#Multicloud
Explore tagged Tumblr posts
Text
DevOps Multicloud is revolutionizing the DevOps game by allowing teams to deploy and manage applications seamlessly across multiple cloud environments, enhancing flexibility, resilience, and scalability.
2 notes
·
View notes
Text
IT Modernization and Cloud Adoption Services in Dallas, Texas, Spearhead Technology
IT Modernization and Cloud Adoption Services in Dallas, Texas, Spearhead Technology
IT modernization and cloud adoption services are designed to help organizations update their technology infrastructure and move their operations to the cloud.
This can include migrating applications and data to cloud-based platforms, implementing new tools and technologies to improve efficiency and security, and leveraging analytics and automation to drive innovation and growth.
The benefits of IT modernization and cloud adoption can include reduced costs, improved agility and scalability, enhanced collaboration and communication, and better overall performance and customer satisfaction.
However, it is important to work with a reputable and experienced provider to ensure a smooth and successful transition to the cloud.
#cloudadoption#cloudmigration#cloudstrategy#cloudconsulting#cloudtransformation#cloudcomputing#cloudtechnology#hybridcloud#multicloud#publiccloud#privatecloud#cloudsecurity#cloudmanagement#cloudoptimization#cloudarchitecture#clouddeployment#cloudintegration#cloudsolutions#cloudservices#itmodernization#DigitalTransformation#BusinessTransformation#Spearhead#SpearheadTechnology#Dallas#Texas#USA#startups
2 notes
·
View notes
Text
Is Your Organization Ready for Multi-Cloud? Key Insights for 2024
As organizations expand their digital footprints, multi-cloud management has emerged as a cornerstone for operational flexibility and efficiency. Valued at $8.03 billion in 2023, the multi-cloud market is projected to grow at a CAGR of 28.0% from 2024 to 2030. This growth reflects the rising demand for redundancy, vendor flexibility, and optimized resource allocation. Multi-cloud strategies allow companies to avoid vendor lock-in, balance workloads, and customize services based on unique needs. Interoperability remains a priority, with tools like Kubernetes and Cloud Management Platforms facilitating smooth cross-cloud integrations.
#multicloud#cloudcomputing#digitaltransformation#cloudmanagement#industry40#cloudinfrastructure#technologytrends#businessinnovation#cloudsecurity#digitalstrategy
0 notes
Text
Dell PowerMax For Multicloud Cybersecurity Improvements

PowerMax Innovations Increase Cybersecurity, Multicloud Agility, and AI-Powered Efficiency. Businesses want IT solutions that can keep up with current demands and foresee future requirements in the fast-paced digital environment of today.
Dell is releasing major updates to Dell PowerMax today that boost cyber resilience, provide seamless multicloud mobility, and improve AI-driven efficiency. PowerMax, the consistently cutting-edge, highly secure storage solution tailored for mission-critical workloads, now has additional features that make it simpler than ever for clients to adapt to changing business needs.
AI-Driven Efficiency for Mission-Critical Workloads
Businesses want storage solutions that can keep up with existing demands and foresee future requirements in the fast-paced digital environment of today. Herein lies the potential of artificial intelligence. Customers may benefit from a number of AI-powered features in this version that can assist maximize performance, lower maintenance costs, and stop problems before they start by:
Performance optimization: By using AI to reduce latency and increase speed without requiring administration overhead, its are utilizing dynamic cache optimization via pattern recognition and predictive analytics.
Management that is both proactive and predictive: Intelligent threshold settings for autonomous health checks enable self-healing and remedial measures, addressing problems before they occur (e.g. storage capacity levels, loose cabling).
Network fabric performance optimization (FPIN) that is automated: PowerMax can resolve incidents up to 8 times quicker by rapidly identifying Fibre Channel network congestion (slow drain) and identifying the underlying reason.
Infrastructure optimization is made quick and simple with Dell’s AIOps Assistant, which has Gen AI natural language questions.
Improved Efficiency
In order to enhance total storage efficiency and provide industry-leading power and environmental monitoring features, the most recent update also adds 92% RAID efficiency (RAID 6 24+2).
In order to increase power efficiency, control energy expenses, and successfully lower energy consumption, customers may now monitor power utilization at three different levels: the array, the rack, and the data center.
Increasing Cyber Resilience
Cyber resilience is essential for all clients at a time when cyberthreats are becoming more complex. PowerMax incorporates innovative cybersecurity features to improve client data safety, minimize attack surfaces, and swiftly recover from cyberattacks. These features include:
PowerMax Cyber Recovery Services: Strong defense against cyberattacks is provided by Dell’s new Professional Service. This customized solution guarantees rapid and effective recovery while assisting clients in meeting strict compliance objectives via the use of a secure PowerMax vault and granular data protection.
YubiKey multifactor authentication: Offers a robust and practical security solution that streamlines the user authentication process and improves protection against unwanted access.
Superior Performance at Scale
With the headroom required for present and future demands, PowerMax keeps raising the bar for outstanding performance at scale. The announcement for today also adds:
PowerMax 8500 may increase IOPS performance by up to 30%.
With new 100Gb Ethernet I/O modules, GbE connection may be up to three times quicker.
With the new 64Gb Fibre Channel I/O modules, FC communication may be up to two times quicker.
Along with these noteworthy improvements, Storage Direct Protection for PowerMax‘s connection with PowerProtect allows for effective, safe, and lightning-fast data protection, providing up to 500TB per day restores and 1PB per day backups.
Reach Multicloud Quickness
Multicloud agility is crucial for optimizing resource use, cutting expenses, and quickly adjusting to change in the rapidly changing digital world. This release assists users in achieving:
Seamless multicloud data mobility: Moving live PowerMax workloads to and from APEX Block Storage, the most robust and adaptable cloud storage available in the market, is now possible using Dell’s easy-to-use solutions. At the same time, multi-hop OS conversions are carried out to update those workloads in a single, seamless operation.
Scalable cloud restorations and backups: Simple, safe, and effective data protection is provided by Storage Direct Protection for PowerMax, giving users the freedom to choose the ideal backup locations. Customers may choose the cloud vendor that best suits their specific needs and prevent vendor lock-in by using APEX Protection Storage‘s seamless integration with popular cloud providers like AWS, Azure, GCP, and Alibaba.
Model of simplified consumption: Customers pay only for the services they use with Dell APEX Subscriptions, which also simplify billing, invoicing, and capacity utilization tracking for improved forecasting and scalability. This paradigm simplifies lifetime management and offers a contemporary consumption experience without requiring a significant initial capital expenditure.
Innovation in Mainframes
PowerMaxOS 10.2 boosts cyber intrusion detection for mainframes (zCID) with auto-learning access pattern detection, lowers latency and improves IOPS performance for imbalanced mainframe workloads, and uses IBM’s System Recovery Boost to recover more quickly during scheduled or unforeseen outages.
Read more on govindhtech.com
#DellPowerMax#Multicloud#CybersecurityImprovement#Cybersecurity#artificialintelligence#GenAI#Cyberresilience#cyberattacks#news#dell#PowerProtect#APEXProtectionStorage#technology#technews#govindhtech
0 notes
Text
Infographic: 15 Cloud Computing Trends That Will Shape 2024-2029

The next five years in cloud computing will bring remarkable innovations. This infographic covers the top 15 trends, including AI integration and multi-cloud adoption.
Find out how these developments will affect your business.
#CloudComputing#CloudTrends#TechTrends#FutureOfCloud#AIinCloud#HybridCloud#EdgeComputing#QuantumComputing#ServerlessArchitecture#MultiCloud
0 notes
Text
Multi-Cloud Strategy! #Kaara can help you in Embrace flexibility, enhance security, and optimize costs.
Reach our experts by emailing us at [email protected]
Know More:- https://kaaratech.com/cloud-and-Infra.html
0 notes
Text
Six ways hosted private cloud adds value to enterprise business

With companies reclaiming control via cloud repatriation, models that involve private cloud, either as is or in tandem with public cloud, meet the needs of organizations in a cost-effective, agile, and controlled manner, offering immense peace of mind. Read More. https://www.sify.com/cloud/six-ways-hosted-private-cloud-adds-value-to-enterprise-business/
0 notes
Text
InfoQ Article on Fluent Bit with MultiCloud
I’m excited to say that we’ve had an article on Fluent Bit and multi-cloud published on InfoQ. Check it out at https://www.infoq.com/articles/multi-cloud-observability-fluent-bit/ . This is another first for me. As you may have guessed from the title, the article is about how Fluent Bit can support multi-cloud use cases. As part of the introduction, I walked through some of the challenges that…
View On WordPress
0 notes
Text
Critical Applications of Hybrid Cloud & Multi-Cloud in 2024
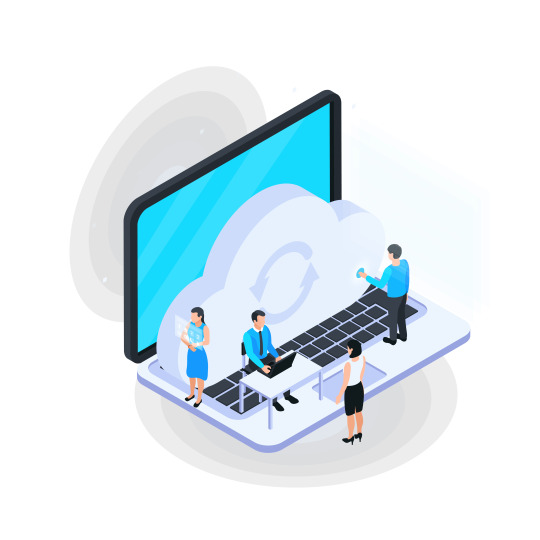
How multi-cloud and hybrid cloud can help you optimize your cloud infrastructure? Go through the blog to know the use cases & differences of these two cloud models, and how to leverage their advantages.
0 notes
Text
Cloud Computing Solutions | Transform Your Business Neo Infoway
Discover the power of cloud computing with our comprehensive solutions. Enhance scalability, security, and efficiency for your business operations. Explore now!
#cloudcomputing#neo#infoway#neoinfoway#cloudservices#cloudinfrastructure#cloudstorage#cloudhosting#publiccloud#privatecloud#hybridcloud#multicloud#clouddeploymentmodels#infrastructureasaservice#platformasaservice#softwareasaservice#cloudmigration#cloudsecurity#cloudarchitecture#cloudmanagement#cloudautomation#cloudscalability
0 notes
Text
Cloud Computing Solutions | Transform Your Business Neo Infoway
Discover the power of cloud computing with our comprehensive solutions. Enhance scalability, security, and efficiency for your business operations. Explore now!
#cloudcomputing#neo#infoway#neoinfoway#cloudservices#cloudinfrastructure#cloudstorage#cloudhosting#publiccloud#privatecloud#hybridcloud#multicloud#clouddeploymentmodels#infrastructureasaservice#platformasaservice#softwareasaservice#cloudmigration#cloudsecurity#cloudarchitecture#cloudmanagement#cloudautomation#cloudscalability
0 notes
Text
BigQuery Omni Cuts Multi-cloud Log Ingestion, Analysis Costs

What is BigQuery Omni?
BigQuery Omni is a multi-cloud data analytics solution that lets you use BigLake tables to perform BigQuery analytics on data kept in Azure Blob Storage or Amazon Simple Storage Service (Amazon S3). It offers a single interface for analyzing data from several public clouds, removing the need to relocate data and allowing you to learn from your data no matter where it is stored.
Many businesses use several public clouds to store their data. Due to the difficulty of gaining insights from all of the data, this data frequently becomes siloed. To evaluate the data, you need a multi-cloud data tool that is quick, affordable, and doesn’t contribute to the costs of decentralized data governance. With a single interface, BigQuery Omni helps us to lower these frictions.
Connecting to Amazon S3 or Blob Storage is a must for performing BigQuery analytics on your external data. You would need to establish a BigLake table that refers data from Blob Storage or Amazon S3 in order to query external data.
Additionally, data can be moved between clouds using cross-cloud transfer, or it can be queried between clouds using cross-cloud joins. One cross-cloud analytics option that BigQuery Omni provides is the freedom to replicate data as needed and the ability to examine data where it resides.
Google BigQuery Omni
Operating hundreds of separate applications across multiple platforms is not unusual in today’s data-centric enterprises. The enormous amount of logs generated by these applications poses a serious problem for log analytics. Furthermore, accuracy and retrieval are made more difficult by the widespread use of multi-cloud solutions, since the dispersed nature of the logs may make it more difficult to derive valuable insights.
In contrast to a traditional strategy, BigQuery Omni was created to help solve this problem and lower overall expenditures. We’ll go over the specifics in this blog post.
Log analysis includes a number of steps, including:
Gathering log data: gathers log data from the applications and/or infrastructure of the enterprise. A popular method for gathering this data is to save it in an object storage program like Google Cloud Storage in JSONL file format. It can be prohibitively expensive to move raw log data between clouds in a multi-cloud setup.
Normalization of log data: Various infrastructures and applications produce distinct JSONL files. The fields in each file are specific to the program or infrastructure that produced it. To make data analysis easier, these disparate fields are combined into a single set, which enables data analysts to do thorough and effective studies throughout the environment.
Indexing and storage: To lower storage and query expenses and improve query performance, normalized data should be stored effectively. Logs are often stored in a compressed columnar file format, such as Parquet.
Querying and visualization: Enable enterprises to run analytics queries to find known threads, abnormalities, or anti-patterns in the log data through querying and visualization.
Data lifecycle: While storage expenses persist, the usefulness of log data declines with age. A data lifecycle procedure must be established in order to maximize costs. Archiving logs after a month (it is rare to query log data older than a month) and deleting them after a year are usual practices. This strategy ensures that crucial data is always available while efficiently controlling storage expenses.
A common Architecture
Many businesses use the following architecture to apply log analysis in a multi-cloud setting:Image credit to Google Cloud
This architecture has advantages and disadvantages.
On the Plus side:
Data lifecycle: By utilizing pre-existing functionality from object storage solutions, data lifecycle management may be implemented really easily. For instance, you can provide the following data lifecycle policy in Cloud Storage: You can use the following policies: (a) delete any item older than a week, which will remove any JSONL files that were available during the Collection process; (b) archive any object older than a month, which will also remove your Parquet files; and (c) delete any object older than a year, which will also remove your Parquet files.
Minimal egress costs: By storing the data locally, you can avoid transmitting large amounts of unprocessed information between cloud providers.
From the negative perspective:
Normalization of log data: You will code and manage an Apache Spark workload for every application with logs you gather. In a time when (a) engineers are in short supply and (b) the use of microservices is expanding quickly, it is wise to steer clear of this.
Querying: You can’t do as much analysis and visualization if you spread your data over several cloud providers.
Querying: Using WHERE clauses to prevent partitions with archived files requires human error and is not a simple solution for excluding archived files created earlier in the data lifetime. Managing the table’s manifest by adding and removing divisions as necessary is one way to work with Iceberg Table. However, it is difficult to play with the Iceberg Table manifest by hand, and using a third-party solution only makes things more expensive.
A better way to address all of these issues would be to use BigQuery Omni, which is shown in the architecture below.
This method’s primary advantage is the removal of several Spark workloads and the need for software engineers to code and maintain them. Having a single product (BigQuery) manage the entire process, aside from storage and visualization, is another advantage of this system. You gain from cost savings as well. Below, we’ll go into more detail about each of these points.
An streamlined procedure for normalizing
The ability of BigQuery to automatically determine the schema of JSONL files and generate an external table pointing to them is a valuable feature. This function is especially helpful when working with multiple log schema formats. The JSONL content of any application can be accessed by defining a simple CREATE TABLE declaration.
Once there, you may program BigQuery to export the JSONL external table into compressed Parquet files in Hive format that are divided into hourly segments. An EXPORT DATA statement that may be programmed to execute once every hour is shown in the query below. This query’s SELECT statement only records the log data that was ingested during the previous hour and transforms it into a Parquet file with columns that have been normalized.
DECLARE hour_ago_rounded_string STRING; DECLARE hour_ago_rounded_timestamp DEFAULT DATETIME_TRUNC(TIMESTAMP_SUB(CURRENT_TIMESTAMP(), INTERVAL 1 HOUR), HOUR);
SET (hour_ago_rounded_string) = ( SELECT AS STRUCT FORMAT_TIMESTAMP(“%Y-%m-%dT%H:00:00Z”, hour_ago_rounded_timestamp, “UTC”) );
EXPORT DATA OPTIONS ( uri = CONCAT(‘[MY_BUCKET_FOR_PARQUET_FILES]/ingested_date=’, hour_ago_rounded_string, ‘/logs-*.parquet’), format = ‘PARQUET’, compression = ‘GZIP’, overwrite = true) AS ( SELECT [MY_NORMILIZED_FIELDS] EXCEPT(ingested_date) FROM [MY_JSONL_EXTERNAL_TABLE] as jsonl_table WHERE DATETIME_TRUNC(jsonl_table.timestamp, HOUR) = hour_ago_rounded_timestamp );
A uniform querying procedure for all cloud service providers
While using the same data warehouse platform across several cloud providers already improves querying, BigQuery Omni’s ability to perform cross-cloud joins is revolutionary for Log Analytics. Combining log data from many cloud providers was difficult prior to BigQuery Omni. Sending the raw data to a single master cloud provider results in large egress expenses due to the volume of data; yet, pre-processing and filtering it limits your capacity to do analytics on it. Cross-cloud joins allow you to execute a single query across several clouds and examine the outcomes.
Reduces TCO
This architecture’s ability to lower total cost of ownership (TCO) is its last and most significant advantage. There are 3 ways to measure this:
Decreased engineering resources: Apache Spark is eliminated from this procedure for two reasons. The first is that Spark code can be worked on and maintained without a software developer. By employing standard SQL queries, the log analytics team can complete the deployment process more quickly. The shared responsibility concept of BigQuery and BigQuery Omni, which are PaaS, is extended to data in AWS and Azure.
Lower compute resources: The most economical environment might not always be provided by Apache Spark. The application itself, the Apache Spark platform, and the virtual machine (VM) make up an Apache Spark solution. In comparison to Apache Spark, BigQuery uses slots (virtual CPUs, not virtual machines) and an export query that is transformed into C-compiled code during the export process can lead to quicker performance for this particular operation.
Lower egress expenses: BigQuery Omni eliminates the need to transfer raw data between cloud providers in order to get a consolidated view of the data by processing data in-situ and egressing only results through cross-cloud joins.
What is the best way to use BigQuery in this setting?
BigQuery has two compute pricing models for query execution:
On-demand pricing (per TiB): This pricing model charges you according to the quantity of bytes each query processes, but it does not charge you for the first 1 TiB of query data handled each month. Using this technique is not advised because log analytics tasks use a lot of data.
Capacity pricing (per slot-hour): Under this pricing model, you are billed for the amount of computing power that is utilized to execute queries over time, expressed in slots (virtual CPUs). This model utilizes editions of BigQuery. Slot commitments, which are dedicated capacity always available for your workloads, are less expensive than on-demand, and you can use the BigQuery autoscaler.
In order to conduct an empirical test, Google assigned 100 slots (baseline 0, maximum slots 100) to a project that aimed to export log JSONL data into a compressed Parquet format. This configuration allowed BigQuery to process 1PB of data daily without using up all 100 slots.
In order to enable the TCO reduction of Log Analytics workloads in a multi-cloud context, it proposed an architecture in this blog post that substitutes SQL queries running on BigQuery Omni for Apache Spark applications. Your particular data environment may benefit from this method’s ability to minimize overall DevOps complexity while lowering engineering, computation, and egress expenses.
BigQuery Omni pricing
Please refer to BigQuery Omni pricing for details on price and time-limited promotions.
Read more on Govindhtech.com
#BigQuery#BigQueryOmni#Multicloud#BigQueryOmniAzure#AzureBlobStorage#AmazonS3#BigQueryanalytics#IcebergTable#News#Technews#Technology#Technologynews#Technologytrends#govindhtech
0 notes
Text
Virtualization in Cloud Computing . . . . for more information and a cloud computing tutorial https://bit.ly/4a9ymrG check the above link
#cloudcomputing#privatecloud#publiccloud#hybridcloud#virtualization#multicloud#communitycloud#computerscience#computerengineering#javatpoint
0 notes
Text

#AWS#CloudEngineer#CloudComputing#AWSCertified#InfrastructureAsCode#DevOps#Serverless#Kubernetes#MultiCloud#CloudArchitecture#Automation#BigData#Security#Networking#SolutionsArchitect#ProfessionalDevelopment#NewBatch#CareerGrowth#TechEducation#uniquesystemskills#training#freshers#ITjobs#career#upskilling#skills#Job#pune#pcmc#kothrud
0 notes