#foundations of discrete mathematics
Explore tagged Tumblr posts
Text
There's this slideshow available online which is really helpful in understanding the various signs used in mathematics
The link is: https://slideplayer.com/slide/17176137/
Here's a preview:
#the relief i feel when i find explanatory stuff online#resources for studying#resources#sources#math resources#math#maths#mathematics#pcm#jee#stem#engineering#dr. dalia m. gil#foundations of discrete mathematics
7 notes
·
View notes
Text
Understanding Different Types of Variables in Statistical Analysis
Summary: This blog delves into the types of variables in statistical analysis, including quantitative (continuous and discrete) and qualitative (nominal and ordinal). Understanding these variables is critical for practical data interpretation and statistical analysis.

Introduction
Statistical analysis is crucial in research and data interpretation, providing insights that guide decision-making and uncover trends. By analysing data systematically, researchers can draw meaningful conclusions and validate hypotheses.
Understanding the types of variables in statistical analysis is essential for accurate data interpretation. Variables representing different data aspects play a crucial role in shaping statistical results.
This blog aims to explore the various types of variables in statistical analysis, explaining their definitions and applications to enhance your grasp of how they influence data analysis and research outcomes.
What is Statistical Analysis?
Statistical analysis involves applying mathematical techniques to understand, interpret, and summarise data. It transforms raw data into meaningful insights by identifying patterns, trends, and relationships. The primary purpose is to make informed decisions based on data, whether for academic research, business strategy, or policy-making.
How Statistical Analysis Helps in Drawing Conclusions
Statistical analysis aids in concluding by providing a structured approach to data examination. It involves summarising data through measures of central tendency (mean, median, mode) and variability (range, variance, standard deviation). By using these summaries, analysts can detect trends and anomalies.
More advanced techniques, such as hypothesis testing and regression analysis, help make predictions and determine the relationships between variables. These insights allow decision-makers to base their actions on empirical evidence rather than intuition.
Types of Statistical Analyses
Analysts can effectively interpret data, support their findings with evidence, and make well-informed decisions by employing both descriptive and inferential statistics.
Descriptive Statistics: This type focuses on summarising and describing the features of a dataset. Techniques include calculating averages and percentages and crating visual representations like charts and graphs. Descriptive statistics provide a snapshot of the data, making it easier to understand and communicate.
Inferential Statistics: Inferential analysis goes beyond summarisation to make predictions or generalisations about a population based on a sample. It includes hypothesis testing, confidence intervals, and regression analysis. This type of analysis helps conclude a broader context from the data collected from a smaller subset.
What are Variables in Statistical Analysis?
In statistical analysis, a variable represents a characteristic or attribute that can take on different values. Variables are the foundation for collecting and analysing data, allowing researchers to quantify and examine various study aspects. They are essential components in research, as they help identify patterns, relationships, and trends within the data.
How Variables Represent Data
Variables act as placeholders for data points and can be used to measure different aspects of a study. For instance, variables might include test scores, study hours, and socioeconomic status in a survey of student performance.
Researchers can systematically analyse how different factors influence outcomes by assigning numerical or categorical values to these variables. This process involves collecting data, organising it, and then applying statistical techniques to draw meaningful conclusions.
Importance of Understanding Variables
Understanding variables is crucial for accurate data analysis and interpretation. Continuous, discrete, nominal, and ordinal variables affect how data is analysed and interpreted. For example, continuous variables like height or weight can be measured precisely. In contrast, nominal variables like gender or ethnicity categorise data without implying order.
Researchers can apply appropriate statistical methods and avoid misleading results by correctly identifying and using variables. Accurate analysis hinges on a clear grasp of variable types and their roles in the research process, interpreting data more reliable and actionable.
Types of Variables in Statistical Analysis

Understanding the different types of variables in statistical analysis is crucial for practical data interpretation and decision-making. Variables are characteristics or attributes that researchers measure and analyse to uncover patterns, relationships, and insights. These variables can be broadly categorised into quantitative and qualitative types, each with distinct characteristics and significance.
Quantitative Variables
Quantitative variables represent measurable quantities and can be expressed numerically. They allow researchers to perform mathematical operations and statistical analyses to derive insights.
Continuous Variables
Continuous variables can take on infinite values within a given range. These variables can be measured precisely, and their values are not limited to specific discrete points.
Examples of continuous variables include height, weight, temperature, and time. For instance, a person's height can be measured with varying degrees of precision, from centimetres to millimetres, and it can fall anywhere within a specific range.
Continuous variables are crucial for analyses that require detailed and precise measurement. They enable researchers to conduct a wide range of statistical tests, such as calculating averages and standard deviations and performing regression analyses. The granularity of continuous variables allows for nuanced insights and more accurate predictions.
Discrete Variables
Discrete variables can only take on separate values. Unlike continuous variables, discrete variables cannot be subdivided into finer increments and are often counted rather than measured.
Examples of discrete variables include the number of students in a class, the number of cars in a parking lot, and the number of errors in a software application. For instance, you can count 15 students in a class, but you cannot have 15.5 students.
Discrete variables are essential when counting or categorising is required. They are often used in frequency distributions and categorical data analysis. Statistical methods for discrete variables include chi-square tests and Poisson regression, which are valuable for analysing count-based data and understanding categorical outcomes.
Qualitative Variables
Qualitative or categorical variables describe characteristics or attributes that cannot be measured numerically but can be classified into categories.
Nominal Variables
Nominal variables categorise data without inherent order or ranking. These variables represent different categories or groups that are mutually exclusive and do not have a natural sequence.
Examples of nominal variables include gender, ethnicity, and blood type. For instance, gender can be classified as male, female, and non-binary. However, there is no inherent ranking between these categories.
Nominal variables classify data into distinct groups and are crucial for categorical data analysis. Statistical techniques like frequency tables, bar charts, and chi-square tests are commonly employed to analyse nominal variables. Understanding nominal variables helps researchers identify patterns and trends across different categories.
Ordinal Variables
Ordinal variables represent categories with a meaningful order or ranking, but the differences between the categories are not necessarily uniform or quantifiable. These variables provide information about the relative position of categories.
Examples of ordinal variables include education level (e.g., high school, bachelor's degree, master's degree) and customer satisfaction ratings (e.g., poor, fair, good, excellent). The categories have a specific order in these cases, but the exact distance between the ranks is not defined.
Ordinal variables are essential for analysing data where the order of categories matters, but the precise differences between categories are unknown. Researchers use ordinal scales to measure attitudes, preferences, and rankings. Statistical techniques such as median, percentiles, and ordinal logistic regression are employed to analyse ordinal data and understand the relative positioning of categories.
Comparison Between Quantitative and Qualitative Variables
Quantitative and qualitative variables serve different purposes and are analysed using distinct methods. Understanding their differences is essential for choosing the appropriate statistical techniques and drawing accurate conclusions.
Measurement: Quantitative variables are measured numerically and can be subjected to arithmetic operations, whereas qualitative variables are classified without numerical measurement.
Analysis Techniques: Quantitative variables are analysed using statistical methods like mean, standard deviation, and regression analysis, while qualitative variables are analysed using frequency distributions, chi-square tests, and non-parametric techniques.
Data Representation: Continuous and discrete variables are often represented using histograms, scatter plots, and box plots. Nominal and ordinal variables are defined using bar charts, pie charts, and frequency tables.
Frequently Asked Questions
What are the main types of variables in statistical analysis?
The main variables in statistical analysis are quantitative (continuous and discrete) and qualitative (nominal and ordinal). Quantitative variables involve measurable data, while qualitative variables categorise data without numerical measurement.
How do continuous and discrete variables differ?
Continuous variables can take infinite values within a range and are measured precisely, such as height or temperature. Discrete variables, like the number of students, can only take specific, countable values and are not subdivisible.
What are nominal and ordinal variables in statistical analysis?
Nominal variables categorise data into distinct groups without any inherent order, like gender or blood type. Ordinal variables involve categories with a meaningful order but unequal intervals, such as education levels or satisfaction ratings.
Conclusion
Understanding the types of variables in statistical analysis is crucial for accurate data interpretation. By distinguishing between quantitative variables (continuous and discrete) and qualitative variables (nominal and ordinal), researchers can select appropriate statistical methods and draw valid conclusions. This clarity enhances the quality and reliability of data-driven insights.
#Understanding Different Types of Variables in Statistical Analysis#Variables in Statistical Analysis#Statistical Analysis#statistics#data science
4 notes
·
View notes
Text
Math isn't as universal as I thought...
Whenever we talk about STEM, or science, technology, engineering, and mathematics, we often say that everything boils down to just the “M”- math. It is the foundation of everything else, which is why we see so many STEM majors in college that have to take mathematics courses all the way through the Calculus track, usually adding in courses like Differential Equations, Linear Algebra, or Discrete Mathematics as well. As much as mathematics ties into every other STEM major, is it a universal language? Is it something that can be shared across many cultures, despite language barriers?
Whiteford writes on how English Language Learners may have been brought up in different cultures that have different significant numbers, use different measuring systems, and have different methods for even our most basic functions like adding, subtracting, multiplying, and dividing. As much as our calculators may spit out the same values no matter where we are, how we rationalize why that value is true can be so different depending on where we learned math.
This week I spent time working on understanding the physics tools in Blender. The lessons started off by explaining how mathematics ties into physics and is the foundation of STEM. I learned how to animate a bowling ball rolling towards 10 pins and knocking them over through the use of the physics mechanics in Blender. I also learned how to model cloth falling onto a ball, a wrecking ball knocking into a pile of cubes, and more. Having access to these mechanics allowed for more realistic looking animations that also allowed me to see where mathematics can truly be applied to modeling, rather than applying modeling to the understanding of mathematics.
Whiteford, Tim. “Is Mathematics a Universal Language?” Teaching Children Mathematics, vol. 16, no. 5, 2009, pp. 276–83. JSTOR, http://www.jstor.org/stable/41199460. Accessed 30 Oct. 2023.
Blender lessons from Sebastián Vargas Molano’s course titled The 2023 Blender Primer: 3D Modeling, Animation, & Rendering
2 notes
·
View notes
Text
Jorge Urrutia Galicia: A Mexican Pioneering Mathematician And Computer Scientist

Jorge Urrutia Galicia is a Mexican computer scientist and mathematician.
Galicia is best known for his work on geometry. He made contributions to many different areas of mathematics, including discrete geometry, discrete optimization, and computational geometry. His specialty in computational geometry has made him recognized as one of the leading researchers worldwide. His research has also focused on combinatorial optimization, which is related to combinatorial game theory.
His early works dealt with problems of separability and visibility, a field in which he is an indisputable authority. While it is clear that mathematics has always played a basic role as the underlying foundation of all technology, especially now, and in this case it is confirmed why the technological scope of Dr. Urrutia’s articles in routing is significant; suffice to mention just one: recently algorithms are being implemented based on the ideas of Dr. Urrutia, to make communication networks that can be used in case of natural disasters.
Since the end of the 20th century, he began to work on routing problems, developing algorithms for both the combinatorial and geometric problems, which literally founded a work area of great importance in its application to wireless and cellular networks. In the 21st century, Dr. Urrutia has also stood out for his numerous contributions to the study of discrete sets of points, on which he has made decisive contributions, both in their solution and formulating various variants.
Dr. Jorge Urrutia Galicia studied a bachelor’s degree in mathematics at the Faculty of Sciences of UNAM from 1971 to 1974, and a master’s and doctoral degree in mathematics at the University of Waterloo, Canada from 1976 to 1980. He has worked at the Metropolitan Autonomous University-Iztapalapa, CIMAT, Carleton University, Ottawa University from 1984-1998, where he was "full professor", and since 1998 at the Institute of Mathematics of the UNAM. On average, he teaches five courses each year (two undergraduate and three postgraduate courses).
Annually, he organizes at least two research workshops in Mexico, one of its main objectives being that its students know and work with renowned researchers and learn to collaborate with them as equals.
From 1990 to 2000, he was editor-in-chief of the journal Computational Geometry, Theory and Applications, published by Elsevier Science Publishers. He has been a member of the editorial boards of the Mexican Mathematical Society Bulletin and of Graphs and Combinatorics (Springer, and Computational Geometry: Theory and Applications (Elsevier). He was also editor of the Handbook of Computational Geometry (2000), one of Elsevier's first published handbooks.
He has published more than 270 articles in conference proceedings and research journals in mathematics and computing, which have received more than 6,000 citations, among the most important are two articles on routing in ad-hoc and wireless networks, which have received more than 2 600 citations together: “Compass Routing in Geometric Graphs” and “Routing with Guaranteed Delivery in Ad Hoc Wireless Networks.” In these investigations, Dr. Urrutia develops new strategies – highly efficient – to send information on wireless networks that take advantage of the characteristics obtained by recent technologies such as GPS, in addition to allowing them to travel through these networks effectively without having knowledge of their topology. It is worth mentioning that in 2012 he was the most cited mathematician of the UNAM.
He has given more than 40 plenary lectures at international congresses on Computational Geometry. He was editor-in-chief of "Computational Geometry, Theory and Applications" from 1990 to 2000. He has supervised more than 55 bachelor, master and doctoral theses.
In 2015, he received the "National University in Research in Exact Sciences" award at UNAM. He is is a member of the National System of Investigators, Level 3 He has organized and participated in the organizing committees of several national congresses including the "Victor Neumann-Lara Colloquium on Graphic Theory and its Applications", the "Canadian Conference on Computational Geometry", the "Japan Conference on Discrete and Computational Geometry" and the "Computational Geometry Meetings" (Spain). Oher countries where he has also participated in this way are Italy, Indonesia, Philippines, China, Canada, Peru and Argentina, as well as his home country, Mexico.
Source: (x) (x) (x)
#🇲🇽#STEM#Jorge Urrutia Galicia#mexico#UNAM#mathematics#geometry#computer science#mexican#latino#hispanic#discrete optimization#combinatorial game theory#natural disaster#technology#wireless network#cellular#Metropolitan Autonomous University-Iztapalapa#CIMAT#Carleton University#Ottawa University#Institute of Mathematics#Elsevier Science Publishers#Mexican Mathematical Society Bulletin#National University in Research in Exact Sciences#canada#europe#spain#japan#italy
4 notes
·
View notes
Text
Everything Is Computation
These days see a tremendous number of significant scientific news, and it is hard to say which one has the highest significance. Climate models indicate that we are past crucial tipping points and are irrevocably headed for a new, difficult age for our civilization. Mark Van Raamsdonk expands on the work of Brian Swingle and Juan Maldacena, and demonstrates how we can abolish the idea of spacetime in favor of a discrete tensor network, thus opening the way for a unified theory of physics. Bruce Conklin, George Church and others have given us CRISPR, a technology that holds the promise for simple and ubiquitous gene editing. Deep Learning starts to tell us how hierarchies of interconnected feature detectors can autonomously form a model of the world, learn to solve problems, and recognize speech, images and video.
It is perhaps equally important to notice where we lack progress: sociology fails to teach us how societies work, philosophy seems to have become barren and infertile, the economical sciences seem to be ill-equipped to inform our economic and fiscal policies, psychology does not comprehend the logic of our psyche, and neuroscience tells us where things happen in the brain, but largely not what they are.
In my view, the 20th century’s most important addition to understanding the world is not positivist science, computer technology, spaceflight, or the foundational theories of physics. It is the notion of computation. Computation, at its core, and as informally described as possible, is very simple: every observation yields a set of discernible differences.
These, we call information. If the observation corresponds to a system that can change its state, we can describe these state changes. If we identify regularity in these state changes, we are looking at a computational system. If the regularity is completely described, we call this system an algorithm. Once a system can perform conditional state transitions and revisit earlier states, it becomes almost impossible to stop it from performing arbitrary computation. In the infinite case, that is, if we allow it to make an unbounded number of state transitions and use unbounded storage for the states, it becomes a Turing Machine, or a Lambda Calculus, or a Post machine, or one of the many other, mutually equivalent formalisms that capture universal computation.
Computational terms rephrase the idea of "causality," something that philosophers have struggled with for centuries. Causality is the transition from one state in a computational system into the next. They also replace the concept of "mechanism" in mechanistic, or naturalistic philosophy. Computationalism is the new mechanism, and unlike its predecessor, it is not fraught with misleading intuitions of moving parts.
Computation is different from mathematics. Mathematics turns out to be the domain of formal languages, and is mostly undecidable, which is just another word for saying uncomputable (since decision making and proving are alternative words for computation, too). All our explorations into mathematics are computational ones, though. To compute means to actually do all the work, to move from one state to the next.
Computation changes our idea of knowledge: instead of treating it as justified true belief, knowledge describes a local minimum in capturing regularities between observables. Knowledge is almost never static, but progressing on a gradient through a state space of possible world views. We will no longer aspire to teach our children the truth, because like us, they will never stop changing their minds. We will teach them how to productively change their minds, how to explore the never ending land of insight.
A growing number of physicists understand that the universe is not mathematical, but computational, and physics is in the business of finding an algorithm that can reproduce our observations. The switch from uncomputable, mathematical notions (such as continuous space) makes progress possible. Climate science, molecular genetics, and AI are computational sciences. Sociology, psychology, and neuroscience are not: they still seem to be confused by the apparent dichotomy between mechanism (rigid, moving parts) and the objects of their study. They are looking for social, behavioral, chemical, neural regularities, where they should be looking for computational ones.
Everything is computation.
6 notes
·
View notes
Text
Explore BSc Mathematics Subjects at Shiv Nadar University for a Bright Future!
At Shiv Nadar University, our BSc Mathematics program offers a diverse range of subjects designed to build a strong foundation in mathematical concepts and applications. Students explore topics such as calculus, algebra, statistics, and discrete mathematics, alongside advanced electives in areas like mathematical modeling and computational mathematics. Our experienced faculty and state-of-the-art facilities ensure a comprehensive learning experience, preparing graduates for careers in academia, finance, data science, and more. Join us to unlock your potential in the world of mathematics!
0 notes
Text
The Brain's Learning System: A Natural Process of Growth

What if we thought about our brain not just as a static organ, but as a dynamic learning system—one that gradually develops and refines itself through experience and practice? The beauty is that this system is always ready to grow. It just needs the right conditions and consistent engagement.
Understanding Neural Growth
Think back to your last "aha!" moment—that instant where everything just clicked. That wasn't just a random occurrence—it was your brain forming new neural pathways, strengthening connections through a process neuroscientists call synaptic plasticity. This biological process is your brain's way of adapting to new challenges and learning.
Three Pathways to Cognitive Development
Growing your cognitive capabilities isn't about grinding harder; it's about upgrading smarter. Here are three evidence-based approaches to trigger your next system update:
Progressive Challenge Gradually expose yourself to increasingly complex material in your areas of interest. Read papers slightly above your current understanding. Engage in discussions that stretch your thinking. Your brain builds new connections most effectively when working at the edge of your current capabilities.
Cross-Domain Learning Research shows that learning across different domains enhances cognitive flexibility. Whether it's combining music with mathematics, or art with programming, each new skill you develop creates neural networks that can strengthen your primary areas of expertise. Choose activities that interest you and commit to steady progress.
Structured Stress and Recovery Moderate challenge, followed by adequate rest and reflection, creates optimal conditions for learning. Take on projects that push your boundaries, but remember to build in time for processing and integration. This rhythm of engagement and recovery allows for sustainable growth.
Observable Changes
Here’s what happens when your system updates:
Sharper Intuition: Decision-making becomes faster and more accurate. You just "know."
Enhanced Pattern Recognition: You see patterns and connections across different domains that others might miss.
Increased Creative Connections: Ideas flow like a well-oiled machine, linking seemingly unrelated concepts.
Greater Metacognitive Awareness: You gain clarity about how you think and learn, which enhances your growth potential.
The Continuous Nature of Growth
Learning isn't about passing discrete tests or reaching fixed levels—it's an ongoing journey of small improvements. Each challenge you tackle builds upon previous learning, creating an increasingly rich foundation for future growth. There’s no endpoint; there’s always room for refinement and development.
Your Learning Journey
Consider this: What small step could you take today toward expanding your understanding? Perhaps it's spending 30 minutes with a challenging book, starting a new creative project, or engaging with someone whose expertise differs from yours.
Remember that cognitive development is gradual and cumulative. The key isn't dramatic transformation but consistent, intentional engagement with learning opportunities. Your brain's capacity for growth is remarkable—it just needs your patient, persistent participation in the process.
What matters most isn't reaching some predefined level of achievement, but maintaining curiosity and commitment to gradual improvement. The journey of learning itself becomes the reward.
Take Action Towards Financial Independence
If this article has sparked your interest in the transformative potential of Bitcoin, there's so much more to explore! Dive deeper into the world of financial independence and revolutionize your understanding of money by following my blog and subscribing to my YouTube channel.
🌐 Blog: Unplugged Financial Blog Stay updated with insightful articles, detailed analyses, and practical advice on navigating the evolving financial landscape. Learn about the history of money, the flaws in our current financial systems, and how Bitcoin can offer a path to a more secure and independent financial future.
📺 YouTube Channel: Unplugged Financial Subscribe to our YouTube channel for engaging video content that breaks down complex financial topics into easy-to-understand segments. From in-depth discussions on monetary policies to the latest trends in cryptocurrency, our videos will equip you with the knowledge you need to make informed financial decisions.
👍 Like, subscribe, and hit the notification bell to stay updated with our latest content. Whether you're a seasoned investor, a curious newcomer, or someone concerned about the future of your financial health, our community is here to support you on your journey to financial independence.
Support the Cause
If you enjoyed what you read and believe in the mission of spreading awareness about Bitcoin, I would greatly appreciate your support. Every little bit helps keep the content going and allows me to continue educating others about the future of finance.
Donate Bitcoin: bc1qpn98s4gtlvy686jne0sr8ccvfaxz646kk2tl8lu38zz4dvyyvflqgddylk
#BrainGrowth#NeuralPlasticity#LifelongLearning#PersonalDevelopment#GrowthMindset#CognitiveUpgrade#Neuroscience#LearningJourney#SelfImprovement#KnowledgeIsPower#CrossDomainLearning#MentalGrowth#UnlockYourPotential#BrainTraining#DynamicMindset#ContinuousLearning#ChallengeYourself#SynapticPlasticity#CuriosityDriven#MindOverMatter#bitcoin#cryptocurrency#financial experts#digitalcurrency#financial education#blockchain#financial empowerment#finance#globaleconomy#unplugged financial
1 note
·
View note
Text
Unraveling the BTech CSE 1st Year Syllabus: A Guide to Success

Embarking on a Bachelor of Technology in Computer Science and Engineering (BTech CSE) is an exhilarating journey filled with challenges and opportunities. The BTech CSE 1st year syllabus forms the bedrock of your academic and professional growth, offering a blend of foundational subjects that pave the way for your expertise in technology.
In this article, we’ll explore the intricacies of the BTech CSE 1st year syllabus, covering its core subjects, the challenges students face, and practical strategies to excel. Whether you’re just starting or planning your study routine, Aktu Hub is here to guide you every step of the way.
Overview of the BTech CSE 1st Year Syllabus
The first year of BTech CSE introduces you to the fundamentals of computer science, programming, and engineering concepts. This is a critical phase for building a strong academic foundation and preparing for advanced topics in subsequent years.
Introduction to the BTech CSE Program
Welcome to the exciting world of BTech CSE! This program offers a unique opportunity to explore computers, algorithms, and the ever-evolving tech landscape. Prepare to sharpen your problem-solving abilities and embrace a future shaped by innovation.
Key Components of the BTech CSE 1st Year Syllabus
The first-year curriculum is designed to balance technical, analytical, and communication skills. Here's a breakdown of the core subjects you'll encounter:
1. Foundational Computer Science Courses
These courses introduce you to the basics of computing and programming. Expect to learn:
Fundamentals of algorithms and data structures.
Basics of programming languages like C or Python.
Overview of computer architecture and operating systems.
2. Mathematics and Analytical Skills
Mathematics is an integral part of the syllabus, encompassing:
Calculus and Linear Algebra for problem-solving.
Probability and Statistics for data analysis.
Discrete Mathematics to understand computational logic.
3. Engineering Concepts
Broader engineering topics ensure a well-rounded understanding:
Engineering Mechanics introduces physical principles.
Basic Electrical and Electronics Engineering covers circuit fundamentals.
4. Communication Skills and Ethics
Courses on professional ethics and communication enhance your ability to articulate ideas and uphold ethical standards in the tech world.
Why Understanding the Syllabus is Crucial
1. Setting Academic Expectations
Familiarizing yourself with the BTech CSE 1st year syllabus allows you to set realistic academic goals, ensuring a smooth transition into the program.
2. Aligning with Career Goals
Understanding the curriculum helps you align your studies with your career aspirations, be it software development, cybersecurity, or artificial intelligence.
Common Challenges Faced by Students
1. Transitioning from School to College
The leap from high school to college-level studies can feel overwhelming. New subjects, increased responsibilities, and the need for self-discipline demand significant adjustments.
2. Managing a Heavy Workload
The 1st year comes with assignments, projects, and exams that test your time management and organizational skills. Effective planning is key to staying on top of your workload.
Tips for Success in BTech CSE 1st Year
1. Master the Basics
Focus on core subjects like programming, algorithms, and mathematics.
Practice coding daily to develop logical and analytical skills.
2. Utilize Resources
Use online platforms and study materials from Aktu Hub to deepen your understanding.
Access past papers, notes, and tutorials to prepare effectively for exams.
3. Manage Your Time
Create a study schedule that balances academics, rest, and extracurricular activities.
Break down large tasks into smaller, manageable goals.
4. Seek Help When Needed
Collaborate with peers and join study groups to enhance learning.
Don’t hesitate to reach out to professors for guidance on challenging topics.
Aktu Hub: Your Guide to Academic Excellence
At Aktu Hub, we provide curated resources tailored to the BTech CSE 1st year syllabus. Whether it’s detailed subject guides, programming tutorials, or tips for managing your studies, our platform is designed to support your academic journey.
Visit Aktu Hub today for comprehensive materials and insights to help you succeed in BTech CSE.
Conclusion
The BTech CSE 1st year syllabus is your gateway to the dynamic field of computer science and engineering. By understanding its components, tackling challenges strategically, and leveraging resources like Aktu Hub, you can navigate this foundational year with confidence.
Embrace the journey, focus on building a strong base, and get ready to explore the exciting opportunities that await in the world of technology.
#Aktu b tech cse syllabus#Aktu b tech It syllabus#BTech CSE 1st year syllabus#b.tech 1st year syllabus pdf aktu#B Tech CSE 1st year Syllabus PDF#Aktu b.tech 1st year syllabus
0 notes
Text
The Philosophy of Calculus
The philosophy of calculus explores the foundational, conceptual, and metaphysical questions surrounding calculus as a branch of mathematics. It delves into the nature of continuity, infinity, limits, and the relationship between mathematics and the real world. Since calculus involves concepts such as infinitesimally small quantities, rates of change, and accumulation, philosophers have long debated its implications for our understanding of space, time, and motion.
Key Concepts:
Continuity and Change
Foundational Questions: Calculus, by its very nature, addresses the study of continuous change. Philosophers explore how calculus formalizes change, especially how it captures instantaneous rates of change (derivatives) and accumulations (integrals). The question of how change can be captured mathematically while still being grounded in reality is a central issue.
Zeno's Paradoxes: The ancient Greek philosopher Zeno posed paradoxes, such as the paradox of Achilles and the tortoise, that question how motion and continuity can exist if space and time can be infinitely divided. Calculus provides a mathematical resolution to these paradoxes by showing how infinite processes (like summing infinite series) can yield finite results.
Limits and Infinity
Nature of the Infinite: Calculus heavily relies on the concept of limits, where values approach some end point, often involving infinity or infinitesimally small quantities. Philosophers question what it means for something to approach a value (limit) without ever actually reaching it. What is the status of infinity in calculus? Is it a real, physical entity or a useful mathematical abstraction?
Infinitesimals: In the development of calculus, the idea of infinitesimals—infinitely small quantities—played a crucial role. Philosophers like George Berkeley famously criticized early calculus for relying on "ghosts of departed quantities" that seemed to have no clear ontological basis. Later, the rigorous formalization of limits by Augustin-Louis Cauchy and Karl Weierstrass helped address these concerns, though the concept of infinitesimals remains a subject of philosophical debate.
Mathematical Realism vs. Formalism
Realism: Mathematical realists believe that the objects and structures of calculus (such as limits, derivatives, and integrals) exist independently of human thought, either as abstract objects or as part of the fabric of reality. This perspective raises questions about the relationship between mathematical concepts and the physical world: Does calculus describe real processes in nature, or is it a mere human invention?
Formalism: Formalists, on the other hand, argue that mathematics, including calculus, is merely a system of symbols and rules that humans have devised to model reality. For formalists, the power of calculus lies in its logical consistency and utility, not in the existence of mathematical entities as part of the physical world.
Applications to Space and Time
Mathematics of Motion: Isaac Newton and Gottfried Wilhelm Leibniz, the co-founders of calculus, developed it to study the motion of bodies through space and time. Philosophers ask how calculus models space and time and what this implies for our understanding of reality. Are space and time continuous or discrete, and how does calculus help answer this question?
Relativity and Quantum Mechanics: In modern physics, calculus plays a vital role in theories like general relativity and quantum mechanics. Philosophers of science consider how calculus provides the tools to describe the curvature of spacetime or the probabilistic nature of particles. This brings forth metaphysical debates on the nature of the universe and whether our mathematical tools truly capture its essence.
The Role of Approximation
Calculus as Approximation: In many cases, calculus provides approximations rather than exact answers, especially when dealing with real-world phenomena. Philosophers ask whether this means that calculus only approximates reality, and if so, how accurate these approximations are. What does this say about the limits of human knowledge and the nature of truth in mathematics?
Constructivism vs. Platonism
Platonism: Many mathematicians, such as Kurt Gödel, held that mathematical objects exist in a Platonic realm, independent of human minds. In the philosophy of calculus, this view would suggest that limits, derivatives, and integrals exist "out there" in some ideal form, waiting to be discovered.
Constructivism: On the other hand, constructivists believe that mathematical objects only exist when they are constructed or proven to exist through logical processes. In this view, the objects of calculus, like infinitesimals, do not exist in an abstract realm but are instead tools we create to solve problems.
Historical Development and Philosophical Insights:
Newton vs. Leibniz:
Newton's Fluxions: Newton saw calculus as a way to describe motion and change in the natural world. He introduced the idea of "fluxions" to represent instantaneous rates of change. His view of calculus was closely tied to physical processes, such as the movement of planets.
Leibniz's Infinitesimals: Leibniz focused on the mathematical notation and formal structure of calculus, introducing the concept of infinitesimals. Leibniz’s approach was more abstract, focusing on symbolic representation rather than direct physical interpretation.
Berkeley's Critique:
Criticism of Infinitesimals: The philosopher George Berkeley famously criticized early calculus in his work The Analyst (1734), calling infinitesimals "ghosts of departed quantities." Berkeley’s critique focused on the lack of rigor in the concept of infinitesimals, pushing mathematicians to develop a more solid foundation for calculus, leading to the formal concept of limits.
Modern Foundations:
Limit Theory: The development of rigorous limit theory by mathematicians like Cauchy and Weierstrass resolved many of the early philosophical objections to calculus. By defining limits rigorously, they provided a solid foundation for understanding how calculus could deal with infinity and infinitesimally small quantities.
Non-standard Analysis: In the 20th century, Abraham Robinson developed non-standard analysis, a formal mathematical system that reintroduced infinitesimals in a logically rigorous way. This has revived philosophical discussions about the nature of infinitesimals and their role in calculus.
The philosophy of calculus examines the underlying concepts of change, infinity, and continuity that are central to calculus. It raises questions about the nature of mathematical objects, the relationship between mathematics and reality, and the limits of human knowledge. While calculus has provided powerful tools for understanding the natural world, it also raises deep philosophical questions about the nature of space, time, and motion.
#philosophy#epistemology#knowledge#learning#education#chatgpt#ontology#metaphysics#Calculus#Philosophy of Mathematics#Infinity#Limits#Continuity#Infinitesimals#Mathematical Realism#Formalism#Space and Time#Mathematical Foundations
1 note
·
View note
Text
Online BCA vs B.Sc in Computer Science: Making the Right Choice

In today’s digital age, the demand for tech-savvy professionals is growing rapidly, and two of the most popular undergraduate programs for tech enthusiasts are the Bachelor of Computer Applications (BCA) and the Bachelor of Science in Computer Science (B.Sc. CS). Both degrees offer a solid foundation in technology but differ in their approach, scope, and outcomes. This guide will help you understand the key differences between the two programs and how to choose the right one for your career goals.
Understanding the Basics: BCA vs. B.Sc in Computer Science
Bachelor of Computer Applications (BCA) A BCA degree is designed to prepare students for careers in software development, IT services, and application management. Online BCA programs provide flexibility, making it ideal for those who must balance work, family, or other commitments with their studies. The curriculum typically focuses on software development, application programming, and web development, often including business-related courses.
Bachelor of Science in Computer Science (B.Sc CS) A B.Sc in Computer Science covers more theoretical aspects of computing, including algorithms, data structures, and computer theory. The course is more research-oriented, with a focus on the mathematical foundations of computing. This program is ideal for those interested in software engineering, data science, or advanced computing roles.
Key Differences Between Online BCA and B.Sc in Computer Science
Aspect
Online BCA
B.Sc in Computer Science
Focus Area
Application Development, Software Programming
Theoretical Computing, Algorithms, Research
Flexibility
High (often designed for online study)
Moderate (usually requires lab-based learning)
Course Structure
Application-oriented, with business courses
Theory-oriented, with a focus on mathematical concepts
Career Pathways
IT, Software Development, Application Support
Data Science, Software Engineering, Research
Prerequisites
Open to students from various backgrounds
Typically requires a strong background in math
Ideal for
Practical learners interested in IT roles
Those aiming for advanced or research positions
Course Content and Structure
Online BCA Curriculum The BCA program is designed with a more practical and application-oriented approach, covering areas such as:
Programming Languages (e.g., Java, Python, C++)
Web Development (HTML, CSS, JavaScript)
Database Management (SQL, NoSQL)
Software Engineering (principles and methodologies)
Business Studies (basic understanding of business to apply tech solutions)
This course is often shorter than a B.Sc. CS typically lasts three years and is usually designed to be accessible to students with varied academic backgrounds.
B.Sc in Computer Science Curriculum In contrast, a B.Sc in Computer Science covers:
Data Structures and Algorithms
Computer Networks and Operating Systems
Advanced Mathematics (Calculus, Linear Algebra, Discrete Mathematics)
Artificial Intelligence and Machine Learning
System Programming and Compiler Design
The course is research-intensive and suitable for those interested in a deep understanding of computing principles, mathematical rigor, and analytical thinking.
Career Opportunities: Where Each Degree Can Lead
Online BCA Graduates With an online BCA, graduates are well-prepared for roles in IT and application development, such as:
Software Developer
Web Developer
Database Administrator
IT Support Specialist
Since BCA graduates are trained in industry-relevant programming languages and software applications, they can readily contribute to tech firms, startups, and IT service providers.
B.Sc in Computer Science Graduates Graduates of a B.Sc in Computer Science are often sought for roles requiring strong analytical and technical skills, such as:
Data Scientist
Software Engineer
System Analyst
Research Scientist
With a foundation in computing theory and data structures, B.Sc. CS graduates are well-equipped for positions in AI, machine learning, cybersecurity, and software development.
Choosing the Right Path for You
When choosing between an online BCA and a B.Sc in Computer Science, consider your career goals, preferred learning style, and interest in theory vs. application.
Your Career Goals
If your goal is to work in IT services, application development, or software support, the BCA may be the better fit.
If you’re aiming for roles in data science, research, or advanced technical fields, a B.Sc in Computer Science could be more suitable.
Learning Style
BCA is typically more hands-on and suited for learners who prefer practical application.
B.Sc CS involves rigorous theoretical work and is ideal for those who enjoy problem-solving and analytical thinking.
Schedule and Flexibility
If you need flexibility, an online BCA program can allow you to balance other responsibilities.
B.Sc CS programs, though increasingly available online, might still require in-person lab work for a full experience.
Final Thoughts
Both degrees offer excellent career prospects in the ever-evolving field of technology. An online BCA is a flexible, practical choice for students eager to enter the workforce quickly in IT-related roles, while a B.Sc in Computer Science provides a more research-intensive foundation suitable for careers in advanced computing and data science.
Ultimately, your decision should reflect your career goals, interests, and learning preferences. Whether you choose an online BCA or a B.Sc in Computer Science, both paths offer substantial opportunities in the digital era.
0 notes
Text
All You Need to Know About BCA Syllabus and Curriculum at GGSIPU
Guru Gobind Singh Indraprastha University (GGSIPU), one of Delhi's premier educational institutions, is known for its academic rigor and industry-oriented programs. If you're planning to join GGSIPU or are already a student, understanding the syllabus and curriculum is key to your success. This blog covers everything you need to know about the syllabus structure, key subjects, and how the curriculum is designed to prepare you for your career.
Overview of GGSIPU's Academic Structure
GGSIPU offers undergraduate, postgraduate, and doctoral programs in diverse fields such as engineering, management, law, computer applications, medical sciences, and more. The university is committed to providing a comprehensive education that combines theoretical knowledge with practical experience.
Each program follows a credit-based semester system, with each academic year divided into two semesters. The curriculum is designed to ensure students gain in-depth knowledge in their respective fields, along with essential soft skills and industry exposure.
BCA Syllabus at GGSIPU
For students pursuing a Bachelor of Computer Applications (BCA), GGSIPU’s syllabus is well-structured to cover both foundational concepts and advanced technical skills. Here’s an outline of the key components of the BCA curriculum:
1. Core Subjects
Programming Languages: The curriculum emphasizes languages such as C, C++, Java, Python, and web development technologies.
Data Structures & Algorithms: These subjects form the backbone of programming and software development.
Database Management Systems: Students learn to manage, retrieve, and manipulate data using popular database tools like MySQL and Oracle.
Operating Systems: Understanding OS concepts, such as process management, memory management, and file systems, is crucial.
Computer Networks: The basics of networking, protocols, and data communication are explored.
2. Mathematics and Statistics
Discrete Mathematics, Probability, and Statistics are included to strengthen analytical and problem-solving skills, crucial for IT professionals.
3. Software Engineering
This subject focuses on software development life cycles, project management, and software testing, helping students understand the intricacies of large-scale software projects.
4. Web Development and Application Development
Web technologies (HTML, CSS, JavaScript) and mobile app development are covered to prepare students for real-world application development.
5. Advanced Topics
Artificial Intelligence (AI), Machine Learning (ML), Cloud Computing, and Cyber Security are introduced in later semesters to ensure students are updated with cutting-edge technologies.
6. Practical Labs
GGSIPU places a strong emphasis on practical exposure. Most technical subjects are accompanied by lab sessions where students get hands-on experience with coding, software tools, and real-life problem-solving.
Electives and Specializations
The university offers elective courses in the final year, allowing students to choose subjects based on their interests or career goals. Electives may range from Advanced Database Systems, Data Mining, Internet of Things (IoT), or even non-core subjects like entrepreneurship and business communication.
Internships and Project Work
GGSIPU’s curriculum also includes mandatory internships and project work, typically in the final year of the program. Internships provide students with valuable industry exposure, allowing them to apply theoretical knowledge in real-world situations. The final year project often serves as a culmination of their learning, where students build a working application or conduct research under the guidance of faculty.
Continuous Assessment and Examinations
The evaluation process at GGSIPU is designed to keep students engaged throughout the semester. It includes:
Internal Assessments: Class tests, quizzes, assignments, and lab performance make up a significant portion of the internal marks.
End-Semester Exams: The major component of grading, these exams test the theoretical and practical knowledge acquired over the semester.
Practical Exams: Subjects with lab components include separate practical exams to test hands-on skills.
Syllabus Flexibility and Updates
One of the strengths of GGSIPU’s curriculum is its flexibility. The university regularly revises its syllabus to stay updated with the latest industry trends. Students are also encouraged to participate in workshops, seminars, and certification programs to further their knowledge.
How to Access the GGSIPU Syllabus
Students can easily access the detailed syllabus for each semester and subject from the official GGSIPU website. The syllabus outlines the topics covered, reference books, and evaluation schemes for each subject.
Steps to Access the Syllabus:
Visit the official GGSIPU website.
Navigate to the "Academics" section.
Click on "Syllabus" and select your course and semester.
This ensures students have a clear understanding of what to expect each semester and can prepare accordingly.
Why Understanding the Syllabus is Important
Better Preparation: Knowing what subjects and topics will be covered helps in planning your studies and revision.
Effective Time Management: You can allocate time for each subject based on its complexity and your personal strengths.
Career Alignment: By understanding the curriculum, you can identify subjects that align with your career aspirations and focus on gaining deeper knowledge in those areas.
Conclusion
GGSIPU’s syllabus and curriculum are designed to ensure students are industry-ready with a strong foundation in their chosen fields. Whether you're a computer science student diving into the world of coding, or a management student learning business strategy, the curriculum is tailored to meet academic and professional demands. Staying updated with the syllabus and effectively planning your studies will not only help you excel in exams but also prepare you for a successful career post-graduation.
0 notes
Text
Bachelor Of Computer Science University In Ranchi— Course, Placements & More!
Computer Science is a field that is evolving and growing rapidly in this era. Therefore making a career in computer science is a viable option, as it brings tons of career opportunities. A Bachelor of Computer Science (B.Sc. CS) degree provides a strong foundation in the principles and applications of computer science, equipping students with the skills and knowledge to grow in this digital world.
But the question arises: from where should one pursue this course? And which college is the best bachelor of computer science university in Ranchi? Let’s find out.
Skills To Pursue A Computer Science Degree
To shine in the field of computer science, it is crucial to have a bunch of technical and soft skills. While some of them you learn while pursuing this course, a few basic skills are still required, and those are:
Problem Solving: The ability that is required to analyze and solve complex problems is a must-have skill to pursue a computer science degree.
Logical Thinking: Computer Science involves logical reasoning, algorithmic thinking, and a lot more, to design efficient programs. Thus, possessing this skill is important.
Mathematical Skills: A strong foundation in mathematics, particularly in algebra, calculus, and discrete mathematics, is helpful.
Communication Skills: Having effective communication, both verbal and written is necessary for collaborating with other teams and presenting ideas.
Creativity: The ability to think creatively and come up with innovative solutions is often required in Computer Science.
Therefore, these are the major basic skills that are necessary, if one wants to have a career in computer science. Now, let’s have a closer look at the ways to identify a proper college to achieve your goal in computer science.
Identifying The Right College
As we have understood the importance of the skills that are required to pursue this degree, let’s now look at how one can identify the right college. If you are someone from Ranchi, then pursuing a computer science degree from Amity University can be a wise decision. Amity is Jharkhand’s top university, and it has constantly ranked as the best private university.
Because of its top-notch curriculum, renowned faculty, world-class facilities, vibrant campus life, and stellar placement records— Amity emerges as the premier Computer Science/ IT college in Jharkhand. The graduates often get the high pay packages in some of the top tech companies and MNCs.
TCS, Wipro, IBM, and many other companies visit Amity for the placement drive. And speaking of the placements — in the session of 2022-23, more than 15,000+ students have been placed from Amity. These advantages make Amity the best in the town.
Conclusion
Pursuing a degree in computer science from Amity University can open doors to many exciting career opportunities. It is one of those degrees that can set you apart from the crowd and equip you for an exciting odyssey. So, if you are considering studying computer science at a top university in Ranchi — Apply at Amity. Admissions Open!
Source: https://sites.google.com/view/top-university-in-ranchi--/home?authuser=1
#Bachelor of Computer Science University in Ranchi#Computer Science/ IT college in Jharkhand#top university in ranchi
0 notes
Text
Top 12 Career Opportunities After Completing an MSc in Mathematics: An In-Depth Guide

Mathematics is often seen as the foundation of many fields, from engineering to finance. Completing an MSc in Mathematics opens doors to various career opportunities in both academia and industry. In this guide, we’ll explore 12 top career paths you can pursue after earning your MSc in Mathematics, each offering exciting challenges and rewarding prospects.https://internshipgate.com
1. Data Scientist
With businesses relying more on data-driven decision-making, data scientists are in high demand. As a mathematics graduate, you’re equipped with strong analytical and problem-solving skills, making you a perfect fit for roles in data science. Data scientists analyze large datasets, create algorithms, and develop predictive models to help organizations make informed decisions.
Key Skills: Statistics, programming, data analysis, machine learning.
Industries: Tech companies, finance, healthcare, retail.
2. Quantitative Analyst (Quant)
Quantitative analysts use mathematical models to assist in making financial and investment decisions. Working in financial firms, hedge funds, and investment banks, quants develop algorithms that predict market trends, manage risks, and enhance portfolio management.
Key Skills: Financial mathematics, probability, statistical modeling, programming.
Industries: Finance, banking, investment management.
3. Actuary
Actuaries assess financial risks using mathematics, statistics, and financial theory. They are essential in industries like insurance, where they calculate premiums and determine risk levels for policies. Actuarial roles require deep knowledge of probability and statistics, making an MSc in Mathematics an excellent qualification for this career.
Key Skills: Risk analysis, financial mathematics, statistical modeling.
Industries: Insurance, pensions, investment, healthcare.
4. Mathematics Educator/Professor
If you’re passionate about teaching, becoming a mathematics educator or professor is a fulfilling career path. With an MSc, you can teach at the university or college level, sharing your knowledge with the next generation of mathematicians. Alternatively, you can teach at high schools or community colleges.
Key Skills: Teaching, communication, problem-solving.
Industries: Education, academic institutions.
5. Operations Research Analyst
Operations research analysts use mathematical methods to help organizations optimize their operations. Whether it's improving supply chain efficiency, optimizing production schedules, or designing efficient transportation routes, these professionals apply mathematical models to solve real-world business challenges.
Key Skills: Optimization techniques, linear programming, simulation, data analysis.
Industries: Manufacturing, logistics, government, healthcare.
6. Cryptographer
In today’s digital age, ensuring data security is paramount. Cryptographers develop algorithms to protect sensitive information, working in industries such as cybersecurity and defense. Cryptography requires an advanced understanding of number theory, algebra, and discrete mathematics, making it a great career option for MSc Mathematics graduates.
Key Skills: Encryption, number theory, algorithm design, computer security.
Industries: Cybersecurity, defense, finance, tech companies.
7. Statistician
Statisticians design experiments, collect data, and interpret results to provide insights into various fields such as healthcare, government policy, and business. With your background in advanced statistics from an MSc in Mathematics, you can work in diverse industries that rely on data to make decisions.
Key Skills: Statistical analysis, data collection, hypothesis testing.
Industries: Healthcare, government, market research, business analytics.
8. Financial Analyst
Financial analysts assess the performance of stocks, bonds, and other financial instruments to help clients make investment decisions. Your mathematical skills will enable you to create financial models, forecast economic trends, and assess risks, making you a valuable asset in finance.
Key Skills: Financial modeling, data analysis, economics, risk assessment.
Industries: Finance, banking, investment firms, consulting.
9. Research Scientist
If you enjoy diving deep into complex problems, a career as a research scientist might be for you. Research scientists work in academic, government, or industrial research labs, contributing to advancements in fields such as mathematics, physics, and computer science.
Key Skills: Research methodologies, problem-solving, advanced mathematical theories.
Industries: Academia, government agencies, research institutions, technology companies.
10. Artificial Intelligence and Machine Learning Specialist
Artificial intelligence (AI) and machine learning (ML) are transforming industries worldwide. Mathematics graduates are particularly well-suited for roles in AI and ML, as these fields heavily rely on statistics, linear algebra, and calculus to develop algorithms that drive innovation in tech.
Key Skills: Machine learning algorithms, neural networks, programming, data analysis.
Industries: Technology, healthcare, autonomous systems, finance.
11. Risk Analyst
Risk analysts assess and manage potential risks that businesses might face. This can range from financial risks to operational or strategic risks. With your mathematical skills, you can analyze data, identify patterns, and use statistical models to predict and mitigate risks.
Key Skills: Risk management, probability, data analysis, financial forecasting.
Industries: Finance, insurance, government, consulting.
12. Consultant
As a mathematics consultant, you can work with various companies to solve specific business challenges using mathematical models and analysis. Consultants often work on short-term projects, providing expert advice on everything from improving operational efficiency to making data-driven decisions.
Key Skills: Problem-solving, mathematical modeling, project management.
Industries: Consulting firms, finance, government, healthcare, manufacturing.https://internshipgate.com
Conclusion
An MSc in Mathematics is not only a prestigious qualification but also a versatile one. Whether you’re inclined toward academia or the corporate world, the opportunities are vast. By leveraging your mathematical expertise and combining it with skills such as programming, data analysis, or financial modeling, you can carve out a fulfilling and lucrative career in various fields.
#career#virtualinternship#internship#internshipgate#internship in india#education#mathematics#careeropportunities#job
1 note
·
View note
Text
Discover the Best BCA Colleges in Chandigarh: Your Path to a Successful Tech Career
Chandigarh, known for its well-planned infrastructure and educational institutions, is a hub for students aspiring to build a career in technology. Among the various programs offered, the Bachelor of Computer Applications (BCA) stands out as a popular choice for those passionate about computers and software development. If you're looking to pursue a BCA degree, Chandigarh offers some of the best colleges that provide quality education, industry exposure, and excellent placement opportunities.
Why Choose Chandigarh for Your BCA Studies? Educational Hub: Chandigarh is home to some of the top educational institutions in India, offering a conducive environment for academic growth.
Industry Exposure: The city has a growing IT sector, providing students with ample opportunities for internships, projects, and placements in leading tech companies.
Experienced Faculty: BCA colleges in Chandigarh boast experienced faculty members who are experts in their fields, ensuring that students receive a comprehensive education.
Modern Infrastructure: Colleges in Chandigarh are equipped with state-of-the-art facilities, including computer labs, libraries, and digital classrooms, providing students with the best learning environment.
BCA Course Structure and Curriculum The BCA program in Chandigarh colleges typically spans three years and is divided into six semesters. The curriculum is designed to provide a strong foundation in computer science and its applications. Here’s an overview of the subjects covered:
Programming Languages: C, C++, Java, Python, etc. Database Management: SQL, Oracle, etc. Web Development: HTML, CSS, JavaScript, etc. Software Engineering: Software development life cycle, project management. Data Structures: Concepts of data organization, algorithms. Operating Systems: Windows, Linux, etc. Networking: Basics of networking, network security. Mathematics for Computing: Discrete mathematics, statistics.
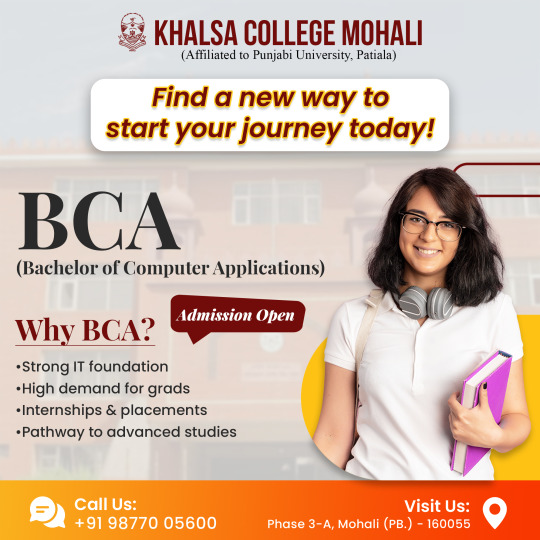
#college#bca college#bca#bca course#india#mbacollege#artificial intelligence#edtech#education#student#school#robotics#uttaranchaluniversity
0 notes
Text
What are the prerequisities for data science?
Hi
If you’re aiming to become a data scientist, there are some key skills and knowledge areas you’ll need to develop. Here’s a simple rundown of the prerequisites:
Mathematics and Statistics:
Mathematics: Understanding linear algebra, calculus, and discrete math helps with data manipulation and algorithm development.
Statistics: Proficiency in probability, hypothesis testing, and statistical modeling is crucial for analyzing data and drawing meaningful conclusions.
Programming Skills:
Python: Python is widely used in data science for its readability and extensive libraries (like Pandas, NumPy, and Scikit-learn).
R: R is another popular language for statistical analysis and data visualization.
SQL: SQL is essential for querying and managing databases, which is a big part of handling data.
Data Manipulation and Analysis:
Data Cleaning: You need to be skilled at cleaning and preparing data for analysis, as real-world data is often messy.
Data Analysis: Knowing how to analyze data using various techniques and tools is fundamental to uncovering insights.
Data Visualization:
Tools: Familiarity with visualization tools like Matplotlib, Seaborn (for Python), or ggplot2 (for R) is important for presenting data findings clearly.
Visualization Principles: Understanding how to effectively communicate data through charts and graphs is key.
Machine Learning Basics:
Algorithms: Knowledge of basic machine learning algorithms (like regression, classification, clustering) and how they work.
Libraries: Experience with machine learning libraries like Scikit-learn or TensorFlow can be helpful.
Domain Knowledge:
Understanding the specific industry or field you want to work in can help you apply data science techniques more effectively to solve relevant problems.
Problem-Solving Skills:
Analytical Thinking: Ability to approach problems methodically and think critically about data and its implications.
Creativity: Finding innovative ways to analyze and interpret data can set you apart.
Communication Skills:
Reporting: Being able to explain complex data insights to non-technical stakeholders is crucial.
Storytelling: Crafting a narrative around your data findings helps make your analysis more impactful.
In summary, becoming a data scientist involves a mix of technical skills, mathematical knowledge, and the ability to communicate insights effectively. Building a solid foundation in these areas will prepare you for a successful career in data science.
1 note
·
View note
Text
How To Make Career In Quantum Computing Jobs Purpose Skills

Possibilities for a career in quantum computing
Quantum Computing Jobs
As interest in Quantum Computing Jobs, there are plenty of chances for candidates to study and sharpen their talents in this subject. By creating better and better candles, you cannot create a better lightbulb. One noteworthy observation from Shalini Ghose’s “A Beginner’s Guide To Quantum Computing” TED Talk was this.
“Building better and better candles will not result in a better light bulb.” One noteworthy observation from Shalini Ghose’s “A Beginner’s Guide To Quantum Computing” TED Talk was this.
Remote. thrilling. confusing. Shyness. For me, it’s too early. These are usually some of the first things that come up when someone starts talking about quantum computing. Let’s be sincere. Because the industry is diverse, working settings vary, and most crucially, rules are different, everyone in this arena is a newbie. even strange.
The move is away from the binary method to computing that is now in use and towards utilizing everything that is in the range of 0 and 1. These options (probably) contain the solutions to the problems of the world, the mind, the universe, and the cosmos.
Gaining Momentum
The last several years have seen a notable increase in the discourse and deliberations surrounding quantum computing. This comes after special interests from the GCCs were successfully persuaded to establish their R&D departments due to the country’s skill pool and strategic location.
According to a NASSCOM estimate, more than $1 billion will be invested on Quantum Computing Jobs advancements over the next five years.
Quantum Computing Jobs
Kinds of jobs in Quantum Computing
Academic institutions contributing to the development of curricula and materials, service providers offering programs and certifications that help further specialize in various areas of quantum computing and mechanics, and start-up communities serving as a link between vision and reality.
The most crucial and next question to ask after the subject is established these are the Quantum Computing Jobs are available.
Conditions
Software development encompasses various fields such as transpilers, open-source development ecosystems, quantum programming languages, and more.
Hardware development includes nuclear magnetic resonance, superconducting architectures, and other things.
Allied technologies: These handle the cathode ray oscilloscopes, optical benches, and cryogenic parts that make the device work.
Future Of Quantum Computing
Fundamentals of Quantum Computing are still the same, even though its laws are new. Compared to digital computing, one requires a strong educational foundation and a focus on the below:
Physics: Understanding the workings of particles and waves is essential for understanding quantum computing ideas.
Engineering: To develop the hardware and peripheral requirements, specifically Electrical, Materials Science, and Computer Engineering.
Math: Proficiency in topics including probability theory, discrete mathematics, algebra, and calculus.
While Ph.D. holders in any of these disciplines are strongly encouraged, internship and employment prospects can also be attained with the help of specializations or certificates from IBM or Microsoft courses as well as practical experience.
How to Make Career in Quantum Computing
Experts in classical computing are at a disadvantage in this situation because quantum computing requires transferable abilities such as:
The creation and development of algorithms, where quantum algorithms can be created by using knowledge from traditional computers.
Proficiency in software development, including coding, debugging, testing, and deployment, is essential.
Tackling a wide range of previously unheard-of difficulties in quantum space problems, whether they involve software or hardware architecture.
To promote a collaborative work environment, professionals with effective project management, communication, and interpersonal abilities and attributes will be highly valued.
Quantum Computing Skills
The time is right to look at employment options and develop the skills you’ll need. To get started, identify your skills, address any weaknesses in your education or experience, look for mentors, secure internships for real-world experience, and prepare yourself as a holistic professional to accept change.
Skills Required For Quantum Computing
Due to its rapid advancements and potential effect, quantum computing provides several fascinating job options. You can establish a quantum computing profession in these areas:
Software Quantum Development
Role: Creating quantum computer algorithms and software.
Ability to use Qiskit, Cirq, Shor’s, and Grover’s algorithms, and classical programming.
Quantum Hardware Engineering
Quantum computer and component design and construction.
Qualifications: Quantum mechanics, solid-state physics, electrical engineering, and experimental setup and manufacturing experience.
Quantum Algorithm Research
Developing and optimising quantum algorithms through research.
Knowledge of quantum information theory, mathematics, and research are needed.
Quantum Cryptography
Responsible for developing quantum-secure communication technologies.
Cryptography, quantum key distribution, and network security knowledge required.
Scientist in Quantum Computing
Performing fundamental quantum computing research and publishing findings in scholarly publications.
Skills needed: Ph.D. in related discipline, excellent analytical skills, and independent research.
Quantum ML
Role: Quantum computing for machine learning and AI.
Quantum computing, machine learning, and related software skills required.
Quantum Consultant
Helping organizations integrate quantum computing into their commercial plans.
Strong communication, business acumen, and quantum computing application knowledge are needed.
Academic Careers
Must have advanced degree, teaching experience, and outstanding publishing record.
Industrial Quantum Computing
Work: R&D in IT, banking, pharmaceutical, and other quantum computing-using industries.
Quantum computing skills in real-world situations and industry knowledge are needed.
Quantum Computing Skills Startups
Skills needed for quantum computing
Job: Joining or establishing quantum computing firms.
Skills needed: Entrepreneurship, fast-paced work, and transdisciplinary skills.
Complex numbers, linear algebra, and probability.
Physical science: Quantum and solid-state physics.
Programming languages, algorithms, and data structures.
Research: Studying and contributing to scholarly writing.
Read more on govindhtech.com
#QuantumComputing#JobsPurposeSkills#QuantumComputingJobs#ibm#Softwaredevelopment#hardware#Microsoft#QuantumComputingskills#machinelearning#ai#computing#SkillsStartups#QuantumML#technology#technews#news#govindhtech
0 notes