#AI in materials engineering
Explore tagged Tumblr posts
Text
Researchers at the Pritzker School of Molecular Engineering at the University of Chicago have created a tool that lets them represent collections of long, complex polymers in representations that can be easily processed by computers and artificial intelligence programs. This is an exciting step toward being able to streamline the process of new polymer development.
33 notes
·
View notes
Text
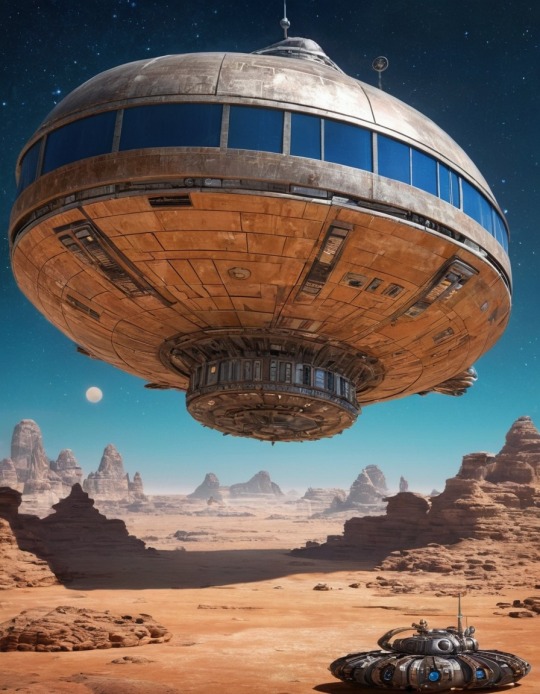
14 notes
·
View notes
Text
#digital art#future#artwork#technology#futurism#my art#graphic design#sports car racing#cars#car#cyberpunk aesthetic#cybernetics#cyberpunk#cyberpunk art#ai art#ai art gallery#ai artwork#factory#industrial#industries#mechanical#materials#engineering#product
4 notes
·
View notes
Text
What can AI do in materials science and engineering?
AI (Artificial Intelligence) plays a transformative role in materials science and engineering, offering a wide range of capabilities that enhance research, development, and application of materials.
AI intervenes in materials discovery and design, high-throughput screening and experimentation, materials characterization and analysis, materials modeling and simulation, materials performance optimization, materials lifecycle and sustainability, and collaborative platforms and knowledge sharing.
Read more:
#materials science#science#engineering#materials#materials science and engineering#AI#artificial intelligence#materials informatics#ai
9 notes
·
View notes
Text
Researchers leverage AI to unveil key insights into 2D halide perovskites for stable, efficient solar cells
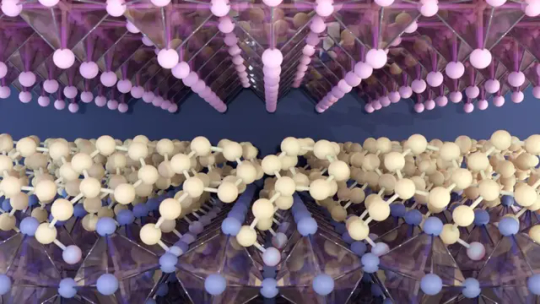
- By Nuadox Crew -
Researchers at Chalmers University of Technology in Sweden have used computer simulations and machine learning to better understand how 2D halide perovskite materials function, paving the way for more efficient and stable solar cells.
These perovskites, seen as promising alternatives to traditional silicon in solar energy and optical applications, are cost-effective and efficient in absorbing and emitting light. However, their tendency to degrade quickly has been a challenge.
By simulating the atomic behavior of 2D perovskites under different conditions, the research team gained detailed insights into how the materials respond to heat, light, and other factors.
Their findings highlight the role of organic molecules in controlling atomic movements within the material layers, which affect the material's optical properties and stability.
Header image: The illustration depicts the 2D perovskite material studied by the researchers, with the yellow sections representing the linker molecules and the purple and pink areas indicating the perovskite layer. Credit: Julia Wiktor, Chalmers University of Technology.
Read more at Chalmers University of Technology
Scientific paper: Impact of Organic Spacers and Dimensionality on Templating of Halide Perovskites Erik Fransson, Julia Wiktor, and Paul Erhart ACS Energy Letters 2024 9 (8), 3947-3954 DOI: 10.1021/acsenergylett.4c01283
Read Also
Off the roof: The quest to harness energy from facades
#ai#machine learning#solar panels#solar energy#engineering#perovskites#materials#energy#ecology#green energy
2 notes
·
View notes
Text
What is the Internet of Things (iot)?
The Internet of Things (IoT) is a network of physical devices, vehicles, home appliances, and other objects embedded with electronics, software, sensors, and connectivity. These devices can collect and exchange data using the internet. Imagine a world where your refrigerator orders groceries when it's running low, your thermostat adjusts the temperature based on your location, and your car can drive itself. This is the power of the internet of things (iot).
At WikiGlitz, we're passionate about exploring the endless possibilities of IoT. From smart homes to industrial automation, we'll keep you informed about the latest trends and innovations. Whether you're a tech enthusiast or a business professional, our content will help you understand the impact of IoT on your daily life and industry.
If you like to know more about IoT in the Automobile Industry: Driving Incredible Innovation then check out our in-depth article. Visit our official blog for the latest updates. Our Official Blog Site:https://wikiglitz.co/
#virtual reality#augmented reality#blockchain technology#blockchain#ai technology#technology#technology tips#chatgpt#gemini ai#cloud computing#machine learning#latest technology in artificial intelligence#future of artificial intelligence#renewable energy and sustainability#biotechnology and genetic engineering#quantum computing#virtual and augmented reality (vr/ar)#internet of things (iot)#blockchain and cryptocurrency#artificial intelligence#brain-computer interfaces#robotics#cybersecurity#big data and analytics#space exploration#materials science#nanotechnology#artificial general intelligence (agi)#brain-computer interfaces (bcis)
1 note
·
View note
Text
The Future of Construction is Here! Are You Ready? The construction industry is evolving faster than ever with cutting-edge technology! From AI-driven project management to 3D-printed buildings, the future is all about efficiency, sustainability, and cost-effectiveness. 🏗️💡 🔹 Key Innovations Shaping Construction: ✅ AI & Machine Learning – Automating workflows & reducing delays ✅ 3D Printing – Faster, cost-effective, and sustainable buildings ✅ Green Building Materials – Net-zero energy & eco-friendly solutions ✅ Modular Construction – Speedy, low-waste, and future-ready homes ✅ Digital Twins – Smart technology for better infrastructure planning 💬 What do you think is the biggest game-changer? Drop your thoughts below! 👇 Stay ahead of industry trends! Follow @TheCivilStudies for the latest updates. #ConstructionInnovation #AIinConstruction #3DPrinting #Sustainability #FutureOfConstruction #TheCivilStudies
#AI#Building Construction#Climate change#Construction material#Environmental Engineering#Future of Construction#Green Building#Green Construction#Green Tech#sustainable development
0 notes
Text
Artificial Intelligence for Developers in pune | India
Artificial intelligence developers are concentrating on developing algorithms that allow robots to emulate human intelligence. These people create artificial intelligence systems that are capable of sorting through massive amounts of data, identifying patterns, projecting results, and resolving challenging problems.
#Artificial Intelligence for Developers Pune#AI-Based solutions#Energy Management Centre with Cutting-Edge Solutions#Global Engineering Services Outsourcing Pune#Services India#Panel Design Electrical Pune#Eplan Electrical#Material handling systems
0 notes
Text
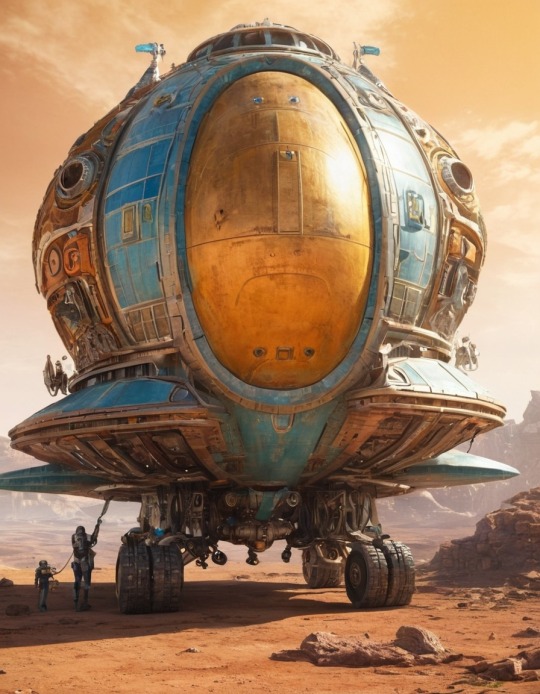
#sustainability#spaceship#digitalart#technology#aiart#science fiction#art#recycled materials#innovation#engineering#ai
0 notes
Text
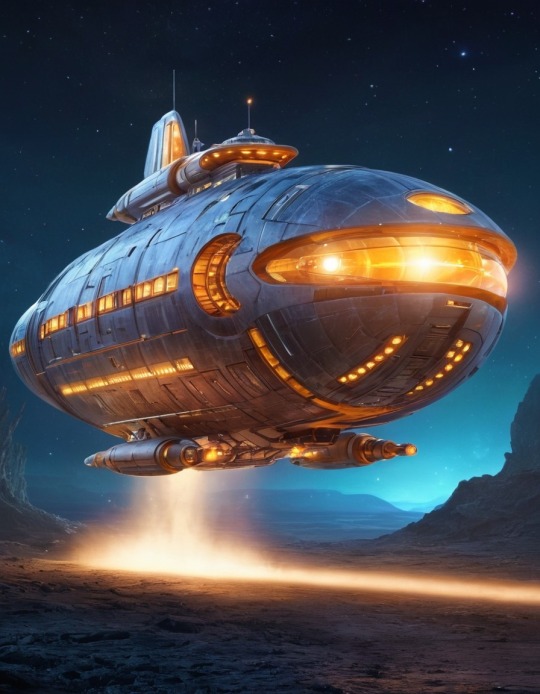
#art#innovation#sustainability#technology#science fiction#recycled materials#aiart#digitalart#engineering#spaceship#ai
0 notes
Text

#ai#spaceship#science fiction#innovation#sustainability#recycled materials#engineering#digitalart#aiart#technology#art
0 notes
Text
The Impact of AI-Generated Content
In recent years, the rapid advancement of artificial intelligence (AI) has transformed various industries, including content creation. AI algorithms are increasingly being used to generate written content for websites, blogs, and marketing materials. As AI-generated content becomes more prevalent, questions about transparency and ethics arise, leading us to ponder whether it should be labeled as such. In this article, we explore how Google treats AI content and the debate surrounding the labeling of AI-generated content.
Google's Stance on AI-Generated Content
Google, as the dominant search engine, plays a crucial role in shaping how online content is discovered and ranked. To understand its approach to AI-generated content, we must delve into its search algorithms and policies.
Google's algorithms are designed to assess the quality, relevance, and usefulness of web content. In doing so, they evaluate factors such as keywords, backlinks, user engagement, and more. The goal is to provide users with the most valuable and accurate information in response to their queries.
As AI-generated content continues to proliferate, Google's algorithms adapt to assess the quality of such content. Google has stated that it doesn't specifically penalize or favor AI-generated content. Instead, it evaluates it based on the same criteria as any other content. This means that AI-generated content can rank well in search results if it meets Google's quality guidelines.
Examine Google's official statements regarding content generated by artificial intelligence.
From Google Search Liaison Danny Sullivan’s November 2022 tweets:
“We haven’t said AI content is bad. We’ve said, pretty clearly, content written primarily for search engines rather than humans is the issue. That’s what we’re focused on.”
Gary Illyes’ statement on labeling AI-generated content, June 16, 2023:
“We do not label it as AI-generated content. Again, it’s not whether the AI wrote it, but whether it’s high quality.”
The Need for Transparency
While Google may not penalize AI-generated content, the question of labeling remains contentious. Many argue that transparency is essential, as it ensures that users are aware of the content's origin and can make informed judgments.
Labeling AI-generated content can serve several purposes:
User Awareness: When content is generated by AI, labeling it as such informs users that they are consuming machine-generated material. This transparency can help users assess the reliability and trustworthiness of the information.
Ethical Considerations: Disclosing AI-generated content promotes ethical practices in content creation. It ensures that creators give credit to AI tools while also taking responsibility for the content they publish.
Content Originality: Labeling AI content helps distinguish it from human-generated content. This distinction can be crucial when it comes to issues of copyright and intellectual property.
Algorithmic Fairness: Transparency in labeling AI content contributes to the fairness of search engine results. Users and content creators should understand how algorithms work and how AI influences what they see in search results.
Balancing Act: Benefits and Concerns
The debate on labeling AI-generated content is not without its complexities. On one hand, transparency is critical to building trust and ensuring ethical content creation. On the other hand, labeling AI content might inadvertently stigmatize it or lead users to dismiss it as less credible.
Additionally, labeling raises questions about what constitutes AI-generated content. Is it content entirely generated by machines, or does it include content that is edited or curated by humans using AI assistance? Striking the right balance is crucial to avoid stifling innovation while upholding transparency.
The rise of AI-generated content presents both opportunities and challenges for content creators, consumers, and search engines like Google. While Google does not discriminate against AI-generated content, the labeling debate underscores the importance of transparency, ethics, and user awareness.
As AI continues to shape the content landscape, it is essential for stakeholders to engage in a thoughtful dialogue about the role of AI-generated content and the best practices for its integration into the digital ecosystem. Ultimately, finding a balance that promotes innovation, preserves trust, and upholds ethical content creation is key to navigating this evolving landscape successfully.
Engage with us to discover the potential with Mystic Web Designn!
#Mystic#Web Designn#Ultimately#promotes innovation#user awareness#content creators#consumers#search engines#like Google#AI-generated#Balancing Act#websites#blogs#marketing materials
0 notes
Text
Video: Melanie Gonick/MIT
A team of engineers has developed a new 3D inkjet printing system that utilizes computer vision for contact-free 3D printing, letting engineers print with high-performance materials they couldn’t use before.
Learn more.
Make sure to follow us on Tumblr!
#robot#robotics#3d printing#material science#machine learning#ai#electrical engineering#computer science#computer vision#engineering#Youtube
0 notes
Note
(half rant half story)
I'm a physicist. I work for a company that helps develop car parts. Essentially, car companies come to us with ideas on what they want from a part or material, and we make/test the idea or help them make/test it. Usually this means talking to other scientists and engineers and experts and it's all fine. Sometimes this means talking to businesspeople and board execs and I hate them
A bit ago when AI was really taking off in the zeitgeist I went to a meeting to talk about some tweaks Car Company A wanted to make to their hydraulics- specifically the master cylinder, but it doesn't super matter. I thought I'd be talking to their engineers - it ends up being just me, their head supervisor (who was not a scientist/engineer) and one of their executives from a different area (also not a scientist/engineer). I'm the only one in the room who actually knows how a car works, and also the lowest-level employee, and also aware that these people will give feedback to my boss based on how I 'represent the company ' whilst I'm here.
I start to explain my way through how I can make some of the changes they want - trying to do so in a way they'll understand - when Head Supervisor cuts me off and starts talking about AI. I'm like "oh well AI is often integrated into the software for a car but we're talking hardware right now, so that's not something we really ca-"
"Can you add artificial intelligence to the hydraulics?"
"..sorry, what was that?"
"Can you add AI to the hydraulics system?"
can i fucking what mate "Sir, I'm sorry, I'm a little confused - what do you mean by adding AI to the hydraulics?"
"I just thought this stuff could run smoother if you added AI to it. Most things do"
The part of the car that moves when you push the acceleration pedal is metal and liquid my dude what are you talking about "You want me to .add AI...to the pistons? To the master cylinder?"
"Yeah exactly, if you add AI to the bit that makes the pistons work, it should work better, right?"
IT'S METAL PIPES it's metal pipes it's metal pipes "Sir, there isn't any software in that part of the car"
"I know, but it's artificial intelligence, I'm sure there's a way to add it"
im exploding you with my mind you cannot seriously be asking me to add AI to a section of car that has as much fucking code attached to it as a SOCK what do you MEAN. The most complicated part of this thing is a SPRING you can't be serious
He was seriously asking. I've met my fair share of idiots but I was sure he wasn't genuinely seriously asking that I add AI directly to a piston system, but he was. And not even in the like "oh if we implement a way for AI to control that part" kind of way, he just vaguely thought that AI would "make it better" WHAT THE FUCK DO YOU MEANNNNN I HAD TO SPEND 20 MINUTES OF MY HARD EARNED LIFE EXPLAINING THAT NEITHER I NOR ANYONE ELSE CAN ADD AI TO A GOD DAMNED FUCKING PISTON. "CAN YOU ADD AI TO THE HYDRAULICS" NO BUT EVEN WITHOUT IT THAT METAL PIPE IS MORE INTELLIGENT THAN YOU
Posted by admin Rodney.
13K notes
·
View notes
Text
AI-assisted approach improves structural health monitoring of concrete bridges
- By Nuadox Crew -
A recent study published in Transportation Research Record by University of Central Florida (UCF) Engineering Professor Necati Catbas and his former Ph.D. student Marwan Debees—currently Bridge Program Manager at NASA—presents an AI-supported method for evaluating the structural condition of concrete bridges.
The system integrates infrared thermography, high-definition imaging, and neural networks to assist in bridge inspections by enhancing data analysis and potentially improving the consistency and efficiency of evaluations.
The study addresses challenges associated with inspecting the more than 650,000 bridges in the United States, many of which are aging and require maintenance. Traditional inspection methods typically rely on visual assessments and subjective judgments, which can be impacted by resource limitations. The authors propose that combining advanced sensing technologies with artificial intelligence could offer a more systematic and data-supported alternative to current practices.
The process outlined in the study draws on concepts from medical diagnostics. Similar to how doctors use imaging tools to supplement physical exams, engineers can apply infrared thermography and visual imaging to detect structural issues not visible on the surface. The thermal data may help identify subsurface problems such as delamination, moisture infiltration, or voids. Supplementary high-resolution images offer additional context. The sensors can be deployed on vehicles and boats, allowing for data collection from various parts of a bridge without major disruptions to traffic.
After data collection, environmental noise is filtered out, and the refined information is analyzed using a neural network. Trained on historical data and expert evaluations, the AI model classifies defects, assesses their severity, and marks locations that may require further inspection. Rather than replacing inspectors, the system is intended to serve as a decision-support tool, offering visual outputs and analysis that may help with prioritizing maintenance tasks.
The method has been applied in a real-world context at NASA, where it was used to reassess a bridge previously flagged for repair. According to the study, the system's analysis suggested that the bridge was structurally sound, potentially allowing for the reallocation of maintenance resources. The authors suggest that this approach could help public agencies make more targeted use of limited infrastructure budgets.
Although the study centers on concrete bridges, the researchers indicate that the methodology could be adapted to other types of infrastructure, including steel bridges and industrial structures. The key contribution lies in the integration of imaging technologies and AI to develop a potentially scalable approach to structural health monitoring.
Catbas, who directs UCF’s Civil Infrastructure Technologies for Resilience and Safety (CITRS) Initiative, and Debees, who is currently applying the method in a professional setting at NASA, present this work as a contribution to the growing field of AI applications in civil engineering. Their study aims to inform ongoing efforts to improve infrastructure monitoring and maintenance practices through technological integration.
Read more at University of Central Florida
Scientific paper: Marwan Debees et al, Rapid Evaluation and Decision-Making for Concrete Bridges, Transportation Research Record: Journal of the Transportation Research Board (2025). DOI: 10.1177/03611981251330892
Related Content
Novel material facilitates measurement of concrete deterioration in buildings and other structures
Other Recent News
Designing quieter data centers for residential areas: Experts highlight the need for clearer noise regulations and collaborative solutions as data centers expand into communities.
#bridge#ai#imaging#engineering#concrete#materials#nasa#machine learning#Infrared thermography#infrastructure
0 notes
Text
Future of Artificial Intelligence
The Future of Artificial Intelligence: A Glimpse into Tomorrow
Artificial intelligence (AI) is rapidly transforming industries and reshaping our world. As WikiGlitz explores the future of AI, we delve into the exciting possibilities and challenges that lie ahead.
From self-driving cars and intelligent personal assistants to advanced medical diagnostics and personalized learning experiences, AI has the potential to revolutionize countless aspects of our lives. However, ethical considerations, job displacement, and the potential for misuse are important factors to consider.
As AI continues to evolve, WikiGlitz will keep you informed about the latest breakthroughs, applications, and implications for the future. Stay tuned for more insights into the exciting world of artificial intelligence. If you like to know more about the AI Breakthroughs: 6 Amazing Advances You Need to Know then check out our in-depth article. Visit our official blog for the latest updates.
#virtual reality#augmented reality#blockchain technology#blockchain#ai technology#technology#technology tips#chatgpt#gemini ai#cloud computing#machine learning#latest technology in artificial intelligence#future of artificial intelligence#renewable energy and sustainability#biotechnology and genetic engineering#quantum computing#virtual and augmented reality (vr/ar)#internet of things (iot)#blockchain and cryptocurrency#artificial intelligence#brain-computer interfaces#robotics#cybersecurity#big data and analytics#space exploration#materials science#nanotechnology#artificial general intelligence (agi)#brain-computer interfaces (bcis)
1 note
·
View note