#apacheflink
Explore tagged Tumblr posts
Text
Apache Beam For Beginners: Building Scalable Data Pipelines
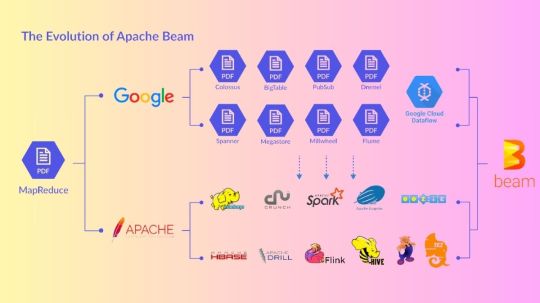
Apache Beam
Apache Beam, the simplest method for streaming and batch data processing. Data processing for mission-critical production workloads can be written once and executed anywhere.
Overview of Apache Beam
An open source, consistent approach for specifying batch and streaming data-parallel processing pipelines is called Apache Beam. To define the pipeline, you create a program using one of the open source Beam SDKs. One of Beam’s supported distributed processing back-ends, such as Google Cloud Dataflow, Apache Flink, or Apache Spark, then runs the pipeline.
Beam is especially helpful for situations involving embarrassingly parallel data processing, where the issue may be broken down into numerous smaller data bundles that can be handled separately and concurrently. Beam can also be used for pure data integration and Extract, Transform, and Load (ETL) activities. These operations are helpful for loading data onto a new system, converting data into a more suitable format, and transferring data between various storage media and data sources.Image credit to Apache Beam
How Does It Operate?
Sources of Data
Whether your data is on-premises or in the cloud, Beam reads it from a wide range of supported sources.
Processing Data
Your business logic is carried out by Beam for both batch and streaming usage cases.
Writing Data
The most widely used data sinks on the market receive the output of your data processing algorithms from Beam.
Features of Apache Beams
Combined
For each member of your data and application teams, a streamlined, unified programming model for batch and streaming use cases.
Transportable
Run pipelines across several execution contexts (runners) to avoid lock-in and provide flexibility.
Wide-ranging
Projects like TensorFlow Extended and Apache Hop are built on top of Apache Beam, demonstrating its extensibility.
Open Source
Open, community-based support and development to help your application grow and adapt to your unique use cases.
Apache Beam Pipeline Runners
The data processing pipeline you specify with your Beam program is converted by the Beam Pipeline Runners into an API that works with the distributed processing back-end of your choosing. You must designate a suitable runner for the back-end where you wish to run your pipeline when you run your Beam program.
Beam currently supports the following runners:
The Direct Runner
Runner for Apache Flink Apache Flink
Nemo Runner for Apache
Samza the Apache A runner Samza the Apache
Spark Runner for Apache Spark by Apache
Dataflow Runner for Google Cloud Dataflow on Google Cloud
Jet Runner Hazelcast Jet Hazelcast
Runner Twister 2
Get Started
Get Beam started on your data processing projects.
Visit our Getting started from Apache Spark page if you are already familiar with Apache Spark.
As an interactive online learning tool, try the Tour of Beam.
For the Go SDK, Python SDK, or Java SDK, follow the Quickstart instructions.
For examples that demonstrate different SDK features, see the WordCount Examples Walkthrough.
Explore our Learning Resources at your own speed.
on detailed explanations and reference materials on the Beam model, SDKs, and runners, explore the Documentation area.
Learn how to run Beam on Dataflow by exploring the cookbook examples.
Contribute
The Apache v2 license governs Beam, a project of the Apache Software Foundation. Contributions are highly valued in the open source community of Beam! Please refer to the Contribute section if you would want to contribute.
Apache Beam SDKs
Whether the input is an infinite data set from a streaming data source or a finite data set from a batch data source, the Beam SDKs offer a uniform programming model that can represent and alter data sets of any size. Both bounded and unbounded data are represented by the same classes in the Beam SDKs, and operations on the data are performed using the same transformations. You create a program that specifies your data processing pipeline using the Beam SDK of your choice.
As of right now, Beam supports the following SDKs for specific languages:
Java SDK for Apache Beam Java
Python’s Apache Beam SDK
SDK Go for Apache Beam Go
Apache Beam Python SDK
A straightforward yet effective API for creating batch and streaming data processing pipelines is offered by the Python SDK for Apache Beam.
Get started with the Python SDK
Set up your Python development environment, download the Beam SDK for Python, and execute an example pipeline by using the Beam Python SDK quickstart. Next, learn the fundamental ideas that are applicable to all of Beam’s SDKs by reading the Beam programming handbook.
For additional details on specific APIs, consult the Python API reference.
Python streaming pipelines
With Beam SDK version 2.5.0, the Python streaming pipeline execution is possible (although with certain restrictions).
Python type safety
Python lacks static type checking and is a dynamically typed language. In an attempt to mimic the consistency assurances provided by real static typing, the Beam SDK for Python makes use of type hints both during pipeline creation and runtime. In order to help you identify possible issues with the Direct Runner early on, Ensuring Python Type Safety explains how to use type hints.
Managing Python pipeline dependencies
Because the packages your pipeline requires are installed on your local computer, they are accessible when you execute your pipeline locally. You must, however, confirm that these requirements are present on the distant computers if you wish to run your pipeline remotely. Managing Python Pipeline Dependencies demonstrates how to enable remote workers to access your dependencies.
Developing new I/O connectors for Python
You can develop new I/O connectors using the flexible API offered by the Beam SDK for Python. For details on creating new I/O connectors and links to implementation guidelines unique to a certain language, see the Developing I/O connectors overview.
Making machine learning inferences with Python
Use the RunInference API for PyTorch and Scikit-learn models to incorporate machine learning models into your inference processes. You can use the tfx_bsl library if you’re working with TensorFlow models.
The RunInference API allows you to generate several kinds of transforms since it accepts different kinds of setup parameters from model handlers, and the type of parameter dictates how the model is implemented.
An end-to-end platform for implementing production machine learning pipelines is called TensorFlow Extended (TFX). Beam has been integrated with TFX. Refer to the TFX user handbook for additional details.
Python multi-language pipelines quickstart
Transforms developed in any supported SDK language can be combined and used in a single multi-language pipeline with Apache Beam. Check out the Python multi-language pipelines quickstart to find out how to build a multi-language pipeline with the Python SDK.
Unrecoverable Errors in Beam Python
During worker startup, a few typical mistakes might happen and stop jobs from commencing. See Unrecoverable faults in Beam Python for more information on these faults and how to fix them in the Python SDK.
Apache Beam Java SDK
A straightforward yet effective API for creating batch and streaming parallel data processing pipelines in Java is offered by the Java SDK for Apache Beam.
Get Started with the Java SDK
Learn the fundamental ideas that apply to all of Beam’s SDKs by beginning with the Beam Programming Model.
Further details on specific APIs can be found in the Java API Reference.
Supported Features
Every feature that the Beam model currently supports is supported by the Java SDK.
Extensions
A list of available I/O transforms may be found on the Beam-provided I/O Transforms page.
The following extensions are included in the Java SDK:
Inner join, outer left join, and outer right join operations are provided by the join-library.
For big iterables, sorter is a scalable and effective sorter.
The benchmark suite Nexmark operates in both batch and streaming modes.
A batch-mode SQL benchmark suite is called TPC-DS.
Euphoria’s Java 8 DSL for BEAM is user-friendly.
There are also a number of third-party Java libraries.
Java multi-language pipelines quickstart
Transforms developed in any supported SDK language can be combined and used in a single multi-language pipeline with Apache Beam. Check out the Java multi-language pipelines quickstart to find out how to build a multi-language pipeline with the Java SDK.
Read more on govindhtech.com
#ApacheBeam#BuildingScalableData#Pipelines#Beginners#ApacheFlink#SourcesData#ProcessingData#WritingData#TensorFlow#OpenSource#GoogleCloud#ApacheSpark#ApacheBeamSDK#technology#technews#Python#machinelearning#news#govindhtech
0 notes
Link
0 notes
Photo

Data Flair online learning portal have any information visit our website : https://data-flair.training/blogs/
0 notes
Photo
AWS supports the @ApacheFlink community & is a platinum sponsor at @FlinkForward Europe 2019. Join us at the conference with code FFEU19-AWS. https://t.co/5jnC685gwn https://t.co/5bU7DyZ2ER (via Twitter http://twitter.com/awscloud/status/1178734760832782336)
0 notes
Link
More dynamic sources (varying number of segments rather than fix number of partitions) have many operational advantages. But they need a bit more finesse to provide consistent semantics. @fpjunqueira and Tom Kaitchuck on how @PravegaIO handles this when working with @ApacheFlink. pic.twitter.com/JlNhkkupxy
— Stephan Ewen (@StephanEwen) April 22, 2020
0 notes
Text
Favorite tweets
Want to know what makes Flink achieve high throughput and low latency? The latest post dives into Flink’s Network Stack and explains what is going on under the hood of your Flink jobs: https://t.co/a8OxXbPaCw pic.twitter.com/0epfMLY3LW
— Apache Flink (@ApacheFlink) June 5, 2019
via IFTTT
0 notes
Text
Benchmarking Streaming Computation Engines at Yahoo!
(Yahoo Storm Team in alphabetical order) Sanket Chintapalli, Derek Dagit, Bobby Evans, Reza Farivar, Tom Graves, Mark Holderbaugh, Zhuo Liu, Kyle Nusbaum, Kishorkumar Patil, Boyang Jerry Peng and Paul Poulosky.
DISCLAIMER: Dec 17th 2015 data-artisans has pointed out to us that we accidentally left on some debugging in the flink benchmark. So the flink numbers should not be directly compared to the storm and spark numbers. We will rerun and repost the numbers when we have fixed this.
UPDATE: Dec 18, 2015 there was a miscommunication and the code that was checked in was not the exact code we ran with for flink. The real code had the debugging removed. Data-Artisans has looked at the code and confirmed it and the current numbers are good. We will still rerun at some point soon.
Executive Summary - Due to a lack of real-world streaming benchmarks, we developed one to compare Apache Flink, Apache Storm and Apache Spark Streaming. Storm 0.10.0, 0.11.0-SNAPSHOT and Flink 0.10.1 show sub- second latencies at relatively high throughputs with Storm having the lowest 99th percentile latency. Spark streaming 1.5.1 supports high throughputs, but at a relatively higher latency.
At Yahoo, we have invested heavily in a number of open source big data platforms that we use daily to support our business. For streaming workloads, our platform of choice has been Apache Storm, which replaced our internally developed S4 platform. We have been using Storm extensively, and the number of nodes running Storm at Yahoo has now reached about 2,300 (and is still growing).
Since our initial decision to use Storm in 2012, the streaming landscape has changed drastically. There are now several other noteworthy competitors including Apache Flink, Apache Spark (Spark Streaming), Apache Samza, Apache Apex and Google Cloud Dataflow. There is increasing confusion over which package offers the best set of features and which one performs better under which conditions (for instance see here, here, here, and here).
To provide the best streaming tools to our internal customers, we wanted to know what Storm is good at and where it needs to be improved compared to other systems. To do this we started to look for stream processing benchmarks that we could use to do this evaluation, but all of them were lacking in several fundamental areas. Primarily, they did not test with anything close to a real world use case. So we decided to write one and released it as open source https://github.com/yahoo/streaming-benchmarks. In our initial evaluation we decided to limit our test to three of the most popular and promising platforms (Storm, Flink and Spark), but welcome contributions for other systems, and to expand the scope of the benchmark.
Benchmark Design
The benchmark is a simple advertisement application. There are a number of advertising campaigns, and a number of advertisements for each campaign. The job of the benchmark is to read various JSON events from Kafka, identify the relevant events, and store a windowed count of relevant events per campaign into Redis. These steps attempt to probe some common operations performed on data streams.
The flow of operations is as follows (and shown in the following figure):
Read an event from Kafka.
Deserialize the JSON string.
Filter out irrelevant events (based on event_type field)
Take a projection of the relevant fields (ad_id and event_time)
Join each event by ad_id with its associated campaign_id. This information is stored in Redis.
Take a windowed count of events per campaign and store each window in Redis along with a timestamp of the time the window was last updated in Redis. This step must be able to handle late events.
The input data has the following schema:
user_id: UUID
page_id: UUID
ad_id: UUID
ad_type: String in {banner, modal, sponsored-search, mail, mobile}
event_type: String in {view, click, purchase}
event_time: Timestamp
ip_address: String
Producers create events with timestamps marking creation time. Truncating this timestamp to a particular digit gives the begin-time of the time window the event belongs in. In Storm and Flink, updates to Redis are written periodically, but frequently enough to meet a chosen SLA. Our SLA was 1 second, so once per second we wrote updated windows to Redis. Spark operated slightly differently due to great differences in its design. There’s more details on that in the Spark section. Along with the data, we record the time at which each window in Redis was last updated.
After each run, a utility reads windows from Redis and compares the windows’ times to their last_updated_at times, yielding a latency data point. Because the last event for a window cannot have been emitted after the window closed but will be very shortly before, the difference between a window’s time and its last_updated_at time minus its duration represents the time it took for the final tuple in a window to go from Kafka to Redis through the application.
window.final_event_latency = (window.last_updated_at – window.timestamp) – window.duration
This is a bit rough, but this benchmark was not purposed to get fine-grained numbers on these engines, but to provide a more high-level view of their behavior.
Benchmark setup
10 second windows
1 second SLA
100 campaigns
10 ads per campaign
5 Kafka nodes with 5 partitions
1 Redis node
10 worker nodes (not including coordination nodes like Storm’s Nimbus)
5-10 Kafka producer nodes
3 ZooKeeper nodes
Since the Redis node in our architecture only performs in-memory lookups using a well-optimized hashing scheme, it did not become a bottleneck. The nodes are homogeneously configured, each with two Intel E5530 processors running at 2.4GHz, with a total of 16 cores (8 physical, 16 hyperthreading) per node. Each node has 24GiB of memory, and the machines are all located within the same rack, connected through a gigabit Ethernet switch. The cluster has a total of 40 nodes available.
We ran multiple instances of the Kafka producers to create the required load since individual producers begin to fall behind at around 17,000 events per second. In total, we use anywhere between 20 to 25 nodes in this benchmark.
The use of 10 workers for a topology is near the average number we see being used by topologies internal to Yahoo. Of course, our Storm clusters are larger in size, but they are multi-tenant and run many topologies.
To begin the benchmarks Kafka is cleared, Redis is populated with initial data (ad_id to campaign_id mapping), the streaming job is started, and then after a bit of time to let the job finish launching, the producers are started with instructions to produce events at a particular rate, giving the desired aggregate throughput. The system was left to run for 30 minutes before the producers were shut down. A few seconds were allowed for all events to be processed before the streaming job itself was stopped. The benchmark utility was then run to generate a file containing a list of window.last_updated_at – window.timestamp numbers. These files were saved for each throughput we tested and then were used to generate the charts in this document.
Flink
The benchmark for Flink was implemented in Java by using Flink’s DataStream API. The Flink DataStream API has many similarities to Storm’s streaming API. For both Flink and Storm, the dataflow can be represented as a directed graph. Each vertex is a user defined operator and each directed edge represents a flow of data. Storm’s API uses spouts and bolts as its operators while Flink uses map, flatMap, as well as many pre-built operators such as filter, project, and reduce. Flink uses a mechanism called checkpointing to guarantee processing which offers similar guarantees to Storm’s acking. Flink has checkpointing off by default and that is how we ran this benchmark. Notable configs we used in Flink is listed below:
taskmanager.heap.mb: 15360
taskmanager.numberOfTaskSlots: 16
The Flink version of the benchmark uses the FlinkKafkaConsumer to read data in from Kafka. The data read in from Kafka—which is in a JSON formatted string— is then deserialized and parsed by a custom defined flatMap operator. Once deserialized, the data is filtered via a custom defined filter operator. Afterwards, the filtered data is projected by using the project operator. From there, the data is joined with data in Redis by a custom defined flapMap function. Lastly, the final results are calculated from the data and written to redis.
The rate at which Kafka emitted data events into the Flink benchmark is varied from 50,000 events/sec to 170,000 events/sec. For each Kafka emit rate, the percentile latency for a tuple to be completely processed in the Flink benchmark is illustrated in the graph below.
The percentile latency for all Kafka emit rates are relatively the same. The percentile latency rises linearly until around the 99th percentile, where the latency appears to increase exponentially.
Spark
For the Spark benchmark, the code was written in Scala. Since the micro-batching methodology of Spark is different than the pure streaming nature of Storm, we needed to rethink parts of the benchmark. Storm and Flink benchmarks would update the Redis database once a second to try and meet our SLA, keeping the intermediate update values in a local cache. As a result, the batch duration in the Spark streaming version was set to 1 second, at least for smaller amounts of traffic. We had to increase the batch duration for larger throughputs.
The benchmark is written in a typical Spark style using DStreams. DStreams are the streaming equivalent of regular RDDs, and create a separate RDD for every micro batch. Note that in the subsequent discussion, we use the term “RDD” instead of “DStream” to refer to the RDD representation of the DStream in the currently active microbatch. Processing begins with the direct Kafka consumer included with Spark 1.5. Since the Kafka input data in our benchmark is stored in 5 partitions, this Kafka consumer creates a DStream with 5 partitions as well. After that, a number of transformations are applied on the DStreams, including maps and filters. The transformation involving joining data with Redis is a special case. Since we do not want to create a separate connection to Redis for each record, we use a mapPartitions operation that can give control of a whole RDD partition to our code. This way, we create one connection to Redis and use this single connection to query information from Redis for all the events in that RDD partition. The same approach is used later when we update the final results in Redis.
It should be noted that our writes to Redis were implemented as a side-effect of the execution of the RDD transformation in order to keep the benchmark simple, so this would not be compatible with exactly-once semantics.
We found that with high enough throughput, Spark was not able to keep up. At 100,000 messages per second the latency greatly increased. We considered adjustments along two control dimensions to help Spark cope with increasing throughput.
The first is the microbatch duration. This is a control dimension that is not present in a pure streaming system like Storm. Increasing the duration increases latency while reducing overhead and therefore increasing maximum throughput. The challenge is that the choice of the optimal batch duration that minimizes latency while allowing spark to handle the throughput is a time consuming process. Essentially, we have to set a batch duration, run the benchmark for 30 minutes, check the results and decrease/increase the duration.
The second dimension is parallelism. However, increasing parallelism is simpler said than done in the case of Spark. For a true streaming system like Storm, one bolt instance can send its results to any number of subsequent bolt instances by using a random shuffle. To scale, one can increase the parallelism of the second bolt. In the case of a micro batch system like Spark, we need to perform a reshuffle operation similar to how intermediate data in a Hadoop MapReduce program are shuffled and merged across the cluster. But the reshuffling itself introduces considerable overhead. Initially, we thought our operations were CPU-bound, and so the benefits of reshuffling to a higher number of partitions would outweigh the cost of reshuffling. Instead, we found the bottleneck to be scheduling, and so reshuffling only added overhead. We suspect that at higher throughput rates or with operations that are CPU-bound, the reverse would be true.
The final results are interesting. There are essentially three behaviors for a Spark workload depending on the window duration. First, if the batch duration is set sufficiently large, the majority of the events will be handled within the current micro batch. The following figure shows the resulting percentile processing graph for this case (100K events, 10 seconds batch duration).
But whenever 90% of events are processed in the first batch, there is possibility of improving latency. By reducing the batch duration sufficiently, we get into a region where the incoming events are processed within 3 or 4 subsequent batches. This is the second behavior, in which the batch duration puts the system on the verge of falling behind, but is still manageable, and results in better latency. This situation is shown in the following figure for a sample throughput rate (100K events, 3 seconds batch duration).
Finally, the third behavior is when Spark streaming falls behind. In this case, the benchmark takes a few minutes after the input data finishes to process all of the events. This situation is shown in the following figure. Under this undesirable operating region, Spark spills lots of data onto disks, and in extreme cases we could end up running out of disk space.
One final note is that we tried the new back pressure feature introduced in Spark 1.5. If the system is in the first operating region, enabling back pressure does nothing. In the second operating region, enabling back pressure results in longer latencies. The third operating region is where back pressure shows the most negative impact. It changes the batch length, but Spark still cannot cope with the throughput and falls behind. This is shown in the next figures. Our experiments showed that the current back pressure implementation did not help our benchmark, and as a result we disabled it.
Performance without back pressure (top), and with back pressure enabled (bottom). The latencies with the back pressure enabled are worse (70 seconds vs 120 seconds). Note that both of these results are unacceptable for a streaming system as both fall behind the incoming data. Batch duration was set to 2 seconds for each run, with 130,000 throughput.
Storm
Storm’s benchmark was written using the Java API. We tested both Apache Storm 0.10.0 release and a 0.11.0 snapshot. The snapshot’s commit hash was a8d253a. One worker process per host was used, and each worker was given 16 tasks to run in 16 executors - one for each core.
Storm 0.10.0:
Storm 0.11.0:
Storm compared favorably to both Flink and Spark Streaming. Storm 0.11.0 beat Storm 0.10.0, showing the optimizations that have gone in since the 0.10.0 release. However, at high-throughput both versions of Storm struggled. Storm 0.10.0 was not able to handle throughputs above 135,000 events per second.
Storm 0.11.0 performed similarly until we disabled acking. In the benchmarking topology, acking was used for flow control but not for processing guarantees. In 0.11.0, Storm added a simple back pressure controller, allowing us to avoid the overhead of acking. With acking enabled, 0.11.0 performed terribly at 150,000/s—slightly better than 0.10.0, but still far worse than anything else. With acking disabled, Storm even beat Flink for latency at high throughput. However, with acking disabled, the ability to report and handle tuple failures is disabled also.
Conclusions and Future Work
It is interesting to compare the behavior of these three systems. Looking at the following figure, we can see that Storm and Flink both respond quite linearly. This is because these two systems try to process an incoming event as it becomes available. On the other hand, the Spark Streaming system behaves in a stepwise function, a direct result from its micro-batching design.
The throughput vs latency graph for the various systems is maybe the most revealing, as it summarizes our findings with this benchmark. Flink and Storm have very similar performance, and Spark Streaming, while it has much higher latency, is expected to be able to handle much higher throughput.
We did not include the results for Storm 0.10.0 and 0.11.0 with acking enabled beyond 135,000 events per second, because they could not keep up with the throughput. The resulting graph had the final point for Storm 0.10.0 in the 45,000 ms range, dwarfing every other line on the graph. The longer the topology ran, the higher the latencies got, indicating that it was losing ground.
All of these benchmarks except where otherwise noted were performed using default settings for Storm, Spark, and Flink, and we focused on writing correct, easy to understand programs without optimizing each to its full potential. Because of this each of the six steps were a separate bolt or spout. Flink and Spark both do operator combining automatically, but Storm (without Trident) does not. What this means for Storm is that events go through many more steps and have a higher overhead compared to the other systems.
In addition to further optimizations to Storm, we would like to expand the benchmark in terms of functionality, and to include other stream processing systems like Samza and Apex. We would also like to take into account fault tolerance, processing guarantees, and resource utilization.
The bottom line for us is Storm did great. Writing topologies is simple, and it’s easy to get low latency comparable to or better than Flink up to fairly high throughputs. Without acking, Storm even beat Flink at very high throughput, and we expect that with further optimizations like combining bolts, more intelligent routing of tuples, and improved acking, Storm with acking enabled would compete with Flink at very high throughput too.
The competition between near real time streaming systems is heating up, and there is no clear winner at this point. Each of the platforms studied here have their advantages and disadvantages. Performance is but one factor among others, such as security or integration with tools and libraries. Active communities for these and other big data processing projects continue to innovate and benefit from each other’s advancements. We look forward to expanding this benchmark and testing newer releases of these systems as they come out.
#apachestorm#apachespark#apacheflink#streaming#realtime#benchmark#yahoo#performance#open source#yahoo engineering
42 notes
·
View notes
Text
BigQuery Engine For Apache Flink: Fully Serverless Flink
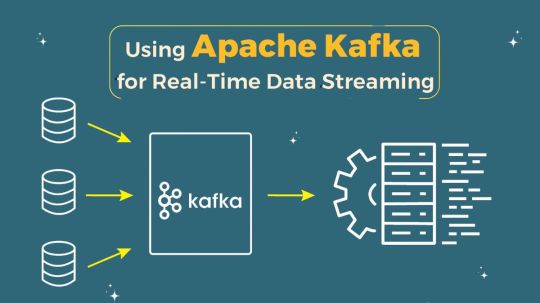
The goal of today’s companies is to become “by-the-second” enterprises that can quickly adjust to shifts in their inventory, supply chain, consumer behavior, and other areas. Additionally, they aim to deliver outstanding customer experiences, whether it is via online checkout or support interactions. All businesses, regardless of size or budget, should have access to real-time intelligence, in opinion, and it should be linked into a single data platform so that everything functions as a whole. With the release of BigQuery Engine for Apache Flink in preview today, we’re making significant progress in assisting companies in achieving these goals.
BigQuery Engine for Apache Flink
Construct and operate applications that are capable of real-time streaming by utilizing a fully managed Flink service that is linked with BigQuery.
Features
Update your unified data and AI platform with real-time data
Using a scalable and well-integrated streaming platform built on the well-known Apache Flink and Apache Kafka technologies, make business decisions based on real-time insights. You can fully utilize your data when paired with Google’s unique AI/ML capabilities in BigQuery. With built-in security and governance, you can scale efficiently and iterate quickly without being constrained by infrastructure management.
Use a serverless Flink engine to save time and money
Businesses use Google Cloud to develop streaming apps in order to benefit from real-time data. The operational strain of administering self-managed Flink, optimizing innumerable configurations, satisfying the demands of various workloads while controlling expenses, and staying up to date with updates, however, frequently weighs them down. The serverless nature of BigQuery Engine for Apache Flink eases this operational load and frees its clients to concentrate on their core competencies, which include business innovation.
Compatible with Apache Flink, an open source project
Without rewriting code or depending on outside services, BigQuery Engine for Apache Flink facilitates the lifting and migration of current streaming applications that use the free source Apache Flink framework to Google Cloud. Modernizing and migrating your streaming analytics on Google Cloud is simple when you combine it with Google Managed Service for Apache Kafka (now GA).
Streamling ETL
ETL streaming for your data platform that is AI-ready
An open and adaptable framework for real-time ETL is offered by Apache Flink, which enables you to ingest data streams from sources like as Kafka, carry out transformations, and then immediately load them into BigQuery for analysis and storage. With the advantages of open source extensibility and adaptation to various data sources, this facilitates quick data analysis and quicker decision-making.
Create applications that are event-driven
Event-driven apps assist businesses with marketing personalization, recommendation engines, fraud detection models, and other issues. The managed Apache Kafka service from Google Cloud can be used to record real-time event streams from several sources, such as user activity or payments. These streams are subsequently processed by the Apache Flink engine with minimal latency, allowing for sophisticated tasks like real-time processing.
Build a real-time data and AI platform
Apache’s BigQuery Engine You may use Flink for stream analytics without having to worry about infrastructure management. Use the SQL or DataStream APIs in Flink to analyze data in real time. Stream your data to BigQuery and link it to visualization tools to create dashboards. Use Flink’s libraries for streaming machine learning and keep an eye on work performance.
The cutting-edge real-time intelligence platform offered by BigQuery Engine for Apache Flink enables users to:
Utilize Google Cloud‘s well-known streaming technology. Without rewriting code or depending on outside services, BigQuery Engine for Apache Flink facilitates the lifting and migration of current streaming applications that use the open-source Apache Flink framework to Google Cloud. Modernizing and migrating your streaming analytics on Google Cloud is simple when you combine it with Google Managed Service for Apache Kafka (now GA).
Lessen the strain on operations. Because BigQuery Engine for Apache Flink is completely serverless, it lessens operational load and frees up clients to concentrate on their core competencies innovating their businesses.
Give AI real-time data. A scalable and well-integrated streaming platform built on the well-known Apache Flink and Apache Kafka technologies that can be combined with Google’s unique AI/ML capabilities in BigQuery is what enterprise developers experimenting with gen AI are searching for.
With the arrival of BigQuery Engine for Apache Flink, Google Cloud customers are taking advantage of numerous real-time analytics innovations, such as BigQuery continuous queries, which allow users to use SQL to analyze incoming data in BigQuery in real-time, and Dataflow Job Builder, which assists users in defining and implementing a streaming pipeline through a visual user interface.
Google cloud streaming offering now includes popular open-source Flink and Kafka systems, SQL-based easy streaming with BigQuery continuous queries, and sophisticated multimodal data streaming with Dataflow, including support for Iceberg, thanks to BigQuery Engine for Apache Flink. These features are combined with BigQuery, which links your data to top AI tools in the market, such as Gemma, Gemini, and open models.
New AI capabilities unlocked when your data is real-time
It is evident that generative AI has rekindled curiosity about the possibilities of data-driven experiences and insights as a turn to the future. When AI, particularly generative AI, has access to the most recent context, it performs best. Retailers can customize their consumers’ purchasing experiences by fusing real-time interactions with historical purchase data. If your business provides financial services, you can improve your fraud detection model by using real-time transactions. Fresh data for model training, real-time user support through Retrieval Augmented Generation (RAG), and real-time predictions and inferences for your business applications including incorporating tiny models like Gemma into your streaming pipelines are all made possible by real-time data coupled to AI.
In order to enable real-time data for your future AI use cases, it is adopting a platform approach to introduce capabilities across the board, regardless of the particular streaming architecture you want or the streaming engine you select. Building real-time AI applications is now easier than ever with to features like distributed counting in Bigtable, the RunInference transform, support for Vertex AI text-embeddings, Dataflow enrichment transforms, and many more.
When it comes to enabling your unified data and AI platform to function in real-time data, Google cloud are thrilled to put these capabilities in your hands and keep providing you with additional options and flexibility. Get started utilizing BigQuery Engine for Apache Flink right now in the Google Cloud console by learning more about it.
Read more on Govindhtech.com
#BigQuery#BigQueryEngine#ApacheFlink#AI#SQL#ApacheKafka#generativeAI#cloudcomputing#VertexAI#News#Technews#Technology#Technologynews#Technologytrends#govindhtech
0 notes
Link
0 notes
Photo

DataFlair provides certified training courses for Big Data and Hadoop, Apache Spark and Scala, Flink, Data Science, Kafka, Storm, Hadoop Admin, Hadoop Architect.For more information visit our website: https://data-flair.training/
0 notes
Text
Get Started with IBM Event Automation on AWS Marketplace
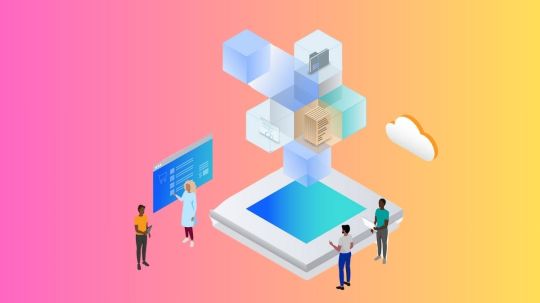
Businesses can expedite their event-driven initiatives, no matter where they are in their journey, with IBM Event Automation, a completely composable solution. Unlocking the value of events requires an event-driven architecture, which is made possible by the event streams, event processing capabilities, and event endpoint management.
IBM Event Automation
The volume of data generated in an organisation is increasing at an exponential rate due to the hundreds of events that take place there. Imagine being able to access these continuous information streams, make connections between seemingly unrelated occurrences, and identify emerging patterns, client problems, or threats from competitors as they arise.
IBM Event Automation has the following features:
Event Streams: Use enterprise-grade Apache Kafka to gather and disseminate raw streams of business events occurring in real time. With a single, integrated interface, manage your Apache Kafka deployments, balance workloads, browse messages, and keep an eye on important metrics. Utilise the Kafka Connect framework to link easily with hundreds of popular endpoints, such as IBM MQ, SAP, and more.
Event Endpoint Management: Enable control and governance while encouraging the sharing and repurposing of your event sources. With ease, describe and record your events using the AsyncAPI specification, which is available as open source. Provide a self-service event source catalogue that users can peruse, use, and distribute. Using an event gateway, enforce runtime restrictions to protect and manage access to anything that can talk the Kafka protocol.
Event Processing: Create and test stream processing flows in real time using an easy-to-use authoring canvas by utilising the power of Apache Flink. At each stage, receive support and validation as you filter, collect, alter, and connect streams of events. Give business and IT users the tools they need to create business scenarios, recognise them when they happen, and take immediate action.
Information on Prices
Below are the total costs for these different subscription durations. Additional taxes or fees may apply.UnitsDescription12 MONTHSIBM Event Automation VPCsEntitlement to IBM Event Automation VPCs$3,396
Information on Usage
Fulfilment Options
SaaS, or software as a service
A software distribution paradigm known as “software as a service” involves the vendor hosting and managing the program online. Consumers do not own the underlying infrastructure; they only pay to use the software. Customers will pay for consumption through their AWS payment when using SaaS Contracts.
End-User License Contract
You accept the terms and conditions stated in the product’s End User License Agreement (EULA) by subscribing to this product.
Supporting Details
Software: IBM Event Automation
A benefit of IBM Event Automation is having access to IBM Support, which is offered everywhere, around-the-clock, every day of the year. Use this link to reach IBM Support: http://www.ibm.com/mysupport
Refund Guidelines
No reimbursements without IBM’s consent
You may now get IBM Event Automation on the AWS Marketplace. This represents a major advancement in deployment flexibility and accessibility for global enterprise clients. As of right now, companies can use their current AWS Marketplace Enterprise Discount Program (EDP) funds to purchase IBM Event Automation as self-managed software. This makes it possible for companies to purchase subscription licenses using the bring your own license (BYOL) strategy.
This breakthrough creates new opportunities for enterprises looking for seamless integration of sophisticated event-driven architecture capabilities into their AWS infrastructures. IBM ensures that customers can effortlessly build and maintain event-driven software capabilities while using AWS’s massive infrastructure and worldwide reach by listing IBM Event Automation on the AWS Marketplace.
With open plans available in more than 80 countries, IBM Event Automation is accessible worldwide. Clients can still seek access to IBM Event Automation by contacting their local IBM representative, even if they do not reside in one of the 80 mentioned countries. Because of its widespread availability, companies of all shapes and sizes can take use of its potential to successfully optimise their event management procedures. Clients can maximise their AWS budget under the BYOL subscription model by utilising their current IBM Event Automation licenses. This strategy aligns with cost optimisation initiatives and lowers upfront expenditures.
The ability to seamlessly integrate with other AWS services is made possible by hosting on the AWS Marketplace. This improves operational efficiency and gives clients access to a vast cloud ecosystem for their event-led integration requirements. AWS container environments, such Red Hat OpenShift Service on AWS (ROSA) and Amazon Elastic Kubernetes Service (EKS), can be deployed using IBM Event Automation. This containerised method is ideal for contemporary cloud-native designs since it ensures scalability, flexibility, and economical resource usage.
Customers can purchase IBM Event Automation on AWS Marketplace and install it directly in their own AWS environment, giving them more control over deployment details and customisation. Businesses planning to use the AWS Marketplace to implement IBM Event Automation should take into account a number of operational factors:
Infrastructure readiness refers to making sure the AWS environment satisfies the required security and performance standards and is ready to host containerised applications.
Licensing management: Controlling license usage to maximise cost-effectiveness, adhere to AWS Marketplace standards, and comply with IBM’s BYOL terms.
Integration and support: To expedite integration and address any operational obstacles, take advantage of IBM’s experience and AWS support services.
To sum up, the inclusion of IBM Event Automation in the AWS Marketplace is a calculated step towards providing businesses with advanced event management tools for their AWS setups. Organisations can improve cost optimisation, scalability, and operational efficiency by implementing containerised deployment choices and implementing a BYOL subscription model. Additionally, these strategies can facilitate smooth integration with AWS services. Businesses’ growing need for effective event-driven operations may be supported by IBM Event Automation on AWS Marketplace as they continue to embrace digital transformation and cloud adoption.
Read more on govindhtech.com
#GetStartedwithIBM#Automation#AWSMarketplace#usingSaaS#news#ApacheFlink#ApacheKafka#IBMEventAutomation#IBMSupport#AsyncAPIspecification#RedHatOpenShiftServiceonAWS#awsservices#technology#technews#govindhtech
0 notes
Link
I'll be showcasing some Stateful Serverless apps in action with @ApacheFlink @statefun_io and FaaS solutions, using @awscloud Lambda as an example! If you are interested in Stateful Functions, also highly recommend attending keynote by @StephanEwen today @FlinkForward Virtual https://t.co/EPchnoq3PZ
— Tzu-Li (Gordon) Tai (@tzulitai) April 22, 2020
0 notes
Link
Learn how to dynamically reconfigure the business logic of your Apache Flink application at runtime in the latest story by @alex_fedulov on the #Flink blog: https://t.co/scOgdSDq3m pic.twitter.com/c6SpQDFNB8
— Apache Flink (@ApacheFlink) March 24, 2020
0 notes
Link
Check out the second part of my "Fraud-Detection with @ApacheFlink" case study series to learn how to use Flink's broadcast mechanism to dynamically update application logic at runtime.#streamprocessing #apacheflinkhttps://t.co/rMN6XQP68d pic.twitter.com/qtoUz93ZoV
— Alexander Fedulov (@alex_fedulov) March 24, 2020
0 notes
Link
@ApacheFlink has a sophisticated SQL engine for both incremental view materialization and for really fast batch queries. UDFs in Java/Scala/Python. Plus you can "escape" from SQL into DataStreams for additional functionality.https://t.co/AFYjHzQarFhttps://t.co/DsXufKAbCi
— Stephan Ewen (@StephanEwen) February 21, 2020
0 notes