#yichi
Explore tagged Tumblr posts
Text
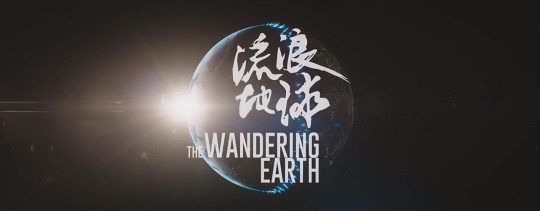


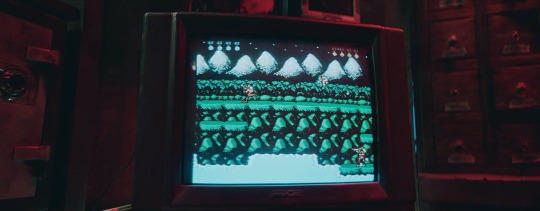
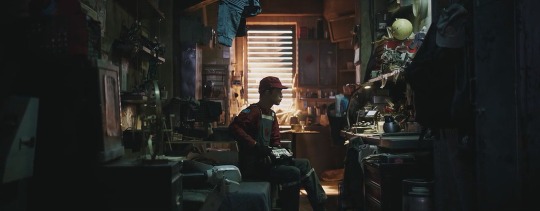
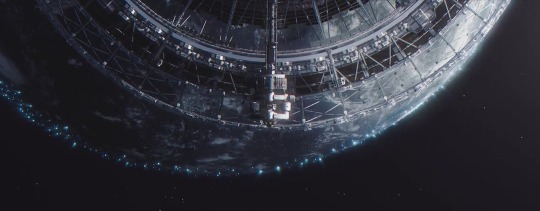
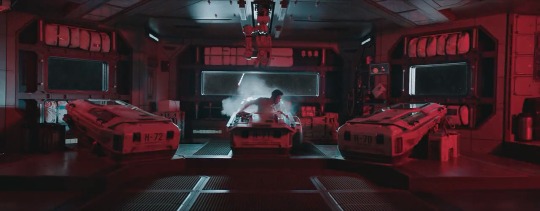

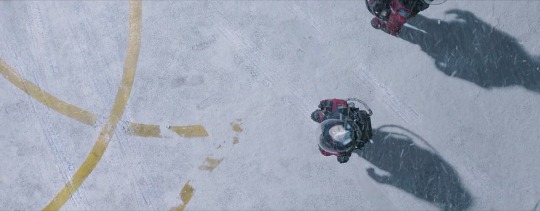




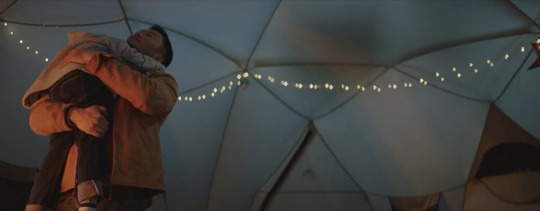
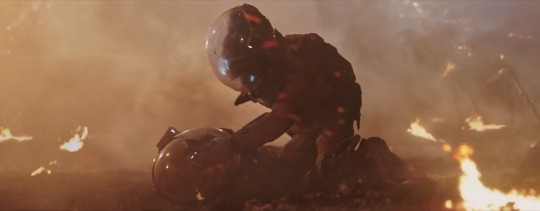

The Wandering Earth (2019)
Director - Frant Gwo, Cinematography - Michael Liu
#scenesandscreens#the wandering earth#Frant Gwo#Jiang Zhigang#zhang huan#Lei Jiayin#Yang Haoyu#Li Hongchen#Yang Yi#Mike Sui#Qu Jingjing#Zhang Yichi#zhao jinmai#wu jing#Arkady Sharogradsky#Qu Chuxiao#Li Guangjie#Ng Man-tat#The Wandering Earth 2#Michael Liu
44 notes
·
View notes
Text
Do you want to play board games?
From this incorrect quote by: @yichi-is-a-bitch
This… took longer than I expected. Shading with just black was weird I'VE BEEN TRYING TO FINISH THIS FOR WEEKS, the most difficult thing was undoubtedly Epel's pose, but now it doesn't matter :D
Without text:
#fuck tumblr#the quality was lowered#to better appreciate the fanart pick the pic#ෆ — my art#ෆ — sona art#twst comic#Ace trappola#ace#twst#twisted wonderland#twisted wonderland fanart#art#digital art#yuu#yuusona#twst ace#deuce spade#epel felmier#art of tumblr#my art#art of the day#incorrect quote
3K notes
·
View notes
Note
Hello! I just want to ask. I see you know Miss the Dragon. Hey doesn't every episode feel like déjà vu? Yuchi does the same thing all the time; rescues Liu Ying. Of course the series has something in it, it's romantic and funny but sometimes I felt that Yuchi was just "pushing" Liu Ying and Liu Ying was overly clingy. Like how she kept yelling, ''Yichi gege!'' I apologize to Miss the Dragon fans but sometimes I found her behavior a bit childish. Otherwise the series was fine, for example Qing Qing and Xue Qianxun were great! And as for Immortal of Fate; I didn't expect him to be such a bastard in the end. I didn't expect such plots but I liked them. Ily bye!🩷
Hello! To answer your question, yes it was a bit déjà vu. I personally don't mind, because of the little changes and such, but I can definitely see how it gets annoying fast for some. As for the yichi gege, that's not abnormal for Chinese dramas nor is the more childish behavior and I've gotten used to it due to other drama's. You kind of to accept it, unfortunately, but in the drama it makes a bit of sense since the FL is still pretty young in every recarnation and thus a bit more childlike. Overall, it has a good story and cast, I'm afraid it was just a bit too long and thus falls in repeat pretty often.
Hope that this answer makes it clear 😊 Definitely isn't the worst I've seen with female/male lead's, Ahro from Hwarang makes my blood boil for example 😂
2 notes
·
View notes
Text
Tesla’nın robotaksi hamlesine Çin’den tokat gibi yanıt!
Baidu, sürücüsüz taksi hizmeti Apollo Go ile otonom araç sektöründe önemli bir adım attı. “Çinli Google” olarak da bilinen Baidu, JMC ile geliştirdiği Yichi 06 (Apollo RT6) modelini 29 bin dolar gibi bir fiyatla piyasaya sürdü ve teslimatlara başladı. Bu hamle, Tesla’nın yeni tanıttığı Cybercab modeli için önemli bir rakip. Tesla Cybercab vs Baidu Yichi 06 (Apollo RT6) Yichi 06; minimalist…

View On WordPress
0 notes
Link
0 notes
Text
Flying Qudit: Membuka Dimensi Baru Komunikasi Kuantum
Gambar 1. Foton sinyal, yang dimanipulasi oleh sirkuit fotonik terpadu, menciptakan qudit 4D yang direpresentasikan oleh sekumpulan bola oranye. Sementara itu, foton diam, yang direpresentasikan oleh bola biru, bertindak sebagai pengendali jarak jauh untuk foton sinyal.Kredit: Haoqi Zhao, Yichi Zhang, Zihe Gao, Jieun Yim, Shuang Wu, Natalia M. Litchinitser, Li Ge, dan Liang Feng, diedit Para…
0 notes
Text
'You won’t find any tea in Liquorland,’ Yu Yichi replied. ‘Liquor is our tea.'
— Mo Yan, The Republic of Wine
0 notes
Text
Google at NeurIPS 2023
New Post has been published on https://thedigitalinsider.com/google-at-neurips-2023/
Google at NeurIPS 2023
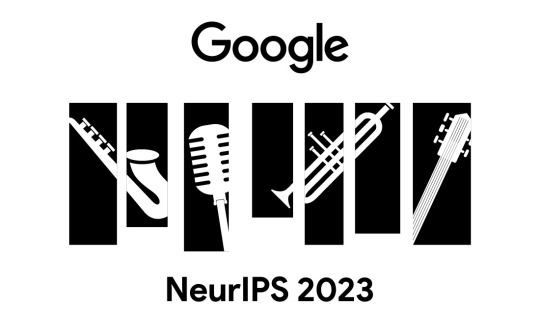
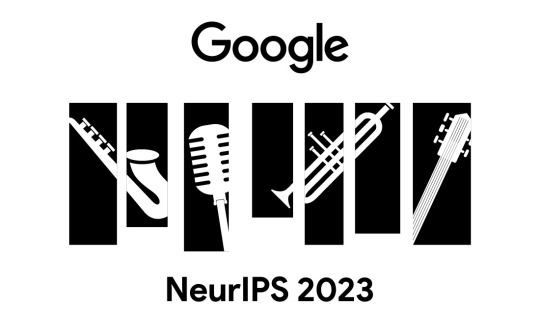
This week the 37th annual Conference on Neural Information Processing Systems (NeurIPS 2023), the biggest machine learning conference of the year, kicks off in New Orleans, LA. Google is proud to be a Diamond Level sponsor of NeurIPS this year and will have a strong presence with >170 accepted papers, two keynote talks, and additional contributions to the broader research community through organizational support and involvement in >20 workshops and tutorials. Google is also proud to be a Platinum Sponsor for both the Women in Machine Learning and LatinX in AI workshops. We look forward to sharing some of our extensive ML research and expanding our partnership with the broader ML research community.
Attending for NeurIPS 2023 in person? Come visit the Google Research booth to learn more about the exciting work we’re doing to solve some of the field’s most interesting challenges. Visit the @GoogleAI X (Twitter) account to find out about Google booth activities (e.g., demos and Q&A sessions).
You can learn more about our latest cutting edge work being presented at the conference in the list below (Google affiliations highlighted in bold). And see Google DeepMind’s blog to learn more about their participation at NeurIPS 2023.
Anonymous Learning via Look-Alike Clustering: A Precise Analysis of Model Generalization Adel Javanmard, Vahab Mirrokni
Better Private Linear Regression Through Better Private Feature Selection Travis Dick, Jennifer Gillenwater*, Matthew Joseph
Binarized Neural Machine Translation Yichi Zhang, Ankush Garg, Yuan Cao, Łukasz Lew, Behrooz Ghorbani*, Zhiru Zhang, Orhan Firat
BoardgameQA: A Dataset for Natural Language Reasoning with Contradictory Information Mehran Kazemi, Quan Yuan, Deepti Bhatia, Najoung Kim, Xin Xu, Vaiva Imbrasaite, Deepak Ramachandran
Boosting with Tempered Exponential Measures Richard Nock, Ehsan Amid, Manfred Warmuth
Concept Algebra for (Score-Based) Text-Controlled Generative Models Zihao Wang, Lin Gui, Jeffrey Negrea, Victor Veitch
Deep Contract Design via Discontinuous Networks Tonghan Wang, Paul Dütting, Dmitry Ivanov, Inbal Talgam-Cohen, David C. Parkes
Diffusion-SS3D: Diffusion Model for Semi-supervised 3D Object Detection Cheng-Ju Ho, Chen-Hsuan Tai, Yen-Yu Lin, Ming-Hsuan Yang, Yi-Hsuan Tsai
Eliciting User Preferences for Personalized Multi-Objective Decision Making through Comparative Feedback Han Shao, Lee Cohen, Avrim Blum, Yishay Mansour, Aadirupa Saha, Matthew Walter
Gradient Descent with Linearly Correlated Noise: Theory and Applications to Differential Privacy Anastasia Koloskova*, Ryan McKenna, Zachary Charles, J Keith Rush, Hugh Brendan McMahan
Hardness of Low Rank Approximation of Entrywise Transformed Matrix Products Tamas Sarlos, Xingyou Song, David P. Woodruff, Qiuyi (Richard) Zhang
Module-wise Adaptive Distillation for Multimodality Foundation Models
Chen Liang, Jiahui Yu, Ming-Hsuan Yang, Matthew Brown, Yin Cui, Tuo Zhao, Boqing Gong, Tianyi Zhou
Multi-Swap k-Means++ Lorenzo Beretta, Vincent Cohen-Addad, Silvio Lattanzi, Nikos Parotsidis
OpenMask3D: Open-Vocabulary 3D Instance Segmentation Ayça Takmaz, Elisabetta Fedele, Robert Sumner, Marc Pollefeys, Federico Tombari, Francis Engelmann
Order Matters in the Presence of Dataset Imbalance for Multilingual Learning Dami Choi*, Derrick Xin, Hamid Dadkhahi, Justin Gilmer, Ankush Garg, Orhan Firat, Chih-Kuan Yeh, Andrew M. Dai, Behrooz Ghorbani
PopSign ASL v1.0: An Isolated American Sign Language Dataset Collected via Smartphones Thad Starner, Sean Forbes, Matthew So, David Martin, Rohit Sridhar, Gururaj Deshpande, Sam Sepah, Sahir Shahryar, Khushi Bhardwaj, Tyler Kwok, Daksh Sehgal, Saad Hassan, Bill Neubauer, Sofia Vempala, Alec Tan, Jocelyn Heath, Unnathi Kumar, Priyanka Mosur, Tavenner Hall, Rajandeep Singh, Christopher Cui, Glenn Cameron, Sohier Dane, Garrett Tanzer
Semi-Implicit Denoising Diffusion Models (SIDDMs) Yanwu Xu*, Mingming Gong, Shaoan Xie, Wei Wei, Matthias Grundmann, Kayhan Batmanghelich, Tingbo Hou
State2Explanation: Concept-Based Explanations to Benefit Agent Learning and User Understanding Devleena Das, Sonia Chernova, Been Kim
StoryBench: A Multifaceted Benchmark for Continuous Story Visualization Emanuele Bugliarello*, Hernan Moraldo, Ruben Villegas, Mohammad Babaeizadeh, Mohammad Taghi Saffar, Han Zhang, Dumitru Erhan, Vittorio Ferrari, Pieter-Jan Kindermans, Paul Voigtlaender
Subject-driven Text-to-Image Generation via Apprenticeship Learning Wenhu Chen, Hexiang Hu, Yandong Li, Nataniel Ruiz, Xuhui Jia, Ming-Wei Chang, William W. Cohen
TpuGraphs: A Performance Prediction Dataset on Large Tensor Computational Graphs Phitchaya Mangpo Phothilimthana, Sami Abu-El-Haija, Kaidi Cao*, Bahare Fatemi, Mike Burrows, Charith Mendis*, Bryan Perozzi
Training Chain-of-Thought via Latent-Variable Inference Du Phan, Matthew D. Hoffman, David Dohan*, Sholto Douglas, Tuan Anh Le, Aaron Parisi, Pavel Sountsov, Charles Sutton, Sharad Vikram, Rif A. Saurous
Unified Lower Bounds for Interactive High-dimensional Estimation under Information Constraints Jayadev Acharya, Clement L. Canonne, Ziteng Sun, Himanshu Tyagi
What You See is What You Read? Improving Text-Image Alignment Evaluation Michal Yarom, Yonatan Bitton, Soravit Changpinyo, Roee Aharoni, Jonathan Herzig, Oran Lang, Eran Ofek, Idan Szpektor
When Does Confidence-Based Cascade Deferral Suffice? Wittawat Jitkrittum, Neha Gupta, Aditya Krishna Menon, Harikrishna Narasimhan, Ankit Singh Rawat, Sanjiv Kumar
Accelerating Molecular Graph Neural Networks via Knowledge Distillation Filip Ekström Kelvinius, Dimitar Georgiev, Artur Petrov Toshev, Johannes Gasteiger
AVIS: Autonomous Visual Information Seeking with Large Language Model Agent Ziniu Hu*, Ahmet Iscen, Chen Sun, Kai-Wei Chang, Yizhou Sun, David Ross, Cordelia Schmid, Alireza Fathi
Beyond Invariance: Test-Time Label-Shift Adaptation for Addressing “Spurious” Correlations Qingyao Sun, Kevin Patrick Murphy, Sayna Ebrahimi, Alexander D’Amour
Collaborative Score Distillation for Consistent Visual Editing Subin Kim, Kyungmin Lee, June Suk Choi, Jongheon Jeong, Kihyuk Sohn, Jinwoo Shin
CommonScenes: Generating Commonsense 3D Indoor Scenes with Scene Graphs Guangyao Zhai, Evin Pınar Örnek, Shun-Cheng Wu, Yan Di, Federico Tombari, Nassir Navab, Benjamin Busam
Computational Complexity of Learning Neural Networks: Smoothness and Degeneracy Amit Daniely, Nathan Srebro, Gal Vardi
A Computationally Efficient Sparsified Online Newton Method Fnu Devvrit*, Sai Surya Duvvuri, Rohan Anil, Vineet Gupta, Cho-Jui Hsieh, Inderjit S Dhillon
DDF-HO: Hand-Held Object Reconstruction via Conditional Directed Distance Field Chenyangguang Zhang, Yan Di, Ruida Zhang, Guangyao Zhai, Fabian Manhardt, Federico Tombari, Xiangyang Ji
Double Auctions with Two-sided Bandit Feedback Soumya Basu, Abishek Sankararaman
Grammar Prompting for Domain-Specific Language Generation with Large Language Models Bailin Wang, Zi Wang, Xuezhi Wang, Yuan Cao, Rif A. Saurous, Yoon Kim
Inconsistency, Instability, and Generalization Gap of Deep Neural Network Training Rie Johnson, Tong Zhang*
Large Graph Property Prediction via Graph Segment Training Kaidi Cao*, Phitchaya Mangpo Phothilimthana, Sami Abu-El-Haija, Dustin Zelle, Yanqi Zhou, Charith Mendis*, Jure Leskovec, Bryan Perozzi
On Computing Pairwise Statistics with Local Differential Privacy Badih Ghazi, Pritish Kamath, Ravi Kumar, Pasin Manurangsi, Adam Sealfon
On Student-teacher Deviations in Distillation: Does it Pay to Disobey? Vaishnavh Nagarajan, Aditya Krishna Menon, Srinadh Bhojanapalli, Hossein Mobahi, Sanjiv Kumar
Optimal Cross-learning for Contextual Bandits with Unknown Context Distributions Jon Schneider, Julian Zimmert
Near-Optimal k-Clustering in the Sliding Window Model David Woodruff, Peilin Zhong, Samson Zhou
Post Hoc Explanations of Language Models Can Improve Language Models Satyapriya Krishna, Jiaqi Ma, Dylan Z Slack, Asma Ghandeharioun, Sameer Singh, Himabindu Lakkaraju
Recommender Systems with Generative Retrieval Shashank Rajput*, Nikhil Mehta, Anima Singh, Raghunandan Hulikal Keshavan, Trung Vu, Lukasz Heldt, Lichan Hong, Yi Tay, Vinh Q. Tran, Jonah Samost, Maciej Kula, Ed H. Chi, Maheswaran Sathiamoorthy
Reinforcement Learning for Fine-tuning Text-to-Image Diffusion Models Ying Fan, Olivia Watkins, Yuqing Du, Hao Liu, Moonkyung Ryu, Craig Boutilier, Pieter Abbeel, Mohammad Ghavamzadeh*, Kangwook Lee, Kimin Lee*
Replicable Clustering Hossein Esfandiari, Amin Karbasi, Vahab Mirrokni, Grigoris Velegkas, Felix Zhou
Replicability in Reinforcement Learning Amin Karbasi, Grigoris Velegkas, Lin Yang, Felix Zhou
Riemannian Projection-free Online Learning Zihao Hu, Guanghui Wang, Jacob Abernethy
Sharpness-Aware Minimization Leads to Low-Rank Features Maksym Andriushchenko, Dara Bahri, Hossein Mobahi, Nicolas Flammarion
What is the Inductive Bias of Flatness Regularization? A Study of Deep Matrix Factorization Models Khashayar Gatmiry, Zhiyuan Li, Ching-Yao Chuang, Sashank Reddi, Tengyu Ma, Stefanie Jegelka
Block Low-Rank Preconditioner with Shared Basis for Stochastic Optimization Jui-Nan Yen, Sai Surya Duvvuri, Inderjit S Dhillon, Cho-Jui Hsieh
Blocked Collaborative Bandits: Online Collaborative Filtering with Per-Item Budget Constraints Soumyabrata Pal, Arun Sai Suggala, Karthikeyan Shanmugam, Prateek Jain
Boundary Guided Learning-Free Semantic Control with Diffusion Models Ye Zhu, Yu Wu, Zhiwei Deng, Olga Russakovsky, Yan Yan
Conditional Adapters: Parameter-efficient Transfer Learning with Fast Inference Tao Lei, Junwen Bai, Siddhartha Brahma, Joshua Ainslie, Kenton Lee, Yanqi Zhou, Nan Du*, Vincent Y. Zhao, Yuexin Wu, Bo Li, Yu Zhang, Ming-Wei Chang
Conformal Prediction for Time Series with Modern Hopfield Networks Andreas Auer, Martin Gauch, Daniel Klotz, Sepp Hochreiter
Does Visual Pretraining Help End-to-End Reasoning? Chen Sun, Calvin Luo, Xingyi Zhou, Anurag Arnab, Cordelia Schmid
Effective Robustness Against Natural Distribution Shifts for Models with Different Training Data Zhouxing Shi*, Nicholas Carlini, Ananth Balashankar, Ludwig Schmidt, Cho-Jui Hsieh, Alex Beutel*, Yao Qin
Improving Neural Network Representations Using Human Similarity Judgments Lukas Muttenthaler*, Lorenz Linhardt, Jonas Dippel, Robert A. Vandermeulen, Katherine Hermann, Andrew K. Lampinen, Simon Kornblith
Label Robust and Differentially Private Linear Regression: Computational and Statistical Efficiency Xiyang Liu, Prateek Jain, Weihao Kong, Sewoong Oh, Arun Sai Suggala
Mnemosyne: Learning to Train Transformers with Transformers Deepali Jain, Krzysztof Choromanski, Avinava Dubey, Sumeet Singh, Vikas Sindhwani, Tingnan Zhang, Jie Tan
Nash Regret Guarantees for Linear Bandits Ayush Sawarni, Soumyabrata Pal, Siddharth Barman
A Near-Linear Time Algorithm for the Chamfer Distance Ainesh Bakshi, Piotr Indyk, Rajesh Jayaram, Sandeep Silwal, Erik Waingarten.
On Differentially Private Sampling from Gaussian and Product Distributions Badih Ghazi, Xiao Hu*, Ravi Kumar, Pasin Manurangsi
On Dynamic Programming Decompositions of Static Risk Measures in Markov Decision Processes Jia Lin Hau, Erick Delage, Mohammad Ghavamzadeh*, Marek Petrik
ResMem: Learn What You Can and Memorize the Rest Zitong Yang, Michal Lukasik, Vaishnavh Nagarajan, Zonglin Li, Ankit Singh Rawat, Manzil Zaheer, Aditya Krishna Menon, Sanjiv Kumar
Responsible AI (RAI) Games and Ensembles Yash Gupta, Runtian Zhai, Arun Suggala, Pradeep Ravikumar
RoboCLIP: One Demonstration Is Enough to Learn Robot Policies Sumedh A Sontakke, Jesse Zhang, Sébastien M. R. Arnold, Karl Pertsch, Erdem Biyik, Dorsa Sadigh, Chelsea Finn, Laurent Itti
Robust Concept Erasure via Kernelized Rate-Distortion Maximization Somnath Basu Roy Chowdhury, Nicholas Monath, Kumar Avinava Dubey, Amr Ahmed, Snigdha Chaturvedi
Robust Multi-Agent Reinforcement Learning via Adversarial Regularization: Theoretical Foundation and Stable Algorithms Alexander Bukharin, Yan Li, Yue Yu, Qingru Zhang, Zhehui Chen, Simiao Zuo, Chao Zhang, Songan Zhang, Tuo Zhao
Simplicity Bias in 1-Hidden Layer Neural Networks Depen Morwani*, Jatin Batra, Prateek Jain, Praneeth Netrapalli
SLaM: Student-Label Mixing for Distillation with Unlabeled Examples Vasilis Kontonis, Fotis Iliopoulos, Khoa Trinh, Cenk Baykal, Gaurav Menghani, Erik Vee
SNAP: Self-Supervised Neural Maps for Visual Positioning and Semantic Understanding Paul-Edouard Sarlin*, Eduard Trulls, Marc Pollefeys, Jan Hosang, Simon Lynen
SOAR: Improved Indexing for Approximate Nearest Neighbor Search Philip Sun, David Simcha, Dave Dopson, Ruiqi Guo, Sanjiv Kumar
StyleDrop: Text-to-Image Synthesis of Any Style Kihyuk Sohn, Lu Jiang, Jarred Barber, Kimin Lee*, Nataniel Ruiz, Dilip Krishnan, Huiwen Chang*, Yuanzhen Li, Irfan Essa, Michael Rubinstein, Yuan Hao, Glenn Entis, Irina Blok, Daniel Castro Chin
Three Towers: Flexible Contrastive Learning with Pretrained Image Models Jannik Kossen*, Mark Collier, Basil Mustafa, Xiao Wang, Xiaohua Zhai, Lucas Beyer, Andreas Steiner, Jesse Berent, Rodolphe Jenatton, Efi Kokiopoulou
Two-Stage Learning to Defer with Multiple Experts Anqi Mao, Christopher Mohri, Mehryar Mohri, Yutao Zhong
AdANNS: A Framework for Adaptive Semantic Search Aniket Rege, Aditya Kusupati, Sharan Ranjit S, Alan Fan, Qingqing Cao, Sham Kakade, Prateek Jain, Ali Farhadi
Cappy: Outperforming and Boosting Large Multi-Task LMs with a Small Scorer Bowen Tan*, Yun Zhu, Lijuan Liu, Eric Xing, Zhiting Hu, Jindong Chen
Causal-structure Driven Augmentations for Text OOD Generalization Amir Feder, Yoav Wald, Claudia Shi, Suchi Saria, David Blei
Dense-Exponential Random Features: Sharp Positive Estimators of the Gaussian Kernel Valerii Likhosherstov, Krzysztof Choromanski, Avinava Dubey, Frederick Liu, Tamas Sarlos, Adrian Weller
Diffusion Hyperfeatures: Searching Through Time and Space for Semantic Correspondence Grace Luo, Lisa Dunlap, Dong Huk Park, Aleksander Holynski, Trevor Darrell
Diffusion Self-Guidance for Controllable Image Generation Dave Epstein, Allan Jabri, Ben Poole, Alexei A Efros, Aleksander Holynski
Fully Dynamic k-Clustering in Õ(k) Update Time Sayan Bhattacharya, Martin Nicolas Costa, Silvio Lattanzi, Nikos Parotsidis
Improving CLIP Training with Language Rewrites Lijie Fan, Dilip Krishnan, Phillip Isola, Dina Katabi, Yonglong Tian
<!–k-Means Clustering with Distance-Based Privacy Alessandro Epasto, Vahab Mirrokni, Shyam Narayanan, Peilin Zhong
–>
LayoutGPT: Compositional Visual Planning and Generation with Large Language Models Weixi Feng, Wanrong Zhu, Tsu-Jui Fu, Varun Jampani, Arjun Reddy Akula, Xuehai He, Sugato Basu, Xin Eric Wang, William Yang Wang
Offline Reinforcement Learning for Mixture-of-Expert Dialogue Management Dhawal Gupta*, Yinlam Chow, Azamat Tulepbergenov, Mohammad Ghavamzadeh*, Craig Boutilier
Optimal Unbiased Randomizers for Regression with Label Differential Privacy Ashwinkumar Badanidiyuru, Badih Ghazi, Pritish Kamath, Ravi Kumar, Ethan Jacob Leeman, Pasin Manurangsi, Avinash V Varadarajan, Chiyuan Zhang
Paraphrasing Evades Detectors of AI-generated Text, but Retrieval Is an Effective Defense Kalpesh Krishna, Yixiao Song, Marzena Karpinska, John Wieting, Mohit Iyyer
ReMaX: Relaxing for Better Training on Efficient Panoptic Segmentation Shuyang Sun*, Weijun Wang, Qihang Yu*, Andrew Howard, Philip Torr, Liang-Chieh Chen*
Robust and Actively Secure Serverless Collaborative Learning Nicholas Franzese, Adam Dziedzic, Christopher A. Choquette-Choo, Mark R. Thomas, Muhammad Ahmad Kaleem, Stephan Rabanser, Congyu Fang, Somesh Jha, Nicolas Papernot, Xiao Wang
SpecTr: Fast Speculative Decoding via Optimal Transport Ziteng Sun, Ananda Theertha Suresh, Jae Hun Ro, Ahmad Beirami, Himanshu Jain, Felix Yu
Structured Prediction with Stronger Consistency Guarantees Anqi Mao, Mehryar Mohri, Yutao Zhong
Affinity-Aware Graph Networks Ameya Velingker, Ali Kemal Sinop, Ira Ktena, Petar Veličković, Sreenivas Gollapudi
ARTIC3D: Learning Robust Articulated 3D Shapes from Noisy Web Image Collections Chun-Han Yao*, Amit Raj, Wei-Chih Hung, Yuanzhen Li, Michael Rubinstein, Ming-Hsuan Yang, Varun Jampani
Black-Box Differential Privacy for Interactive ML Haim Kaplan, Yishay Mansour, Shay Moran, Kobbi Nissim, Uri Stemmer
Bypassing the Simulator: Near-Optimal Adversarial Linear Contextual Bandits Haolin Liu, Chen-Yu Wei, Julian Zimmert
DaTaSeg: Taming a Universal Multi-Dataset Multi-Task Segmentation Model
Xiuye Gu, Yin Cui*, Jonathan Huang, Abdullah Rashwan, Xuan Yang, Xingyi Zhou, Golnaz Ghiasi, Weicheng Kuo, Huizhong Chen, Liang-Chieh Chen*, David Ross
Easy Learning from Label Proportions Robert Busa-Fekete, Heejin Choi*, Travis Dick, Claudio Gentile, Andres Munoz Medina
Efficient Data Subset Selection to Generalize Training Across Models: Transductive and Inductive Networks Eeshaan Jain, Tushar Nandy, Gaurav Aggarwal, Ashish Tendulkar, Rishabh Iyer, Abir De
Faster Differentially Private Convex Optimization via Second-Order Methods Arun Ganesh, Mahdi Haghifam*, Thomas Steinke, Abhradeep Guha Thakurta
Finding Safe Zones of Markov Decision Processes Policies Lee Cohen, Yishay Mansour, Michal Moshkovitz
Focused Transformer: Contrastive Training for Context Scaling Szymon Tworkowski, Konrad Staniszewski, Mikołaj Pacek, Yuhuai Wu*, Henryk Michalewski, Piotr Miłoś
Front-door Adjustment Beyond Markov Equivalence with Limited Graph Knowledge Abhin Shah, Karthikeyan Shanmugam, Murat Kocaoglu
H-Consistency Bounds: Characterization and Extensions Anqi Mao, Mehryar Mohri, Yutao Zhong
Inverse Dynamics Pretraining Learns Good Representations for Multitask Imitation David Brandfonbrener, Ofir Nachum, Joan Bruna
Most Neural Networks Are Almost Learnable Amit Daniely, Nathan Srebro, Gal Vardi
Multiclass Boosting: Simple and Intuitive Weak Learning Criteria Nataly Brukhim, Amit Daniely, Yishay Mansour, Shay Moran
NeRF Revisited: Fixing Quadrature Instability in Volume Rendering Mikaela Angelina Uy, Kiyohiro Nakayama, Guandao Yang, Rahul Krishna Thomas, Leonidas Guibas, Ke Li
Privacy Amplification via Compression: Achieving the Optimal Privacy-Accuracy-Communication Trade-off in Distributed Mean Estimation Wei-Ning Chen, Dan Song, Ayfer Ozgur, Peter Kairouz
Private Federated Frequency Estimation: Adapting to the Hardness of the Instance Jingfeng Wu*, Wennan Zhu, Peter Kairouz, Vladimir Braverman
RETVec: Resilient and Efficient Text Vectorizer Elie Bursztein, Marina Zhang, Owen Skipper Vallis, Xinyu Jia, Alexey Kurakin
Symbolic Discovery of Optimization Algorithms Xiangning Chen*, Chen Liang, Da Huang, Esteban Real, Kaiyuan Wang, Hieu Pham, Xuanyi Dong, Thang Luong, Cho-Jui Hsieh, Yifeng Lu, Quoc V. Le
A Tale of Two Features: Stable Diffusion Complements DINO for Zero-Shot Semantic Correspondence Junyi Zhang, Charles Herrmann, Junhwa Hur, Luisa F. Polania, Varun Jampani, Deqing Sun, Ming-Hsuan Yang
A Trichotomy for Transductive Online Learning Steve Hanneke, Shay Moran, Jonathan Shafer
A Unified Fast Gradient Clipping Framework for DP-SGD William Kong, Andres Munoz Medina
Unleashing the Power of Randomization in Auditing Differentially Private ML Krishna Pillutla, Galen Andrew, Peter Kairouz, H. Brendan McMahan, Alina Oprea, Sewoong Oh
(Amplified) Banded Matrix Factorization: A unified approach to private training Christopher A Choquette-Choo, Arun Ganesh, Ryan McKenna, H Brendan McMahan, Keith Rush, Abhradeep Guha Thakurta, Zheng Xu
Adversarial Resilience in Sequential Prediction via Abstention Surbhi Goel, Steve Hanneke, Shay Moran, Abhishek Shetty
Alternating Gradient Descent and Mixture-of-Experts for Integrated Multimodal Perception Hassan Akbari, Dan Kondratyuk, Yin Cui, Rachel Hornung, Huisheng Wang, Hartwig Adam
Android in the Wild: A Large-Scale Dataset for Android Device Control Christopher Rawles, Alice Li, Daniel Rodriguez, Oriana Riva, Timothy Lillicrap
Benchmarking Robustness to Adversarial Image Obfuscations Florian Stimberg, Ayan Chakrabarti, Chun-Ta Lu, Hussein Hazimeh, Otilia Stretcu, Wei Qiao, Yintao Liu, Merve Kaya, Cyrus Rashtchian, Ariel Fuxman, Mehmet Tek, Sven Gowal
Building Socio-culturally Inclusive Stereotype Resources with Community Engagement Sunipa Dev, Jaya Goyal, Dinesh Tewari, Shachi Dave, Vinodkumar Prabhakaran
Consensus and Subjectivity of Skin Tone Annotation for ML Fairness Candice Schumann, Gbolahan O Olanubi, Auriel Wright, Ellis Monk Jr*, Courtney Heldreth, Susanna Ricco
Counting Distinct Elements Under Person-Level Differential Privacy Alexander Knop, Thomas Steinke
DICES Dataset: Diversity in Conversational AI Evaluation for Safety Lora Aroyo, Alex S. Taylor, Mark Diaz, Christopher M. Homan, Alicia Parrish, Greg Serapio-García, Vinodkumar Prabhakaran, Ding Wang
Does Progress on ImageNet Transfer to Real-world Datasets? Alex Fang, Simon Kornblith, Ludwig Schmidt
Estimating Generic 3D Room Structures from 2D Annotations Denys Rozumnyi*, Stefan Popov, Kevis-kokitsi Maninis, Matthias Nießner, Vittorio Ferrari
Large Language Model as Attributed Training Data Generator: A Tale of Diversity and Bias Yue Yu, Yuchen Zhuang, Jieyu Zhang, Yu Meng, Alexander Ratner, Ranjay Krishna, Jiaming Shen, Chao Zhang
MADLAD-400: A Multilingual And Document-Level Large Audited Dataset Sneha Kudugunta, Isaac Caswell, Biao Zhang, Xavier Garcia, Derrick Xin, Aditya Kusupati, Romi Stella, Ankur Bapna, Orhan Firat
Mechanic: A Learning Rate Tuner Ashok Cutkosky, Aaron Defazio, Harsh Mehta
NAVI: Category-Agnostic Image Collections with High-Quality 3D Shape and Pose Annotations Varun Jampani, Kevis-kokitsi Maninis, Andreas Engelhardt, Arjun Karpur, Karen Truong, Kyle Sargent, Stefan Popov, Andre Araujo, Ricardo Martin Brualla, Kaushal Patel, Daniel Vlasic, Vittorio Ferrari, Ameesh Makadia, Ce Liu*, Yuanzhen Li, Howard Zhou
Neural Ideal Large Eddy Simulation: Modeling Turbulence with Neural Stochastic Differential Equations Anudhyan Boral, Zhong Yi Wan, Leonardo Zepeda-Nunez, James Lottes, Qing Wang, Yi-Fan Chen, John Roberts Anderson, Fei Sha
Restart Sampling for Improving Generative Processes Yilun Xu, Mingyang Deng, Xiang Cheng, Yonglong Tian, Ziming Liu, Tommi Jaakkola
Rethinking Incentives in Recommender Systems: Are Monotone Rewards Always Beneficial? Fan Yao, Chuanhao Li, Karthik Abinav Sankararaman, Yiming Liao, Yan Zhu, Qifan Wang, Hongning Wang, Haifeng Xu
Revisiting Evaluation Metrics for Semantic Segmentation: Optimization and Evaluation of Fine-grained Intersection over Union Zifu Wang, Maxim Berman, Amal Rannen-Triki, Philip Torr, Devis Tuia, Tinne Tuytelaars, Luc Van Gool, Jiaqian Yu, Matthew B. Blaschko
RoboHive: A Unified Framework for Robot Learning Vikash Kumar, Rutav Shah, Gaoyue Zhou, Vincent Moens, Vittorio Caggiano, Abhishek Gupta, Aravind Rajeswaran
SatBird: Bird Species Distribution Modeling with Remote Sensing and Citizen Science Data Mélisande Teng, Amna Elmustafa, Benjamin Akera, Yoshua Bengio, Hager Radi, Hugo Larochelle, David Rolnick
Sparsity-Preserving Differentially Private Training of Large Embedding Models Badih Ghazi, Yangsibo Huang*, Pritish Kamath, Ravi Kumar, Pasin Manurangsi, Amer Sinha, Chiyuan Zhang
StableRep: Synthetic Images from Text-to-Image Models Make Strong Visual Representation Learners Yonglong Tian, Lijie Fan, Phillip Isola, Huiwen Chang, Dilip Krishnan
Towards Federated Foundation Models: Scalable Dataset Pipelines for Group-Structured Learning Zachary Charles, Nicole Mitchell, Krishna Pillutla, Michael Reneer, Zachary Garrett
Universality and Limitations of Prompt Tuning Yihan Wang, Jatin Chauhan, Wei Wang, Cho-Jui Hsieh
Unsupervised Semantic Correspondence Using Stable Diffusion Eric Hedlin, Gopal Sharma, Shweta Mahajan, Hossam Isack, Abhishek Kar, Andrea Tagliasacchi, Kwang Moo Yi
YouTube-ASL: A Large-Scale, Open-Domain American Sign Language-English Parallel Corpus Dave Uthus, Garrett Tanzer, Manfred Georg
The Noise Level in Linear Regression with Dependent Data Ingvar Ziemann, Stephen Tu, George J. Pappas, Nikolai Matni
#2023#3d#ai#algorithm#amp#Analysis#android#applications#approach#Bias#Blog#box#Building#cascade#citizen science#collaborative#Collections#communication#Community#compression#computing#conference#continuous#conversational ai#cutting#data#datasets#decision making#DeepMind#Design
0 notes
Text
Researchers develop soft robot that shifts from land to sea with ease
Most robots cannot. But researchers at Carnegie Mellon University have created soft robots that can seamlessly shift from walking to swimming, for example, or crawling to rolling. “We were inspired by nature to develop a robot that can perform different tasks and adapt to its environment without adding actuators or complexity,” said Dinesh K. Patel, a post-doctoral fellow in the Morphing Matter Lab in the School of Computer Science’s Human-Computer Interaction Institute. “Our bistable actuator is simple, stable and durable, and lays the foundation for future work on dynamic, reconfigurable soft robotics.” The bistable actuator is made of 3D-printed soft rubber containing shape-memory alloy springs that react to electrical currents by contracting, which causes the actuator to bend. The team used this bistable motion to change the actuator or robot’s shape. Once the robot changes shape, it is stable until another electrical charge morphs it back to its previous configuration. “Matching how animals transition from walking to swimming to crawling to jumping is a grand challenge for bio-inspired and soft robotics,” said Carmel Majidi, a professor in the Mechanical Engineering Department in CMU’s College of Engineering. For example, one robot the team created has four curved actuators attached to the corners of a cellphone-sized body made of two bistable actuators. On land, the curved actuators act as legs, allowing the robot to walk. In the water, the bistable actuators change the robot’s shape, putting the curved actuators in an ideal position to act as propellers so it can swim. “You need to have legs to walk on land, and you need to have a propeller to swim in the water. Building a robot with separate systems designed for each environment adds complexity and weight,” said Xiaonan Huang, an assistant professor of robotics at the University of Michigan and Majidi’s former Ph.D. student. “We use the same system for both environments to create an efficient robot.” The team created two other robots: one that can crawl and jump, and one inspired by caterpillars and pill bugs that can crawl and roll. The actuators require only a hundred millisecond of electrical charge to change their shape, and they are durable. The team had a person ride a bicycle over one of the actuators a few times and changed their robots’ shapes hundreds of times to demonstrate durability. In the future, the robots could be used in rescue situations or to interact with sea animals or coral. Using heat-activated springs in the actuators could open up applications in environmental monitoring, haptics, and reconfigurable electronics and communication. “There are many interesting and exciting scenarios where energy-efficient and versatile robots like this could be useful,” said Lining Yao, the Cooper-Siegel Assistant Professor in HCII and head of the Morphing Matter Lab. The team’s research, “Highly Dynamic Bistable Soft Actuator for Reconfigurable Multimodal Soft Robots,” was featured on the cover of the January 2023 issue of Advanced Materials Technologies. The research team included co-first authors Patel and Huang; Yao; Majidi; Yichi Luo, a mechanical engineering master’s student at CMU; and Mrunmayi Mungekar and M. Khalid Jawed, both from the Department of Mechanical and Aerospace Engineering at the University of California, Los Angeles.
0 notes
Photo

有趣分享 Fun to share 回顧2008年金融海嘯,道指期貨由2007年10月11日最高點的14,267點,跌至2009年3月6日的最低點的6,460點,跌幅為54.7%。十一年後,道指期貨由2020年2月13日最高點的29,543點開始計算,跌幅將會是多少?假設仍是54.7%的話,終點將為16,166點了!!! 以上提供的資料、數據、分析及意見 (1) 並不構成任何投資建議;(2) 僅提供作參考用途;(3) 並未就所載資料的完整性、準確性及時間性作出任何保證���對於閣下使用任何相關資料而作出的任何有關交易決定、傷害及其他損失均不承擔任何責任。 歡迎轉載,請列明出處。 According to the financial tsunami in the years among 2008, the Dow futures fell from 14,267 points, the highest point on October 11, 2007, to 6,460 points, the lowest point on March 6, 2009, a drop of 54.7%. After 11 years, the Dow futures started to fall from 29,543, the highest point on February 13, 2020. What will the goal be? Assuming the drop percentage is still 54.7%, the target would be 16,166 points!!! The information, data, analysis and opinions provided above (1) do not constitute any investment advice; (2) are provided for reference only; (3) no guarantee is given as to the completeness, accuracy and timeliness of the information . We will not be responsible for any related transaction decisions, injuries and other losses made by you using any relevant information. Welcome for your forwarding. Please specify the source. #2017 #2018 #2019 #2020 #一持 #馬一持 #玄學 #八字 #風水 #奇門遁甲 #私人教學 #術數 #紫微斗數 #香港 #道指 #yichi #yichi8998 #ichingtarot #eightwords #fengshui #qmdj #qimendunjia #ziweidoushu #ZiBai #XuanKong #hongkong #DowJones https://www.instagram.com/p/B9qmmeXpUsh/?igshid=dfhrppmptm19
#2017#2018#2019#2020#一持#馬一持#玄學#八字#風水#奇門遁甲#私人教學#術數#紫微斗數#香港#道指#yichi#yichi8998#ichingtarot#eightwords#fengshui#qmdj#qimendunjia#ziweidoushu#zibai#xuankong#hongkong#dowjones
0 notes
Text
LINE WALKER 2: INVISIBLE SPY (2019) ★★★★☆
LINE WALKER 2: INVISIBLE SPY (2019) ★★★★☆
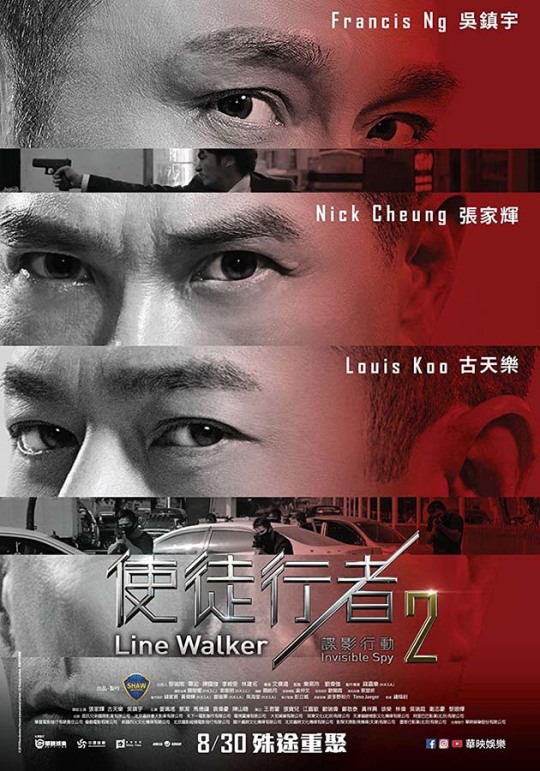
View On WordPress
#Benjamin Yuen#Francis Ng#Huang Zhizhong#Jazz Boon#Jiang Peiyao#Joe Ma#Joel Chan#Liu Yuning#Louis Koo#Nick Cheung#Zhang Yichi
0 notes
Photo

© 覃俊毅
#ANTICIPATE#idol producer#qin junyi#li rang#lou zibo#wu lianjie#zhang yichi#li zichen#su zhiheng#omg thanks to zhutwns!! I found out their names c:
29 notes
·
View notes
Text
Account of the Braided Bandits (SS095)
On one side of the River, Daxi Jin and Lord Black-Lance. On the other side at the Tiger's Pen, Mao Dezu.
The Braided Heads Bandits have the family name Tuoba. Their ancestors were descendants of the Han general Li Ling. Ling surrendered to the Xiongnu and had several hundreds and thousands of offspring, each one established fame and reputation. The Braided Heads likewise are one of them. At the beginning of Jin, the Braided Heads kind had their section groups of several ten thousand families in Yunzhong.
At the end of Emperor Hui's regin, the Inspector of Bing province, the Duke of Dongying, Sima Teng, was besieged by the Xiongnu at Jinyang. The Shanyu of the Braided Heads, Yichi, dispatched an army to help Teng.
Emperor Huai's 3rd Year of Yongjia [309 AD], Chi's younger brother Lu led the section groups from Yunzhong to enter Yanmen. He went to the Inspector of Bing province, Liu Kun, to press for Loufan etc., five counties. Kun was not able to hold authority, and moreover wished to depend on Lu for aid. He therefore sent up words:
Lu's older brother Chi had merit in saving Teng. Old achievements ought to be recorded. [I] request to move the people of the five counties to Xinxing, and use their land to settle him.
Kun also petitioned to ennoble Lu as Duke of Dai commandery.
At the beginning of Emperor Min's regin, he again advanced Lu to be King of Dai, and added revenue from Changshan commandery. Afterwards within Lu's state there was great chaos, and when Lu died, his son was also young and immature. The section groups divided and scattered.
Lu's grandson Shiyijian was brave and strong, the multitudes then adhered to him. He was titled Duke of Shangluo, to the north he had the Sand Desert, to the south he occupied Yin Mountain, his multitudes numbered several hundred thousands. Afterwards he was routed by Fu Jian, who took him back to Chang'an. Later he was allowed to return north.
[Shiyi]jian died, his son Kai, courtesy name Shegui, was installed in replacement. Before this, Murong Chui of the Xianbei usurped the title in Zhongshan. Xiaowu of Jin's 21st Year of Taiyuan [396 AD], Chui died. Kai led 100 000 cavalry to besiege Zhongshan. Next Year, 4th Month [13 May – 11 June 397], he overcame it. Thereupon he ruled the central provinces, declared himself as Wei, and titled the year Tianci [“Heaven's Bestowal”]. 1st Year, he set his seat at Pingcheng in Dai commandery's Sangqian county, established schools and officials, and set up boards of the Masters of Writing.
Kai was quite learned and informed, and comprehended astronomy. His customs was to sacrifice to Heaven in the 4th Month, and at the end of the 6th Month lead a great multitude to Yin Mountain. He spoke of it as turning back the frost. The distance between Yin Mountain and Pingcheng is 600 li. There is deep, far-reaching and rich forest, and frost and snow have never once melted away, perhaps he intended to use warm air to turn back the cold.
When he died, he was secretly buried, without a place for the grave mound. Reaching the seeing off of the burial, they had both emptily built an inner coffin and erected a barrow and outer coffin. All of the chariots, horses, and implements he had made use of while alive they burned to see off the perished.
Kai was violent, cruel and fond of killing, the people could not bear his instructions. Before this, there was a spirit magician who warned Kai he would have a violent misfortune, only by executing Qinghe and killing ten thousand people could it be avoided. Kai therefore wiped out Qinghe, one commandery. He often killed people with his own hand, wishing to make it number a full ten thousand. Sometimes, he would drive a small carriage, and with his own hand hold the sword [and?] strike the carriage rim at people's brain. When one person died, another person replaced them, all in one action. The dead were several tens. At night he constantly changed and altered the place where he slept, so that people did not know. Only a loved concubine named Wanren [“Ten thousand Persons”] knew about his location. Wanren had secret intercourse with Kai's son, the King of Qinghe. He worried the affair would become known and wished to kill Kai. He made Wanren his inner agent. At night they waited until Kai was alone at the place, and killed him. As Kai was approaching death, he said:
The talk about Qinghe and ten thousand people then were about you.
That year was Emperor An's 5th Year of Yixi [409 AD].
Kai's second son, the King of Qi, Si, courtesy name Mumo, apprehended the King of Qinghe, and responded to him with shouting and weeping, saying:
The weightiest in a person's life is the father. Why are you talking of making rebellion?
He pressured and made him kill himself. Si was installed in replacement. He posthumously titled Kai as the Guiding and Martial [daowu] August Emperor.
13th Year [417 AD], Gaozu went west to attack Chang'an. Si had previously taken as wife a daughter of Yao Xing, and therefore dispatched 100 cavalry to gather and join up north of the He to save him. They were greatly routed by Gaozu, the affair is in the biographies of Zhu Chaoshi and others. Hence he dispatched envoys to seek peace, and from then envoys and instructions passed through yearly. Gaozu dispatched the General Within the Halls, Shen Fan, Suo Jisun as responding envoys. They were already returning from instructing and had reached the He but not yet crossed, when Si heard the news of Gaozu's collapse. He pursued and apprehended Fan and others, and cut of peaceful relations. Only when Taizu was enthroned did he dispatch Fan and others back home.
3rd Year of Yongchu, 10th Month [31 October 422 – 29 November 422], Si himself led a multitude to arrive at Fangcheng. He dispatched the General of Zheng Troops and Inspector of Yang province, the Duke of Shanyang, Daxi Jin, the General of Wu Troops and Inspector of Guang province, the Duke of Cangwu, Gongsun Biao, and the Master of Writing Hua Ji, to lead more than 20 000 infantry and cavalry, cross south south-west of Huatai at Shiji [the “Stone Crossing”] on the border of Dongyan county, with the supply wagons, the weak and tired, accompanying himself.
The Defence Master of Huatai, General who Soothes the Distant and Grand Warden of Dong commandery, Wang Jingdu, hurried to report to the General of the Best of the Army and Inspector of Si province, Mao Dezu. He defended Hulao, and dispatched Marshal Zhai Guang to lead the Army Advisor Pang Zi, Grand Warden of Shangdang, Liu Tanzhi, and others with 3 000 infantry and cavalry to resist them.
The army stayed at Tulou in Juan county. The bandits moved camp to two li east of Huatai City. They constructed assault implements and went daily to threaten the city. Dezu, since the defenders of Huatai were few, made Zhai Guang recruit strong soldiers among the army, and dispatched the General who Soothes the Distant, Liu Fangzhi, to lead them, and help Jingdu with the defence. Fangzhi brought along more than 80 people, and broke through to enter the city.
Dezu also dispatched the General who Chastise the Bandits and Grand Warden of Hongnong, Dou Yingming, leading 500 people, and the General who Establishes the Martial, Dou Ba, leading 250 people. Both were to use water forces and succeed each other in issuing out, and would together be under the authority of Zhai Guang.
Earlier, the fugitive Sima Chuzhi and others would often hide and conceal themselves on the borders of Chenliu commandery. When the bandits had crossed south, they hurried to join up with them. They chased away and fomented in the border areas, and greatly became a worry for the people. Dezu dispatched the Prefect of Changshe, Wang Fazheng, to lead 500 people and occupy Shaoling, while general Liu Lian led 200 cavalry to reach Yongiu and defend it. Chuzhi assaulted Lian at Baima county, and was routed by Lian. By chance army supplies sent off from the palace arrived, and Lian went to welcome them, but a commoner from Suanzao, Wang Yu, knew that Lian was to the south, and hurried to report to the bandits. The bandit general Hua Ji led a thousand to drive a raid on Cangyuan, the troops and personnel fully went over the walls to scatter and flee. The Grand Warden of Chenliu, Yan Man, was captured by the bandits. The bandits immediately employed Wang Yu as Grand Warden of Chenliu, controlling the troops defending Cangyuan.
11th Month [30 November – 28 December 422], the bandits attacked the walls of Huatai with full strength. The north-eastern walls collapsed into ruin, and Wang Jingdu set out and ran. Jingdu's Marshal Yang Zan stood firm in defence, and did not move. [Though] the multitudes dispersed, he was unyielding and steadfast and did not surrender, and was killed by the bandits.
Dou Yingming struck the bandits' supply wagons at Shiji, and routed them. He killed more than 500 of the thieves, and beheaded their Defence Masters [lacuna]-lian Neitou, Zhang Suo'er and others. Yingming from Shiji proceeded to Huatai, heard the city was already lost, and thereupon advanced to station at Yinmao. Dou Ba hurried to go to Zhai Guang.
When the bandits had overcome Huatai, they combined their strength towards Guang and others. His strength was no match, he pulled back and withdrew, turned to fight and then went forward. For two days and one night, he cut down travel to ten or so li. The bandits' infantry armies continuously arrived. Guang and others' arrows were exhausted and their strength at an end, they were greatly defeated. Guang, Ba, Tanzhi, and others each dispersed on their own and turned back. The bandits exploited the victory to then arrive at Hulao. Dezu set out with infantry and cavalry intending to strike them. The bandits withdrew and stationed at Tulao, and again withdrew to turn back to Huatai.
The people of Chang'an, Weichang, and Lantian counties lived beside Hulao. Dezu in all cases made them enter the city. The bandits separately dispatched Lord Black-Lance to lead 3 000 people to Heyang, intending to cross south and capture Jinyong. Dezu dispatched the General who Rouses Power and Prefect of Heyin, Dou Huang, with 500 people to defend Xiaolei [lit. “small ramparts”], the Prefect of Goushi, Wang Yu, with 400 people to occupy Jiancang, the Prefect of Gong, Chen Chen, with 500 people to strengthen Xiaoping, and the Army Advisor Supervising Protector, Zhang Ji, to station at Niulan. He also dispatched the general and leader Ma Dui [?], together with the Prefect of Luoyang, Yang Yi, a combined 200 cavalry, to hem the banks of the He and follow the moment to go and link up.
12th Month [29 December 422 – 27 January 423], the bandits set up defences at the Luo Stream's small ramparts. Dezu dispatched Zhai Guang to hurriedly go and strike them. The bandits withdrew and fled. Guang calmly erected defensive dikes, repaired and organized the walls and fortifications, and then turned back to Hulao.
The Inspector of Yu province, Liu Cui, dispatched the [Assistant at] Headquarters Gao Daojin, to lead 500 infantry and cavalry to occupy Xiang. He also dispatched Marshal Xu Qiong to support him. The palace dispatched generals Fu Boqian, Yao Zhen, Du Tan, Liang Lingzai, and others with various naval and infantry forces to carry on the advance. The Inspector of Xu province, Wang Zhongde, led an army to stay at Hulu.
Lord Black-Lance dispatched his Senior Clerk to bring along 1 000 people to pressure Dou Huang and Yang Yi. Huang and others confronted, struck, and seized him, capturing alive 200 people. Afterwards, the General of Zheng Troops with 5 000 cavalry unexpectedly assaulted Huang and others. Black-Lance crossed and combined strength with him, and they attacked the ramparts on four sides. Huang and others' strength was little and their multitudes scattered. Huang and Yi both were heavily wounded.
The bandits' general, the Duke of Anping, E Qing, crossed south with two armies of 7 000 people, east below of Que'ao and arrived at Sidoukou, about 100 li from Yinmao. The Inspector of Yan province, Xu Yan, abandoned the army and garrisons, and fled. Hence Taishan and other commanderies equally neglected defences.
Zheng Troops, together with Gongsun Bao and the General of Song Troops and Inspector of Yan province, the Marquis of Jiaozhi, Pu Ji, with 15 000 cavalry then went towards Hulao. They formed camp 5 li south-east of the city, and divided off infantry and cavalry unfolding [?] from Chenggao towards the western gate in Hulao's outer walls. Dezu confronted and struck them, killing and wounding more than a hundred people. The bandits withdrew to protect the camp.
The General who Garrisons the North, Tan Daoji, led a navy north to rescue. The General of Chariots and Cavalry, the King of Luling, Yizhen, dispatched the Dragon-Prancing General, Shen Shuli, with 3 000 people to go to the Inspector of Yu province, Liu Cui, to measure the suitability of hurrying aid[?].
9 notes
·
View notes
Text

A Queen Band picture from the day they were guests at "Star Sen Yichi Ya" in Japan.
Thanks to _letusclingtogether_ on Instagram for sharing.
#queen#freddie mercury#roger taylor#brian may#john deacon#queen band#japan#1975#70s#my post#my stuff
58 notes
·
View notes