#code detector
Explore tagged Tumblr posts
Text
i sincerely hope we get a flashback of how mark started out at lumon as everything he's ever mentioned suggests that he was the same as helly until they beat him into submission
#severance#the fact that they had to ask him 18 times who he is then he threatned petey he would kill him#he knows about the bad soap#he knows about swallowed messages#he knows the code detectors can pick up lettering anywhere#he said “milchick can't always be nice like that”#etc etc#he definitely went through the horrors#pine rambles
162 notes
·
View notes
Note
are you a bot or just neurodivergent?
Pokemon detected:
are you a bot or just neurodivergent?
Aron !
#ask the detector#pokemon detector#pokemon#the answer is “and” rather than “or”#i'm a person AND i wrote some code to parse text and detect pokemon
23 notes
·
View notes
Text
don’t misunderstand this as a dig against helly, it isn’t, but also if i was mark s and id just lost my emotional support coworker with no warning and his replacement was someone who was really loud and broke rules and started fights all the time id flip shit in the worst way
#N posts stuff#AND HES TRAINING. gd i used to Hate training new employees at my last job it’s the worst even when the person listens to you#mark s. should have been allowed one absolute meltdown on company time#thinking about mark’s two dead wives a lot this morning#also wondering to myself whether the code detectors go off for things that Could be code marks but aren’t#(the user is thinking about bite marks)
4 notes
·
View notes
Note
i wish for you to translate this message Leon sent on the discord :sob: ⌓⍕✡ ⍦⧰⍕⩢ ⏦⚠⩈⌓ ⩢⚠⩥⩥ ⨈☍⏃⨈ ⧖ ☊⏃⧰⧰⍕⨈ ⛦⍕ ⚛⏃⩈ ⚛⩈⍕⚴ ⌓⍕✡ -Overcomplicated Anon
I'm sorry I have never seen that type of code.
4 notes
·
View notes
Text
sometimes I'm not a stereotypical engineering student. other times my flatmate catches me heading to me room at 2am with a bowl of oatmeal and an energy drink.
#one time i fried an esp chip and it started smoking and set off the smoke detector#i then went out at 10am to meet my friend and poach her old one#then i fried that one too#another time my code went into infinite loop and....some other things happened and soon enough the uni computer i was using froze#this is why i use uni computers to try stuff like this instead of my own#another time i got a 42% on my assignment#....i was the second highest in my class#the highest was 44%#...im a CHEMICAL engineering student
2 notes
·
View notes
Text

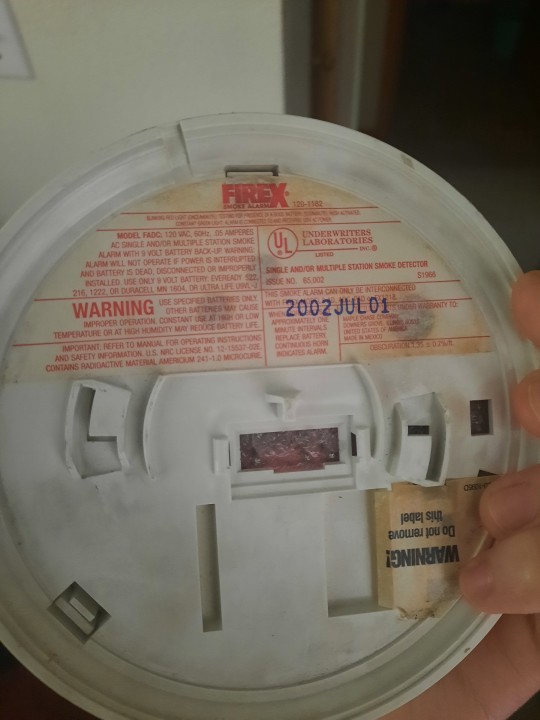
My son Tony who has every disease
#for reference this smoke alarm is 2 months younger than me. i am 21.#and a smoke detector should NEVER be in service longer than 10 years.#so yes everyone this is my geriatric and senile son who sounded like a car horn whenever he went off.#rip tony you served us for too long. we should have never met but alas fate had other plans.#(all of our detectors are this old btw. i'm in the middle of reporting my leasing agency for this. that's a building code violation.)
9 notes
·
View notes
Text
But who is using ML to create a regularly-updated regex + image filter capable of detecting all references to W*ndsors so I never have to see a single post about them
6 notes
·
View notes
Text
Instantly Detect Java Code Plagiarism with Codequiry

Protecting your Java code integrity is more important than ever, and with Codequiry, you can detect Java code plagiarism instantly. This powerful Java Code Plagiarism Checker scans your code for similarities, ensuring it remains original. Whether you’re working on individual projects or collaborating in teams, Codequiry’s advanced detection system supports multiple programming languages and provides a seamless experience in safeguarding your intellectual property. Stay ahead of the game and check Java code for plagiarism with ease!
#code plagiarism checker#plagiarism checker#code plagiarism#source code checker#detect code plagiarism#code plagiarism detector#code similarity checker#codequiry#website plagiarism checker#java code plagiarism checker#check java code plagiarism
0 notes
Text
The ‘Secret Routes’ That Can Foil Pedestrian Recognition Systems
New Post has been published on https://thedigitalinsider.com/the-secret-routes-that-can-foil-pedestrian-recognition-systems/
The ‘Secret Routes’ That Can Foil Pedestrian Recognition Systems
A new research collaboration between Israel and Japan contends that pedestrian detection systems possess inherent weaknesses, allowing well-informed individuals to evade facial recognition systems by navigating carefully planned routes through areas where surveillance networks are least effective.
With the help of publicly available footage from Tokyo, New York and San Francisco, the researchers developed an automated method of calculating such paths, based on the most popular object recognition systems likely to be in use in public networks.
The three crossings used in the study: Shibuya Crossing in Tokyo, Japan; Broadway, New York; and Castro District, San Francisco. Source: https://arxiv.org/pdf/2501.15653
By this method, it’s possible to generate confidence heatmaps that demarcate areas within the camera feed where pedestrians are least likely to provide a positive facial recognition hit:
On the right, we see the confidence heatmap generated by the researchers’ method. The red areas indicate low confidence, and a configuration of stance, camera pose and other factor that are likely to impede facial recognition.
In theory such a method could be instrumentalized into a location-aware app, or some other kind of platform to disseminate the least ‘recognition-friendly’ paths from A to B in any calculated location.
The new paper proposes such a methodology, titled Location-based Privacy Enhancing Technique (L-PET); it also proposes a countermeasure titled Location-Based Adaptive Threshold (L-BAT), which essentially runs exactly the same routines, but then uses the information to reinforce and improve the surveillance measures, instead of devising ways to avoid being recognized; and in many cases, such improvements would not be possible without further investment in the surveillance infrastructure.
The paper therefore sets up a potential technological war of escalation between those seeking to optimize their routes to avoid detection and the ability of surveillance systems to make full use of facial recognition technologies.
Prior methods of foiling detection are less elegant than this, and center on adversarial approaches, such as TnT Attacks, and the use of printed patterns to confuse the detection algorithm.
The 2019 work ‘Fooling automated surveillance cameras: adversarial patches to attack person detection’ demonstrated an adversarial printed pattern capable of convincing a recognition system that no person is detected, allowing a kind of ‘invisibility. Source: https://arxiv.org/pdf/1904.08653
The researchers behind the new paper observe that their approach requires less preparation, with no need to devise adversarial wearable items (see image above).
The paper is titled A Privacy Enhancing Technique to Evade Detection by Street Video Cameras Without Using Adversarial Accessories, and comes from five researchers across Ben-Gurion University of the Negev and Fujitsu Limited.
Method and Tests
In accordance with previous works such as Adversarial Mask, AdvHat, adversarial patches, and various other similar outings, the researchers assume that the pedestrian ‘attacker’ knows which object detection system is being used in the surveillance network. This is actually not an unreasonable assumption, due to the widespread adoption of state-of-the-art open source systems such as YOLO in surveillance systems from the likes of Cisco and Ultralytics (currently the central driving force in YOLO development).
The paper also assumes that the pedestrian has access to a live stream on the internet fixed on the locations to be calculated, which, again, is a reasonable assumption in most of the places likely to have an intensity of coverage.
Sites such as 511ny.org offer access to many surveillance cameras in the NYC area. Source: https://511ny.or
Besides this, the pedestrian needs access to the proposed method, and to the scene itself (i.e., the crossings and routes in which a ‘safe’ route is to be established).
To develop L-PET, the authors evaluated the effect of the pedestrian angle in relation to the camera; the effect of camera height; the effect of distance; and the effect of the time of day. To obtain ground truth, they photographed a person at the angles 0°, 45°, 90°, 135°, 180°, 225°, 270°, and 315°.
Ground truth observations carried out by the researchers.
They repeated these variations at three different camera heights (0.6m, 1.8m, 2.4m), and with varied lighting conditions (morning, afternoon, night and ‘lab’ conditions).
Feeding this footage to the Faster R-CNN and YOLOv3 object detectors, they found that the confidence of the object depends on the acuteness of the angle of the pedestrian, the pedestrian’s distance, the camera height, and the weather/lighting conditions*.
The authors then tested a broader range of object detectors in the same scenario: Faster R-CNN; YOLOv3; SSD; DiffusionDet; and RTMDet.
The authors state:
‘We found that all five object detector architectures are affected by the pedestrian position and ambient light. In addition, we found that for three of the five models (YOLOv3, SSD, and RTMDet) the effect persists through all ambient light levels.’
To extend the scope, the researchers used footage taken from publicly available traffic cameras in three locations: Shibuya Crossing in Tokyo, Broadway in New York, and the Castro District in San Francisco.
Each location furnished between five and six recordings, with approximately four hours of footage per recording. To analyze detection performance, one frame was extracted every two seconds, and processed using a Faster R-CNN object detector. For each pixel in the obtained frames, the method estimated the average confidence of the ‘person’ detection bounding boxes being present in that pixel.
‘We found that in all three locations, the confidence of the object detector varied depending on the location of people in the frame. For instance, in the Shibuya Crossing footage, there are large areas of low confidence farther away from the camera, as well as closer to the camera, where a pole partially obscures passing pedestrians.’
The L-PET method is essentially this procedure, arguably ‘weaponized’ to obtain a path through an urban area that is least likely to result in the pedestrian being successfully recognized.
By contrast, L-BAT follows the same procedure, with the difference that it updates the scores in the detection system, creating a feedback loop designed to obviate the L-PET approach and make the ‘blind areas’ of the system more effective.
(In practical terms, however, improving coverage based on obtained heatmaps would require more than just an upgrade of the camera sitting in the expected position; based on the testing criteria, including location, it would require the installation of additional cameras to cover the neglected areas – therefore it could be argued that the L-PET method escalates this particular ‘cold war’ into a very expensive scenario indeed)
The average pedestrian detection confidence for each pixel, across diverse detector frameworks, in the observed area of Castro Street, analyzed across five videos. Each video was recorded under different lighting conditions: sunrise, daytime, sunset, and two distinct nighttime settings. The results are presented separately for each lighting scenario.
Having converted the pixel-based matrix representation into a graph representation suitable for the task, the researchers adapted the Dijkstra algorithm to calculate optimal paths for pedestrians to navigate through areas with reduced surveillance detection.
Instead of finding the shortest path, the algorithm was modified to minimize detection confidence, treating high-confidence regions as areas with higher ‘cost’. This adaptation allowed the algorithm to identify routes passing through blind spots or low-detection zones, effectively guiding pedestrians along paths with reduced visibility to surveillance systems.
A visualization depicting the transformation of the scene’s heatmap from a pixel-based matrix into a graph-based representation.
The researchers evaluated the impact of the L-BAT system on pedestrian detection with a dataset built from the aforementioned four-hour recordings of public pedestrian traffic. To populate the collection, one frame was processed every two seconds using an SSD object detector.
From each frame, one bounding box was selected containing a detected person as a positive sample, and another random area with no detected people was used as a negative sample. These twin samples formed a dataset for evaluating two Faster R-CNN models – one with L-BAT applied, and one without.
The performance of the models was assessed by checking how accurately they identified positive and negative samples: a bounding box overlapping a positive sample was considered a true positive, while a bounding box overlapping a negative sample was labeled a false positive.
Metrics used to determine the detection reliability of L-BAT were Area Under the Curve (AUC); true positive rate (TPR); false positive rate (FPR); and average true positive confidence. The researchers assert that the use of L-BAT enhanced detection confidence while maintaining a high true positive rate (albeit with a slight increase in false positives).
In closing, the authors note that the approach has some limitations. One is that the heatmaps generated by their method are specific to a particular time of day. Though they do not expound on it, this would indicate that a greater, multi-tiered approach would be needed to account for the time of day in a more flexible deployment.
They also observe that the heatmaps will not transfer to different model architectures, and are tied to a specific object detector model. Since the work proposed is essentially a proof-of-concept, more adroit architectures could, presumably, also be developed to remedy this technical debt.
Conclusion
Any new attack method for which the solution is ‘paying for new surveillance cameras’ has some advantage, since expanding civic camera networks in highly-surveilled areas can be politically challenging, as well as representing a notable civic expense that will usually need a voter mandate.
Perhaps the biggest question posed by the work is ‘Do closed-source surveillance systems leverage open source SOTA frameworks such as YOLO?’. This is, of course, impossible to know, since the makers of the proprietary systems that power so many state and civic camera networks (at least in the US) would argue that disclosing such usage might open them up to attack.
Nonetheless, the migration of government IT and in-house proprietary code to global and open source code would suggest that anyone testing the authors’ contention with (for example) YOLO might well hit the jackpot immediately.
* I would normally include related table results when they are provided in the paper, but in this case the complexity of the paper’s tables makes them unilluminating to the casual reader, and a summary is therefore more useful.
First published Tuesday, January 28, 2025
#2025#adoption#ai surveillance#algorithm#ambient#app#approach#Art#Artificial Intelligence#bat#box#Cameras#Cisco#CNN#code#Collaboration#complexity#course#deployment#detection#detector#development#driving#facial recognition#Facial Recognition Technology#factor#false positives#Fujitsu#Full#Global
0 notes
Note
s y l v e o n ?
Pokemon detected:
s y l v e o n ?
Sylveon !
14 notes
·
View notes
Video
youtube
AI or Human? Detect the Source of Code with AI Code Detector Pro!
0 notes
Text
Code AI Detector: Verify AI-Generated Code with ZeroGPT
ZeroGPT’s Code AI Detector allows you to check if code has been generated by AI models like ChatGPT. Whether you're reviewing programming assignments, software documentation, or professional code, this tool helps you detect any sections created by AI. As AI becomes more involved in coding, it's important to ensure that your code is human-written and authentic. ZeroGPT provides a fast, reliable solution for verifying AI-generated code, giving you peace of mind that your work is original. Use ZeroGPT’s Code AI Detector to ensure the integrity of your code.
0 notes
Text
The Rise of AI: How Zerogpt Helps You Detect and Search GPT-Generated Content
Artificial Intelligence (AI) is transforming the way we interact with technology, creating new opportunities across industries. However, this surge in AI capabilities also brings challenges, especially in identifying content generated by AI. Zerogpt is at the forefront of addressing these challenges, offering powerful tools like the AI detector and the ability to search for GPT-generated content. In this blog, we’ll explore the significance of these tools and how they can be invaluable for various users.
Understanding the Need for AI Detection
As AI-generated content becomes more prevalent, distinguishing between human-written and AI-generated text has become increasingly difficult. From news articles and academic papers to social media posts, AI is capable of producing content that closely mimics human writing. While this has numerous benefits, such as streamlining content creation and enhancing automation, it also raises concerns about the authenticity and reliability of the information.
For educators, researchers, and content creators, the ability to verify the origin of content is crucial. This is where tools like Zerogpt’s Code AI Detector come into play. Whether you’re checking the authenticity of an article or ensuring that your content remains original, Zerogpt’s AI detection capabilities provide a robust solution.
How Zerogpt’s AI Detector Works
Zerogpt’s AI detector is designed to analyze text and determine whether it was generated by a machine or written by a human. The tool leverages advanced algorithms that examine various linguistic patterns, sentence structures, and word choices typical of AI-generated content. By comparing these elements with known AI outputs, the detector can identify content that has been created using models like GPT (Generative Pre-trained Transformer).
One of the key features of Zerogpt’s AI detector is its accuracy. It’s not just about flagging content as potentially AI-generated; it provides insights into the level of AI involvement. This can be particularly useful in academic settings, where the integrity of work is paramount, or in the media industry, where the authenticity of information is critical.
The Importance of AI Detection in Various Fields
Education: In academia, the rise of AI tools has made it easier for students to generate essays, reports, and even research papers. While AI can be a helpful resource, it’s important to ensure that students are producing original work. Zerogpt’s AI detector can help educators verify that assignments are student-generated, promoting academic integrity.
Publishing: For publishers, maintaining the quality and authenticity of content is essential. With the influx of AI-generated articles, there’s a risk of publishing content that may not meet the required standards of originality or factual accuracy. Using Zerogpt’s AI detector, publishers can screen submissions to ensure that content is both original and credible.
Content Creation: Content creators often rely on AI to assist in generating ideas or drafting content. However, there’s a fine line between using AI as a tool and allowing it to dominate the creative process. Zerogpt helps creators strike this balance by identifying when content has crossed into the territory of being primarily AI-generated, allowing them to refine their work accordingly.
Search GPT: Finding AI-Generated Content
Beyond detection, another valuable tool offered by Zerogpt is the ability to search for GPT-generated content. This feature is particularly useful for researchers, marketers, and anyone interested in tracking the influence of AI across the internet.
The Search GPT function allows users to input queries and find content that has likely been generated by GPT models. This can be used to study trends in AI-generated content, monitor the use of AI in specific domains, or simply to explore how AI is shaping online discourse.
For instance, a researcher studying the impact of AI on journalism might use the search GPT tool to identify articles written by AI. This could provide valuable insights into how AI is being used in the media industry and what this means for the future of journalism.
Why Choose Zerogpt?
Zerogpt stands out for its dedication to accuracy, usability, and relevance in the rapidly evolving world of AI. Here’s why it’s the go-to tool for detecting and searching AI-generated content:
Precision: Zerogpt’s tools are built on cutting-edge algorithms that prioritize accuracy. Whether you’re detecting AI-generated content or searching for GPT outputs, you can trust that the results are reliable.
User-Friendly Interface: Despite its advanced capabilities, Zerogpt offers an intuitive interface that’s easy to navigate, making it accessible to both tech-savvy users and those new to AI detection.
Versatility: Zerogpt caters to a wide range of users, from educators and researchers to marketers and content creators. Its tools are versatile, meeting the needs of various industries and applications.
Continuous Updates: AI technology is constantly evolving, and so is Zerogpt. The platform is regularly updated to keep pace with advancements in AI, ensuring that its detection and search capabilities remain at the forefront of the industry.
Conclusion
As AI continues to integrate into our daily lives, the ability to detect and search for AI-generated content becomes increasingly important. Zerogpt provides the tools necessary to navigate this new landscape, offering reliable AI detection and the ability to search for GPT-generated content with ease. Whether you’re in education, publishing, or content creation, Zerogpt empowers you to maintain the integrity and originality of your work in an AI-driven world.
0 notes
Note
("Hmm... Hmm...!!") *shinigami thoughtfully rubs her chin while staring at yuta, her smile growing even wider as she watches his ahoge waggling about!*
("Since that dumb strand of idiot hair is in the shape of a question mark, what if it starts wiggling whenever there's a mystery nearby! What if my twin masters have a built-in mystery detector?! Or maybe it's just that little, green sprout that coconuts have when they're ready to grown into a palm tree, kyahaha~!!")
("...?") Yuta stares up at her with a confused look on his face, of course being unaware of his waggling ahoge!!
("H-Hey, lay off the insults! ...And I highly doubt that's what it actually is, Shinigami. If that was the case, Yuma and I would probably be detecting mysteries all the time, then.")
And at that coconut joke, he squints even harder, reaching up to 'bap' her!! ("No it isn't. Good grief... I-I'll never go a day without a stupid coconut joke, will I...Don't make me bap you with a ghost plushie, now!")
#their ahoges being mystery detectors!!! 🔎 <-❔〽️!!!!!!!!!!!!!!!!!!#Shinigami cracking da code!!! KJDNKDJS AND A KOKONUT JOKE!!!#YUTA IS VERY /NOT/ AMUSED!!!!#HE'S GONNA PRETEND TO IGNORE HER ANY SECOND NOWWW!!!#Yuta answers;;#essenceofjustice
1 note
·
View note
Text
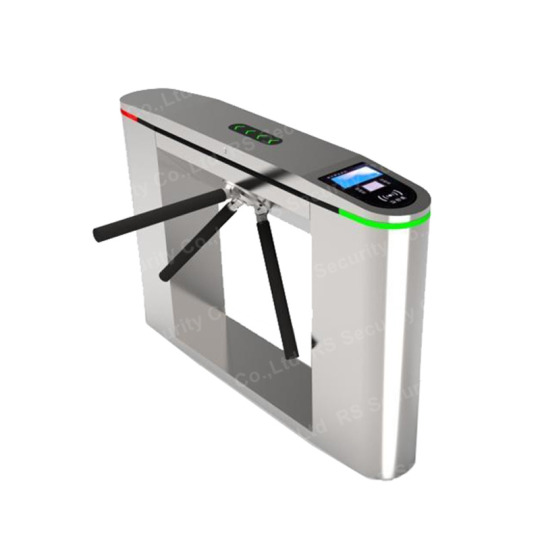
Tripod Turnstile Overview Tripod Turnstile, Swing Turnstile, as well as Flap Turnstile( RS Security Co., Ltd: www.szrssecurity.com) are contemporary control gadgets for pedestrian passages. They are used in position where the entry as well as departure of individuals need to be regulated, such as smart communities, canteens, hotels, galleries, gyms, clubs, trains, stations, docks, and so on place. Using Tripod Turnstile, Swing Turnstile, and also Flap Turnstile can make the flow of people organized. Tripod Turnstile, Swing Turnstile, Flap Turnstile are made use of in mix with smart cards, fingerprints, barcodes and also other recognition system equipment to create a smart gain access to control channel control system; they are made use of in combination with computers, accessibility control, presence, billing monitoring, ticket systems and also other software program to form a The intelligent Turnstile Gate detailed management system can recognize functions such as access control, attendance, usage, ticketing, as well as present restricting. This Turnstile Gate monitoring system is part of the "all-in-one card" and is mounted at flows such as communities, manufacturing facilities, smart structures, canteens, etc. It can complete various administration features such as employee card travel control, presence at leave job and also dishes, as well as dining. Tripod Turnstile system attributes Convenient as well as quick: review the card in and out with one swipe. Use the licensed IC card and wave it before the clever Tripod Turnstile visitor to finish the Tripod Turnstile gate opening and charge recording work. The card reading is non-directional as well as the analysis as well as composing time is 0.1 seconds, which is practical and also quick. Safety and security and also confidentiality: Use history or neighborhood confirmation, authorized issuance, and also one-of-a-kind identification, that is, the card can just be utilized in this system, and also it is risk-free and also personal. Dependability: Card radio frequency induction, stable as well as trusted, with the capability to court and also assume. Flexibility: The system can flexibly set access as well as leave control employees consents, period control, cardholder validity and blacklist loss reporting, adding cards as well as various other features. Versatility: Through consent, the user card can be utilized for "one-card" administration such as car parking, presence, accessibility control, patrol, usage, and so on, making it very easy to recognize multiple uses of one card. Simpleness: Easy to mount, easy to link, the software application has a Chinese user interface and is easy to operate. Tripod Turnstile, Swing Turnstile, as well as Flap Turnstile( RS Security Co., Ltd: www.szrssecurity.com) are modern control devices for pedestrian flows. The use of Tripod Turnstile, Swing Turnstile, and also Flap Turnstile can make the flow of people orderly. Use the accredited IC card as well as wave it in front of the clever Tripod Turnstile viewers to complete the Tripod Turnstile gate opening and charge recording job.
#3 Arms Turnstile#Strike Lock#Access Control#Loop Coil Cable#Face Recognition#79g Radar Detector#Scan Qr Code Reader#Timmy Access Control#Gate With Face Reader#6 Meters Boom Barrier
0 notes