#using ai to match patients with clinical trials
Explore tagged Tumblr posts
Text
https://kitsa.ai/the-future-is-now-ai-innovations-in-the-pharmaceutical-industry/
#ai in clinical trials#ai in pharma#clinical trial companies#clinical trials recruitment ai#accelerating clinical trials#how ai is transforming clinical trials#using ai to match patients with clinical trials#clinical trials blogs#becoming a principal investigator
0 notes
Text
How Can You Ensure Data Quality in Healthcare Analytics and Management?
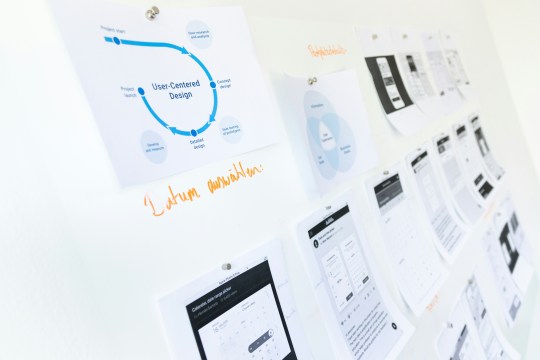
Healthcare facilities are responsible for the patient’s recovery. Pharmaceutical companies and medical equipment manufacturers also work toward alleviating physical pain, stress levels, and uncomfortable body movement issues. Still, healthcare analytics must be accurate for precise diagnosis and effective clinical prescriptions. This post will discuss data quality management in the healthcare industry.
What is Data Quality in Healthcare?
Healthcare data quality management includes technologies and statistical solutions to verify the reliability of acquired clinical intelligence. A data quality manager protects databases from digital corruption, cyberattacks, and inappropriate handling. So, medical professionals can get more realistic insights using data analytics solutions.
Laboratories have started emailing the test results to help doctors, patients, and their family members make important decisions without wasting time. Also, assistive technologies merge the benefits of the Internet of Things (IoT) and artificial intelligence (AI) to enhance living standards.
However, poor data quality threatens the usefulness of healthcare data management solutions.
For example, pharmaceutical companies and authorities must apply solutions that remove mathematical outliers to perform high-precision data analytics for clinical drug trials. Otherwise, harmful medicines will reach the pharmacist’s shelf, endangering many people.
How to Ensure Data Quality in the Healthcare Industry?
Data quality frameworks utilize different strategies to prevent processing issues or losing sensitive intelligence. If you want to develop such frameworks to improve medical intelligence and reporting, the following 7 methods can aid you in this endeavor.
Method #1| Use Data Profiling
A data profiling method involves estimating the relationship between the different records in a database to find gaps and devise a cleansing strategy. Data cleansing in healthcare data management solutions has the following objectives.
Determine whether the lab reports and prescriptions match the correct patient identifiers.
If inconsistent profile matching has occurred, fix it by contacting doctors and patients.
Analyze the data structures and authorization levels to evaluate how each employee is accountable for specific patient recovery outcomes.
Create a data governance framework to enforce access and data modification rights strictly.
Identify recurring data cleaning and preparation challenges.
Brainstorm ideas to minimize data collection issues that increase your data cleaning efforts.
Ensure consistency in report formatting and recovery measurement techniques to improve data quality in healthcare.
Data cleaning and profiling allow you to eliminate unnecessary and inaccurate entries from patient databases. Therefore, healthcare research institutes and commercial life science businesses can reduce processing errors when using data analytics solutions.
Method #2| Replace Empty Values
What is a null value? Null values mean the database has no data corresponding to a field in a record. Moreover, these missing values can skew the results obtained by data management solutions used in the healthcare industry.
Consider that a patient left a form field empty. If all the care and life science businesses use online data collection surveys, they can warn the patients about the empty values. This approach relies on the “prevention is better than cure” principle.
Still, many institutions, ranging from multispecialty hospitals to clinical device producers, record data offline. Later, the data entry officers transform the filled papers using scanners and OCR (optical character recognition).
Empty fields also appear in the database management system (DBMS), so the healthcare facilities must contact the patients or reporting doctors to retrieve the missing information. They use newly acquired data to replace the null values, making the analytics solutions operate seamlessly.
Method #3| Refresh Old Records
Your physical and psychological attributes change with age, environment, lifestyle, and family circumstances. So, what was true for an individual a few years ago is less likely to be relevant today. While preserving historical patient databases is vital, hospitals and pharma businesses must periodically update obsolete medical reports.
Each healthcare business maintains a professional network of consulting physicians, laboratories, chemists, dietitians, and counselors. These connections enable the treatment providers to strategically conduct regular tests to check how patients’ bodily functions change throughout the recovery.
Therefore, updating old records in a patient’s medical history becomes possible. Other variables like switching jobs or traveling habits also impact an individual’s metabolism and susceptibility to illnesses. So, you must also ask the patients to share the latest data on their changed lifestyles. Freshly obtained records increase the relevance of healthcare data management solutions.
Method #4| Standardize Documentation
Standardization compels all professionals to collect, store, visualize, and communicate data or analytics activities using unified reporting solutions. Furthermore, standardized reports are integral to improving data governance compliance in the healthcare industry.
Consider the following principles when promoting a documentation protocol to make all reports more consistent and easily traceable.
A brand’s visual identities, like logos and colors, must not interfere with clinical data presentation.
Observed readings must go in the designated fields.
Both the offline and online document formats must be identical.
Stakeholders must permanently preserve an archived copy of patient databases with version control as they edit and delete values from the records.
All medical reports must arrange the data and insights to prevent ambiguity and misinterpretation.
Pharma companies, clinics, and FDA (food and drug administration) benefit from reporting standards. After all, corresponding protocols encourage responsible attitudes that help data analytics solutions avoid processing problems.
Method #5| Merge Duplicate Report Instances
A report instance is like a screenshot that helps you save the output of visualization tools related to a business query at a specified time interval. However, duplicate reporting instances are a significant quality assurance challenge in healthcare data management solutions.
For example, more than two nurses and one doctor will interact with the same patients. Besides, patients might consult different doctors and get two or more treatments for distinct illnesses. Such situations result in multiple versions of a patient’s clinical history.
Data analytics solutions can process the data collected by different healthcare facilities to solve the issue of duplicate report instances in the patients’ databases. They facilitate merging overlapping records and matching each patient with a universally valid clinical history profile.
Such a strategy also assists clinicians in monitoring how other healthcare professionals prescribe medicine to a patient. Therefore, they can prevent double dosage complications arising from a patient consuming similar medicines while undergoing more than one treatment regime.
Method #6| Audit the DBMS and Reporting Modules
Chemical laboratories revise their reporting practices when newly purchased testing equipment offers additional features. Likewise, DBMS solutions optimized for healthcare data management must receive regular updates.
Auditing the present status of reporting practices will give you insights into efficient and inefficient activities. Remember, there is always a better way to collect and record data. Monitor the trends in database technologies to ensure continuous enhancements in healthcare data quality.
Simultaneously, you want to assess the stability of the IT systems because unreliable infrastructure can adversely affect the decision-making associated with patient diagnosis. You can start by asking the following questions.
Questions to Ask When Assessing Data Quality in Healthcare Analytics Solutions
Can all doctors, nurses, agents, insurance representatives, patients, and each patient’s family members access the required data without problems?
How often do the servers and internet connectivity stop functioning correctly?
Are there sufficient backup tools to restore the system if something goes wrong?
Do hospitals, research facilities, and pharmaceutical companies employ end-to-end encryption (E2EE) across all electronic communications?
Are there new technologies facilitating accelerated report creation?
Will the patient databases be vulnerable to cyberattacks and manipulation?
Are the clinical history records sufficient for a robust diagnosis?
Can the patients collect the documents required to claim healthcare insurance benefits without encountering uncomfortable experiences?
Is the presently implemented authorization framework sufficient to ensure data governance in healthcare?
Has the FDA approved any of your prescribed medications?
Method #7| Conduct Skill Development Sessions for the Employees
Healthcare data management solutions rely on advanced technologies, and some employees need more guidance to use them effectively. Pharma companies are aware of this as well, because maintaining and modifying the chemical reactions involved in drug manufacturing will necessitate specialized knowledge.
Different training programs can assist the nursing staff and healthcare practitioners in developing the skills necessary to handle advanced data analytics solutions. Moreover, some consulting firms might offer simplified educational initiatives to help hospitals and nursing homes increase the skill levels of employees.
Cooperation between employees, leadership, and public authorities is indispensable to ensure data quality in the healthcare and life science industries. Otherwise, a lack of coordination hinders the modernization trends in the respective sectors.
Conclusion
Healthcare analytics depends on many techniques to improve data quality. For example, cleaning datasets to eliminate obsolete records, null values, or duplicate report instances remains essential, and multispecialty hospitals agree with this concept.
Therefore, medical professionals invest heavily in standardized documents and employee education to enhance data governance. Also, you want to prevent cyberattacks and data corruption. Consider consulting reputable firms to audit your data operations and make clinical trials more reliable.
SG Analytics is a leader in healthcare data management solutions, delivering scalable insight discovery capabilities for adverse event monitoring and medical intelligence. Contact us today if you want healthcare market research and patent tracking assistance.
3 notes
·
View notes
Text
Genomic Contextualization: Personalized Treatment Protocols in Modern Internal Medicine
Introduction
Genomic contextualization is a cornerstone of modern internal medicine, enabling the development of personalized treatment protocols that are tailored to an individual's unique genetic profile. This approach leverages advances in genomic technologies, bioinformatics, and data analytics to provide precise and effective healthcare. Here, we delve into the concept of genomic contextualization and its implications for personalized treatment protocols in internal medicine.
The Role of Genomics in Personalized Medicine
Genomics plays a pivotal role in personalized medicine by providing a detailed understanding of an individual's genetic makeup. This information can be used to predict disease susceptibility, diagnose conditions accurately, and select the most effective treatments. The Human Genome Project and subsequent genomic initiatives have laid the foundation for this approach, enabling the identification of genetic variants associated with various diseases.
Whole exome sequencing (WES) and targeted sequencing are particularly useful in clinical practice, as they balance cost and benefit by focusing on protein-coding regions of the genome. These techniques have been integrated into clinical genetics laboratories and large-scale projects such as the 1000 Genome Project and the Exome Aggregation Consortium (ExAC) to catalog population variants and identify diseases associated with rare genetic variants.
Genomic Contextualization and Disease Management
Genomic contextualization involves correlating an individual's genetic profile with clinical-pathological indexes to devise personalized diagnostic, prognostic, and therapeutic strategies. This approach is particularly beneficial in managing complex diseases such as cancer, diabetes, and endocrine disorders.
In oncology, for example, precision medicine involves identifying oncogenes and tumor suppressor genes to design targeted therapies. Multi-regional sequencing of tumors reveals genetic heterogeneity, allowing for the selection of patients who may benefit from biomarker-driven therapies. Studies like the NCI MATCH trial and the TAPUR study demonstrate the potential of this approach in improving patient outcomes by matching genetic aberrations with targeted therapies.
Pharmacogenomics and Personalized Treatment
Pharmacogenomics, a subfield of precision medicine, studies how genetic variations affect an individual's response to medications. This knowledge enables healthcare providers to select medications and adjust dosages based on a patient's genetic makeup, maximizing therapeutic benefits while minimizing adverse reactions. For instance, in diabetes management, pharmacogenomics can guide the use of metformin and other antidiabetic drugs, ensuring optimal efficacy and safety.
Integration of Advanced Technologies and Data Analytics
The integration of advanced technologies such as next-generation sequencing (NGS), machine learning, and artificial intelligence (AI) is crucial for genomic contextualization. These tools facilitate large-scale genomic sequencing, predictive modeling, and the analysis of vast datasets to identify disease patterns and predict treatment responses.
Machine learning algorithms can analyze genomic data in conjunction with clinical and environmental factors to develop personalized treatment algorithms. Telehealth platforms and digital health applications enable real-time monitoring of patient health metrics, medication adherence, and lifestyle modifications, further enhancing patient-centered care.
Ethical and Regulatory Considerations
While genomic contextualization offers significant benefits, it also raises ethical and regulatory concerns. Ensuring patient data privacy and security is paramount, given the sensitive nature of genetic information. Regulatory frameworks must be established to govern the use of genomic data in healthcare, ensuring compliance with laws such as HIPAA and preventing the misuse of genetic information.
Future Directions and Challenges
The future of genomic contextualization in internal medicine is promising but also presents several challenges. There is a need for continued research to refine these approaches and address issues such as data quality, class imbalance, and the integration of diverse data sources.
Expanding genomic research, biomarker discovery, and therapeutic innovations will be essential for enhancing treatment efficacy and disease prevention. Additionally, educating healthcare professionals in genomics and precision medicine will be critical for the widespread adoption and effective implementation of these personalized treatment protocols.
Conclusion
Genomic contextualization is revolutionizing internal medicine by enabling the development of personalized treatment protocols that are tailored to an individual's unique genetic profile. By integrating genomic information with advanced technologies and data analytics, healthcare providers can offer precise and effective care, improving patient outcomes and enhancing the quality of life for individuals with complex medical conditions. As this field continues to evolve, addressing ethical and regulatory concerns while ensuring the accuracy and reliability of genomic data will be essential for maximizing the benefits of personalized medicine.
0 notes
Text
The Future of Clinical Trials: Integrating AI with Clinical SAS for Better Insights
As the healthcare landscape evolves, the integration of Artificial Intelligence (AI) with Clinical SAS is poised to revolutionize clinical trials, enhancing efficiency and improving outcomes. This synergy holds the promise of transforming how we conduct clinical research, analyze data, and ultimately bring new therapies to market.
Understanding Clinical SAS and Its Importance
Clinical SAS is a specialized programming language used to manage and analyze clinical trial data. It plays a critical role in ensuring the accuracy, integrity, and compliance of data submissions to regulatory agencies like the FDA. SAS programmers are responsible for data manipulation, statistical analysis, and generating reports that summarize trial outcomes.
However, the sheer volume of data generated in clinical trials can be overwhelming. Traditional data analysis methods may struggle to keep pace with this influx, making the integration of AI a vital consideration.
The Role of AI in Clinical Trials
AI technologies, including machine learning and natural language processing, have the potential to enhance various aspects of clinical trials:
Data Management and Cleaning: AI can automate the data cleaning process, identifying inconsistencies, missing values, and outliers in clinical datasets. By reducing the manual effort involved in data preparation, researchers can focus more on analysis and interpretation.
Predictive Analytics: Machine learning algorithms can analyze historical clinical trial data to identify trends and predict patient outcomes. This capability can inform trial design, optimize patient recruitment strategies, and improve the likelihood of success for new therapies.
Patient Recruitment and Retention: AI can analyze electronic health records and other data sources to identify eligible patients for clinical trials. By matching patients with appropriate studies, AI can streamline recruitment processes and enhance retention strategies, ensuring that trials remain adequately powered.
Real-Time Monitoring: AI enables real-time monitoring of clinical trial data, allowing for timely adjustments to protocols based on emerging trends. This adaptability can enhance patient safety and data integrity, leading to more reliable results.
Data Visualization and Reporting: AI-driven analytics tools can create dynamic visualizations of clinical trial data, making it easier for stakeholders to interpret findings. Enhanced reporting capabilities can facilitate quicker decision-making and more effective communication with regulatory agencies.
The Synergy of AI and Clinical SAS
Integrating AI with Clinical SAS offers a powerful combination that can elevate clinical research. Here’s how they work together:
Enhanced Analytical Capabilities: By incorporating AI algorithms into SAS programming, clinical researchers can automate complex analyses and derive deeper insights from their data. This integration can lead to more robust statistical models and improved interpretation of results.
Improved Workflow Efficiency: Automating repetitive tasks within Clinical SAS using AI can significantly reduce the time and effort required for data analysis. This efficiency enables researchers to allocate resources more effectively, ultimately accelerating the drug development process.
Informed Decision-Making: The insights derived from AI-enhanced data analysis can inform decision-making at every stage of a clinical trial, from design to execution. By leveraging predictive analytics, researchers can make proactive adjustments to trial protocols based on real-time data, increasing the likelihood of success.
Challenges and Considerations
While the integration of AI and Clinical SAS presents significant opportunities, there are also challenges to address:
Data Quality and Integrity: The effectiveness of AI algorithms relies heavily on the quality of the data being analyzed. Ensuring data integrity is paramount to avoid erroneous conclusions.
Regulatory Compliance: The use of AI in clinical trials must align with regulatory requirements. Researchers need to ensure that AI-driven insights are interpretable and can withstand regulatory scrutiny.
Skills Gap: There may be a skills gap in the workforce regarding the application of AI in clinical research. Training and education will be crucial to equip SAS programmers with the knowledge needed to leverage AI effectively.
Conclusion
The future of clinical trials is bright, with the integration of AI and Clinical SAS paving the way for more efficient, insightful, and effective research. By embracing these technologies, clinical researchers can enhance their ability to analyze data, improve patient outcomes, and expedite the drug development process. As we move forward, it will be essential to navigate the challenges and harness the full potential of AI in clinical SAS to drive innovation in clinical trials and ultimately improve healthcare for all.
0 notes
Text
Unlocking New Possibilities in Healthcare with AI
New Post has been published on https://thedigitalinsider.com/unlocking-new-possibilities-in-healthcare-with-ai/
Unlocking New Possibilities in Healthcare with AI
Healthcare in the United States is in the early stages of a significant potential disruption due to the use of Machine Learning and Artificial Intelligence. This shift has been underway for over a decade, but with recent advances, seems poised for more rapid changes. Much work remains to be done to understand the safest and most effective applications of AI in healthcare, to build trust among clinicians in the use of AI, and to adjust our clinical education system to drive better use of AI-based systems.
Applications of AI in Healthcare
AI has been in evolution for decades in healthcare, both in patient-facing and back-office functions. Some of the earliest and most extensive work has occurred in the use of deep learning and computer vision models.
First, some terminology. Traditional statistical approaches in research–e.g. observational studies and clinical trials–have used population-focused modeling approaches that rely on regression models, in which independent variables are used to predict outcomes. In these approaches, while more data is better, there is a plateau effect in which above a certain data set size, no better inferences can be obtained from the data.
Artificial intelligence brings a newer approach to prediction. A structure called a perceptron processes data that is passed forward a row at a time, and is created as a network of layers of differential equations to modify the input data, to produce an output. During training, each row of data as it passes through the network–called a neural network–modifies the equations at each layer of the network so that the predicted output matches the actual output. As the data in a training set is processed, the neural network learns how to predict the outcome.
Several types of networks exist. Convolutional neural networks, or CNNs, were among the first models to find success in healthcare applications. CNNs are very good at learning from images in a process called computer vision and have found applications where image data is prominent: radiology, retinal exams, and skin images.
A newer neural network type called the transformer architecture has become a dominant approach due to its incredible success for text, and combinations of text and images (also called multimodal data). Transformer neural networks are exceptional when given a set of text, at predicting subsequent text. One application of the transformer architecture is the Large Language Model or LLM. Multiple commercial examples of LLMs include Chat GPT, Anthropics Claude, and Metas Llama 3.
What has been observed with neural networks, in general, is that a plateau for improvement in learning has been hard to find. In other words, given more and more data, neural networks continue to learn and improve. The main limits on their capability are larger and larger data sets and the computing power to train the models. In healthcare, the creation of privacy-protecting data sets that faithfully represent true clinical care is a key priority to advance model development.
LLMs may represent a paradigm shift in the application of AI for healthcare. Because of their facility with language and text, they are a good match to electronic records in which almost all data are text. They also do not require highly annotated data for training but can use existing data sets. The two main flaws with these models are that 1) they do not have a world model or an understanding of the data that is being analyzed (they have been called fancy autocomplete), and 2) they can hallucinate or confabulate, making up text or images that appear accurate but create information presented as fact.
Use cases being explored for AI include automation and augmentation for reading of radiology images, retinal images, and other image data; reducing the effort and improving the accuracy of clinical documentation, a major source of clinician burnout; better, more empathic, patient communication; and improving the efficiency of back-office functions like revenue cycle, operations, and billing.
Real-world Examples
AI has been incrementally introduced into clinical care overall. Typically, successful use of AI has followed peer-reviewed trials of performance that have demonstrated success and, in some cases, FDA approval for use.
Among the earliest use cases in which AI performs well have been AI detecting disease in retinal exam images and radiology. For retinal exams, published literature on the performance of these models has been followed by the deployment of automated fundoscopy to detect retinal disease in ambulatory settings. Studies of image segmentation, with many published successes, have resulted in multiple software solutions that provide decision support for radiologists, reducing errors and detecting abnormalities to make radiologist workflows more efficient.
Newer large language models are being explored for assistance with clinical workflows. Ambient voice is being used to enhance the usage of Electronic Health Records (EHRs). Currently, AI scribes are being implemented to aid in medical documentation. This allows physicians to focus on patients while AI takes care of the documentation process, improving efficiency and accuracy.
In addition, hospitals and health systems can use AI’s predictive modeling capabilities to risk-stratify patients, identifying patients who are at high or increasing risk and determining the best course of action. In fact, AI’s cluster detection capabilities are being increasingly used in research and clinical care to identify patients with similar characteristics and determine the typical course of clinical action for them. This can also enable virtual or simulated clinical trials to determine the most effective treatment courses and measure their efficacy.
A future use case may be the use of AI-powered language models in doctor-patient communication. These models have been found to have valid responses for patients that simulate empathetic conversations, making it easier to manage difficult interactions. This application of AI can greatly improve patient care by providing quicker and more efficient triage of patient messages based on the severity of their condition and message.
Challenges and Ethical Considerations
One challenge with AI implementation in healthcare is ensuring regulatory compliance, patient safety, and clinical efficacy when using AI tools. While clinical trials are the standard for new treatments, there is a debate on whether AI tools should follow the same approach. Another concern is the risk of data breaches and compromised patient privacy. Large language models trained on protected data can potentially leak source data, which poses a significant threat to patient privacy. Healthcare organizations must find ways to protect patient data and prevent breaches to maintain trust and confidentiality. Bias in training data is also a critical challenge that needs to be addressed. To avoid biased models, better methods to avoid bias in training data must be introduced. It is crucial to develop training and academic approaches that enable better model training and incorporate equity in all aspects of healthcare to avoid bias.
The use of AI has opened a number of new concerns and frontiers for innovation. Further study of where true clinical benefit may be found in AI use is needed. To address these challenges and ethical concerns, healthcare provider organizations and software companies must focus on developing data sets that accurately model healthcare data while ensuring anonymity and protecting privacy. Additionally, partnerships between healthcare providers, systems, and technology/software companies must be established to bring AI tools into practice in a safe and thoughtful manner. By addressing these challenges, healthcare organizations can harness the potential of AI while upholding patient safety, privacy, and fairness.
#ai#AI in healthcare#ai tools#AI-powered#ambient#applications#approach#architecture#artificial#Artificial Intelligence#autocomplete#automation#Bias#burnout#challenge#Chat GPT#claude#cluster#communication#Companies#compliance#computer#Computer vision#computing#course#courses#data#Data Breaches#decision support#Deep Learning
1 note
·
View note
Text
AI In Clinical Trials: Revolutionizing Clinical Trials How Artificial Intelligence (AI) Speeds Up Research And Discoveries

Clinical trials are a lengthy process, and one of the biggest hurdles is finding and screening eligible patients to participate. Artificial intelligence can help speed up this stage in important ways. AI-powered chatbots and voice assistants can pre-screen patients for eligibility based on medical history and demographic information. This initial screening using AI means researchers no longer have to manually review every single potential candidate. AI can also analyze Patient Health Record data and other sources to proactively identify patients that closely match study criteria. By flagging eligible prospects, AI reduces the time spent recruiting participants. Several startup companies now offer AI recruitment solutions that have helped clinical trials meet enrollment goals faster.
Protocol Design And AI In Clinical Trials
Designing clinical trial protocols and determining optimal drug dosing is as much an art as it is a science. AI and machine learning are giving researchers new tools to refine these processes based on historical trial data. AI in Clinical Trials by analyzing trends across many past studies, AI systems can predict the most promising treatment regimens and endpoint assessments for a new indication. This datadriven approach to protocol design aims to avoid wasting time and resources on options that are unlikely to succeed. AI is also being applied to dosage optimization, comparing toxin levels, side effects and outcomes across different dose amounts or schedules from previous trials. This enables researchers to select dosing that maximizes efficacy and safety from the beginning.
Endpoint Evaluation And Adverse Event Detection
Gathering and analyzing clinical trial endpoint data like lab results, symptom surveys and physician exams is a longtime manual process prone to errors and inconsistencies. AI can classify and extract meaningful insights from this wide variety of endpoint evidence much faster and more objectively than humans. Deep learning algorithms trained on retrospective endpoint data can also identify subtle patterns and adverse events that may have gone unnoticed previously. AI-powered safety monitoring may eventually allow for earlier intervention if worsening side effects are automatically flagged. This could lead to improved outcomes overall.
Predictive Analytics And Risk Mitigation
By combining protocol design insights with learnings from ongoing trials, AI offers predictive analytics to reduce risks. As patient endpoint information flows in, AI can spot troubling trends that may indicate futility and advise course corrections to trial leadership in real-time. Likewise, AI may foresee enrollee populations or sites that are behind on goals and could jeopardize trial integrity if not addressed preemptively. Rather than waiting until an analysis is scheduled, AI monitoring runs continuously to alert researchers proactively about potential problems. The ability to identify issues earlier allows for preventative mitigation that protects data quality and overall trial integrity.
Reporting And Results Analysis
The final analysis and reporting stage can also gain efficiency boosts from AI. Processing vast streams of endpoint data and safety monitoring results to generate required reports is laborious. AI excels at automating the aggregation and curation of clinical trial evidence into clear, standardized reports. By taking over routine reporting tasks, AI frees up researchers to focus on higher-level strategic analysis and writing. AI's number crunching further enhances results analysis by automatically surfacing unanticipated correlations between outcomes, subgroups and other variables in the trial datasets. Researchers can explore these emergent findings to develop new hypotheses for future study.
As these examples illustrate, artificial intelligence is capable of accelerating each step in the clinical trials process. By taking over routine and repetitive manual work, AI gives researchers more time for creative, strategic thinking that moves treatments to patients faster. Early adopters of AI for clinical research are reaping benefits today like reduced costs, quicker timelines and better outcomes. As the technology matures, its full potential to transform drug development through smarter trials will be realized.
Get more insights on this topic: https://www.pressreleasebulletin.com/ai-in-clinical-trials-how-artificial-intelligence-is-revolutionizing-clinical-trials/
Author Bio:
Alice Mutum is a seasoned senior content editor at Coherent Market Insights, leveraging extensive expertise gained from her previous role as a content writer. With seven years in content development, Alice masterfully employs SEO best practices and cutting-edge digital marketing strategies to craft high-ranking, impactful content. As an editor, she meticulously ensures flawless grammar and punctuation, precise data accuracy, and perfect alignment with audience needs in every research report. Alice's dedication to excellence and her strategic approach to content make her an invaluable asset in the world of market insights. (LinkedIn: www.linkedin.com/in/alice-mutum-3b247b137 )
*Note: 1. Source: Coherent Market Insights, Public sources, Desk research 2. We have leveraged AI tools to mine information and compile it
0 notes
Text
Revolutionizing Cancer Treatment: The Transformative Role of Artificial Intelligence in Oncology
The integration of artificial intelligence (AI) in oncology has revolutionized the way we approach cancer treatment, offering a beacon of hope for millions of patients worldwide. AI’s ability to analyze vast datasets and recognize complex patterns has led to significant advancements in personalized medicine, early detection, and treatment efficacy.
Personalized Treatment Plans One of the most promising applications of AI in oncology is the development of personalized treatment plans. By analyzing a patient’s genetic makeup, medical history, and lifestyle factors, AI algorithms can predict how different treatments will interact with their unique biology. This allows oncologists to tailor therapies that are more effective and have fewer side effects. For instance, AI systems like IBM Watson for Oncology have been trained to suggest treatment options by cross-referencing patient data against a vast array of medical literature and clinical guidelines. Early Detection and Diagnosis Early detection is crucial in the fight against cancer. AI-powered diagnostic tools can detect malignancies at stages when they are most treatable. Deep learning algorithms, a subset of AI, have been trained to interpret medical images such as MRIs and CT scans with a level of precision that matches or even surpasses human radiologists. These algorithms can identify subtle changes in tissue that may indicate the presence of cancer, often long before a patient exhibits any symptoms. Drug Development and Clinical Trials AI is also accelerating the pace of drug development and the design of clinical trials. By simulating how potential drugs interact with cancer cells, AI can help researchers identify promising compounds quickly, saving time and resources. Additionally, AI can optimize the selection of clinical trial participants by identifying individuals who are most likely to benefit from a particular treatment, thereby increasing the likelihood of successful trial outcomes. Predictive Analytics Predictive analytics is another area where AI shines in oncology. By analyzing patterns in large datasets, AI can predict patient outcomes, the likelihood of disease recurrence, and even potential complications. This information helps doctors make informed decisions about ongoing care and surveillance, potentially improving long-term survival rates. Challenges and Ethical Considerations Despite these advancements, the use of AI in oncology is not without challenges. Data privacy and security are paramount, as patient data must be protected from unauthorized access. There are also ethical considerations regarding the potential for AI to make decisions that affect patient care. It is essential that AI tools are used to support, not replace, the clinical judgment of healthcare professionals. Conclusion In conclusion, AI’s role in oncology is a testament to the incredible potential of technology to improve human health. As AI systems become more sophisticated, they will continue to enhance our ability to fight cancer, offering hope for more effective treatments and better patient outcomes. The future of oncology is one where AI and human expertise work hand in hand to conquer one of humanity’s most formidable adversaries. This narrative underscores the transformative impact of AI in oncology, highlighting its potential to personalize treatment, enhance early detection, expedite drug development, and provide predictive insights, all while navigating the challenges and ethical considerations inherent in its application. As we continue to harness AI’s capabilities, the horizon of cancer treatment and patient care is poised to expand dramatically, ushering in a new era of hope and healing. Meet Dr. Tim at LinkedIn: https://www.linkedin.com/in/timothy-r-allen-md-phd%F0%9F%87%BA%F0%9F%87%B8%F0%9F%8E%97%EF%B8%8F-54459b297/
1 note
·
View note
Text
AWS helps Owkin careers precision medicine generative AI
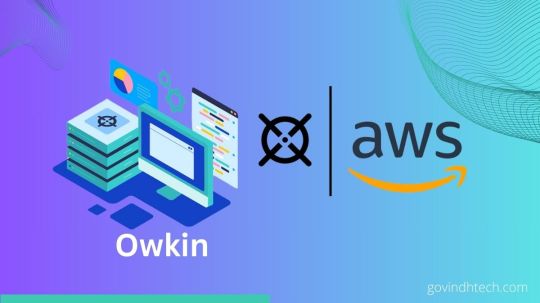
Owkin careers(Owkin), a tech-bio company that uses artificial intelligence and human judgment to match each patient with the best course of treatment, announced today that it is collaborating with AWS to develop AI diagnostics, de-risk and expedite clinical trials, and revolutionize drug discovery. Owkin intends to utilize AWS’s dependable, secure, and expansive cloud platform in addition to its proven global infrastructure to improve data operations, achieve operational excellence, and further precision medicine research.
“Owkin was founded on the belief that technologies that securely and privately extract insights from hospitals and research facilities’ massive amounts of patient data will be key to precision medicine’s future,” says cofounder and CEO Thomas Clozel. Collective intelligence through teamwork and cutting-edge technology are our greatest assets. They can expand their research by working with AWS to leverage the cloud’s strength, security, and adaptability.
By utilizing machine learning and high-performance computing, Owkin careers and AWS hope to spur biotech innovation. Through the integration of AWS’s secure and scalable cloud infrastructure and services with Owkin’s specialized biotech research and development, the company is able to meet its storage needs up to petabytes develop generative AI applications, and create and modify foundation models. In order to improve patient health outcomes, Owkin careers will use Amazon SageMaker to develop, train, and implement machine learning models, optimize the processing of high-quality data at scale, and advance the deployment of its portfolio of AI solutions through research partners, pathology labs, and biopharma.
In order to solve important precision medicine challenges and determine the best course of action for each patient, Owkin careers and specialists from AWS’s Healthcare and Life Sciences team, who bring practical experience from the pharmaceutical, medical device, biotech, and government health and regulatory organizations will collaborate to leverage technology. Owkin careers and AWS hope to use their respective areas of expertise to support groundbreaking discoveries and propel the creation of game-changing solutions for global healthcare issues.
“The cloud and the advent of computational biochemistry significantly accelerated the pace of innovation in healthcare and life sciences, and applying generative AI enables another huge leap forward,” stated Dan Sheeran, General Manager of Healthcare and Life Sciences at AWS. They are thrilled to work with Owkin to provide flexible, safe, and scalable infrastructure as well as purpose-built generative AI tools to help them reduce costs and find and develop better, more targeted treatments more quickly.
Owkin intends to leverage AWS’s cutting-edge networking and scalability, along with its high-performance, affordable, and scalable infrastructure, to develop and implement its applications. These applications will include Amazon Elastic Compute Cloud (Amazon EC2) UltraClusters of P5 instances, which are driven by NVIDIA H100 Tensor Core GPUs. Using second-generation Elastic Fabric Adapter (EFA) technology, these instances support networking rates of up to 3,200 Gbps.
This allows Owkin to scale up to 20,000 H100 GPUs in EC2 UltraClusters for on-demand access to supercomputer-class performance. Machine learning model training times are sped up with P5 instances, going from days to hours. Additionally, Owkin Careers plans to test out AWS chips designed specifically for cloud computing, such as AWS Graviton, a general-purpose CPU that offers the best value performance for a variety of workloads, and AWS Trainium, a high-performance machine learning accelerator.
According to the AWS Digital Sovereignty Pledge, Owkin will have complete control over their data by utilizing some of the most robust sovereignty controls available today, such as data residency guardrails, specialized hardware and software to prevent outside access during processing on Amazon Elastic Compute Cloud, and data encryption whether the data is in transit, at rest, or in memory (Amazon EC2). As a result, Owkin careers will be able to maintain its solid and safe partnership building while producing and working with the best possible data. Additionally, Owkin careers will gain from AWS’s extensive compliance controls, which support 143 security standards and compliance certifications and assist partners in meeting global regulatory requirements.
Concerning Owkin Careers
A TechBio company called Owkin makes sure every patient receives the best care possible by fusing artificial and human intelligence. AI helps us comprehend complex biology, which helps us find new treatments, expedite and reduce risk in clinical trials, and create AI diagnostics. Owkin unlocks the potential of artificial intelligence to power precision medicine by leveraging privacy-enhancing federation to access current multimodal patient data. We combine cutting-edge AI methods with wet lab experiments to build a potent feedback loop for accelerated innovation and discovery.
MOSAIC, the largest multi-omics atlas for cancer research worldwide, was founded by Owkin.
Through investments from prestigious biopharma companies (including Sanofi and BMS) and venture funds (including Fidelity, GV, and BPI), Owkin has raised over $300 million.
MOSAIC & Owkin: Building the World’s Largest Spatial Omics Dataset in Oncology
MOSAIC and Owkin are two entities working together on a groundbreaking initiative: creating the largest spatial omics dataset in oncology. Here’s some key information:
MOSAIC:
Stands for Multi Omic Spatial Atlas in Cancer.
It’s a large-scale research project led by Owkin with partners like NanoString and research institutions.
Aims to collect and analyze 7,000 tumor samples across seven different cancer types (e.g., lung, breast, glioblastoma).
Uses cutting-edge spatial omics technologies to map cancer cells and their surrounding environment at high resolution.
Owkin:
An AI biotech company specializing in artificial intelligence and data science for oncology research.
Provides foundational expertise in AI, data science, and oncology for the MOSAIC project.
Develops the data platform and applies AI/ML to analyze the MOSAIC data and unlock new insights.
What makes MOSAIC unique?
Scale: 100x larger than existing spatial omics datasets, offering unprecedented depth and granularity.
Multimodal: Combines various data types like gene expression, protein levels, and spatial information.
AI-powered: Leverages Owkin’s AI expertise to extract meaningful insights from the vast dataset.
Potential impact:
Advanced drug discovery: Identify new drug targets and develop more effective therapies.
Personalized medicine: Understand individual tumors better and develop tailored treatment approaches.
Improved diagnosis: Develop non-invasive methods for early cancer detection and more accurate diagnoses.
Read more on Govindhtech.com
0 notes
Text
Machine Learning's Influence on the Pharma Industry
The pharmaceutical industry has long been a cornerstone of global healthcare, consistently pushing the boundaries of innovation and research. In recent years, it has witnessed a dramatic transformation, largely driven by the integration of artificial intelligence (AI) and machine learning (ML) into various facets of drug discovery, development, and patient care. With the sector poised to reach a staggering $1.5 trillion economy by 2023, the adoption of more efficient and automated processes is not only a choice but a necessity.
AI in Pharma: A Paradigm Shift
The integration of AI in the pharmaceutical industry has been a game-changer, revolutionizing the landscape of drug discovery and development. AI empowers pharmaceutical companies to advance precision medicine, ensuring that healthcare treatments reach the right patients at the right time. This transformative influence of AI extends from the early stages of drug discovery to the improved understanding and utilization of clinical trial data, marking a consistent and progressive integration of AI technologies.
Here Are Four AI & ML Trends in the Pharma Industry
Drug Discovery and Development using AI in Pharma
The journey from conceptualizing a new drug to bringing it to market is arduous, taking 7-10 years and costing a staggering $2 billion. Furthermore, the process is fraught with high failure rates at various stages. Companies like Cyclica and Bayer are leading the charge in this sector, collaborating to expedite drug discovery. Cyclica, a biotechnology firm, has harnessed AI and computational biophysics to screen small molecule drugs against existing repositories of proteins, dramatically reducing discovery time. Their algorithms predict ADMET (Absorption, Distribution, Metabolism, Excretion, and Toxicity) to enhance drug efficacy.
Verge Genomics, a neuroscience firm, is partnering with pharmaceutical companies and tissue banks to leverage AI in bringing AI-generated compounds to market for the treatment of neurodegenerative diseases. Through large databases of patient tissues and AI-driven analysis, they discover novel gene targets.
Rare Diseases & Personalized Medicine
Historically, pharma companies focused on diseases affecting large populations, driven by regulatory requirements and the need for affordable drugs. However, AI has opened doors to drug development for rare diseases. The partnership between Tencent Holdings and Huma exemplifies the power of AI in detecting and diagnosing rare neurological diseases. AI algorithms are also used to create personalized drug treatments based on an individual’s genes, environment, and lifestyle. This shift towards personalized medicine is epitomized by the collaboration between Aprecia and Cycle Pharmaceuticals in the development of orphan drugs.
Clinical Trials
Clinical trials are a critical phase in drug development, but they face significant challenges. According to a study, 80% of clinical trials fail to meet enrollment timelines, and 1/3 of phase 3 trials terminate due to enrollment difficulties. Start-ups like Deep and Antidote are addressing these challenges using machine learning algorithms. Deep6's AI software analyzes a wide range of clinical data to streamline patient selection for pharmaceutical firms, while Antidote's AI platform matches patients with suitable trials based on their health conditions.
Drug Adherence and Dosage
Maintaining patient adherence and precise dosage during clinical trials is essential. AbbVie and AiCure use smartphone technology with machine learning algorithms to monitor drug adherence, particularly in diseases like schizophrenia. Curate.Ai, on the other hand, assists clinicians in identifying the right drug combination and dosing strategy based on individual patient data, especially relevant in complex diseases like cancer.
AI in Pharmaceuticals: A Healthcare Revolution
The integration of AI in the pharmaceutical industry is drastically altering how medicines are formulated and brought to market. The traditionally prolonged and costly process has evolved into an efficient and collaborative endeavor, thanks to AI. Pharmaceutical companies are partnering with technology firms, resulting in the development of groundbreaking products. AI's influence extends throughout the industry, making it an intelligent assistant for scientists and doctors, enabling the faster and more cost-effective development of superior medicines.
The expedited introduction of new treatments enhances healthcare for all. BirdzAI, a data management solution that combines AI and ML, empowers the industry with insights and predictions to make data-driven decisions. Data360, another solution, leverages machine learning to predict outcomes more accurately than humans using a multitude of variables.
The pharmaceutical industry is at the cusp of a transformative era, driven by the power of AI and ML. From drug discovery and development to clinical trials, rare diseases, and drug adherence, AI is changing the game. It's not just about making processes more efficient; it's about improving patient care, enhancing the quality of medicines, and ushering in a new era of healthcare. The integration of AI is not just a trend; it's a revolution that's here to stay. As we move forward, the pharmaceutical industry, in partnership with AI, will continue to unlock new possibilities and reach even greater heights.
To learn more about all of P360’s innovative products, visit P360.com
0 notes
Text
AI-based Clinical Trial Solutions For Patient Matching Market Segmented On The Basis Of Patient Matching Market Report Based On The Therapeutic Application, End-Use, Region And Forecast To 2030: Grand View Research Inc.
San Francisco, 11 Aug 2023: The Report AI-based Clinical Trial Solutions For Patient Matching Market Size, Share & Trends Analysis Report By Therapeutic Application, By End-use, By Region, And Segment Forecasts, 2022 – 2030 The global AI-based clinical trial solutions for patient matching market size is expected to reach USD 1.9 billion by 2030, registering a compound annual growth rate (CAGR)…
View On WordPress
#AI-based Clinical Trial Solutions For Patient Matching Industry#AI-based Clinical Trial Solutions For Patient Matching Market 2030#AI-based Clinical Trial Solutions For Patient Matching Market Revenue#AI-based Clinical Trial Solutions For Patient Matching Market Share#AI-based Clinical Trial Solutions For Patient Matching Market Size
0 notes
Text
https://kitsa.ai/team/
#ai in clinical trials#ai in pharma#clinical trials recruitment ai#accelerating clinical trials#clinical trial companies#how ai is transforming clinical trials#using ai to match patients with clinical trials
0 notes
Text
What are the top digital healthcare solutions?
More and more patients are demanding self-managed care and expecting healthcare providers to have the digital and remote patient monitoring capabilities according to a Digital Health Consumer Survey by a leading company.
This impetus of necessity has increased exponentially as the world faces the public health crisis-COVID-19.
As a result, healthcare organizations are turning into active partners with their patients to provide them personalized patient-centered care.
In order to match the pace with peers, improve clinical workflows and withstand a new level of care, healthcare app developers are helping providers develop resilient digital health solutions and utilize new technologies that are promising to deliver a positive patient experience.

1. Telehealth and Remote Patient Monitoring Applications
Patients avoid hour-long commutes, long waiting lines, spending extra money and time to make an in-person clinical visit.
In a survey, 24 percent Gen Z respondents underlined the dire requirement of convenience of the clinical settings. While around 25 percent respondents wanted greater transparency in the treatment taken.
To meet these rising demands of sustainable care, telehealth applications have become a new normal to not only ascertain the patients’ needs but also to reach-out at-risk patients who needs emergency care in their home with an assurance of safety.
Telhealth/telemedicine technology or services connects patients with provider with their smartphones or personal computer, get care from the comfort of the home and establish a good bond with care provider with an ongoing online in-person visit.
Whereas, on the same account Doctors can reduce their overhead expenses, save themselves from exposure to infections and illness, and increase patient satisfaction.
That said, telehealth app development integrated with clinical continuum functionalities is counted as a top digital health solution to improve clinical workflows.
2. Artificial intelligence for Clinical Trial Research and Health Data Management
The convergence of AI and Big Data (patient data generated from medical research, hospitals and care providers) has led to an evolution of intelligent health data analytics. This data analytics helps in clinical decision making and early discovery of potential threats that in turn, reduces the number of in-person doctor visits.
Plus, AI’s smart predictive analytics in clinical trials help healthcare professionals deliver accurate results that brings down the time and money invested in clinical trials.
3. Smart Asset Tracking with RFID and IoT
RFID or radio-frequency identification, another digital health solution helps in better tracking of the treatment process. The solution is present in multiple forms- from tracking surgical tools to tracking patients and staff.
RFID solution can be deployed into different healthcare facilities for tracking real-time visibility of equipment, inventory or even people in hospital. Some of its major applications are:
Patient and staff tracking
Surgical tools
Inventory tracking
Asset tracking
RFID can be tagged to patients’ wristband upon mutual agreement. Also known as smart patient tracking this helps in monitoring patient’s location when the staff is unable to find them or when they get lost in the hospital while wandering. This significantly reduces the time spent in searching patients.
RFID tracking inbuilt in apps helps to remind patients of upcoming scheduled events like taking medication, attending a doctor’s appointment, screening procedures, etc. Thereby, helping in smart tracking of treatment processes.
Some hospitals and pharmaceutical manufacturers also use RFID for inventory tracking or supply chain management. The wireless technology when deployed on barcode helps in reductions in shrinkage, increased product availability, and efficient handling of medicines. In addition, it can also help in drug authentication or as an anti-counterfeiting resource.
MAPS
Our mission is to advance the Medical Affairs profession and increase its impact across the biopharmaceutical and device industry by
Promoting excellence across Medical Affairs functions
Developing guidelines to support industry standards and best practices
Fostering advocacy for the Medical Affairs profession
Building capabilities and provide a platform that supports the practice of Medical Affairs
To know more visit:
Medical Affairs Professional Society602 Park Point Drive, Suite 225, Golden, CO 80401 – +1 303.495.2073 © 2022 Medical Affairs Professional Society (MAPS). All Rights Reserved Worldwide.
0 notes
Text
Global Life Sciences Software Market is valued at ~$11 billion in 2020 and is anticipated to grow at a CAGR of ~11% to reach $18 billion by 2025

The market for life sciences software (such as eTMF, LIMS, computer-aided drug discovery, RWE, ERP, CRM, QMS, virtual trials, patient recruitment software, patient & outcomes data management, etc.) is driven by rising R&D spend and pipeline, expanding number and size of biotechs, Covid-accelerated decentralized trials, increasing cost efficiencies and shorter time to market.
The globalLife Sciences Software marketis valued at ~$11 billion in 2020 and is expected to grow at a CAGR of ~11% to reach $18 billion by 2025. The Life Sciences Software market growth is being fueled by increased R&D efforts around the globe, stringent regulatory compliance/reporting, increasing use of AI & ML during the drug discovery and clinical trial phases, and growing demand for novel software solutions especially designed for biologics/cell, and gene therapy.
Drug Discovery Software Likely to Witness Fastest Growth
The rising pressure on pharma and biotech companies to lower costs during the research and preclinical stages of drug development, reduce timelines, and increase transparency through the use of deep learning software tools has resulted in a niche market for drug discovery software that is expanding quickly.
“Large players present in this segment are trying to consolidate the market by becoming a one stop shop. Moreover, companies are also considering to combine their solutions with bioinformatics and LIMS tools to have a competitive edge.”- Executive, Leading Software Provider, US
Explore Premium Report on Life Sciences Software Market @ https://meditechinsights.com/life-sciences-software-market/
Mounting Interest in Novel Software Solutions Specifically Designed for Biologics
With quickly growing research on advanced therapies such as cell and gene therapies and personalized vaccines, there is robust demand for software solutions to maximize process and workflow efficiencies across services. The FDA predicts that by 2025, 10 to 20 cell and gene therapy products will be approved a year. Companies are entering or growing into the biologics software space to take advantage of growth opportunities. Some of the key technology providers for CGT include Vineti, TrakCel, Be The Match, SAP, WellSky, Synthace and Cytiva.
Covid-19 Impact on Life Sciences Software Market
All stages of drug development have been impacted by Covid-19, but the clinical trials stage has been the most affected. This paved way for technological solutions to overcome such hurdles. After the pandemic, virtual trials have gained more importance than ever. There were hardly any M&As related to virtual trials taking place before the pandemic, but the market observed ~10 M&A deals in 2020. The companies are rapidly consolidating through M&As to improve their technology/service offerings for decentralized trials and leverage growth opportunities.
Competitive Landscape Analysis of Life Sciences Software Market
The global life sciences software market is highly competitive and fragmented. Some of the key players include Dassault Systèmes, LabVantage, PerkinElmer, Schrodinger, ICON, Veeva, PhlexGlobal, Saama, IQVIA, Parexel, Signant Health, Abbott, Science37, Medidata, IBM, Koneksa, SAP, Oracle, Optibrium, and Instem.
For More Detailed Insights, Contact Us @ https://meditechinsights.com/contact-us/
About Medi-Tech Insights
Medi-Tech Insights is a healthcare-focused business research & insights firm. Our clients include Fortune 500 companies, blue-chip investors & hyper-growth start-ups. We have completed 100+ projects in Digital Health, Healthcare IT, Medical Technology, Medical Devices & Pharma Services.
Contact:
Ruta Halde
Associate, Medi-Tech Insights
+32 498 86 80 79
1 note
·
View note
Text
Artificial Intelligence - Critical Prerequisite of Clinical Trials
A vast majority of researchers and specialized tech startup companies have started investing in developing Big Data and Artificial Intelligence (AI) tools to serve the pharmaceutical and medical devices companies, which is believed to transform the clinical trial process. But what exactly is the main outcome of AI?
The buzz around AI in clinical trials is due to its potential as the lynchpin for dramatically improving the probability of success and reducing the timelines.
How does AI really increase Clinical Trial Success Rates?
The answer lies in predictive analysis from available historical data. The main idea of the AI revolution is to bring about “efficient and faster decision making”, provide precision in clinical trials and bringing an effective product from the lab-to-market. The average timeline for a drug molecule to be released from lab-to-market is 9 years with a median development cost of $2 billion. The objective of AI implementation is to eliminate unnecessary repeated clinical evaluations, save costs & time and thereby ensure successful clinical trials.
AI based Clinical Trial transformation process can be divided into three main components:
Innovative Study Design
The first and foremost prerequisite for AI implementation is data mining.
Cross-comparison analysis of historical similar molecule protocol designs
Centralized Monitoring of Trial Risks
Measuring Drug Responses, Trial Outcomes, and Site Performance Prediction
Patient Recruitment and Retention Metrics
The optimum utilization of patient data will ensure effective patient recruitment and lower dropout rates. Several AI startups such as “DEEP6” and “antidote” have invested in developing patient trial matching software.
Tapping EMR/EHR to match the right patient trials
Patient eligibility Good-fit analysis for trials and predicting precise responsiveness
Smart Data-Driven Analysis
Effective Go/No-Go decision for every stage of the clinical trial process
Artificial intelligence is now being used for predicting cancer treatment type based on the combination of genes in the clinical trials right in the planning stage.
Predictive result analysis based on the drug molecule behavioral pattern
Dynamic Real-time access to data and sharing as and when there are updates
Leverage AI in Clinical Trials with Salesforce Einstein Platform Enablement
Cloudbyz has developed a CTMS solution natively built on Salesforce Platform with the flexibility to integrate AI tools and strategies complementing the end-to-end clinical trial process.
The Salesforce Einstein platform can act as a smart assistant which can be integrated into Cloudbyz CTMS. The Einstein Voice and Einstein predictive builder can help leverage artificial Intelligence in clinical trials in the following ways:
Discover insights: The Einstein Discovery feature enables CROs and Sponsors to analyze every data point, pulling together data from external sources, to create an effective study design in the planning stage of a clinical trial.
Predict outcomes: The Einstein Prediction Builder feature can be used to build a customized predictive score model to analyze required patient data tapped from EHR / EMR. Based on the analysis score, the closest trial outcomes can be predicted to match the patients suitable for the clinical study.
Recommend: The platform also enables users to analyze site performance metrics, which will help in recommending appropriate sites for selection based on responses.
Automate routine tasks: All monitoring activities for scheduling monitoring visits, audit trails, approval of documents, reporting and updations based on recommendations can be automated at any stage with Cloudbyz Centralized RBM solutions. All the activities and reminders can be set up hands-free using Einstein Voice feature.
The Final Word
Investment in AI and Big Data will certainly improve the clinical trial process. However, initial investments on tools and technologies need to be taken into consideration. Further, market experience with AI tools will bring down the costs and time-to-market of the drug. How AI can transform the drug discovery process, and make the above statement true - Only time will tell.
References
https://www.himss.org/library/ehr
https://www.himss.org/electronic-medical-records
https://www.ijclinicaltrials.com/index.php/ijct/article/download/125/94
https://www.jpmorgan.com/commercial-banking/insights/ai-transform-clinical-trials
https://healthcareweekly.com/artificial-intelligence-clinical-trials/
http://www.ia-grp.com/views/3_types_artificial_intelligence_clinical_research/
http://www.clinicalinformaticsnews.com/2017/09/29/the-intelligent-trial-ai-comes-to-clinical-trials.aspx
https://www.salesforce.com/in/products/einstein/overview/
https://www.salesforce.com/video/193342/
https://www.salesforce.com/products/einstein-analytics/products/einstein-discovery/
https://www.salesforce.com/products/einstein-analytics/products/einstein-discovery/#prediction-builder
https://www.salesforce.com/products/einstein/einstein-voice/
Further queries or thoughts please comment or feel free to contact:
Srinivas Sashidhar K
Domain Specialist - Life Sciences
Cloudbyz Inc.
Email: [email protected]
Linkedin: https://www.linkedin.com/in/srinivas-sashidhar-27449a15/
1 note
·
View note
Text
NLP in Healthcare and Life Sciences Market Strategy and Remarkable Growth Rate By 2027
According to a research report "NLP in Healthcare and Life Sciences Market by Component (Solutions and Services), NLP Type (Statistical, Hybrid), NLP Technique (IVR, OCR), Application (Clinical Trail Matching, Drug Discovery), End Users and Region - Global Forecast to 2027" published by MarketsandMarkets, the NLP in healthcare and life sciences market size to grow from USD 2.2 billion in 2022 to USD 7.2 billion by 2027, at a Compound Annual Growth Rate (CAGR) of 27.1% during the forecast period. Various factors such as increasing demand of analyzing and extracting insights from narrative text and huge amount of clinical data, and the evolution of cognitive computing to fuel the demand for NLP solutions for precision medicine applications are expected to drive the adoption of NLP solutions in healthcare and life sciences market.
According to Lexalytics, NLP is a computing technique that analyzes text to determine its meaning, enabling computer applications that can communicate effectively with humans. Healthcare providers, pharmaceutical companies, and biotechnology firms all use text analytics and NLP technology to improve patient outcomes, streamline operations, and manage regulatory compliances.
Automated Registry Reporting segment to register the highest CAGR during the forecast period
The segmentation of the NLP in healthcare and life sciences market by the application segment includes sentiment analysis, drug discovery, clinical trial matching, dictation & EMR implications, automated registry reporting, AI chatbots & virtual scribe, and other applications (review management, question answering, spell correction, and email filtration). The automated registry reporting segment is developing rapidly due to many major technological advancements resulting in enhancing the efficiency of the overall industry. Health systems will need to recognize when an ejection percent is recorded as part of a note and preserve each number in a format that can be used by the organization’s analytics platform for automated registry reporting to implement automated reporting.
Large Enterprises segment to register for the highest market size during the forecast period
Based on organization size, the NLP in healthcare and life sciences market is segmented into large enterprises and SMEs. Currently, the market share of large enterprises is higher; however, the market for SMEs is expected to increase at a higher CAGR during the forecast period. These organizations generate a large volume of data, which must be implemented in their work through collaboration among different teams. The data generated from these large enterprises can then be stored at a lower cost without compromising safety and agility through NLP solutions.
APAC to have a higher CAGR during the forecast period
The NLP in healthcare and life sciences market has been segmented into five major regions: North America, Europe, Asia Pacific, Latin America, and Middle East and Africa. Asia Pacific is expected to have the highest CAGR during the forecast period. The region is growing at a good pace because of the major multinational players coming over and a lot of new entrepreneur setups are adopting cloud-based NLP solutions, as using these solutions healthcare and life sciences firms harness the relevant insights and concepts from the clinical data that was previously considered buried in the text form. China, Japan, and India are displaying ample growth opportunities in the NLP in healthcare and life sciences market.
Some major players in the NLP in healthcare and life sciences market include IBM (US), Microsoft (US), Google (US), AWS (US), IQVIA (US), Oracle (US), Inovalon(US), Dolbey (US), Averbis (Germany), SAS Institute (US), Press Ganey (US), Ellipsis Health (US), Health Fidelity (US), Centene (US), Caption Health (US), Clinithink (US), HPE (US), Oncora Medical (US), Flatiron Health (US), Apixio (US), Forsee Medical (US), Gnani.ai (India), Notable (US), Biofourmis (US), Suki (US), Wave Health Technologies (US), Corti (Denmark), CloudMedx (US), MedlnReal (US), Emtelligent (US).
About MarketsandMarkets™
MarketsandMarkets™ provides quantified B2B research on 30,000 high growth niche opportunities/threats which will impact 70% to 80% of worldwide companies’ revenues. Currently servicing 7500 customers worldwide including 80% of global Fortune 1000 companies as clients. Almost 75,000 top officers across eight industries worldwide approach MarketsandMarkets™ for their painpoints around revenues decisions.
Our 850 fulltime analyst and SMEs at MarketsandMarkets™ are tracking global high growth markets following the "Growth Engagement Model – GEM". The GEM aims at proactive collaboration with the clients to identify new opportunities, identify most important customers, write "Attack, avoid and defend" strategies, identify sources of incremental revenues for both the company and its competitors. MarketsandMarkets™ now coming up with 1,500 MicroQuadrants (Positioning top players across leaders, emerging companies, innovators, strategic players) annually in high growth emerging segments. MarketsandMarkets™ is determined to benefit more than 10,000 companies this year for their revenue planning and help them take their innovations/disruptions early to the market by providing them research ahead of the curve.
MarketsandMarkets’s flagship competitive intelligence and market research platform, "Knowledgestore" connects over 200,000 markets and entire value chains for deeper understanding of the unmet insights along with market sizing and forecasts of niche markets.
Contact: Mr. Aashish Mehra MarketsandMarkets™ INC. 630 Dundee Road Suite 430 Northbrook, IL 60062 USA : 1-888-600-6441 [email protected]
0 notes
Link
[사이언스] 환자 진단하고 치료법 제시하는 인공지능
Technological advances are being made every day, and the medical field is no exception. Some cutting-edge technology is now helping doctors diagnose illnesses and suggest treatment plans with as much accuracy as real doctors. Let's check out these developments in medical technology!
As a quick note, today's article is taken from a news site meant for kids. If you're at a comfortable intermediate level or above, I think you should be able to tackle this article with not too much difficulty!
[사이언스] 환자 진단하고 치료법 제시하는 인공지능
김보민 기자, 서진명인턴기자
10초 만에 치료법 ‘뚝딱’
뚝딱 — onomatopoeia used for when something is done quickly
환자의 병을 진단하고 치료를 돕는 의료용 인공지능(AI) 프로그램을 정부가 2020년까지 개발한다. 정부가 나서 국내 대형 병원 및 정보기술(IT) 기업들과 협력해 미국 IBM의 ‘왓슨’과 같은 AI 의사를 개발하겠다는 것. 왓슨은 미국 IBM에서 개발한 AI 소프트웨어로 의과대학, 병원, 전문연구기관 등에서 나오는 의학 관련 자료들을 실시간으로 습득해 환자의 질병 정보를 분석하고 환자에게 가장 적합한 처방을 내놓는다.
습득하다 習得 — to acquire, learn
적합하다 適合 — suitable, fit
과학기술정보통신부는 한국판 AI 의사인 ‘닥터 앤서’를 개발하기 위한 ‘AI 기반 정밀의료 솔루션 사업’을 추진하기로 하고, 지난달 30일 서울아산병원에서 사업 출범식(새로운 일의 시작을 알리는 의식)을 열었다. 닥터 앤서는 의료 영상, 유전체 정보 등 의료 빅데이터(엄청나게 많은 정보들)를 기반으로 환자 특성에 맞는 진단을 하고 치료 방법을 알려준다. 유방암, 대장암, 심장질환, 치매, 소아 희귀·난치성 유전질환 등에 적용할 수 있게 개발할 예정이다.
기반 基盤— base, groundwork
정밀하다 精密 — detailed, precise
추진하다 推進 — to push forward, move forward
유방암 乳房癌 — breast cancer
대장암 大腸癌 — colon cancer
소아 희귀·난치성 유전질환 小兒 稀貴·難治性 遺傳疾患 — rare and difficult to treat genetic disorders in children
닥터 앤서처럼 의사를 도와 환자의 병을 진단하고 치료를 돕는 의료용 AI들을 알아보자.
치료법을 추천해요
가천대 길병원(인천 남동구)은 2016년 12월 AI 소프트웨어 ‘왓슨’을 이용해 암을 진료하는 ‘IBM 왓슨 인공지능 암센터’를 열었다. 왓슨 인공지능 암센터에서는 위암, 폐암, 유방암, 대장암, 난소암, 자궁경부암, 방광암, 전립선암 총 8개의 암에 대해 진료가 가능하다. 예약 후 진료를 받으러 가면 주치의가 왓슨에 나이, 성별, 검사 결과 등 환자 정보를 입력한다. 왓슨은 10초 안에 초록색(강력 추천), 주황색(추천), 분홍색(비추천) 세 가지 색으로 환자에게 맞는 치료법을 추천한다.
위암 胃癌 — stomach cancer
난소암 卵巢癌 — ovarian cancer
자궁경부암 子宮頸部癌 — cervical cancer
방광암 膀胱癌 — bladder cancer
전립선암 前立腺癌 — prostate cancer
주치의 主治醫 — the doctor in charge of one’s care
이때 관련 의학논문을 통해 자신의 의견에 대한 근거도 제시한다. 담당 주치의는 왓슨의 의견과 여러 분야 의사들의 의견을 합친 결과를 종합적으로 판단해 최종 진단을 내린다. 가천대 길병원 관계자는 “왓슨과 의사의 진단 결과는 ‘강력 추천’과 ‘추천’의 경우 약 80% 일치했다”면서 “왓슨을 통해 환자는 자신에게 맞는 최적의 진료를 받을 수 있는 것”이라고 설명했다.
종합적 綜合的 — synthesized; overall
의사를 도와요
사람의 뼈 나이를 알려주는 AI도 있다.
국내 AI 소프트웨어 개발 스타트업(새로 생긴 벤처기업) 뷰노에서 개발한 AI 의료기기인 ‘뷰노매드 본 에이지’는 방대한 양의 데이터를 스스로 학습한다. X선으로 촬영한 손의 뼈 영상을 병원이 가지고 있는 다른 뼈 영상들과 분석해 가장 유사한 나이를 알려준다. 이는 성장기에 있는 어린이의 성장판이 닫혔는지 판단하는 데 효과적.
방대하다 厖大 — enormous, huge
유사하다 類似 — similar, alike, resembling
매일 수천 명의 환자들이 방문하는 대형 병원의 경우, 의사 한 명 당 수많은 환자를 진료하고 진단 결과를 분석한다. 본 에이지가 영상을 대신 분석해 의사를 도와 빠른 일처리를 가능하게 하는 것. 본 에이지는 현재 임상시험을 끝내고 식품의약품안전처에 의료기기 2등급 허가를 요청한 상태. 의료기기로 승인되면 병원에서 사용할 수 있다.
임상시험 臨床試驗 — clinical trial
식품의약품안전처 食品醫藥品安全處 — Ministry of Food and Drug Safety
의사만큼 정확해요
얼핏 보면 알기 힘든 피부암도 AI가 진단한다.
얼핏 — in a flash, in a moment
검은 반점처럼 생긴 악성 흑색종은 피부암의 한 종류로, 일찍 알게 되면 치료가 쉽지만 치료시기를 놓치면 간이나 폐로 옮��져 생명에 위협이 될 수 있다. 이런 악성 흑색종을 AI로 알아낼 수 있다는 연구결과가 발표됐다.
악성 惡性 — malignant
흑색종 黑色腫 — melanoma
장성은 서울아산병원(서울 송파구) 피부과 교수팀은 마이크로소프트가 공개한 AI 모델 ‘레스넷-152’에 2만여 장의 피부 종양 사진을 학습시켜 피부암을 80~90%의 정확도로 감별(보고 알아냄)해냈다. 높은 성능의 영상 인식 기능을 가진 레스넷-152는 악성 흑색종인지 아닌지 90%의 정확도 감별해내며 의료진에 버금가는 진단 정확성과 적중률을 보였다.
종양 腫瘍 — tumor
성능 性能 — performance, function
버금가다 — to come close to
English translation — [Science] AI that diagnoses patients and suggests treatment plans
Reporter Kim Bomin, Intern reporter Seo MinMyung
Treatment plans banged out in 10 seconds
The government will develop a medical AI program that can diagnose patients’ illnesses and help with treatment by 2020. They have come forward and said that they will work with major hospitals and IT companies to develop an AI like American company IBM’s Watson. The Watson AI software processes medical materials coming out of medical universities, hospitals, and specialized research institutes in real time, analyzes patients’ information, and offers the most appropriate treatment.
The Ministry of Science and ICT, in order to develop a Korean AI doctor called “Doctor Answer,” has decided to push forward with an AI-based precision medical solutions company and, on May 30th, held an opening ceremony for the company at Seoul Asan Hospital. Doctor Answer uses medical big data such as medical images and genetic information as its base to give the most fitting diagnosis and treatment options for the patient’s particular case. There are plans to develop it so that it can be utilized with breast cancer, colon cancer, heart disease, dementia, and rare and difficult to treat childhood and genetic diseases.
Let’s learn about some other medical AI that help doctors diagnose and treat patients like Doctor Answer does.
Suggesting courses of treatment
In December 2016, Gachon University Gil Hospital (located in Incheon's Namdong-Gu) used the AI software "Watson" to open the IBM Watson AI Cancer Center. At the Watson AI Cancer Center eight types of cancer—stomach cancer, lung cancer, breast cancer, colon cancer, ovarian cancer, cervical cancer, bladder cancer, and prostate cancer—can be treated. After making an appointment when one goes in for treatment, the doctor inputs the patient's age, sex, test results, and other information into Watson. Within ten seconds, Watson suggests color-coded treatment plans—green (strongly recommended), orange (recommended), and pink (not recommended)—to match the patient.
At this point, Watson gives the rationale for its opinion via related medical papers.The primary physician judges Watson’s opinion together with the opinions of doctors in various fields and makes the final diagnosis. A representative for Gachon University Gil Hospital explained, “Watson and the doctor’s diagnosis overlapped with the ‘strongly recommended’ and ‘recommended’ diagnoses about 80% of the time,” and that “through Watson, patients can receive the most suitable treatment for their case.”
Helping doctors
There is also an AI that can tell a person’s bone age.
The medical AI medical device 'VUNO Med® – Bone Age', deveolped by AI software development startup Vuno, studies an enormous amount of data on autonomously. Analyzing x-ray images of hand bones with other bone images in the hospital's possession, it tells the most likely age of the bones. It is effective for determining whether the growth plates of children in their developmental period have closed.
In the case of major hospitals that see thousands of patients each day, each doctor must treat and analyze the diagnostic results of numerous patients. Bone Age analyzes images for the doctors and makes it possible for them to work more quickly. The AI is currently finishing clinical trials and waiting for level 2 medical device clearance from the Ministry of Food and Drug Safety. Once approved as a medical device, it can be used in hospitals.
As accurate as doctors
The AI can even diagnose skin cancer hard to catch at a glimpse.
A type of skin cancer that appears as a black dot, malignant melanoma is easily treated when caught early but can move to the liver or lungs and threaten one's life if the treatment window is missed. Research results have been released showing that these malignant melanomas can be identified by AI.
Dermatology professor Jang SeongEun's team at Seoul Asan Hospital (located in Seoul Songpa-Gu) trained Microsoft's AI model ResNet-152 on about 20,000 images of skin tumors, and it identified skin cancer with an accuracy rate of 80~90%. Accurately identifying malignant melanoma 90% of the time with its high-performance image recognition function, ResNet-152 displayed levels of accuracy and precision close to those of actual medical teams.
#korean news#donga kids#medical ai#intermediate korean#korean reading#korean translation#studyblr#langblr#koreand studyblr#korean langblr
68 notes
·
View notes