#lejhro
Explore tagged Tumblr posts
Text
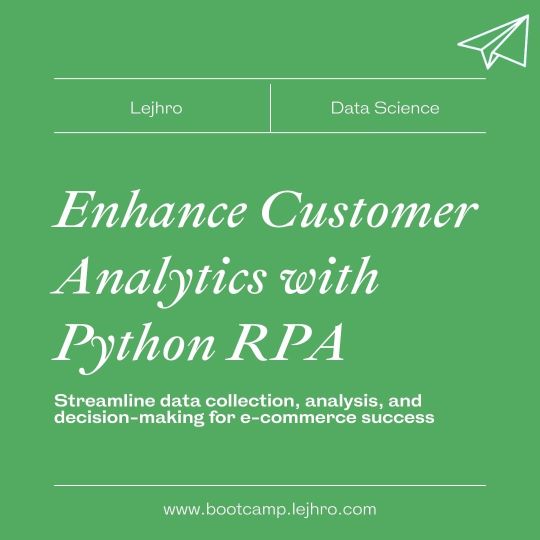
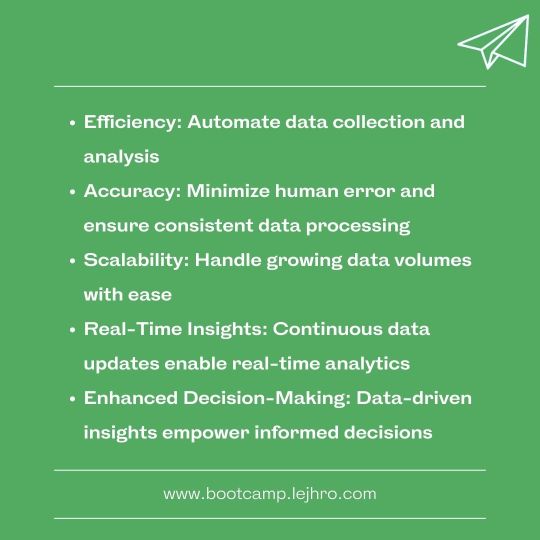
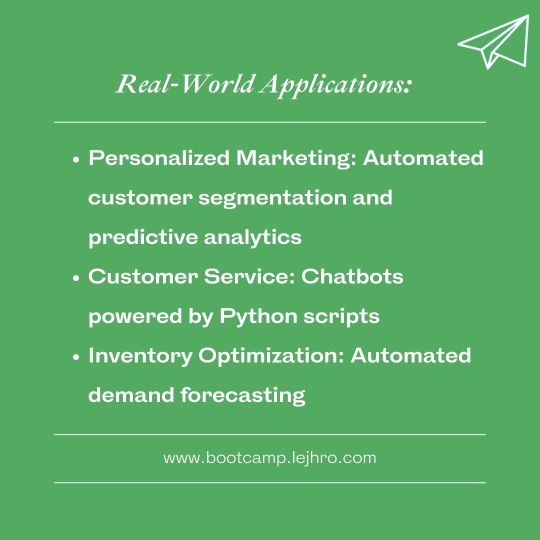
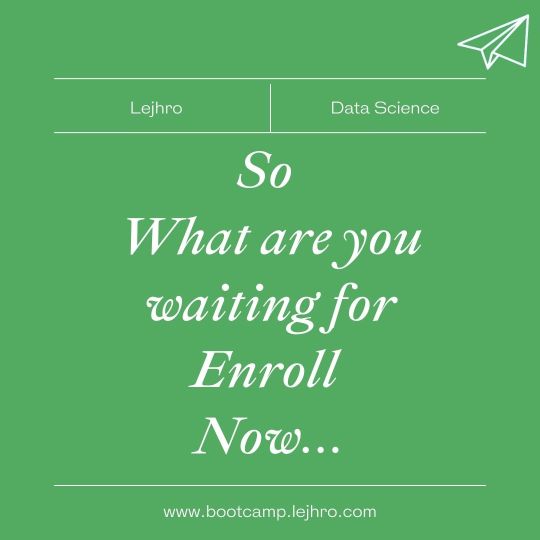
🚀 Boost Your E-commerce Game with Python RPA! 🚀
Enhance customer analytics with Python-based Robotic Process Automation (RPA) and stay ahead of the competition!
💥 Efficiency, accuracy, and scalability - what more could you ask for? 🤔
💥Learn More: www.bootcamp.lejhro.com/resources/python/improving-customer-analytics-in-e-commerce
💥The latest scoop, straight to your mailbox : http://surl.li/omuvuv
#Lejhro#LejhroBootcamp#Ecommerce#Python#RPA#CustomerAnalytics#BusinessGrowth#DigitalTransformation#DataScience#ArtificialIntelligence#MachineLearning#Automation#TechTrends#Innovation#MarketingTips#Ebusiness
2 notes
·
View notes
Text
Exploring MasterClass: Unlocking Your Potential with Expert-Led Learning
MasterClass is a renowned online learning platform that offers high-quality classes taught by some of the world’s most celebrated experts across various fields. Whether you aim to enhance your cooking skills, dive into the world of writing, or master photography, MasterClass has something for everyone. The platform provides an immersive learning experience through engaging video lessons, allowing you to learn directly from the best in the business.
Some great classes available on MasterClass include:
Data Science Essentials: Delve into the foundational concepts and practical applications of data science.
Data Analytics Mastery: Learn how to analyze and interpret complex datasets to drive informed decision-making.
Digital Marketing Strategies: Discover the techniques to effectively market your brand in the digital age.
Full Stack Development Bootcamp: Gain the skills necessary to become a proficient full stack developer, covering both front-end and back-end technologies.
My Experience with Lejhro
Having attended a MasterClass at Lejhro, I can confidently say that it was an incredible experience. Lejhro offers a unique blend of hands-on learning and expert guidance that sets it apart from other platforms. The instructors are not only knowledgeable but also passionate about sharing their expertise.
During my time at Lejhro, I gained valuable insights and practical skills that I could immediately apply. The interactive nature of the classes allowed for engaging discussions and networking opportunities with fellow learners. This supportive community environment made learning even more enjoyable and enriching.
If you’re considering taking a MasterClass, I highly recommend exploring the options available on the platform. Whether you’re interested in cooking, writing, or any other creative endeavor, there’s something for everyone. And if you’re looking for a more personalized and hands-on experience, consider attending a class at Lejhro. You won’t regret it!
MasterClass is a fantastic way to learn from the best, and with platforms like Lejhro offering similar quality education, you have all the tools you need to unlock your potential and achieve your goals.
#MasterClass#OnlineLearning#DataScience#DataAnalytics#DigitalMarketing#FullStackDevelopment#Lejhro#HandsOnLearning#LifelongLearning#SkillDevelopment#LearningJourney#CreativeEducation#ExpertInstructors#CommunityLearning#ProfessionalGrowth#EducationForAll#UnlockYourPotential#PersonalizedLearning#ImmersiveLearning#NetworkingOpportunities
1 note
·
View note
Text
What is the significance of understanding data mining techniques, algorithms, and their real-world applications?
Hi,
Understanding data mining techniques and algorithms is essential because they help turn raw data into meaningful insights. Data mining is the process of discovering patterns, correlations, or trends in large datasets. It involves several key algorithms, such as decision trees, clustering, and association rule learning, each of which serves a specific purpose depending on the data and the problem at hand.
For example, clustering algorithms group similar data points together, which can help businesses segment customers based on their behaviors. Decision trees are commonly used for classification tasks, such as determining whether a customer is likely to churn based on past behaviors.
The real-world applications of data mining are vast. In retail, data mining helps companies understand customer buying patterns and optimize inventory management. In healthcare, it’s used to identify risk factors for diseases by analyzing patient records. Financial institutions rely on data mining for fraud detection by identifying unusual transaction patterns.
Mastering these techniques allows data scientists to derive actionable insights, make better predictions, and solve complex problems across different industries. Data mining also serves as a foundation for machine learning and AI, further amplifying its significance.
0 notes
Text
#full stack web development#full stack training#full stack python training in ameerpet#full stack developer#full stack software developer
2 notes
·
View notes
Text
A TURN FROM B.Com OR BBA GRADUATE TO
DATA ANALYST
The business world is changing, and so are the opportunities within it. If you've finished your studies in Bachelor of Commerce (B.Com) or Bachelor of Business Administration (BBA), you might be wondering how to switch into the field of data analysis. Data analysts play an important role these days, finding useful information in data to help with decisions. In this blog post, we'll look at the steps you can take to smoothly change from a B.Com or BBA background to the exciting world of data analysis.
What You Already Know:
Even though it might feel like a big change, your studies in B.Com or BBA have given you useful skills. Your understanding of how businesses work, finances, and how organisations operate is a great base to start from.
Step 1: Building Strong Data Skills:
To make this change, you need to build a strong foundation in data skills. Begin by getting to know basic statistics, tools to show data visually, and programs to work with spreadsheets. These basic skills are like building blocks for learning about data.
I would like to suggest the best online platform where you can learn these skills. Lejhro bootcamp has courses that are easy to follow and won't cost too much.
Step 2: Learning Important Tools:
Data analysts use different tools to work with data. Learning how to use tools like Excel, SQL, and Python is really important. Excel is good for simple stuff, SQL helps you talk to databases, and Python is like a super tool that can do lots of things.
You can learn how to use these tools online too. Online bootcamp courses can help you get good at using them.
Step 3: Exploring Data Tricks:
Understanding how to work with data is the core of being a data analyst. Things like looking closely at data, testing ideas, figuring out relationships, and making models are all part of it. Don't worry, these sound fancy, but they're just different ways to use data.
Step 4: Making a Strong Collection:
A collection of things you've done, like projects, is called a portfolio. You can show this to others to prove what you can do. As you move from B.Com or BBA to data analysis, use your business knowledge to pick projects. For example, you could study how sales change, what customers do, or financial data.
Write down everything you do for these projects, like the problem, the steps you took, what tools you used, and what you found out. This collection will show others what you're capable of.
Step 5: Meeting People and Learning More:
Join online groups and communities where people talk about data analysis. This is a great way to meet other learners, professionals, and experts in the field. You can ask questions and talk about what you're learning.
LinkedIn is also a good place to meet people. Make a strong profile that shows your journey and what you can do. Follow data analysts and companies related to what you're interested in to stay up to date.
Step 6: Gaining Experience:
While you learn, it's also good to get some real experience. Look for internships, small jobs, or freelance work that lets you use your skills with real data. Even if the job isn't all about data, any experience with data is helpful.
Step 7: Updating Your Resume:
When you're ready to apply for data jobs, change your resume to show your journey. Talk about your B.Com or BBA studies, the skills you learned, the courses you took, your projects, and any experience you got. Explain how all of this makes you a great fit for a data job.
Using Lejhro Bootcamp:
When you're thinking about becoming a data analyst, think about using Lejhro Bootcamp. They have a special course just for people like you, who are switching from different fields. The Bootcamp teaches you practical things, has teachers who know what they're talking about, and helps you find a job later.
Moving from B.Com or BBA to a data analyst might seem big, but it's totally doable. With practice, learning, and real work, you can make the switch. Your knowledge about business mixed with data skills makes you a special candidate. So, get ready to learn, practice, and show the world what you can do in the world of data analysis!
***********
3 notes
·
View notes
Text
AI Made Easy: Your Step-by-Step Guide to Getting Started
Thinking to start learning Artificial intelligence but sick of searching through the internet? No coding background, no required degree , no guidance but everyone is saying “AI IS THE FUTURE” , “AI WILL REPLACE YOU”, “AI WILL KICK YOU OUT OF THE JOB” and many more. Before I start let me tell you some true facts that internet will not tell you and you will end up in wasting your time so if you think AI don’t require prior knowledge of coding then it is a lie “A clear cut lie”. Being a AI developer and AI expert is completely 2 different things, if you want to develop AI tools then off course you need knowledge of coding , data structures , math, stats but if you are AI tools expert then yes you don’t require prior knowledge of these. So firstly, decide you want to learn AI tools or want to develop AI tools?
How do I start learning AI?
Is AI hard to learn ? Not really, but people may get intimidated by its complexity and mechanism. It is not entirely accurate to say that programming is universally daunting; however, its complexity and underlying mechanisms can indeed intimidate some individuals. Mastering programming languages and coding is not an effortless task for everyone. Each language possesses its own unique syntax and structure, which can pose challenges for novices. Nevertheless, with a strong commitment to learning, it is certainly achievable. Begin with foundational concepts and gradually progress to more advanced languages — eventually, the pieces will come together. Furthermore, artificial intelligence encompasses multiple domains, rather than being a singular field of study.
Python : Python ranks among the most widely utilized programming languages, suitable for tasks ranging from simple to complex. Its learning curve is relatively gentle, making it accessible for beginners. Mastering Python will empower you to effectively develop and implement artificial intelligence algorithms.
Fundamentals of Machine Learning : It is essential to familiarize yourself with the foundational concepts of machine learning. This knowledge will facilitate your understanding of both straightforward and intricate AI algorithms.
Statistics, Probability, and Mathematics : The ability to collect and analyze data necessitates a solid understanding of statistics, probability, and mathematics. Therefore, it is crucial to study the theories within these fields to prepare yourself for engaging with new and complex data structures.
Natural Language Processing (NLP): NLP allows computers to replicate human language by analyzing textual data. This vital area of artificial intelligence should be one of your initial focuses.
Problem-Solving Skills: The domain of artificial intelligence revolves around addressing various challenges. Whether it involves debugging or managing missing values in datasets, you must be adept at identifying the root causes of issues and devising appropriate solutions. Aim to apply your AI expertise to solve real-world problems.
Commitment to Continuous Learning : The field of AI demands ongoing education, so it is important to possess the determination and commitment to stay updated. You should be aware of the latest techniques for data acquisition and transformation into actionable insights. Proficiency in logical reasoning and decision-making will also be advantageous.
Testing and Self-Improvement : The development of AI algorithms and models requires a commitment to continuous enhancement. You must be skilled in conducting thorough tests and implementing necessary adjustments to achieve optimized outcomes.
Entering the industry can present challenges, particularly for newcomers. But, with unwavering determination and concentration, one can swiftly understand both fundamental and advanced concepts by utilizing online resources, courses, and mentorship opportunities. You can also enroll in an online Bootcamps to connect with industry experts. The Lejhro Bootcamp will give you deeper insights into the implications of AI in the business world. By the end, you’ll be working on real-life projects to prepare yourself to secure your dream job!
Engaging with peers who share similar interests, whether in person or through online platforms, can provide valuable insights into their experiences and the obstacles they have faced. Additionally, it is advisable to seek guidance from AI experts and professionals who are currently active in the field. Their expertise can assist you in navigating challenges and addressing your inquiries. With a clear perspective, the complexities of AI will become more manageable and comprehensible.
#machinelearning#artificialintelligence#datascience#AIForBeginners#ArtificialIntelligence#AIGuide#LearnAI#AIJourney#FutureOfTech#TechEducation#AITrends#AIMadeSimple#AIExplained#UnderstandingAI#TechForEveryone#DigitalTransformation
0 notes
Text
What are some effective ways for beginners in data science to learn from other data scientists?
1. Online Courses and MOOCs
Platforms: Websites like Lejhro Bootcamp, edX, and Udacity offer courses taught by industry experts. Engaging with these can provide insights into real-world applications.
2. Mentorship
Finding a Mentor: Look for mentors in your network or through platforms like LinkedIn. Having a mentor can provide personalized guidance and feedback.
3. Community Engagement
Forums and Groups: Join online communities such as Reddit (r/datascience), Stack Overflow, or data science-focused Slack groups to ask questions and participate in discussions.
Meetups and Conferences: Attend local meetups, workshops, and conferences to network and learn from professionals in the field.
4. Collaborative Projects
Kaggle Competitions: Participate in Kaggle competitions where you can collaborate with others, learn from shared code, and see different approaches to problem-solving.
Open Source Projects: Contribute to open source data science projects on GitHub, where you can learn from the code written by others.
5. Blogging and Writing
Writing about Your Learning: Start a blog or contribute to platforms like Medium, sharing your learning journey. Engaging with feedback can deepen your understanding and connect you with others.
6. Reading Books and Research Papers
Books: Read foundational books on data science and specific topics (e.g., “Python for Data Analysis,” “Deep Learning”).
Papers: Follow recent research in data science through platforms like arXiv or Google Scholar to see how experts are tackling problems.
7. Webinars and Podcasts
Listening to Experts: Attend webinars and listen to podcasts featuring experienced data scientists discussing their work, challenges, and insights.
8. Social Media Engagement
Twitter and LinkedIn: Follow data scientists on social media platforms to stay updated on trends and discussions in the field.
9. Practice with Real-World Datasets
Exploratory Data Analysis: Work on publicly available datasets from sources like UCI Machine Learning Repository or Kaggle Datasets, applying techniques you’ve learned and comparing your approach to others.
10. Feedback and Code Reviews
Seek Feedback: Share your projects and analyses with peers or online communities for constructive criticism and suggestions.
0 notes
Text
How do I learn R, Python and data science?
Learning R, Python, and Data Science: A Comprehensive Guide
Choosing the Right Language
R vs. Python: Both R and Python are very powerful tools for doing data science. R is usually preferred for doing statistical analysis and data visualisations, whereas Python is much more general and currently is very popular for machine learning and general-purpose programming. Your choice of which language to learn should consider your specific goals and preferences.
Building a Strong Foundation
Structured Courses Online Courses and Tutorials: Coursera, edX, and Lejhro offer courses and tutorials in R and Python for data science. Look out for courses that develop theoretical knowledge with practical exercises. Practise your skills with hands-on coding challenges using accompanying datasets, offered on websites like Kaggle and DataCamp.
Books: There are enough books to learn R and Python for data science. You may go through the classical ones: "R for Data Science" by Hadley Wickham, and "Python for Data Analysis" by Wes McKinney.
Learning Data Science Concepts
Statistics: Know basic statistical concepts: probability, distribution, hypothesis testing, and regression analysis.
Cleaning and Preprocessing: Learn how to handle missing data techniques, outliers, and data normalisation.
Data Visualization: Expert libraries to provide informative visualisations, including but not limited to Matplotlib and Seaborn in Python and ggplot2 in R.
Machine Learning: Learn algorithms-Linear Regression, Logistic Regression, Decision Trees, Random Forest, Neural Networks, etc.
Deep Learning: Study deep neural network architecture and how to build and train them using the frameworks TensorFlow and PyTorch.
Practical Experience
Personal Projects: In this, you apply your knowledge to personal projects which help in building a portfolio.
Kaggle Competitions: Participate in Kaggle competitions to solve real-world problems in data science and learn from others.
Contributions to Open-Source Projects: Contribute to some open-source projects for data science in order to gain experience and work with other people.
Other Advice
Join Online Communities: Join online forums or communities such as Stack Overflow and Reddit to ask questions, get help, and connect with other data scientists.
Attend Conferences and Meetups: This is a fantastic way to network with similar working professionals in the field and know the latest trends going on in the industry.
Practice Regularly: For becoming proficient in data science, consistent practice is an indispensable element. Devote some time each day for practising coding challenges or personal projects.
This can be achieved by following the above-mentioned steps and having a little bit of dedication towards learning R, Python, and Data Science.
1 note
·
View note
Text
#artificial intelligence#data science#data visualization#jobsearch#python#big data#datawarehousing#datamining
0 notes
Text
Python Basics and Data Analytics: Your First Step Towards Data Mastery
If you’re looking to dive into the world of data science, starting with Python programming and data analytics is a smart move. Python is one of the most popular programming languages, known for its simplicity and versatility, making it an excellent choice for beginners. This guide will help you understand the fundamental concepts and set you on the path to mastering data analytics.
Why Python? Python is favored by many data scientists due to its readability and extensive libraries like Pandas, NumPy, and Matplotlib. These libraries simplify data manipulation, analysis, and visualization, allowing you to focus on drawing insights rather than getting lost in complex code.
Key Concepts to Learn: Start by familiarizing yourself with Python basics, such as variables, data types, loops, and functions. Once you have a good grasp of the fundamentals, you can move on to data analysis concepts like data cleaning, manipulation, and visualization.
Hands-On Practice: The best way to learn is by doing. Engage in projects that allow you to apply your skills, such as analyzing datasets or creating visualizations. Websites like Kaggle provide real-world datasets for practice.
Resources for Learning: Numerous online platforms offer courses and tutorials tailored for beginners. Consider checking out resources on lejhro bootcamp, or even free tutorials on YouTube.
Free Masterclass Opportunity: To enhance your learning experience, there’s a free masterclass available that covers the essentials of Python programming and data analytics. This is a fantastic chance to deepen your understanding and gain valuable insights from experts. Be sure to visit this Python Programming and Data Analytics Fundamentals to secure your spot!
Embarking on your journey into Python programming and data analytics is an exciting opportunity. By building a solid foundation, you’ll be well-equipped to tackle more complex data challenges in the future. So, start your journey today and take that first step towards data mastery!
#DataScience#PythonProgramming#DataAnalytics#LearnPython#DataVisualization#Pandas#NumPy#Kaggle#MachineLearning#DataCleaning#Analytics#TechEducation#FreeMasterclass#OnlineLearning#DataDriven#ProgrammingForBeginners#HandsOnLearning#LejhroBootcamp#DataAnalysis#CodingJourney
1 note
·
View note
Text
What are some reasons why some people may not view data science as a good career choice? Why should someone consider pursuing a career in data science?
Hey there,
Some people may not view data science as a good career choice due to a few misconceptions and challenges in the field.
One reason is the perceived high barrier to entry. Data science requires a mix of skills programming, statistics, and domain knowledge which can feel overwhelming for beginners. People may also be discouraged by the rapid pace of technological change. New tools and algorithms emerge frequently, which means that data scientists must constantly learn and adapt, potentially causing burnout.
Another reason is the misalignment of expectations. Many people believe data science involves glamorous, cutting-edge machine learning projects, but the reality is often much more mundane. A large part of the job involves cleaning and organizing data tasks that can be tedious and less exciting. The hype around data science can lead to disappointment when the actual work doesn’t match the marketing buzz.
However, data science is still an excellent career choice for many reasons. It offers tremendous opportunities for growth and specialization. The demand for skilled data scientists continues to rise across industries like healthcare, finance, and retail, offering job security and high salaries. Plus, the field allows you to solve real-world problems using data, making it highly rewarding for those who enjoy critical thinking and problem-solving.
In short, while there are challenges in data science, it remains a dynamic and fulfilling career for those who are curious, adaptable, and enjoy working with data.
0 notes
Text
#Software testing#software testing types#software testing unit#Integration Testing#software testing software
1 note
·
View note
Text
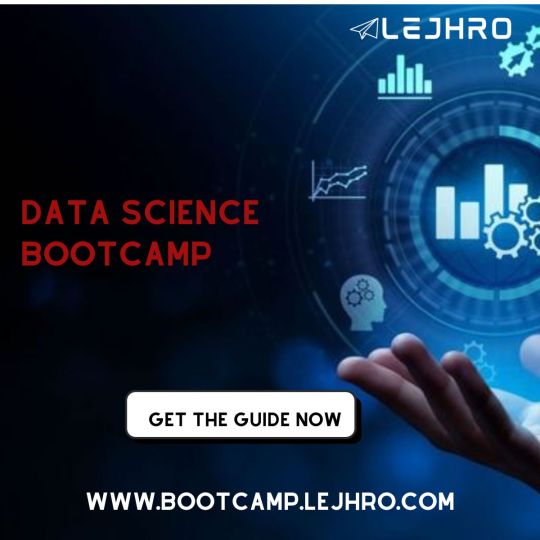
Struggling with a concept? No worries, the Data Science Bootcamp fam is here to help!
0 notes
Text
Technical Support Engineer at LEJHRO | Remote job 2024
Technical Support Engineer at LEJHRO | Remote job 2024 About The Company Lejhro, a pioneering force in the realms of technology, consulting, and outsourcing, emerged onto the corporate landscape in 2013 with a rebellious spirit, driven by an unwavering commitment to leave an indelible mark through technological innovations and remarkable products. Since its inception, the company has…
View On WordPress
0 notes
Text
What is the best way to learn Python quickly and effectively?
How to Learn Python Fast
1. Laying the Basics Right
Online Tutorials and Courses: Websites such as Coursera, edX, and Lejhro have structured courses in various teaching styles.
Interactive Coding Sites: Sites like Codecademy, HackerRank, and LeetCode offer hands-on practice-cum-immediate feedback.
2. Do Practice Regularly
Building Projects: As you go further, implement your knowledge by building minor projects. It helps you get used to the concept and visualizes the usage of Python in real-world applications.
Practice coding challenges: Sites such as Project Euler and Rosalind can help you pinpoint your weaknesses in coding, providing you with problems to improve your skills in problem solving.
3. Join the Community
Online forums and communities: Websites like Stack Overflow and a sub-Reddit named r/learnpython get you help for your questions or just simply hang out with other people learning.
Local meetups: Find Python meetups happening around your area and attend. This will give you contacts with advanced Python programmers where you'll be privileged to listen and learn from their experiences.
4. Learning from Others
Mentorship: Find yourself a mentor who will be able to help you out, answer some questions, and review your code.
Open-source projects: Contribute to open-source projects written in Python. You will be able to learn from senior developers and see how real projects look.
5. Effective Learning
Spaced repetition: Go through regular intervals at which you review for better reinforcement of memory.
Active recall: Test your knowledge by trying to recall something without looking at your notes or resources.
Pomodoro Technique: Break your study into relatively small, focused intervals separated by short breaks.
6. Motivate yourself
Setting reachable objectives: Break down your learning into smaller steps to help you stay motivated.
Celebrate your progress: Give yourself rewards after reaching milestones.
7. Try and Explore
Try different approaches: Do not hesitate to try different ways of learning until you find what works best for you.
Python Libraries: Delve into the world of Python's vast ecosystem of libraries and tools to aid in expanding your knowledge and skill.
Practice these tips habitually, and you will be able to learn Python well enough to attain proficiency in Data Science.
0 notes
Text
In today's data-driven world, the scope of data science is boundless, and its potential is awe-inspiring! 🚀💻
From predicting consumer behavior to optimizing business operations, data science holds the key to unlocking valuable insights that drive innovation and success. As technology advances, so does the demand for skilled data scientists who can make sense of complex datasets and transform them into actionable solutions.
The future of data science is bright, with exciting prospects across industries. From healthcare and finance to marketing and cybersecurity, every sector is embracing the power of data to make informed decisions and gain a competitive edge.
In the coming years, artificial intelligence (AI) and machine learning (ML) will further amplify the impact of data science, revolutionizing the way we live and work. By leveraging algorithms and predictive models, data scientists will transform vast amounts of information into meaningful patterns, enabling personalized experiences, automation, and smarter decision-making.
If you're intrigued by the limitless possibilities of data science, join the journey today @lejhro! Equip yourself with the essential skills, immerse yourself in real-world projects, and embrace the ever-evolving field of data science. Register now: https://www.bootcamp.lejhro.com/data-science-course-training
https://www.bootcamp.lejhro.com/
The future is data-driven, and you can be at the forefront of this exciting revolution. Start your data science adventure now and be part of shaping a brighter tomorrow!
DataScience #FutureTech #Analytics #BigData #AI #MachineLearning #TechRevolution #CareerOpportunities #DataDriven #FutureProspects
1 note
·
View note