#ImproveAI
Explore tagged Tumblr posts
Text
Top 5 Fine Tuning LLM Techniques & Inference To Improve AI
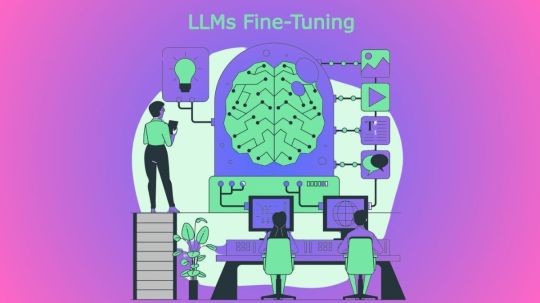
Fine Tuning LLM Techniques
The Top 5 Fine Tuning LLM Techniques and Inference Tricks to Boost Your AI Proficiency. With LLM inference and fine-tuning, your generative AI (GenAI) systems will perform even better.
The foundation of GenAI is LLMs, which allow us to create strong, cutting-edge applications. But like any cutting-edge technology, there are obstacles to overcome before they can be fully used. It may be difficult to install and fine-tune these models for inference. You may overcome these obstacles with the help of these five recommendations from this article.
Prepare Your Data Carefully
The performance of the model is largely dependent on efficient data preparation. Having a clean and well-labeled dataset may greatly improve training results. Noisy data, unbalanced classes, task-specific formatting, and nonstandard datatypes are among the difficulties.
Tips
The columns and structure of your dataset will depend on whether you want to train and fine-tune for teaching, conversation, or open-ended text creation.
Generate fake data from a much bigger LLM to supplement your data. To create data for fine-tuning a smaller 1B parameter model, for instance, utilize a 70B parameter model.
” This still holds true for language models, and it may significantly affect your models’ quality and hallucination. Try assessing 10% of your data by hand at random.
Adjust Hyperparameters Methodically
Optimizing hyperparameters is essential to attaining peak performance. Because of the large search space, choosing the appropriate learning rate, batch size, and number of epochs may be challenging. It’s difficult to automate this using LLMs, and optimizing it usually involves having access to two or more accelerators.
Tips
Utilize random or grid search techniques to investigate the hyperparameter space.
Create a bespoke benchmark for distinct LLM tasks by synthesizing or manually constructing a smaller group of data based on your dataset. As an alternative, make use of common benchmarks from harnesses for language modeling such as EleutherAI Language Model Evaluation Harness.
To prevent either overfitting or underfitting, pay strict attention to training data. Look for circumstances in which your validation loss rises while your training loss stays constant this is a blatant indication of overfitting.
LLM Fine tuning Methods
Employ Cutting-Edge Methods
Training time and memory may be greatly decreased by using sophisticated methods like parameter-efficient fine-tuning (PEFT), distributed training, and mixed precision. The research and production teams working on GenAI applications find these strategies useful and use them.
Tips
For accuracy to be maintained across mixed and non-mixed precision model training sessions, verify your model’s performance on a regular basis.
To make implementation simpler, use libraries that enable mixed precision natively. Above all, PyTorch allows for automated mixed precision with little modifications to the training code.
Model sharding is a more sophisticated and resource-efficient approach than conventional distributed parallel data approaches. It divides the data and the model across many processors. Software alternatives that are popular include Microsoft DeepSpeed ZeRO and PyTorch Fully Sharded Data Parallel (FSDP).
Low-rank adaptations (LoRA), one of the PEFT approaches, let you build “mini-models” or adapters for different tasks and domains. Additionally, LoRA lowers the overall number of trainable parameters, which lowers the fine-tuning process’s memory and computational cost. By effectively deploying these adapters, you may handle a multitude of use scenarios without requiring several huge model files.
Aim for Inference Speed Optimization
Minimizing inference latency is essential for successfully deploying LLMs, but it may be difficult because of their complexity and scale. The user experience and system latency are most directly impacted by this component of AI
Tips
To compress models to 16-bit and 8-bit representations, use methods such as low-bit quantization.
As you try quantization recipes with lower precisions, be sure to periodically assess the model’s performance to ensure accuracy is maintained.
To lessen the computational burden, remove unnecessary weights using pruning procedures.
To build a quicker, smaller model that closely resembles the original, think about model distillation.
Large-Scale Implementation with Sturdy Infrastructure
Maintaining low latency, fault tolerance, and load balancing are some of the issues associated with large-scale LLM deployment. Setting up infrastructure effectively is essential.
Tips
To build consistent LLM inference environment deployments, use Docker software. The management of dependencies and settings across several deployment phases is facilitated by this.
Utilize AI and machine learning tools like Ray or container management systems like Kubernetes to coordinate the deployment of many model instances within a data center cluster.
When language models get unusually high or low request volumes, use autoscaling to manage fluctuating loads and preserve performance during peak demand. In addition to ensuring that the deployment appropriately satisfies the application’s business needs, this may assist reduce money.
While fine-tuning and implementing LLMs may seem like difficult tasks, you may overcome any obstacles by using the appropriate techniques. Overcoming typical mistakes may be greatly aided by the advice and techniques shown above.
Hugging Face fine-tuning LLM
Library of Resources
For aspiring and experienced AI engineers, it provide carefully crafted and written material on LLM fine-tuning and inference in this area. They go over methods and tools such as Hugging Face for the Optimum for Intel Gaudi library, distributed training, LoRA fine-tuning of Llama 7B, and more.
What you will discover
Apply LoRA PEFT to cutting-edge models.
Find ways to train and execute inference with LLMs using Hugging Face tools.
Seek to use distributed training methods, such as PyTorch FSDP, to expedite the process of training models.
On the Intel Tiber Developer Cloud, configure an Intel Gaudi processor node.
Read more on govindhtech.com
#Top5#ImproveAI#TuningLLMTechniques#IntelGaudi#generativeAI#languagemodels#GenAIapplications#machinelearning#IntelTiberDeveloperCloud#SturdyInfrastructure#DataCarefully#technology#technews#news#govindhtech
0 notes
Text
Oracle Roving Edge Device(RED) Utilizing Intel To Improve AI
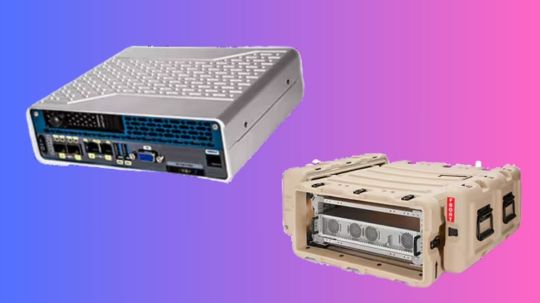
Boosting AI at the Periphery: Oracle Roving Edge Device(RED) Using Intel
Oracle Roving Edge Device
In the rapidly evolving digital terrain of today, enterprises are progressively shifting away from centralized public clouds and toward distributed cloud models that include hybrid, multi-cloud, and edge solutions. This change satisfies crucial requirements for data residency, latency, and security in addition to providing flexibility and scalability.
- Advertisement -
With 78 locations worldwide, Oracle Cloud Infrastructure (OCI), the cloud provider with the quickest pace of growth, is making a major advancement with the release of the second iteration of the Oracle Roving Edge Device. With this most recent addition, Oracle’s portfolio of distributed clouds now offers remarkable processing power, cutting-edge connectivity, and improved security right to the edge even in situations that are unconnected.
The Oracle Roving Edge Device(RED) second version streamlines deployment while offering the cost-efficiencies of cloud technology by enabling enterprises to operate corporate apps, AI models, and specific OCI services directly at the edge. This changes how firms may use edge computing and makes it a vital tool for sectors needing instantaneous, local data processing with strict security constraints.
What Is Special About Oracle Roving Edge Device?
The Oracle Roving Edge Device was first designed to satisfy the tactical requirements of the US Department of Defense, but it has since expanded to fulfill corporate needs in a variety of industries. Building upon this solid basis, Oracle Roving Edge Device has three configurable configurations, Base and Storage-Optimized versions with Intel CPUs among them:
Base Configuration: This version is perfect for a variety of applications needing strong processing since it is powered by an Intel Xeon 8480+ processor (56 cores) and 512GB DDR5 memory.
- Advertisement -
Storage Optimized: With eight 15.38TB NVMe SSDs, this configuration is ideal for data-intensive applications that need large amounts of storage and quick processing.
Using the Llama2-7B model, the Base second generation RED configuration with 4th gen Intel Xeon 8480+ may provide up to 13.6x reduced latency than the original Oracle Roving Edge Device with Intel Xeon 6230T.
The Use of Strategic Edges
RED is a commercial enabler as well as a technical breakthrough. Whether using it at the edge or in a conventional data center, enterprises may deploy the same OCI services, development processes, and CPU/GPU capabilities. Because of this flexibility, businesses in sectors including industrial, government, telecom, retail, and AI/ML may use mission-critical apps, secure networking, real-time analytics, and AI/ML anywhere, even in remote places.
For instance, RED may provide real-time information in crucial contexts like military or healthcare, or it might improve operational efficiency via predictive maintenance in distant industrial locations.
Why It Is Worth Considering
For companies that want local data processing with the dependability and affordability of the cloud, Oracle RED, which is equipped with Intel processors, provides a safe, scalable, and high-performance platform. RED offers an unmatched solution if your company has workloads that are mission-critical and need edge deployment in real-time.
With the speed, security, and flexibility of the second-generation Oracle Roving Edge Device, you can take your operations to the next level and empower your organization with Intel and Oracle.
Cutting-Edge Infrastructure
Roving Edge Infrastructure from Oracle Cloud Infrastructure (OCI) speeds up the deployment of cloud workloads outside of data centers. Ruggedized devices provide quicker processing near the data source and faster insights into the data by delivering cloud computing and storage services at the edge of networks and in remote areas.
Using the same portal and tenancy tools as oracle public regions, existing OCI compute images and object storage may be synced to Oracle Roving Edge device.
Examine Oracle’s Cutting-Edge Infrastructure
Cloud functionality in isolated settings
Expand the use of current cloud environments: Customers in the public and private sectors are able to implement OCI services outside of OCI Dedicated Regions and Oracle Public Cloud regions.
Utilize apps at the edge of the network: Rather of depending on distant services that need several network hops, field operations teams may get very low latency locally for cloud applications that are sensitive to delays.
Execute disjointed tasks: Even when fully unconnected, faraway sites may benefit from Oracle Cloud Infrastructure capabilities thanks to small, portable server nodes.
Use cases for Oracle Roving Edge Infrastructure
Quick field data collecting and processing: Utilize the potent computational capabilities of Roving Edge Infrastructure devices to absorb and handle massive volumes of streaming data from sensors situated in far-off places.
Deploying applications to distant locations: Facilitate the smooth implementation of applications for establishments including embassies and consulates, government offices, military outposts, and distant educational institutions.
Create, test, and deploy at the edge of the cloud: Create, implement, and manage all cloud-based apps and data, extending their reach to the edge as required, all managed via a unified pane of glass.
ML and AI on the periphery: For quicker processing of AI and ML applications, attach VPU/TPU accelerators or use built-in GPUs to avoid depending on network access to Oracle Cloud Infrastructure.
Read more on govindhtech.com
#OracleRovingEdgeDevice#UtilizingIntel#ImproveAI#publicclouds#cloudtechnology#IntelCPU#ai#ml#InteXeon8480#Llama2#cloudcomputing#gpu#cpu#OracleRED#OraclePublicCloud#technology#technews#news#govindhtech
1 note
·
View note