#human Brewster
Explore tagged Tumblr posts
Text
New Leaf cafe ☕️
#animal crossing#animal crossing new leaf#animal crossing fandom#Brewster#ac brewster#human Brewster#self insert#cafe#Brewster cafe#roost sable cookie#brewstoid#cup with saucer#humanization#human counterpart#art#artwork#fan art#animal crossing fanart#ac fanart#my art#motion art#3d art#video game art#anime art#illustration#artists on tumblr#3d model#special effects
3 notes
·
View notes
Text
Finally got around to finishing a piece of human Brewster from Animal Crossing that I sketched and lined? several months ago if not a year ago? lol
Now that I've finished this one, I kind of want to do another. Any suggestions on who I should draw as a human next?
#myart#bobbisbaubles#animal crossing#animal crossing new horizons#acnh#brewster#animal crossing brewster#acnh brewster#human brewster#bust#headshot#artists on tumblr
3 notes
·
View notes
Text







Criminal Minds S4E17, Demonology // Mimi Evangeline, from Girlhood is Godhood // Kristin Chang, from Churching // Frank Bidart, from The War of Vaslav Nijinsky // The Dead (1987), dir. John Huston // James Joyce, in a letter to Lady Gregory // James Joyce, from The Dead
#deeeeep in my feelings about emily and her religious trauma#emily who still insists on seeing beauty and wonder wherever she goes#emily who remains concerned with losing her humanity and her ability to put her finger on the pulse of awe#for more of my web weaves ->#mine*ww#criminal minds#emily prentiss#paget brewster#cmedit#criminalmindsedit#webweaving#web weaving#words#criminalmindsverse#cm#james joyce#kristin chang#intertextuality#parallelism
83 notes
·
View notes
Text
Been replaying a lot of New Leaf so here are two of my favorite Special Characters I always enjoy interacting with
#redd isn't taller than brewster in my mind btw its just the way i placed them together#animal crossing#brewster#crazy redd#gijinka#humanization#character art#art#digital art#artists on tumblr#my art
234 notes
·
View notes
Text

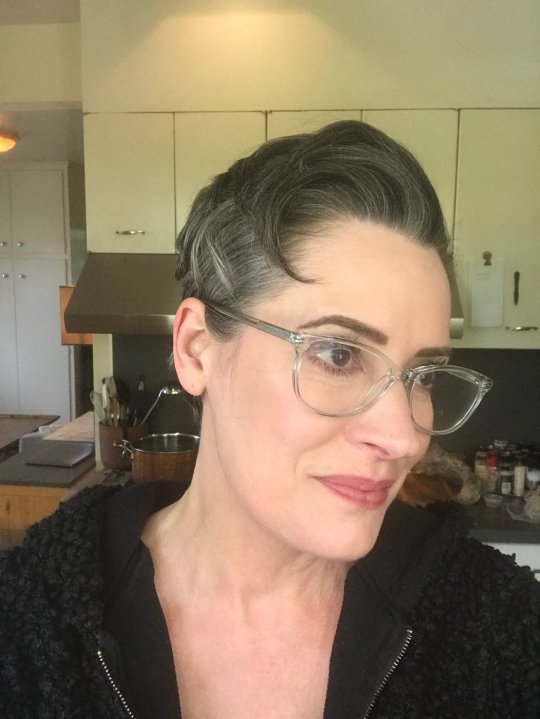

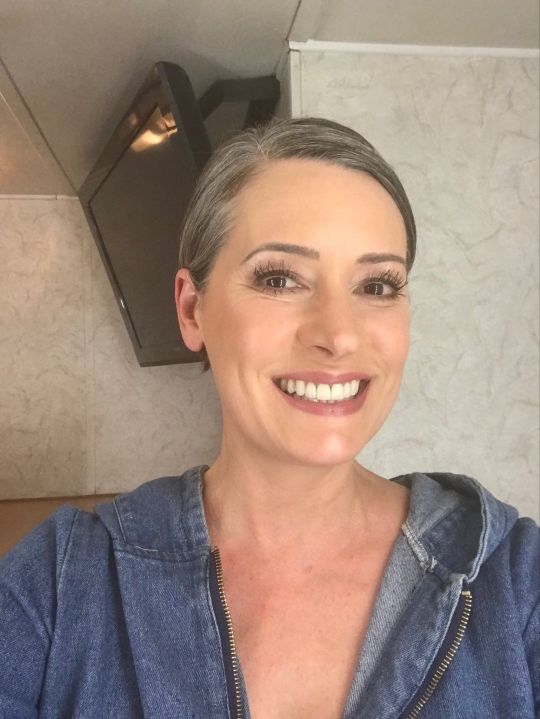

Happy 55th Birthday, to the Gorgeous Human Peacock herself - - Paget V. Brewster
March 10, 1969
227 notes
·
View notes
Text
☕️🌻🍮
#my favorite little pigeon man#out of nowhere I thought about his conversations in wild world#where he starts opening up about his history with blathers#it still gets me it’s so sweet#I’m a simple man you give me a stoic character and make him soft and I’m in shambles#anyway it’s five in the morning :) I have not slept yet ah haha#brewster#animal crossing#humanized
511 notes
·
View notes
Text
Series 12 will always be my favorite season of Bake Off. You mean to tell ME we were blessed with Chigs, Crystelle, Jurgen, Giuseppe, George, Rochica, Freya AND MOST IMPORTANTLY LIZZIE ALL IN ONE TENT???
The moment Lizzie said "I'm gonna stare him down" in her Scouse accent, I was immediately in love.
#GBBO#Lizzie Acker#I follow her on every social media account I have#I cannot express how much I love her#Staaa 'im dowwn#The way she's says “I'm so behiiiiiind” and there are like 6 extra syllables of the letter i#Absolutely adore her#Freya is a close second#“it's all a bit of a faff isn't it really”#I still say “oh thank you so much” in freya's accent all the time#My comfort show#My comfort season#I named my Stardew Valley Chickens after them all#Also#Giuseppe is the human version of Brewster from Animal Crossing#And I am the American Walmart version of Noel Fielding#I want his smiley face sweater from cake week
16 notes
·
View notes
Text

Humanized Chuggington
@masterj
I think I'll call my au, "ChuggingKids" :)
#chuggington#chuggington wilson#chuggington brewster#chuggington koko#my art#humanized#humanoids#anthro#chuggington humanized#chuggington au#chuggingkids
14 notes
·
View notes
Text
4 years ago today, the Adventure Time Distant Lands episode The Obsidian premiered on HBO Max!!!
P2
I have the entire album of this episode on my Spotify playlist!!!🥰🥰🥰
#Adventure Time#Adventure Time Obsidian#cartoonetwork#HBO MAX#marcline the vampire queen#princess bubblegum#Bonnibel Bonnie Bubblegum#Simon Petrikov#Bronwyn#Finn#finn the human#Pendleton Ward#Adam Muto#Miki Brewster#Jeff Liu#Casey James Basichis#Tim Kiefer#Amanda Jones#Dory Bavarsky#Niv Bavarsky#Karen Havey#Fred Seibert#Jennifer Pelphrey#Tramm Wigzell#Brian A. Miller#Rob Sorcher#Sam Register#Scott Malchus
13 notes
·
View notes
Text

Is the animal crossing fandom even alive?
Isabelle and Brewster as humans !! (l'II probably do more characters in the future
#art#drawing#digital art#procreate#digital fanart#digital#acnh community#acnh#acnh villagers#isabelle#brewster#isabelle and brewster as humans#acnh characters as humans#animal crossing#animal crossing new horizons#fanart
37 notes
·
View notes
Text
Original:

#meme redraw#im a simp#ratchet and clank rift apart#destroy all humans#dislyte brewster#rocket raccoon#peppino spaghetti#unicorn warriors eternal#miguel o'hara#helluva striker#welcome home wally
7 notes
·
View notes
Text
The Great Rivalry
I’ve been laid up in bed all week with covid and decided to read “Dracula” for the millionth? time (hey who’s counting). It’s a timeless story about good versus evil battling for dominance. Being the vampire geek that I am, I was again awed at the epic battle between Dracula and Van Helsing. Dracula will stop at nothing to get what he wants from the humans around him, while Van Helsing will stop at nothing to protect them. This powerful human and vampire have become the paradigm for every human and vampire rivalry that has come after.
One such rivalry that has always captivated me is Jerry Dandridge and Charley Brewster from “Fright Night.” I admit that Jerry and Peter Vincent would be a better fit, but there’s always been a certain something about the struggle between Jerry and Charley that grips me in a such a way like no other. With the release of “Fright Night: Origins,” my interest in exploring their dynamic has piqued again. Jerry and Charley represent the struggle between good versus evil, yes, but are so much more: shadow and light, confidence and fear, corruption and innocence, vice and virtue, despair and hope, hate and love, respectively.
I could write a whole damn essay on this. In fact, I think I will.
What are some of your favorite vampire/human rivalries in literature and on the big screen?
#dracula#van helsing#vampire and human#fright night 1985#fright night 2011#fright night origins#jerry dandridge#charley brewster#essay#fright night movie#the shadow self
3 notes
·
View notes
Photo
This fell out of my queue and now I just keep laughing in context of S17. Might I present Emily and Fuckin' Brian 🤣
Frank and Sadie Doyle being absolutely perfect and adorable. (x)
#Sadie Doyle#frank doyle#thrilling adventure hour#otp: clink#paget brewster#paul f tompkins#gorgeous human peacock
433 notes
·
View notes
Text

Paget "15% on the Kinsey Scale" Brewster
#Criminal Minds#Paget Brewster#Cm cast#why is she like this#Gorgeous human peacock#Meanwhile on twitter#Cm reference#Emily Prentiss#cm evolution#Twitter
134 notes
·
View notes
Text
AI’s “human in the loop” isn’t

I'll be in TUCSON, AZ from November 8-10: I'm the GUEST OF HONOR at the TUSCON SCIENCE FICTION CONVENTION.
AI's ability to make – or assist with – important decisions is fraught: on the one hand, AI can often classify things very well, at a speed and scale that outstrips the ability of any reasonably resourced group of humans. On the other hand, AI is sometimes very wrong, in ways that can be terribly harmful.
Bureaucracies and the AI pitchmen who hope to sell them algorithms are very excited about the cost-savings they could realize if algorithms could be turned loose on thorny, labor-intensive processes. Some of these are relatively low-stakes and make for an easy call: Brewster Kahle recently told me about the Internet Archive's project to scan a ton of journals on microfiche they bought as a library discard. It's pretty easy to have a high-res scanner auto-detect the positions of each page on the fiche and to run the text through OCR, but a human would still need to go through all those pages, marking the first and last page of each journal and identifying the table of contents and indexing it to the scanned pages. This is something AI apparently does very well, and instead of scrolling through endless pages, the Archive's human operator now just checks whether the first/last/index pages the AI identified are the right ones. A project that could have taken years is being tackled with never-seen swiftness.
The operator checking those fiche indices is something AI people like to call a "human in the loop" – a human operator who assesses each judgment made by the AI and overrides it should the AI have made a mistake. "Humans in the loop" present a tantalizing solution to algorithmic misfires, bias, and unexpected errors, and so "we'll put a human in the loop" is the cure-all response to any objection to putting an imperfect AI in charge of a high-stakes application.
But it's not just AIs that are imperfect. Humans are wildly imperfect, and one thing they turn out to be very bad at is supervising AIs. In a 2022 paper for Computer Law & Security Review, the mathematician and public policy expert Ben Green investigates the empirical limits on human oversight of algorithms:
https://papers.ssrn.com/sol3/papers.cfm?abstract_id=3921216
Green situates public sector algorithms as the latest salvo in an age-old battle in public enforcement. Bureaucracies have two conflicting, irreconcilable imperatives: on the one hand, they want to be fair, and treat everyone the same. On the other hand, they want to exercise discretion, and take account of individual circumstances when administering justice. There's no way to do both of these things at the same time, obviously.
But algorithmic decision tools, overseen by humans, seem to hold out the possibility of doing the impossible and having both objective fairness and subjective discretion. Because it is grounded in computable mathematics, an algorithm is said to be "objective": given two equivalent reports of a parent who may be neglectful, the algorithm will make the same recommendation as to whether to take their children away. But because those recommendations are then reviewed by a human in the loop, there's a chance to take account of special circumstances that the algorithm missed. Finally, a cake that can be both had, and eaten!
For the paper, Green reviewed a long list of policies – local, national, and supra-national – for putting humans in the loop and found several common ways of mandating human oversight of AI.
First, policies specify that algorithms must have human oversight. Many jurisdictions set out long lists of decisions that must be reviewed by human beings, banning "fire and forget" systems that chug along in the background, blithely making consequential decisions without anyone ever reviewing them.
Second, policies specify that humans can exercise discretion when they override the AI. They aren't just there to catch instances in which the AI misinterprets a rule, but rather to apply human judgment to the rules' applications.
Next, policies require human oversight to be "meaningful" – to be more than a rubber stamp. For high-stakes decisions, a human has to do a thorough review of the AI's inputs and output before greenlighting it.
Finally, policies specify that humans can override the AI. This is key: we've all encountered instances in which "computer says no" and the hapless person operating the computer just shrugs their shoulders apologetically. Nothing I can do, sorry!
All of this sounds good, but unfortunately, it doesn't work. The question of how humans in the loop actually behave has been thoroughly studied, published in peer-reviewed, reputable journals, and replicated by other researchers. The measures for using humans to prevent algorithmic harms represent theories, and those theories are testable, and they have been tested, and they are wrong.
For example, people (including experts) are highly susceptible to "automation bias." They defer to automated systems, even when those systems produce outputs that conflict with their own expert experience and knowledge. A study of London cops found that they "overwhelmingly overestimated the credibility" of facial recognition and assessed its accuracy at 300% better than its actual performance.
Experts who are put in charge of overseeing an automated system get out of practice, because they no longer engage in the routine steps that lead up to the conclusion. Presented with conclusions, rather than problems to solve, experts lose the facility and familiarity with how all the factors that need to be weighed to produce a conclusion fit together. Far from being the easiest step of coming to a decision, reviewing the final step of that decision without doing the underlying work can be much harder to do reliably.
Worse: when algorithms are made "transparent" by presenting their chain of reasoning to expert reviewers, those reviewers become more deferential to the algorithm's conclusion, not less – after all, now the expert has to review not just one final conclusion, but several sub-conclusions.
Even worse: when humans do exercise discretion to override an algorithm, it's often to inject the very bias that the algorithm is there to prevent. Sure, the algorithm might give the same recommendation about two similar parents who are facing having their children taken away, but the judge who reviews the recommendations is more likely to override it for a white parent than for a Black one.
Humans in the loop experience "a diminished sense of control, responsibility, and moral agency." That means that they feel less able to override an algorithm – and they feel less morally culpable when they sit by and let the algorithm do its thing.
All of these effects are persistent even when people know about them, are trained to avoid them, and are given explicit instructions to do so. Remember, the whole reason to introduce AI is because of human imperfection. Designing an AI to correct human imperfection that only works when its human overseer is perfect produces predictably bad outcomes.
As Green writes, putting an AI in charge of a high-stakes decision, and using humans in the loop to prevent its harms, produces a "perverse effect": "alleviating scrutiny of government algorithms without actually addressing the underlying concerns." The human in the loop creates "a false sense of security" that sees algorithms deployed for high-stakes domains, and it shifts the responsibility for algorithmic failures to the human, creating what Dan Davies calls an "accountability sink":
https://profilebooks.com/work/the-unaccountability-machine/
The human in the loop is a false promise, a "salve that enables governments to obtain the benefits of algorithms without incurring the associated harms."
So why are we still talking about how AI is going to replace government and corporate bureaucracies, making decisions at machine speed, overseen by humans in the loop?
Well, what if the accountability sink is a feature and not a bug. What if governments, under enormous pressure to cut costs, figure out how to also cut corners, at the expense of people with very little social capital, and blame it all on human operators? The operators become, in the phrase of Madeleine Clare Elish, "moral crumple zones":
https://estsjournal.org/index.php/ests/article/view/260
As Green writes:
The emphasis on human oversight as a protective mechanism allows governments and vendors to have it both ways: they can promote an algorithm by proclaiming how its capabilities exceed those of humans, while simultaneously defending the algorithm and those responsible for it from scrutiny by pointing to the security (supposedly) provided by human oversight.
Tor Books as just published two new, free LITTLE BROTHER stories: VIGILANT, about creepy surveillance in distance education; and SPILL, about oil pipelines and indigenous landback.

If you'd like an essay-formatted version of this post to read or share, here's a link to it on pluralistic.net, my surveillance-free, ad-free, tracker-free blog:
https://pluralistic.net/2024/10/30/a-neck-in-a-noose/#is-also-a-human-in-the-loop
Image: Cryteria (modified) https://commons.wikimedia.org/wiki/File:HAL9000.svg
CC BY 3.0 https://creativecommons.org/licenses/by/3.0/deed.en ==
289 notes
·
View notes
Note
Omg that last addition to the AC 141 was so cute!!! It just makes me wonder how badly they would freak out when their human misses when trying to catch a tarantula or scorpion and passes out from the sting… high risk high reward
previous
Soap rushes to the museum cafe when it happens, and finds the old lion at a corner table enjoying a cappuccino to some smooth jazz.
“Captain,” he pants, hands on his knees, “the human got stung.”
Price is unperturbed. He blows a little stream of cool air over his mug, eyes closed with pleasure. “Happened before, Soap. Just needs a little medicine, remember? They’ll be fine.”
“No, sir,” Soap gasps, “scorpion.”
A beat. For a moment, he thinks Price won’t react. He remains completely still, expression totally neutral—
Suddenly the lion flings the mug away, splashing an arc of coffee across the table, and then he’s moving fast, sprinting past an indignant, sputtering Brewster, and it’s all Soap can do after his mad dash to the museum to keep up. He doesn’t know exactly how Price knows which direction to run, but he figures it has something to do with the smartphone Price gave you when you first arrived—it hardly matters. They come upon you quickly, on the south side of the island, lying facedown in the grass and attended by Ghost and Gaz.
“Captain!” Gaz barks, visibly panicking. “They won’t wake up!”
Ghost is less flustered than the young wolf but equally distressed. “Price, we gotta do somethin’ fast, humans don’t take well to venom.”
“How the bloody hell did this happen?” Price growls. He gets on his knees to turn you over; you’re out cold, but still breathing easily.
“They were…” Gaz trails, looking bemused.
“Chasing it,” Ghost finishes. “With a net.”
Price blinks several times. He looks between the bear and the lion, and then at the horse. Soap raises both hands.
“What, are we supposed to stop them?” he demands. “You said we gotta let humans be humans, boss, sometimes they’re—well, er, they’re…”
“Mortally stupid,” Price supplies, bushy brows lowering. “Alright. Help me get ‘em home. They should be comfortable at least, while we figure out what to do.”
He says it perfunctorily, as if he’s ambivalent to your life or death, but when Price lifts your shoulders it’s the gentlest any of the 141 have ever seen him touch another living being. Ghost gets a hold on your legs, and between the two of them, with the younger animals following behind, they make it to your front doorstep.
Then—the moment they reach your door, like magic, your eyes suddenly pop open. It startles everyone so badly, Gaz and Soap jump back with shock.
“Price?” you say, blinking. “Ghost?”
“We’re here,” says the lion. “How do you feel, kid?”
“I’m fine,” you say. “What’s going on?”
“You passed out,” Ghost says. “We saw it happen—don’t you remember the scorpion?”
“Gave us a bloody heart attack!” Gaz cries.
“Ohhhh yeah,” you say. “Oh, I’m okay, guys. It’s not the first time it’s happened.”
Dead silence. Soap’s mouth drops open. Price is as still as a statue.
Ghost lets your feet drop to the ground. “Bloody fuckin’ hell.”
He turns away and stalks off, muttering under his breath. If you’re shocked by the profanity (a violation of another of Price’s many rules), you don’t show it.
“Thanks for bringing me home,” you say, looking innocently up to the lion. “I wanted to change my outfit anyway.”
Wordlessly, Price sets you down. You wave to the three remaining animals, and disappear inside.
“My house,” the lion says wearily. He appears as though he’s aged ten years in five minutes. “Someone get Ghost. I’m breaking out the whisky.”
116 notes
·
View notes