#AI-driven chatbots
Explore tagged Tumblr posts
Text
As Melhores IAs de Conversação com Fala Gratuitas
Introdução às IAs de Conversação com Fala Nos últimos anos, as IAs de conversação com fala têm ganhado destaque em diversas áreas, desde assistentes pessoais até chatbots empresariais, passando por sistemas de automação doméstica. Esses sistemas utilizam tecnologias avançadas de reconhecimento de fala, processamento de linguagem natural (NLP) e síntese de fala (Text-to-Speech) para permitir uma…
#AI chatbots#AI for customer service#AI-driven chatbots#Conversational AI#Generative AI#ia#Inteligencia Artificial#Interactive voice response (IVR)#Machine learning (ML) for AI#Natural language processing (NLP)#Speech recognition#Speech-to-text API#Text-to-speech (TTS)#Virtual assistants#Voice assistants#Voice interfaces#Voice recognition
0 notes
Text
Supercharge Your Content Strategy with AI Technology
Overcoming Challenges in AI Adoption In the rapidly evolving landscape of technology, the adoption of Artificial Intelligence (AI) has become a crucial aspect for businesses looking to stay competitive and innovative. However, this adoption is not without its challenges. In this blog section, we will delve into two key challenges faced by organizations in the process of integrating AI into their…
#A/B testing#AI algorithms#AI success stories#AI technology#AI-driven chatbots#AI-Driven Content Creation Process#AI-powered chatbots#AI-powered tools#analyze data#analyzed#anticipate consumer needs#Articoolo#Artificial Intelligence#automated content generation techniques#Benefits of AI-Powered Content Marketing Tools#brand loyalty#Chatbots and Customer Service#competitive edge#connecting with consumers#content creation#content optimization#content optimization tools#content produced#content strategies#continuous refinement#customer experience#data-driven content#data-driven content strategies#distributed content#drive better results
0 notes
Text
#AI#AI Algorithms#AI-driven Chatbots#AI-Driven Interviews#AI-Optimized Resumes#Artificial Intelligence#chatbot#ChatGPT#ChatGPT as Job Search Tool#Company Research#Cover Letter Assistance#Enhanced Job Search#Future of AI in Job Search#Industry Insights#interview preparation#job hunting#Job Matching#Job search#job search related blogs#jobsbuster#openai#Resume Assistance#Technology#UK jobs
0 notes
Text
Revolutionizing Conversations: The Indispensable Role of AI-Driven Chatbots
In today's dynamic digital landscape, AI-driven chatbots have emerged as indispensable tools, transforming the way businesses engage with their audience. This title delves into the pivotal role played by these intelligent virtual assistants, elucidating their impact on customer interactions and operational efficiency. Discover how AI-driven chatbots streamline communication processes, provide instant support, and enhance user experiences across various industries. From automating routine queries to delivering personalized responses, these smart chatbots are revolutionizing the customer service paradigm. Stay abreast of the technological forefront and unlock the potential of AI-driven chatbots to elevate customer satisfaction and optimize business operations. Embrace the future of conversational AI for a seamless and efficient connection with your audience.
0 notes
Text
Top 7 Trends in Salesforce to Watch in 2023
Salesforce has been a trailblazer in the world of customer relationship management (CRM) software for years, helping businesses connect with customers, streamline processes, and drive growth. As we step into 2023, the platform continues to evolve, introducing new features and capabilities to meet the ever-changing demands of businesses and consumers alike. In this blog, we'll explore the top seven trends in Salesforce that are shaping the CRM landscape in 2023 and beyond.
AI-Driven Personalization:
Artificial intelligence (AI) is revolutionizing the way businesses engage with their customers, and Salesforce is no exception. In 2023, expect to see AI-driven personalization take center stage, allowing companies to tailor customer interactions based on their preferences, behavior, and historical data. This will not only enhance customer satisfaction but also boost conversion rates and drive customer loyalty.
Integration of IoT and Salesforce:
The Internet of Things (IoT) is reshaping various industries, and Salesforce is embracing this trend by integrating IoT data into its CRM platform. This integration will enable businesses to gather real-time insights from connected devices, optimize operations, and provide proactive customer support. Expect to see IoT-enabled Salesforce solutions gaining traction across industries such as manufacturing, healthcare, and retail.
Blockchain for Secure Data Management:
Data security and privacy are paramount concerns for businesses and consumers alike. In 2023, Salesforce is likely to incorporate blockchain technology to enhance data integrity and security. Blockchain can provide a tamper-proof, decentralized data storage mechanism, ensuring sensitive customer information remains safe from cyber threats and unauthorized access.
Hyper-Personalization with Customer Data Platforms (CDPs):
Customer Data Platforms (CDPs) are becoming increasingly popular as they consolidate customer data from various sources into a unified profile. Salesforce is expected to leverage CDPs to achieve hyper-personalization in 2023. By understanding customer behavior across channels, businesses can deliver highly targeted and relevant marketing campaigns, fostering deeper customer connections.
Low-Code/No-Code Development:
In 2023, we will witness an acceleration in low-code/no-code development on the Salesforce platform. These user-friendly tools enable businesses to build custom applications and automate processes without extensive coding knowledge. This democratization of development will empower organizations to innovate and respond quickly to changing market demands.
Extended Reality (XR) for Enhanced Customer Experiences:
Extended Reality (XR), which includes Virtual Reality (VR) and Augmented Reality (AR), is gaining traction in the CRM space. Salesforce is likely to integrate XR capabilities to offer unique and immersive customer experiences. From virtual product demonstrations to AR-powered customer support, XR will redefine how businesses engage with their audiences.
Voice Technology Integration:
As voice-activated devices become increasingly popular, Salesforce is expected to integrate voice technology into its CRM platform. This integration will simplify user interactions, making it easier for sales representatives to access information on-the-go, analyze data through voice commands, and improve overall productivity.
Conclusion:
Salesforce continues to be at the forefront of CRM innovation, and 2023 promises to be an exciting year with these emerging trends. AI-driven personalization, IoT integration, blockchain-enabled security, and hyper-personalization through CDPs are all set to revolutionize customer engagement. Furthermore, low-code/no-code development, XR integration, and voice technology will shape the future of the Salesforce platform, making it more accessible, immersive, and efficient for businesses worldwide.
As these trends continue to evolve, businesses must stay agile and adapt their strategies to leverage the full potential of Salesforce and gain a competitive edge in the dynamic world of CRM. Embracing these trends will not only enhance customer experiences but also drive revenue growth and long-term success.
#Salesforce Trends#Customer-Centric Approach#AI and Automation#Data-driven Insights#Augmented Reality (AR) in CRM#Integration and Connectivity#IoT (Internet of Things) and CRM#Omni-channel Engagement#Blockchain in Salesforce#Sales and Marketing Alignment#Virtual Events and Conferences#AI-driven Chatbots#Cloud Computing Advancements
0 notes
Text
AI’s Role in Business Process Automation
Automation has come a long way from simply replacing manual tasks with machines. With AI stepping into the scene, business process automation is no longer just about cutting costs or speeding up workflows—it’s about making smarter, more adaptive decisions that continuously evolve. AI isn't just doing what we tell it; it’s learning, predicting, and innovating in ways that redefine how businesses operate.
From hyperautomation to AI-powered chatbots and intelligent document processing, the world of automation is rapidly expanding. But what does the future hold?
What is Business Process Automation?
Business Process Automation (BPA) refers to the use of technology to streamline and automate repetitive, rule-based tasks within an organization. The goal is to improve efficiency, reduce errors, cut costs, and free up human workers for higher-value activities. BPA covers a wide range of functions, from automating simple data entry tasks to orchestrating complex workflows across multiple departments.
Traditional BPA solutions rely on predefined rules and scripts to automate tasks such as invoicing, payroll processing, customer service inquiries, and supply chain management. However, as businesses deal with increasing amounts of data and more complex decision-making requirements, AI is playing an increasingly critical role in enhancing BPA capabilities.
AI’s Role in Business Process Automation
AI is revolutionizing business process automation by introducing cognitive capabilities that allow systems to learn, adapt, and make intelligent decisions. Unlike traditional automation, which follows a strict set of rules, AI-driven BPA leverages machine learning, natural language processing (NLP), and computer vision to understand patterns, process unstructured data, and provide predictive insights.
Here are some of the key ways AI is enhancing BPA:
Self-Learning Systems: AI-powered BPA can analyze past workflows and optimize them dynamically without human intervention.
Advanced Data Processing: AI-driven tools can extract information from documents, emails, and customer interactions, enabling businesses to process data faster and more accurately.
Predictive Analytics: AI helps businesses forecast trends, detect anomalies, and make proactive decisions based on real-time insights.
Enhanced Customer Interactions: AI-powered chatbots and virtual assistants provide 24/7 support, improving customer service efficiency and satisfaction.
Automation of Complex Workflows: AI enables the automation of multi-step, decision-heavy processes, such as fraud detection, regulatory compliance, and personalized marketing campaigns.
As organizations seek more efficient ways to handle increasing data volumes and complex processes, AI-driven BPA is becoming a strategic priority. The ability of AI to analyze patterns, predict outcomes, and make intelligent decisions is transforming industries such as finance, healthcare, retail, and manufacturing.
“At the leading edge of automation, AI transforms routine workflows into smart, adaptive systems that think ahead. It’s not about merely accelerating tasks—it’s about creating an evolving framework that continuously optimizes operations for future challenges.”
— Emma Reynolds, CTO of QuantumOps
Trends in AI-Driven Business Process Automation
1. Hyperautomation
Hyperautomation, a term coined by Gartner, refers to the combination of AI, robotic process automation (RPA), and other advanced technologies to automate as many business processes as possible. By leveraging AI-powered bots and predictive analytics, companies can automate end-to-end processes, reducing operational costs and improving decision-making.
Hyperautomation enables organizations to move beyond simple task automation to more complex workflows, incorporating AI-driven insights to optimize efficiency continuously. This trend is expected to accelerate as businesses adopt AI-first strategies to stay competitive.
2. AI-Powered Chatbots and Virtual Assistants
Chatbots and virtual assistants are becoming increasingly sophisticated, enabling seamless interactions with customers and employees. AI-driven conversational interfaces are revolutionizing customer service, HR operations, and IT support by providing real-time assistance, answering queries, and resolving issues without human intervention.
The integration of AI with natural language processing (NLP) and sentiment analysis allows chatbots to understand context, emotions, and intent, providing more personalized responses. Future advancements in AI will enhance their capabilities, making them more intuitive and capable of handling complex tasks.
3. Process Mining and AI-Driven Insights
Process mining leverages AI to analyze business workflows, identify bottlenecks, and suggest improvements. By collecting data from enterprise systems, AI can provide actionable insights into process inefficiencies, allowing companies to optimize operations dynamically.
AI-powered process mining tools help businesses understand workflow deviations, uncover hidden inefficiencies, and implement data-driven solutions. This trend is expected to grow as organizations seek more visibility and control over their automated processes.
4. AI and Predictive Analytics for Decision-Making
AI-driven predictive analytics plays a crucial role in business process automation by forecasting trends, detecting anomalies, and making data-backed decisions. Companies are increasingly using AI to analyze customer behaviour, market trends, and operational risks, enabling them to make proactive decisions.
For example, in supply chain management, AI can predict demand fluctuations, optimize inventory levels, and prevent disruptions. In finance, AI-powered fraud detection systems analyze transaction patterns in real-time to prevent fraudulent activities. The future of BPA will heavily rely on AI-driven predictive capabilities to drive smarter business decisions.
5. AI-Enabled Document Processing and Intelligent OCR
Document-heavy industries such as legal, healthcare, and banking are benefiting from AI-powered Optical Character Recognition (OCR) and document processing solutions. AI can extract, classify, and process unstructured data from invoices, contracts, and forms, reducing manual effort and improving accuracy.
Intelligent document processing (IDP) combines AI, machine learning, and NLP to understand the context of documents, automate data entry, and integrate with existing enterprise systems. As AI models continue to improve, document processing automation will become more accurate and efficient.
Going Beyond Automation
The future of AI-driven BPA will go beyond automation—it will redefine how businesses function at their core. Here are some key predictions for the next decade:
Autonomous Decision-Making: AI systems will move beyond assisting human decisions to making autonomous decisions in areas such as finance, supply chain logistics, and healthcare management.
AI-Driven Creativity: AI will not just automate processes but also assist in creative and strategic business decisions, helping companies design products, create marketing strategies, and personalize customer experiences.
Human-AI Collaboration: AI will become an integral part of the workforce, working alongside employees as an intelligent assistant, boosting productivity and innovation.
Decentralized AI Systems: AI will become more distributed, with businesses using edge AI and blockchain-based automation to improve security, efficiency, and transparency in operations.
Industry-Specific AI Solutions: We will see more tailored AI automation solutions designed for specific industries, such as AI-driven legal research tools, medical diagnostics automation, and AI-powered financial advisory services.
AI is no longer a futuristic concept—it’s here, and it’s already transforming the way businesses operate. What’s exciting is that we’re still just scratching the surface. As AI continues to evolve, businesses will find new ways to automate, innovate, and create efficiencies that we can’t yet fully imagine.
But while AI is streamlining processes and making work more efficient, it’s also reshaping what it means to be human in the workplace. As automation takes over repetitive tasks, employees will have more opportunities to focus on creativity, strategy, and problem-solving. The future of AI in business process automation isn’t just about doing things faster—it’s about rethinking how we work all together.
Learn more about DataPeak:
#datapeak#factr#technology#agentic ai#saas#artificial intelligence#machine learning#ai#ai-driven business solutions#machine learning for workflow#ai solutions for data driven decision making#ai business tools#aiinnovation#digitaltools#digital technology#digital trends#dataanalytics#data driven decision making#data analytics#cloudmigration#cloudcomputing#cybersecurity#cloud computing#smbs#chatbots
2 notes
·
View notes
Text
youtube
#digital marketing#@desmondjohnson183#marketing strategy#DeepSeek AI#digital marketing AI#open-source AI#AI in marketing#AI-driven content creation#predictive marketing#AI chatbots#AI-powered advertising#voice search optimization#influencer marketing AI#ethical AI#data analytics#AI customer engagement#AI-powered SEO#future of digital marketing.#Youtube
3 notes
·
View notes
Text
#onlinemarketing#onlinemarketingtips#@desmondjohnson183#DeepSeek AI#digital marketing AI#open-source AI#AI in marketing#AI-driven content creation#predictive marketing#AI chatbots#AI-powered advertising#voice search optimization#influencer marketing AI
3 notes
·
View notes
Text
Scientists use generative AI to answer complex questions in physics
New Post has been published on https://thedigitalinsider.com/scientists-use-generative-ai-to-answer-complex-questions-in-physics/
Scientists use generative AI to answer complex questions in physics
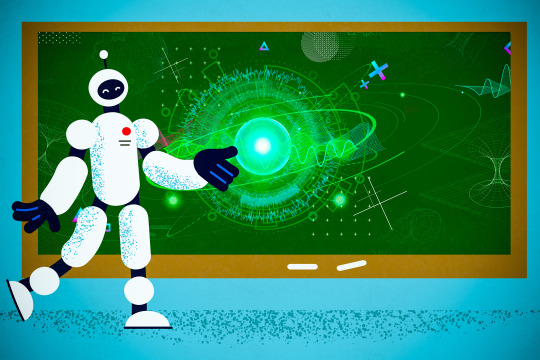
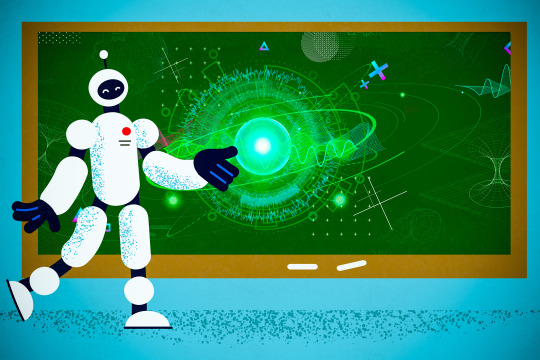
When water freezes, it transitions from a liquid phase to a solid phase, resulting in a drastic change in properties like density and volume. Phase transitions in water are so common most of us probably don’t even think about them, but phase transitions in novel materials or complex physical systems are an important area of study.
To fully understand these systems, scientists must be able to recognize phases and detect the transitions between. But how to quantify phase changes in an unknown system is often unclear, especially when data are scarce.
Researchers from MIT and the University of Basel in Switzerland applied generative artificial intelligence models to this problem, developing a new machine-learning framework that can automatically map out phase diagrams for novel physical systems.
Their physics-informed machine-learning approach is more efficient than laborious, manual techniques which rely on theoretical expertise. Importantly, because their approach leverages generative models, it does not require huge, labeled training datasets used in other machine-learning techniques.
Such a framework could help scientists investigate the thermodynamic properties of novel materials or detect entanglement in quantum systems, for instance. Ultimately, this technique could make it possible for scientists to discover unknown phases of matter autonomously.
“If you have a new system with fully unknown properties, how would you choose which observable quantity to study? The hope, at least with data-driven tools, is that you could scan large new systems in an automated way, and it will point you to important changes in the system. This might be a tool in the pipeline of automated scientific discovery of new, exotic properties of phases,” says Frank Schäfer, a postdoc in the Julia Lab in the Computer Science and Artificial Intelligence Laboratory (CSAIL) and co-author of a paper on this approach.
Joining Schäfer on the paper are first author Julian Arnold, a graduate student at the University of Basel; Alan Edelman, applied mathematics professor in the Department of Mathematics and leader of the Julia Lab; and senior author Christoph Bruder, professor in the Department of Physics at the University of Basel. The research is published today in Physical Review Letters.
Detecting phase transitions using AI
While water transitioning to ice might be among the most obvious examples of a phase change, more exotic phase changes, like when a material transitions from being a normal conductor to a superconductor, are of keen interest to scientists.
These transitions can be detected by identifying an “order parameter,” a quantity that is important and expected to change. For instance, water freezes and transitions to a solid phase (ice) when its temperature drops below 0 degrees Celsius. In this case, an appropriate order parameter could be defined in terms of the proportion of water molecules that are part of the crystalline lattice versus those that remain in a disordered state.
In the past, researchers have relied on physics expertise to build phase diagrams manually, drawing on theoretical understanding to know which order parameters are important. Not only is this tedious for complex systems, and perhaps impossible for unknown systems with new behaviors, but it also introduces human bias into the solution.
More recently, researchers have begun using machine learning to build discriminative classifiers that can solve this task by learning to classify a measurement statistic as coming from a particular phase of the physical system, the same way such models classify an image as a cat or dog.
The MIT researchers demonstrated how generative models can be used to solve this classification task much more efficiently, and in a physics-informed manner.
The Julia Programming Language, a popular language for scientific computing that is also used in MIT’s introductory linear algebra classes, offers many tools that make it invaluable for constructing such generative models, Schäfer adds.
Generative models, like those that underlie ChatGPT and Dall-E, typically work by estimating the probability distribution of some data, which they use to generate new data points that fit the distribution (such as new cat images that are similar to existing cat images).
However, when simulations of a physical system using tried-and-true scientific techniques are available, researchers get a model of its probability distribution for free. This distribution describes the measurement statistics of the physical system.
A more knowledgeable model
The MIT team’s insight is that this probability distribution also defines a generative model upon which a classifier can be constructed. They plug the generative model into standard statistical formulas to directly construct a classifier instead of learning it from samples, as was done with discriminative approaches.
“This is a really nice way of incorporating something you know about your physical system deep inside your machine-learning scheme. It goes far beyond just performing feature engineering on your data samples or simple inductive biases,” Schäfer says.
This generative classifier can determine what phase the system is in given some parameter, like temperature or pressure. And because the researchers directly approximate the probability distributions underlying measurements from the physical system, the classifier has system knowledge.
This enables their method to perform better than other machine-learning techniques. And because it can work automatically without the need for extensive training, their approach significantly enhances the computational efficiency of identifying phase transitions.
At the end of the day, similar to how one might ask ChatGPT to solve a math problem, the researchers can ask the generative classifier questions like “does this sample belong to phase I or phase II?” or “was this sample generated at high temperature or low temperature?”
Scientists could also use this approach to solve different binary classification tasks in physical systems, possibly to detect entanglement in quantum systems (Is the state entangled or not?) or determine whether theory A or B is best suited to solve a particular problem. They could also use this approach to better understand and improve large language models like ChatGPT by identifying how certain parameters should be tuned so the chatbot gives the best outputs.
In the future, the researchers also want to study theoretical guarantees regarding how many measurements they would need to effectively detect phase transitions and estimate the amount of computation that would require.
This work was funded, in part, by the Swiss National Science Foundation, the MIT-Switzerland Lockheed Martin Seed Fund, and MIT International Science and Technology Initiatives.
#ai#approach#artificial#Artificial Intelligence#Bias#binary#change#chatbot#chatGPT#classes#computation#computer#Computer modeling#Computer Science#Computer Science and Artificial Intelligence Laboratory (CSAIL)#Computer science and technology#computing#crystalline#dall-e#data#data-driven#datasets#dog#efficiency#Electrical Engineering&Computer Science (eecs)#engineering#Foundation#framework#Future#generative
2 notes
·
View notes
Text
Unlocking Digital Success: Your Guide to Web & App Development in Kerala

In today's hyper-connected world, a robust online presence is non-negotiable for any business aiming to thrive. From stunning websites to intuitive mobile applications and intelligent AI solutions, the right digital partner can make all the difference. If you're in Kerala, particularly in Trivandrum, you're in luck – the region is a hub for innovative and skilled web development agencies.
When it comes to building dynamic and comprehensive online platforms, a best full stack web development agency in Trivandrum, Kerala is your go-to. Full-stack developers possess expertise across both front-end (what users see) and back-end (the server, database, and application logic) development. This holistic approach ensures seamless functionality, scalability, and a truly integrated digital solution for your business. Agencies in Trivandrum are known for their technical prowess in languages and frameworks like React, Angular, Node.js, Python, and more, delivering everything from custom web applications to enterprise-level software.
For businesses looking to establish or expand their online retail footprint, eCommerce web development services in Kerala are essential. The burgeoning digital marketplace demands expertly crafted online stores that are not only visually appealing but also highly secure, user-friendly, and optimized for conversions. Leading agencies in Kerala specialize in platforms like Shopify, WooCommerce, and Magento, creating bespoke eCommerce solutions that cater to diverse business models, from B2C to B2B marketplaces. They understand the nuances of payment gateway integration, inventory management, and seamless checkout processes, ensuring a smooth shopping experience for your customers.
Taking your online store a step further, the best eCommerce app web development services in Kerala provide native or hybrid mobile applications that offer unparalleled convenience and engagement. With a dedicated app, businesses can leverage push notifications, offline access, and device-specific features to enhance customer loyalty and drive repeat purchases. These agencies combine stunning UI/UX design with robust backend development to deliver high-performing mobile commerce solutions that truly stand out in a crowded app market.
Finally, to truly elevate customer interaction and streamline operations, consider partnering with an AI-driven chatbot development company in Trivandrum. AI chatbots are no longer just simple FAQ responders; they are intelligent virtual assistants capable of personalized conversations, lead qualification, 24/7 customer support, and even complex task automation. Companies in Trivandrum are at the forefront of leveraging Natural Language Processing (NLP) and machine learning to build custom chatbots that understand user intent, provide instant solutions, and free up human resources for more critical tasks.
In conclusion, whether you're seeking a comprehensive full-stack solution, a powerful eCommerce platform, a compelling mobile app, or cutting-edge AI chatbot integration, Kerala, and particularly Trivandrum, offers a wealth of talented agencies ready to transform your digital vision into a tangible success. Partnering with the right experts ensures that your business is not just online, but truly thriving in the digital age.
0 notes
Text
Discover how generative AI creates personalized e-commerce journeys that keep shoppers clicking, buying, and coming back for more.
#AI-Driven Customer Engagement#AI-Powered Email Platforms#Chatbots for E-Commerce#Generative AI#Generative AI for E-Commerce
0 notes
Text
Top AI Features Powering Next-Gen Contact Centers

Introduction
The evolution of contact centers from traditional call hubs to intelligent customer engagement platforms is being driven by artificial intelligence (AI). In a hyper-connected world where customers expect fast, personalized, and efficient service, AI is playing a transformative role. From automating routine tasks to offering real-time analytics and sentiment analysis, AI is redefining the standards of customer support. Modern contact centers, powered by AI, are becoming more responsive, proactive, and insightful—enhancing both customer satisfaction and operational efficiency.
This article explores the top AI features that are revolutionizing next-generation contact centers and how they are helping businesses stay competitive in today’s digital landscape.
1. AI-Powered Chatbots and Virtual Assistants
Perhaps the most visible AI application in contact centers is the use of chatbots and virtual assistants. These tools are capable of handling thousands of customer queries simultaneously across various platforms, including websites, mobile apps, and social media.
Key Benefits:
24/7 availability
Immediate responses to FAQs
Reduced workload for human agents
Seamless integration with CRM systems
Advanced AI chatbots use Natural Language Processing (NLP) and Machine Learning (ML) to understand customer queries better and improve over time. They also support multilingual interactions, expanding a business’s global reach.
2. Intelligent Call Routing
Traditional call routing systems use basic algorithms like round-robin or skill-based routing. AI takes this to the next level with predictive routing, which uses historical data and real-time analytics to match customers with the most suitable agents.
Example: If a customer previously had a billing issue and rated a certain agent highly, AI can route future related calls directly to that agent, ensuring a personalized experience.
Benefits:
Enhanced customer satisfaction
Reduced average handling time
Better utilization of agent expertise
3. Speech and Sentiment Analysis
AI-driven sentiment analysis tools assess the tone, pitch, and language of customer conversations in real-time. This allows agents to adapt their approach based on the emotional state of the caller.
Key Capabilities:
Detect frustration or satisfaction
Real-time alerts for supervisors
Contextual response suggestions for agents
This not only helps in de-escalating potential conflicts but also contributes to training and performance reviews.
4. Real-Time Agent Assistance
AI can provide live suggestions, answers, and prompts to agents during customer interactions. Known as Agent Assist or Co-Pilot systems, these features boost agent efficiency and reduce error rates.
Use Cases:
Auto-suggesting answers based on past tickets or knowledge base
Providing legal or compliance language for regulated industries
Offering upsell/cross-sell suggestions during the call
This enables even less-experienced agents to perform like experts, thereby maintaining service consistency.
5. Predictive and Prescriptive Analytics
Modern AI systems can analyze historical customer data to predict future behaviors and offer prescriptive actions. For example, AI can forecast customer churn and suggest personalized retention strategies.
Key Features:
Trend identification
Churn prediction
Customer lifetime value estimation
Product recommendation modeling
These analytics turn contact centers from reactive to proactive units that can anticipate customer needs and take preventive measures.
6. Automated Quality Monitoring
Quality assurance (QA) in traditional contact centers involves manual listening to a random sample of calls. AI changes this by automatically analyzing 100% of customer interactions for compliance, tone, and performance metrics.
Advantages:
Scalable and unbiased QA process
Immediate feedback loops
Identification of training opportunities
This ensures consistent service quality and helps businesses remain compliant with industry standards and regulations.
7. AI-Driven Self-Service
Customers increasingly prefer solving issues on their own. AI enables robust self-service solutions through intelligent FAQs, voice assistants, and dynamic help centers.
Core Components:
AI-curated knowledge bases
Interactive voice response (IVR) systems
Visual IVRs with dynamic menus based on customer behavior
These systems can deflect a significant volume of queries, saving time and reducing contact center costs.
8. Workforce Optimization (WFO)
AI enhances workforce optimization by analyzing call volumes, customer demand patterns, and agent performance to create optimized schedules and workloads.
Capabilities Include:
Forecasting peak interaction times
Automating shift scheduling
Identifying training needs through performance data
This ensures that the right number of agents with the right skills are available at the right time.
9. Multilingual Support
With global customer bases, multilingual support is essential. AI translation engines powered by NLP enable real-time language translation, allowing agents to assist customers in multiple languages.
Benefits:
Expanded market reach
Consistent support quality
Reduced need for native-speaking agents
Advanced systems even recognize regional dialects and slang, further enhancing communication accuracy.
10. Omnichannel AI Integration
Today’s customers expect consistent service across phone, email, chat, social media, and more. AI enables omnichannel support by centralizing data and ensuring continuity in customer interactions.
Features Include:
Unified customer profiles
Context-aware responses
Seamless channel transitions (e.g., chat to call)
This creates a cohesive customer experience and provides agents with the full context of past interactions, reducing redundancy and frustration.
Conclusion
AI is not just an enhancement to traditional contact center operations—it is a fundamental driver of their transformation. From handling repetitive tasks to offering deep insights into customer behavior, AI is redefining what’s possible in customer service.
By leveraging AI-powered features like chatbots, intelligent routing, sentiment analysis, and predictive analytics, next-generation contact centers are achieving higher efficiency, better customer satisfaction, and lower operational costs. The focus is shifting from handling calls to delivering experiences, and AI is at the heart of that shift.
Businesses that invest in AI capabilities today will be better positioned to adapt to the growing demands of tomorrow’s customers. As AI continues to evolve, contact centers will become smarter, faster, and more human than ever before—setting a new standard for customer engagement in the digital era.
#AI contact centers#AI in customer service#AI-powered chatbots#virtual assistants for support#intelligent call routing#real-time agent assistance#AI sentiment analysis#predictive analytics in contact centers#AI customer experience#automated quality monitoring#AI in workforce optimization#self-service solutions AI#omnichannel customer support AI#speech analytics in call centers#AI call center solutions#AI customer engagement tools#AI-driven customer insights#machine learning in contact centers#AI customer service automation
0 notes
Text
Discover how generative AI creates personalized e-commerce journeys that keep shoppers clicking, buying, and coming back for more.
#AI-Driven Customer Engagement#AI-Powered Email Platforms#Chatbots for E-Commerce#Generative AI#Generative AI for E-Commerce
0 notes
Text
Revolutionize Dining with AI-Based Food Ordering Chatbots
The integration of an ai based food ordering chatbot marks a significant advancement in the restaurant industry, offering a seamless blend of efficiency and personalization. This innovative technology streamlines the ordering process by utilizing artificial intelligence to interact with customers in real-time. Through natural language processing, these chatbots can understand and respond to customer inquiries, making the ordering experience not only faster but also more engaging and intuitive.
An ai-enabled ordering simplifies menu navigation by providing tailored recommendations to users based on their preferences and past orders. This enhanced personalization not only increases customer satisfaction but also encourages higher sales as patrons are more likely to explore and purchase suggested items. By automating routine inquiries and interactions, the chatbot allows restaurant staff to focus on delivering exceptional in-house dining experiences, improving overall service quality.
Moreover, these AI-driven systems reduce wait times and potential errors associated with manual order-taking. Customers can place their orders through messaging apps or directly on a restaurant's website, enjoying a frictionless experience from anywhere, at any time. This convenience is especially valuable for takeout and delivery services, where efficiency and accuracy are paramount.
The integration of chatbots with existing POS systems ensures a cohesive operational flow. Orders are seamlessly transmitted to the kitchen, where staff can prepare meals with precision based on accurate, real-time information. This integration minimizes delays and enhances communication between front-of-house and back-of-house operations, resulting in a smoother service experience.
Beyond improving operational efficiency, an AI-based food ordering provides detailed analytics that offer insights into customer preferences and behavior. Restaurants can leverage this data to refine their menu offerings, optimize pricing strategies, and tailor marketing efforts to individual customer segments. This data-driven approach enhances both revenue and customer loyalty.
Security and privacy are fundamental to the chatbot experience, with advanced algorithms safeguarding customer data throughout the ordering process. This trust ensures that patrons feel confident in their transactions, which is essential for building long-term relationships.
For restaurants aiming to innovate and adapt to modern consumer expectations, investing in an AI-based food ordering chatbot is a strategic step forward. By leveraging cutting-edge technology, you create a dining experience that is both efficient and personalized, setting your business apart in a competitive market.
If you're ready to see how an ai-enabled ordering system can transform your restaurant operations, schedule a demo. This demonstration will provide a firsthand look at the chatbot’s capabilities, showcasing how it can be customized to meet your unique business needs. Experience the potential of AI-driven technology to enhance your service delivery, optimize operations, and drive greater customer satisfaction and loyalty.
0 notes
Text
End-to-End Recruitment Automation with uKnowva HRMS
Hiring the right people at the right time is critical to business success — but it can be a slow, manual process without the right tools. From job posting and resume screening to onboarding, traditional recruitment methods often involve too many handoffs, spreadsheets, and delays. That’s why HR teams are turning to recruitment automation.
With an integrated platform like uKnowva HRMS, companies can streamline the entire hiring journey — saving time, improving candidate experience, and boosting hiring efficiency.
Why Automate Recruitment?
Manual recruitment processes come with several challenges:
Long time-to-hire
Inconsistent candidate communication
Repetitive administrative work
Human bias in screening
Disconnected systems and data
Automating recruitment addresses these pain points by bringing structure, speed, and scalability to hiring operations.
What End-to-End Recruitment Automation Looks Like with uKnowva HRMS
uKnowva HRMS covers the entire recruitment lifecycle in one platform. Here’s how it simplifies each stage:
Job Requisition and Posting Create job openings, define roles, and get them approved through customizable workflows. With integration options, you can publish listings on job boards or your careers page directly from the platform.
Resume Screening and Shortlisting Instead of sifting through hundreds of resumes manually, uKnowva uses intelligent filters to scan applications and surface top candidates based on pre-defined criteria.
Interview Scheduling Automated scheduling tools sync with calendars to find the best interview slots. Candidates and interviewers receive timely notifications and updates, reducing coordination hassles.
Candidate Communication Keep applicants informed with automated emails and chatbot-driven updates. Personalized messaging improves engagement and reduces drop-offs.
Collaboration and Feedback Recruiters, hiring managers, and interviewers can add notes, rate candidates, and track progress from one shared interface — ensuring transparent and collaborative hiring decisions.
Offer Management Generate offer letters with pre-set templates, get digital approvals, and track responses. uKnowva also allows conditional offers and contract automation.
Onboarding Integration Once a candidate accepts, the onboarding process kicks in automatically. New hires receive digital forms, task lists, and orientation materials — all within the same platform.
Key Benefits for HR Teams
Faster Time-to-Hire: Eliminate bottlenecks and reduce manual steps
Improved Candidate Experience: Timely updates and smooth transitions create a positive impression
Consistent Hiring Process: Automated workflows ensure fairness and compliance
Scalability: Handle more requisitions without increasing HR overhead
Why uKnowva HRMS Stands Out
While many tools offer fragmented solutions, uKnowva HRMS integrates recruitment with other HR functions — like onboarding, performance, and payroll. This ensures data consistency and a seamless handoff from hire to employee.
Its user-friendly interface, configurable workflows, and built-in analytics make it suitable for startups and growing enterprises alike. And because it’s cloud-based, the platform supports remote hiring effortlessly.
Conclusion
Recruitment automation isn’t just about reducing workload — it’s about making smarter, faster, and fairer hiring decisions. uKnowva HRMS empowers HR teams to take full control of the hiring process, from job posting to onboarding, all in one place.
If you’re looking to modernize your hiring strategy without adding complexity, end-to-end automation with uKnowva is a powerful way to get there.
#hrms software#hr services#hrms solutions#hr software#uknowva hrms#hr management#ai chatbot#ai driven recruitement#recruitment
0 notes
Text
Virtual Tutors and AI: The New Face of Modern Education
Education is changing—and not just because of new textbooks or digital classrooms. Behind the scenes, AI is quietly reshaping how we learn, teach, and interact with knowledge itself.
The days of the one-size-fits-all classroom are behind us. Thanks to AI, learning today is becoming more personal, more accessible, and more effective. It's not about replacing teachers—it's about empowering them, and making education a more human experience, not less.
Here’s how AI is transforming education:
Personalized Learning Paths AI algorithms analyze a student’s strengths, weaknesses, and pace. Based on that, they create tailor-made study plans—giving every learner the chance to shine on their own terms.
Virtual Tutors Stuck on a math problem at midnight? AI-driven tutors like chatbots and apps provide instant support, explanations, and practice, filling gaps when human help isn’t around.
Real-Time Feedback Instead of waiting for end-of-term report cards, AI tools give students immediate feedback—helping them course-correct and learn more efficiently.
Access to Quality Education Everywhere AI-powered platforms break down geographical barriers, offering top-quality education to students in remote areas, or those who otherwise wouldn’t have access.
Smarter Content Creation AI helps teachers by generating quizzes, lesson plans, and customized learning materials—giving educators more time to focus on what they do best: inspire.
Predictive Analytics for Early Intervention AI can spot when a student is struggling early on, allowing teachers and parents to step in with support before it’s too late.
AI isn’t just making education smarter—it’s making it more human by meeting students where they are. If done right, the future of learning looks brighter, fairer, and full of possibilities.
About US: AI Technology Insights (AITin) is the fastest-growing global community of thought leaders, influencers, and researchers specializing in AI, Big Data, Analytics, Robotics, Cloud Computing, and related technologies. Through its platform, AITin offers valuable insights from industry executives and pioneers who share their journeys, expertise, success stories, and strategies for building profitable, forward-thinking businesses.
Contact Us :
Call Us
+1 (520) 350-7212
Email Address
Local Address
1846 E Innovation Park DR Site 100 ORO Valley AZ 85755
0 notes