ML to fine-tune their marketing strategies, ensuring that their messages reach the right people at the right time.
Case Study: Successful E-commerce Companies in Saudi Arabia Using ML
Several e-commerce companies in Saudi Arabia have already begun leveraging ML to drive growth. For example, Noon uses ML to personalize the shopping experience and optimize its supply chain, leading to increased customer satisfaction and operational efficiency. These companies serve as examples of how ML can be a game-changer in the competitive e-commerce market.
Challenges of Implementing Machine Learning in E-commerce
While the benefits of ML are clear, implementing this technology in e-commerce is not without challenges. Technical hurdles, such as integrating ML with existing systems, can be daunting. Additionally, there are concerns about data privacy, particularly in handling sensitive customer information. Businesses must address these challenges to fully harness the power of ML.
Future Trends in Machine Learning and E-commerce
As ML continues to evolve, new trends are emerging that will shape the future of e-commerce. For instance, the integration of ML with augmented reality (AR) offers exciting possibilities, such as virtual try-ons for products. Businesses that stay ahead of these trends will be well-positioned to lead the market in the coming years.
Influence of Machine Learning on Consumer Behavior in Saudi Arabia
ML is already influencing consumer behavior in Saudi Arabia, with personalized experiences leading to increased customer loyalty. As more businesses adopt ML, consumers can expect even more tailored shopping experiences, further enhancing their satisfaction and engagement.
Government Support and Regulations
The Saudi government is proactively encouraging the integration of cutting-edge technologies, including ML, within the e-commerce industry. Through initiatives like Vision 2030, the government aims to transform the Kingdom into a global tech hub. However, businesses must also navigate regulations related to data privacy and AI to ensure compliance.
Conclusion
Machine Learning is revolutionizing e-commerce in Saudi Arabia, offering businesses new ways to enhance user experiences, optimize operations, and drive growth. By embracing ML, e-commerce companies can not only stay competitive but also set new standards in the industry. The future of e-commerce in Saudi Arabia is bright, and Machine Learning will undoubtedly play a pivotal role in shaping its success.
FAQs
How does Machine Learning contribute to the e-commerce sector? Machine Learning enhances e-commerce by improving personalization, optimizing inventory, enabling dynamic pricing, and enhancing security.
How can Machine Learning improve customer experiences in e-commerce? ML analyzes user data to provide personalized recommendations, faster customer support, and tailored marketing campaigns, improving overall satisfaction.
What are the challenges of integrating ML in e-commerce? Challenges include technical integration, data privacy concerns, and the need for skilled professionals to manage ML systems effectively.
Which Saudi e-commerce companies are successfully using ML? Companies like Noon and Souq are leveraging ML for personalized shopping experiences, inventory management, and customer support.
What is the future of e-commerce with ML in Saudi Arabia? The future looks promising with trends like ML-driven AR experiences and more personalized
#machine learning e-commerce#Saudi Arabia tech#ML-powered apps#e-commerce growth#AI in retail#customer experience Saudi Arabia#digital transformation Saudi#ML app benefits#AI-driven marketing#predictive analytics retail#Saudi digital economy#e-commerce innovation#smart retail solutions#AI tech adoption#machine learning in business
2 notes
·
View notes
Text
𝗥𝗲𝘃𝗼𝗹𝘂𝘁𝗶𝗼𝗻𝗶𝘇𝗲 𝗬𝗼𝘂𝗿 𝗠𝗮𝗻𝘂𝗳𝗮𝗰𝘁𝘂𝗿𝗶𝗻𝗴 𝘄𝗶𝘁𝗵 𝗔𝗜-𝗱𝗿𝗶𝘃𝗲𝗻 𝗘𝗥𝗣 𝗦𝗼𝗹𝘂𝘁𝗶𝗼𝗻𝘀!
Discover how integrating Artificial Intelligence with Enterprise Resource Planning can transform your manufacturing operations. Check out our latest blog post to explore the future of smart manufacturing:
Read more about the next wave of intelligent ERP systems and stay ahead in the digital transformation race!
https://www.codetrade.io/blog/ai-driven-erp-solutions-for-the-manufacturing-industry/
#artificial intelligence#machine learning#erp solution#manufacturing industry#ERP in AI#enterprise resource planning#intelligent ERP system#AI ML#AI-driven ERP solutions
2 notes
·
View notes
Text
85% of Australian e-commerce content found to be plagiarised
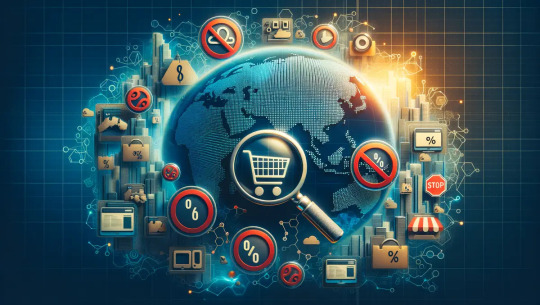
Optidan Published a Report Recently
OptiDan, an Australia-based specialist in AI-driven SEO strategies & Solutions, has recently published a report offering fresh insights into the Australian e-commerce sector. It reveals a striking statistic about content across more than 780 online retailers: 85% of it is plagiarised. This raises severe questions about authenticity and quality in the e-commerce world, with possibly grave implications for both consumers and retailers.
Coming from the founders of OptiDan, this report illuminates an issue that has largely fallen under the radar: content duplication. The report indicates that suppliers often supply identical product descriptions to several retailers, resulting in a sea of online stores harbouring the same content. This lack of uniqueness unfortunately leads to many sites being pushed down in search engine rankings, due to algorithms detecting the duplication. This results in retailers having to spend more on visibility through paid advertising to compensate.
Key Findings in Analysis
Key findings from OptiDan's research include a worrying lack of originality, with 86% of product pages not even meeting basic word count standards. Moreover, even among those that do feature sufficient word counts, Plagiarism is distressingly widespread. Notably, OptiDan's study presented clear evidence of the detrimental impacts of poor product content on consumer trust and return rates.
Founder and former retailer JP Tucker notes, "Online retailers anticipate high product ranking by Google and expect sales without investing in necessary, quality content — an essential for both criteria." Research from 2016 by Shotfarm corroborates these findings, suggesting that 40% of customers return online purchases due to poor product content.
Tucker's industry report reveals that Google usually accepts up to 10% of plagiarism to allow for the use of common terms. Nonetheless, OptiDan's study discovered that over 85% of audited product pages were above this limit. Further, over half of the product pages evidenced plagiarism levels of over 75%.
"Whilst I knew the problem was there, the high levels produced in the Industry report surprised me," said Tucker, expressing the depth of the issue. He's also noted the manufactured absence of the product title in the product description, a crucial aspect of SEO, in 85% of their audited pages. "Just because it reads well, doesn't mean it indexes well."
OptiDan has committed itself to transforming content performance for the online retail sector, aiming to make each brand's content work for them, instead of against them. Tucker guarantees the effectiveness of OptiDan's revolutionary approach: "We specialise in transforming E-commerce SEO content within the first month, paving the way for ongoing optimisation and reindexing performance."
OptiDan has even put a money-back guarantee on its Full Content Optimisation Service for Shopify & Shopify Plus partners. This offer is expected to extend to non-Shopify customers soon. For now, all retailers can utilise a free website audit of their content through OptiDan.
Optidan – Top AI SEO Agency
Optidan is a Trusted AI SEO services Provider Company from Sydney, Australia. Our Services like - Bulk Content Creation SEO, Plagiarism Detection SEO, AI-based SEO, Machine Learning AI, Robotic SEO Automation, and Semantic SEO
We’re not just a service provider; we’re a partner, a collaborator, and a fellow traveller on this exciting digital journey. Together, let’s explore the limitless possibilities and redefine digital success.
Intrigued to learn more? Let’s connect! Schedule a demo call with us and discover how OptiDan can transform your digital performance.
Reference link – Here Click
#Shopify SEO consultant#E-commerce SEO solutions#Shopify integration services#AI technology for efficient SEO#SEO content creation services#High-volume content writing#Plagiarism removal services#AI-driven SEO strategies#Rapid SEO results services#Automated SEO solutions#Best Shopify SEO strategies for retailers
3 notes
·
View notes
Text

Explore how Amazon Bedrock is set to revolutionize industries in 2024 with AI-driven solutions for finance, healthcare, retail, and more. Learn how seamless data integration and dynamic models can boost efficiency, innovation, and customer focus. Unlock your business's potential with Amazon Bedrock.
1 note
·
View note
Text
In the later years of the manufacturing industry, there has been a significant change due to incorporation of artificial intelligence.
#AI Integration In Manufacturing#AI-Driven Design Tools#AI-Driven Solutions#Generative AI In Manufacturing#Generative AI Models#Product Design And Development#Saas Professionals In The USA#Saas Providers
0 notes
Text
In the later years of the manufacturing industry, there has been a significant change due to incorporation of artificial intelligence.
#AI Integration In Manufacturing#AI-Driven Design Tools#AI-Driven Solutions#Generative AI In Manufacturing#Generative AI Models#Product Design And Development#Saas Professionals In The USA#Saas Providers
0 notes