#data analytics & ai
Explore tagged Tumblr posts
Text


132K notes
·
View notes
Text
AI exists and there's nothing any of us can do to change that.
If you have concerns about how AI is being/will be used the solution is not to abstain - it's to get involved.
Learn about it, practice utilising AI tools, understand it. Ignorance will not protect you, and putting your fingers in your ears going 'lalalala AI doesn't exist I don't acknowledge it' won't stop it from affecting your life.
The more the general population fears and misunderstands this technology, the less equipped they will be to resist its influence.
#ai#artificial intelligence#ai technology#tech#technology#singularity#futurism#datascience#data analytics#data harvesting#manipulation#civil rights#civil disobedience#ai discourse
161 notes
·
View notes
Text
instagram
ou can become a data analyst ⤵️📈📊💯 Here’s what you need to do: - believe in yourself - learn Excel -learn SQL - learn Tableau - build Portfolio - update Linkedin - optimize Resume - Use Network -apply for jobs That’s the way. . . .
#data#dataanalyst#dataanalytics#analyst#analytics#workfromhome#codinglife#tech#teknoloji#programming#datascience#programmer#python#machinelearning#artificialintelligence#deeplearning#code#ai#Instagram
5 notes
·
View notes
Text
what u think, to much colour, or less?
https://sdesignt.threadless.com/
#tshirt#animals#design#rainbow#computer#Innovation#AI#Blockchain#Crypto#Tech#Digital#Data#BigData#Automation#Cloud#Cybersecurity#Startup#Entrepreneur#Leadership#Marketing#Business#Ecommerce#Content#Performance#Development#Research#Analytics#Growth#Productivity#Trend
4 notes
·
View notes
Text
Every time I hear about someone using ChatGPT or another LLM to generate code for their job, I am baffled because I hate inheriting code from another person. Even someone who writes clean, performant code will have their own style or choice of solution that is different than mine, so I have to make a mental adjustment when I'm working on something I inherited from someone else, and half the time, I end up reworking a large chunk of what I've been handed.
So, I'm imagining that situation, but also I can't even ask the entity generating the code why they did something, and I just simply don't see the appeal.
I've heard people say they mainly use it for simple-but-tedious code writing, and maybe I'm just built different, but if I find myself writing something over and over again, I...save a template. (I'm being sarcastic. I am not brilliant or unusual for doing this. I just don't know how using ChatGPT for simple things is any less tedious than using a template.) Or, depending on what program I'm using, there are built-in tools and functions like macros or stored procedures that cut down on duplicating effort.
I also find the mere idea of having to explain to a program what I want to be more time-consuming and annoying than just writing it myself, so it might just be that it's not for me. But I also don't fully trust the way the code is being generated. So...
4 notes
·
View notes
Text
Non-fiction books that explore AI's impact on society - AI News
New Post has been published on https://thedigitalinsider.com/non-fiction-books-that-explore-ais-impact-on-society-ai-news/
Non-fiction books that explore AI's impact on society - AI News
.pp-multiple-authors-boxes-wrapper display:none; img width:100%;
Artificial Intelligence (AI) is code or technologies that perform complex calculations, an area that encompasses simulations, data processing and analytics.
AI has increasingly grown in importance, becoming a game changer in many industries, including healthcare, education and finance. The use of AI has been proven to double levels of effectiveness, efficiency and accuracy in many processes, and reduced cost in different market sectors.
AI’s impact is being felt across the globe, so, it is important we understand the effects of AI on society and our daily lives.
Better understanding of AI and all that it does and can mean can be gained from well-researched AI books.
Books on AI provide insights into the use and applications of AI. They describe the advancement of AI since its inception and how it has shaped society so far. In this article, we will be examining recommended best books on AI that focus on the societal implications.
For those who don’t have time to read entire books, book summary apps like Headway will be of help.
Book 1: “Superintelligence: Paths, Dangers, Strategies” by Nick Bostrom
Nick Bostrom is a Swedish philosopher with a background in computational neuroscience, logic and AI safety.
In his book, Superintelligence, he talks about how AI can surpass our current definitions of intelligence and the possibilities that might ensue.
Bostrom also talks about the possible risks to humanity if superintelligence is not managed properly, stating AI can easily become a threat to the entire human race if we exercise no control over the technology.
Bostrom offers strategies that might curb existential risks, talks about how Al can be aligned with human values to reduce those risks and suggests teaching AI human values.
Superintelligence is recommended for anyone who is interested in knowing and understanding the implications of AI on humanity’s future.
Book 2: “AI Superpowers: China, Silicon Valley, and the New World Order” by Kai-Fu Lee
AI expert Kai-Fu Lee’s book, AI Superpowers: China, Silicon Valley, and the New World Order, examines the AI revolution and its impact so far, focusing on China and the USA.
He concentrates on the competition between these two countries in AI and the various contributions to the advancement of the technology made by each. He highlights China’s advantage, thanks in part to its larger population.
China’s significant investment so far in AI is discussed, and its chances of becoming a global leader in AI. Lee believes that cooperation between the countries will help shape the future of global power dynamics and therefore the economic development of the world.
In thes book, Lee states AI has the ability to transform economies by creating new job opportunities with massive impact on all sectors.
If you are interested in knowing the geo-political and economic impacts of AI, this is one of the best books out there.
Book 3: “Life 3.0: Being Human in the Age of Artificial Intelligence” by Max Tegmark
Max Tegmark’s Life 3.0 explores the concept of humans living in a world that is heavily influenced by AI. In the book, he talks about the concept of Life 3.0, a future where human existence and society will be shaped by AI. It focuses on many aspects of humanity including identity and creativity.
Tegmark envisions a time where AI has the ability to reshape human existence. He also emphasises the need to follow ethical principles to ensure the safety and preservation of human life.
Life 3.0 is a thought-provoking book that challenges readers to think deeply about the choices humanity may face as we progress into the AI era.
It’s one of the best books to read if you are interested in the ethical and philosophical discussions surrounding AI.
Book 4: “The Fourth Industrial Revolution” by Klaus Schwab
Klaus Martin Schwab is a German economist, mechanical engineer and founder of the World Economic Forum (WEF). He argues that machines are becoming smarter with every advance in technology and supports his arguments with evidence from previous revolutions in thinking and industry.
He explains that the current age – the fourth industrial revolution – is building on the third: with far-reaching consequences.
He states use of AI in technological advancement is crucial and that cybernetics can be used by AIs to change and shape the technological advances coming down the line towards us all.
This book is perfect if you are interested in AI-driven advancements in the fields of digital and technological growth. With this book, the role AI will play in the next phases of technological advancement will be better understood.
Book 5: “Weapons of Math Destruction: How Big Data Increases Inequality and Threatens Democracy” by Cathy O’Neil
Cathy O’Neil’s book emphasises the harm that defective mathematical algorithms cause in judging human behaviour and character. The continual use of maths algorithms promotes harmful results and creates inequality.
An example given in the book is of research that proved bias in voting choices caused by results from different search engines.
Similar examination is given to research that focused Facebook, where, by making newsfeeds appear on users’ timelines, political preferences could be affected.
This book is best suited for readers who want to adventure in the darker sides of AI that wouldn’t regularly be seen in mainstream news outlets.
Book 6: “The Age of Em: Work, Love, and Life when Robots Rule the Earth” by Robin Hanson
An associate professor of economics at George Mason University and a former researcher at the Future of Humanity Institute of Oxford University, Robin Hanson paints an imaginative picture of emulated human brains designed for robots. What if humans copied or “emulated” their brains and emotions and gave them to robots?
He argues that humans who become “Ems” (emulations) will become more dominant in the future workplace because of their higher productivity.
An intriguing book for fans of technology and those who love intelligent predictions of possible futures.
Book 7: “Architects of Intelligence: The truth about AI from the people building it” by Martin Ford
This book was drawn from interviews with AI experts and examines the struggles and possibilities of AI-driven industry.
If you want insights from people actively shaping the world, this book is right for you!
CONCLUSION
These books all have their unique perspectives but all point to one thing – the advantages of AI of today will have significant societal and technological impact. These books will give the reader glimpses into possible futures, with the effects of AI becoming more apparent over time.
For better insight into all aspects of AI, these books are the boosts you need to expand your knowledge. AI is advancing quickly, and these authors are some of the most respected in the field. Learn from the best with these choice reads.
#2024#ai#ai news#ai safety#Algorithms#Analytics#applications#apps#Article#artificial#Artificial Intelligence#author#background#Bias#Big Data#book#Books#brains#Building#change#China#code#competition#creativity#data#data processing#Democracy#development#double#dynamics
2 notes
·
View notes
Text
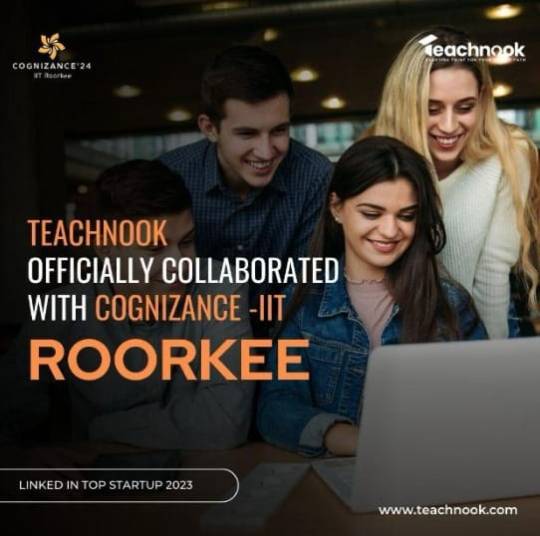
Cognizance IIT Roorkee Internship and Training Program
Registration Link : https://forms.gle/E2cHdnjyzYytKxC39
#engineering#internship#jobs#iit#work from home#student#ai#datascience#data analytics#machinelearning#webde#web development#ui ux development services#graphic design#finance#marketing
3 notes
·
View notes
Text
How do I learn R, Python and data science?
Learning R, Python, and Data Science: A Comprehensive Guide
Choosing the Right Language
R vs. Python: Both R and Python are very powerful tools for doing data science. R is usually preferred for doing statistical analysis and data visualisations, whereas Python is much more general and currently is very popular for machine learning and general-purpose programming. Your choice of which language to learn should consider your specific goals and preferences.
Building a Strong Foundation
Structured Courses Online Courses and Tutorials: Coursera, edX, and Lejhro offer courses and tutorials in R and Python for data science. Look out for courses that develop theoretical knowledge with practical exercises. Practise your skills with hands-on coding challenges using accompanying datasets, offered on websites like Kaggle and DataCamp.
Books: There are enough books to learn R and Python for data science. You may go through the classical ones: "R for Data Science" by Hadley Wickham, and "Python for Data Analysis" by Wes McKinney.
Learning Data Science Concepts
Statistics: Know basic statistical concepts: probability, distribution, hypothesis testing, and regression analysis.
Cleaning and Preprocessing: Learn how to handle missing data techniques, outliers, and data normalisation.
Data Visualization: Expert libraries to provide informative visualisations, including but not limited to Matplotlib and Seaborn in Python and ggplot2 in R.
Machine Learning: Learn algorithms-Linear Regression, Logistic Regression, Decision Trees, Random Forest, Neural Networks, etc.
Deep Learning: Study deep neural network architecture and how to build and train them using the frameworks TensorFlow and PyTorch.
Practical Experience
Personal Projects: In this, you apply your knowledge to personal projects which help in building a portfolio.
Kaggle Competitions: Participate in Kaggle competitions to solve real-world problems in data science and learn from others.
Contributions to Open-Source Projects: Contribute to some open-source projects for data science in order to gain experience and work with other people.
Other Advice
Join Online Communities: Join online forums or communities such as Stack Overflow and Reddit to ask questions, get help, and connect with other data scientists.
Attend Conferences and Meetups: This is a fantastic way to network with similar working professionals in the field and know the latest trends going on in the industry.
Practice Regularly: For becoming proficient in data science, consistent practice is an indispensable element. Devote some time each day for practising coding challenges or personal projects.
This can be achieved by following the above-mentioned steps and having a little bit of dedication towards learning R, Python, and Data Science.
2 notes
·
View notes
Text
Can statistics and data science methods make predicting a football game easier?
Hi,
Statistics and data science methods can significantly enhance the ability to predict the outcomes of football games, though they cannot guarantee results due to the inherent unpredictability of sports. Here’s how these methods contribute to improving predictions:
Data Collection and Analysis:
Collecting and analyzing historical data on football games provides a basis for understanding patterns and trends. This data can include player statistics, team performance metrics, match outcomes, and more. Analyzing this data helps identify factors that influence game results and informs predictive models.
Feature Engineering:
Feature engineering involves creating and selecting relevant features (variables) that contribute to the prediction of game outcomes. For football, features might include team statistics (e.g., goals scored, possession percentage), player metrics (e.g., player fitness, goals scored), and contextual factors (e.g., home/away games, weather conditions). Effective feature engineering enhances the model’s ability to capture important aspects of the game.
Predictive Modeling:
Various predictive models can be used to forecast football game outcomes. Common models include:
Logistic Regression: This model estimates the probability of a binary outcome (e.g., win or lose) based on input features.
Random Forest: An ensemble method that builds multiple decision trees and aggregates their predictions. It can handle complex interactions between features and improve accuracy.
Support Vector Machines (SVM): A classification model that finds the optimal hyperplane to separate different classes (e.g., win or lose).
Poisson Regression: Specifically used for predicting the number of goals scored by teams, based on historical goal data.
Machine Learning Algorithms:
Advanced machine learning algorithms, such as gradient boosting and neural networks, can be employed to enhance predictive accuracy. These algorithms can learn from complex patterns in the data and improve predictions over time.
Simulation and Monte Carlo Methods:
Simulation techniques and Monte Carlo methods can be used to model the randomness and uncertainty inherent in football games. By simulating many possible outcomes based on historical data and statistical models, predictions can be made with an understanding of the variability in results.
Model Evaluation and Validation:
Evaluating the performance of predictive models is crucial. Metrics such as accuracy, precision, recall, and F1 score can assess the model’s effectiveness. Cross-validation techniques ensure that the model generalizes well to new, unseen data and avoids overfitting.
Consideration of Uncertainty:
Football games are influenced by numerous unpredictable factors, such as injuries, referee decisions, and player form. While statistical models can account for many variables, they cannot fully capture the uncertainty and randomness of the game.
Continuous Improvement:
Predictive models can be continuously improved by incorporating new data, refining features, and adjusting algorithms. Regular updates and iterative improvements help maintain model relevance and accuracy.
In summary, statistics and data science methods can enhance the ability to predict football game outcomes by leveraging historical data, creating relevant features, applying predictive modeling techniques, and continuously refining models. While these methods improve the accuracy of predictions, they cannot eliminate the inherent unpredictability of sports. Combining statistical insights with domain knowledge and expert analysis provides the best approach for making informed predictions.
3 notes
·
View notes
Text
2 notes
·
View notes
Text
Data Fragmentation
This is the list of challenges that the businesses face due to data distribution across multiple systems in the digital lending industry
3 notes
·
View notes
Text
Cloud modernization is out, generative AI is in
#now im an ai girlie oh lord save me#this is for data analytics not art so please dont let my joke break containment#im in data engineering if you didn't know#personal
3 notes
·
View notes
Text
Researchers create AI tool to forecast cancer patients' responses to immunotherapy
- By InnoNurse Staff -
NIH scientists have developed an AI tool that uses routine clinical data to predict cancer patients' responses to immunotherapy, potentially aiding in treatment decisions.
Read more at National Institutes of Health (NIH)
///
Other recent news and insights
New analytical tool enhances comprehension of heritable human traits and diseases (University of Oslo/Medical Xpress)
#health informatics#ai#cancer#oncology#immunotherapy#data science#health tech#medtech#analytics#genetics#health it
2 notes
·
View notes
Text
Top 5 Benefits of Low-Code/No-Code BI Solutions
Low-code/no-code Business Intelligence (BI) solutions offer a paradigm shift in analytics, providing organizations with five key benefits. Firstly, rapid development and deployment empower businesses to swiftly adapt to changing needs. Secondly, these solutions enhance collaboration by enabling non-technical users to contribute to BI processes. Thirdly, cost-effectiveness arises from reduced reliance on IT resources and streamlined development cycles. Fourthly, accessibility improves as these platforms democratize data insights, making BI available to a broader audience. Lastly, agility is heightened, allowing organizations to respond promptly to market dynamics. Low-code/no-code BI solutions thus deliver efficiency, collaboration, cost savings, accessibility, and agility in the analytics landscape.
#newfangled#polusai#etl#nlp#data democratization#business data#big data#ai to generate dashboard#business dashboard#bi report#generativeai#business intelligence tool#artificialintelligence#machine learning#no code#data analytics#data visualization#zero coding
3 notes
·
View notes