#Gyrus AI Model
Explore tagged Tumblr posts
Text
#gyrus#gyrus ai#video anonymization#ai video anonymization software#Gyrus AI Technology#Gyrus AI Model#Future of Video Retrieval
0 notes
Text
Study: AI could help triage brain MRIs
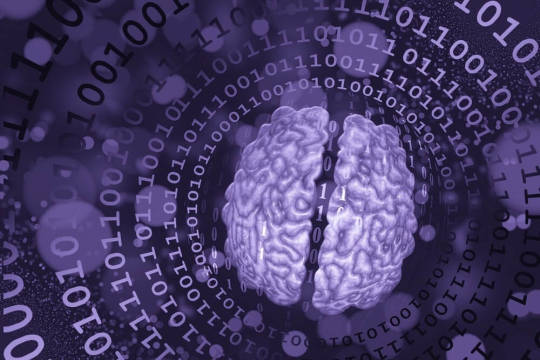
- By Radiological Society of North America -
An artificial intelligence (AI)-driven system that automatically combs through brain MRIs for abnormalities could speed care to those who need it most, according to a study in Radiology: Artificial Intelligence.
“There are an increasing number of MRIs that are performed, not only in the hospital but also for outpatients, so there is a real need to improve radiology workflow,” said study co-lead author Romane Gauriau, PhD, former machine learning scientist at Massachusetts General Hospital and Brigham and Women’s Hospital Center for Clinical Data Science in Boston. “One way of doing that is to automate some of the process and also help the radiologist prioritize the different exams.”
Dr. Gauriau, along with co-lead author Bernardo C. Bizzo, MD, PhD, and colleagues, and in partnership with Diagnosticos da America SA (DASA), a medical diagnostics company in Brazil, developed an automated system for classifying brain MRI scans as either “likely normal” or “likely abnormal.” The approach relies on a convolutional neural network (CNN).
The researchers trained and validated the algorithm on three large datasets totaling more than 9,000 examinations collected from different institutions on two different continents.
Study Demonstrates Ability to Differentiate Normal Vs. Abnormal Exams
In preliminary testing, the model showed relatively good performance to differentiate likely normal or likely abnormal examinations. Testing on a validation dataset acquired at a different time period and from a different institution than the data used to train the algorithm highlighted the generalization capacity of the model. Such a system could be used as a triage tool, according to Dr. Gauriau, with the potential to improve radiology workflow.
Image: Examples of axial FLAIR sequences from studies within dataset A. From left to right: a patient with a 'likely normal' brain; a patient presenting an intraparenchymal hemorrhage within the right temporal lobe; a patient presenting an acute infarct of the inferior division of the right middle cerebral artery; and a patient with known neurocysticercosis presenting a rounded cystic lesion in the left middle frontal gyrus. Credit: Radiological Society of North America.
“The problem we are trying to tackle is very, very complex because there are a huge variety of abnormalities on MRI,” she said. “We showed that this model is promising enough to start evaluating if it can be used in a clinical environment.”
Similar models have been shown to significantly improve turnaround time for the identification of abnormalities in head CTs and chest X-rays. The new model has the potential to further benefit outpatient care by identifying incidental findings.
“Say you fell and hit your head, then went to the hospital and they ordered a brain MRI,” Dr. Gauriau said. “This algorithm could detect if you have brain injury from the fall, but it may also detect an unexpected finding such as a brain tumor. Having that ability could really help improve patient care.”
The work was the first of its kind to leverage a large and clinically relevant dataset and use full volume MRI data to detect overall brain abnormality. The next steps in the research include evaluating the model’s clinical utility and potential value for radiologists. Researchers would also like to develop it beyond the binary outputs of “likely normal” or “likely abnormal.”
“This way we could not only have binary results but maybe something to better characterize the types of findings, for instance, if the abnormality is more likely to be related to tumor or to inflammation,” Dr. Gauriau said. “It could also be very useful for educational purposes.”
Further evaluation is currently ongoing in a controlled clinical environment in Brazil with the research collaborators from DASA.
--
Source: Radiological Society of North America
Full study: “A Deep Learning-Based Model for Detecting Abnormalities on Brain MRI for Triaging: Preliminary Results from a Multi-Site Experience”, Radiology: Artificial Intelligence.
https://doi.org/10.1148/ryai.2021200184
Read Also
Detecting injury and disease via magnetic brain waves
#mri#brain#triage#diagnostics#neuroscience#imaging#radiology#medicine#ai#artificial intelligence#health#health tech#medtech
0 notes
Text
Neuroscience of Empathy
Analysis
Empathy in General:
Cognitive empathy is the ability to understand how a person feels and what they might be thinking. Cognitive empathy makes us better communicators, because it helps us relay information in a way that best reaches the other person.
Emotional empathy (also known as affective empathy) is the ability to share the feelings of another person. Some have described it as "your pain in my heart." This type of empathy helps you build emotional connections with others.
Compassionate empathy (also known as empathic concern) goes beyond simply understanding others and sharing their feelings: it actually moves us to take action, to help however we can.
Resource: https://www.inc.com/justin-bariso/there-are-actually-3-types-of-empathy-heres-how-they-differ-and-how-you-can-develop-them-all.html
Empathy is a crucial component of human emotional experience and social interaction. The ability to share the affective states of our closest ones and complete strangers allows us to predict and understand their feelings, motivations and actions.
Empathy occurs when the observation or imagination of affective states in another induces shared states in the observer. This state is also associated with knowledge that the target is the source of the affective state in the self.
The discovery of mirror neurons, a class of neurons in monkey premotor and parietal cortices activated during execution and observation of actions, provided a neural mechanism for shared representations in the domain of action understanding, creating what is known as mimicry.
Mimicry: the tendency to synchronize the affective expressions, vocalizations, postures, and movements of another person
Resource: The Neural Basis of Empathy Boris C. Bernhardt and Tania Singer Annual Review of Neuroscience 2012 35:1, 1-23
Brain imaging studies that have investigated the neural underpinnings of human empathy. Using mainly functional magnetic resonance imaging (fMRI), the majority of studies suggest that observing affective states in others activates brain networks also involved in the firsthand experience of these states, confirming the notion that empathy is, in part, based on shared networks In particular, anterior insular (AI) and dorsal-anterior/anterior- mid cingulate cortex (dACC/aMCC) play central roles in vicarious responses in the domain of disgust, pleasant or unpleasant tastes, physical and emotional pain and other social emotions such as embarrassment or admiration
Resource: The Neural Basis of Empathy Boris C. Bernhardt and Tania Singer Annual Review of Neuroscience 2012 35:1, 1-23
https://www.ncbi.nlm.nih.gov/pmc/articles/PMC3021497/
Amygdala: a region of the brain that scientists associate with emotional learning and fear conditioning; is important to evaluation and preference development. responsible for the fight, flight or freeze emotional response to stimuli.
What is looks like in Children vs Adults:
Empathic emotional response in the young child may be stronger, whereas sympathetic behavior may be less differentiated. With age and increased maturation of the mPFC, dlPFC and vmPFC, in conjunction with input from interpersonal experiences that are strongly modulated by various contextual and social factors such as ingroup versus outgroup processes, children and adolescents become sensitive to social norms regulating prosocial behavior and, accordingly, may become more selective in their response to others.
A comprehensive social neuroscience theory of empathy requires the specification of various causal mechanisms producing some outcome variable (e.g. helping behavior), the moderator variables (e.g. implicit attitudes, ingroup/outgroup processes) that influence the conditions under which each of these mechanisms operate, and the unique consequences resulting from each of them.
Empathy typically emerges as the child comes to a greater awareness of the experience of others, during the second and third years of life, and arises in the context of a social interaction.
By the age of 12 months, infants begin to comfort victims of distress, and by 14–18 months, children display spontaneous helping behaviors.
Affective responsiveness is known to be present at an early age, is involuntary, and relies on mimicry and somatosensorimotor resonance between other and self. For instance, newborns and infants become vigorously distressed shortly after another infant begins to cry.
A significant negative correlation between age and degree of activation was found in the posterior insula. In contrast, a positive correlation was found in the anterior portion of the insula. This posterior-to-anterior progression of increasingly complex re-representations in the human insula is thought to provide a foundation for the sequential integration of the individual homeostatic condition with one's sensory environment and motivational condition.
Posterior Insula: receives inputs from the ventromedial nucleus of the thalamus, which is highly specialized for conveying emotional and homeostatic information such as pain, temperature, hunger, thirst, itch and cardiorespiratory activity posterior part has been shown to be associated with interoception due to connections with the amygdala, hypothalamus, ACC and OFC
Right Anterior Insula serves to compute a higher-order metarepresentation of the primary interoceptive activity, which is related to the feeling of pain and its emotional awareness
Regulatory mechanisms continue into late adolescence and early adulthood, greater signal change with increasing age was found in the prefrontal regions involved in cognitive control and response inhibition, such as the dlPFC and inferior frontal gyrus. Overall, this pattern of age-related change in the amygdala, insula and PFC can be interpreted in terms of the frontalization of inhibitory capacity, hypothesized to provide a greater top-down modulation of activity within more primitive emotion-processing regions. Another important age-related change was detected in the vmPFC/OFC: activation in the OFC in response to pain inflicted by another shifted from its medial portion in young participants to the lateral portion in older participants.
Cultural Acceptance: (science of bias)
Implicit (unconscious) bias: A bias in behavior and/or judgement that results from subtle cognitive processes and occurs on a level below a person’s conscious awareness, without intentional or conscious control. Based in the subconscious and can be developed overtime due to a natural accumulation of personal experiences.
Amygdala: a region of the brain that scientists associate with emotional learning and fear conditioning; is important to evaluation and preference development. responsible for the fight, flight or freeze emotional response to stimuli.
Implicit, or unconscious bias, as defined above, is a subtle cognitive process that starts in the amygdala. This type of bias can be understood as a “form of rapid ‘social categorization,’ whereby we routinely and rapidly sort people into groups.” 2 Dr. Brainard (MS, MA, PhD), of the Brainard Strategy, highlights the parts of the brain that create bias: Amygdala, Hippocampus, Temporal lobe Media frontal cortex
Resources:
https://www.spectradiversity.com/2017/12/27/unconscious-bias/
https://books.google.com/books?hl=en&lr=&id=JA9nDAAAQBAJ&oi=fnd&pg=PA163&dq=social+neuroscience+approach+cognitive+bias&ots=UXxRc6_yn1&sig=jDpspsU9ge782p_llwa6K9wvI8o#v=onepage&q=social%20neuroscience%20approach%20cognitive%20bias&f=false
Results:
A study published in the Journal of Neuroscience on October 9, 2013, Max Planck researchers identified that the tendency to be egocentric is innate for human beings – but that a part of your brain recognizes a lack of empathy and autocorrects. This specific part of your brain is called the the right supramarginal gyrus. When this brain region doesn't function properly—or when we have to make particularly quick decisions—the researchers found one’s ability for empathy is dramatically reduced.
Until now, social neuroscience models have assumed that people simply rely on their own emotions as a reference for empathy. This only works, however, if we are in a neutral state or the same state as our counterpart. Otherwise, the brain must use the right supramarginal gyrus to counteract and correct a tendency for self-centered perceptions of another’s pain, suffering or discomfort.
Resources:
https://www.psychologytoday.com/us/blog/the-athletes-way/201310/the-neuroscience-empathy
https://www.mpg.de/research/supramarginal-gyrus-empathy
Contrary Points of View:
Yale University professor of psychology and cognitive science Paul Bloom thinks a lot gray resides in such a black-and-white definition, and that there is more danger than good adopting such a simplistic view of empathy. At the heart of his article lies an important distinction between emotional versus cognitive empathy. Briefly, the former is literally feeling what another is experiencing, while the latter implies sympathizing with the other—understanding what’s going on without necessarily going through the same emotional whirlwind or “empathetic distress”.
Paul Bloom, Against Empathy http://bostonreview.net/forum/paul-bloom-against-empathy
Derek Beres, The Case Against Empathy https://bigthink.com/21st-century-spirituality/the-case-against-empathy-2
0 notes
Text
Face-specific brain area responds to faces even in people born blind
More than 20 years ago, neuroscientist Nancy Kanwisher and others discovered that a small section of the brain located near the base of the skull responds much more strongly to faces than to other objects we see. This area, known as the fusiform face area, is believed to be specialized for identifying faces.
Now, in a surprising new finding, Kanwisher and her colleagues have shown that this same region also becomes active in people who have been blind since birth, when they touch a three-dimensional model of a face with their hands. The finding suggests that this area does not require visual experience to develop a preference for faces.
“That doesn’t mean that visual input doesn’t play a role in sighted subjects — it probably does,” she says. “What we showed here is that visual input is not necessary to develop this particular patch, in the same location, with the same selectivity for faces. That was pretty astonishing.”
Kanwisher, the Walter A. Rosenblith Professor of Cognitive Neuroscience and a member of MIT’s McGovern Institute for Brain Research, is the senior author of the study. N. Apurva Ratan Murty, an MIT postdoc, is the lead author of the study, which appears this week in the Proceedings of the National Academy of Sciences. Other authors of the paper include Santani Teng, a former MIT postdoc; Aude Oliva, a senior research scientist, co-director of the MIT Quest for Intelligence, and MIT director of the MIT-IBM Watson AI Lab; and David Beeler and Anna Mynick, both former lab technicians.
Selective for faces
Studying people who were born blind allowed the researchers to tackle longstanding questions regarding how specialization arises in the brain. In this case, they were specifically investigating face perception, but the same unanswered questions apply to many other aspects of human cognition, Kanwisher says.
“This is part of a broader question that scientists and philosophers have been asking themselves for hundreds of years, about where the structure of the mind and brain comes from,” she says. “To what extent are we products of experience, and to what extent do we have built-in structure? This is a version of that question asking about the particular role of visual experience in constructing the face area.”
The new work builds on a 2017 study from researchers in Belgium. In that study, congenitally blind subjects were scanned with functional magnetic resonance imaging (fMRI) as they listened to a variety of sounds, some related to faces (such as laughing or chewing), and others not. That study found higher responses in the vicinity of the FFA to face-related sounds than to sounds such as a ball bouncing or hands clapping.
In the new study, the MIT team wanted to use tactile experience to measure more directly how the brains of blind people respond to faces. They created a ring of 3D-printed objects that included faces, hands, chairs, and mazes, and rotated them so that the subject could handle each one while in the fMRI scanner.
They began with normally sighted subjects and found that when they handled the 3D objects, a small area that corresponded to the location of the FFA was preferentially active when the subjects touched the faces, compared to when they touched other objects. This activity, which was weaker than the signal produced when sighted subjects looked at faces, was not surprising to see, Kanwisher says.
“We know that people engage in visual imagery, and we know from prior studies that visual imagery can activate the FFA. So the fact that you see the response with touch in a sighted person is not shocking because they’re visually imagining what they’re feeling,” she says.
The researchers then performed the same experiments, using tactile input only, with 15 subjects who reported being blind since birth. To their surprise, they found that the brain showed face-specific activity in the same area as the sighted subjects, at levels similar to when sighted people handled the 3D-printed faces.
“When we saw it in the first few subjects, it was really shocking, because no one had seen individual face-specific activations in the fusiform gyrus in blind subjects previously,” Murty says.
Patterns of connection
The researchers also explored several hypotheses that have been put forward to explain why face-selectivity always seems to develop in the same region of the brain. One prominent hypothesis suggests that the FFA develops face-selectivity because it receives visual input from the fovea (the center of the retina), and we tend to focus on faces at the center of our visual field. However, since this region developed in blind people with no foveal input, the new findings do not support this idea.
Another hypothesis is that the FFA has a natural preference for curved shapes. To test that idea, the researchers performed another set of experiments in which they asked the blind subjects to handle a variety of 3D-printed shapes, including cubes, spheres, and eggs. They found that the FFA did not show any preference for the curved objects over the cube-shaped objects.
The researchers did find evidence for a third hypothesis, which is that face selectivity arises in the FFA because of its connections to other parts of the brain. They were able to measure the FFA’s “connectivity fingerprint” — a measure of the correlation between activity in the FFA and activity in other parts of the brain — in both blind and sighted subjects.
They then used the data from each group to train a computer model to predict the exact location of the brain’s selective response to faces based on the FFA connectivity fingerprint. They found that when the model was trained on data from sighted patients, it could accurately predict the results in blind subjects, and vice versa. They also found evidence that connections to the frontal and parietal lobes of the brain, which are involved in high-level processing of sensory information, may be the most important in determining the role of the FFA.
“It’s suggestive of this very interesting story that the brain wires itself up in development not just by taking perceptual information and doing statistics on the input and allocating patches of brain, according to some kind of broadly agnostic statistical procedure,” Kanwisher says. “Rather, there are endogenous constraints in the brain present at birth, in this case, in the form of connections to higher-level brain regions, and these connections are perhaps playing a causal role in its development.”
The research was funded by the National Institutes of Health Shared Instrumentation Grant to the Athinoula Martinos Center at MIT, a National Eye Institute Training Grant, the Smith-Kettlewell Eye Research Institute’s Rehabilitation Engineering Research Center, an Office of Naval Research Vannevar Bush Faculty Fellowship, an NIH Pioneer Award, and a National Science Foundation Science and Technology Center Grant.
Face-specific brain area responds to faces even in people born blind syndicated from https://osmowaterfilters.blogspot.com/
0 notes
Video
Fiction: Lost Memories, p10 [See previous posts] . “So Mr. President we’re going to test your spatial awareness, pattern recognition, and reflexes. All you have to do is play a simple video game.” . The President rubbed his hands together, “I used to play my sons’ Tank Command video game. Man that was fun. I could always trap and destroy them—they were helpless!” . Earlier, I explained to McKennedy how an fMRI machine tracks neuronal activity, measuring even tiny blood flow changes in the brain. The key is looking in the President’s angular gyrus (ANG) and the occipitotemporal cortex (OCT). The ANG is where we’ll find processes for vivid memories and spatial awareness among other goodies. . We’ll train an AI (artificial intelligence) program how he processes visual cues. The AI builds a mathematical model from data. Based on the President’s responses, the AI will present a very specific series of cues. These will translate the brain activity to outlines of real-world, real-time models on-screen. . “Some friends at Berkeley developed ‘neural dust’ that is as thin as a human hair. They’re powered by ultrasonic pulses that we’ll beam at the President. Minute electronics in the ‘dust’ will transmit the brain data out to the AI. We used to have to have to do this by implanting electrodes, but we’ve found less invasive ways to penetrate the blood brain barrier. . Cues hidden among the images related to the Russian leader. Mid-session an outline of a building started to form on the computer screen. It didn’t mean anything to me, but McKennedy looked shocked. He was shaking his head. . [Music: ”Benzodiazepine” provided by Splice App Music Library] https://www.instagram.com/p/Bt14ZMqFiTm/?utm_source=ig_tumblr_share&igshid=vmyqkk4e0t79
0 notes
Text
#gyrus ai#gyrus#anonymization software#video anonymization#AI Video Anonymization Software#Advanced AI and ML Technologies#AI Model Techniques#anonymize PII software#AI-powered video anonymization model
0 notes
Text
The need for efficient search and retrieval of relevant video content has become increasingly important in the fast-paced digital media landscape. In the older scenario, manual tagging and generating metadata were considered the hallmark of any retrieval method. However, this fails to capture the nuanced semantics of a video and its meaning. Embeddings have made a revolutionary technology that allows machines to understand and index video content indexing based on its intrinsic meaning.
Understanding Embeddings in the Context of Video.

Embeddings are continuous vector representations that encapsulate the semantic concept of data, be it text, images, audio, or video. In the video context, embeddings are created by feeding visual frames, audio signals, and textual elements (such as subtitles) to deep learning models in some fashion. Such a process changes complex, high-dimensional data into an ordered format that can be readily analyzed and compared by machines.

Conclusion:
In the scope of applying data science techniques to video content, Embeddings offer a series of solutions ranging from simple keyword search to powerful semantically oriented retrieval systems. By embedding advanced concepts, multimedia videos can be indexed and queried semantically for far more meaningful and efficient access.
#gyrus ai#gyrus#ai in mam#intelligent media search#media asset management#media search#advanced media search#ai media search#ai video anonymization software#Video Content Indexing#AI Media Discovery#Digital Media Asset Management
0 notes
Text
Why Media Houses Prefer On-Premise Solutions?
Intelligent Media Search organizations deal with a large amount of highly sensitive content, including unreleased footage, undisclosed interviews, and proprietary research materials. Keeping such data within the organization and processing it on-premises reduces the risk of exposure to breaches or unauthorized access outside the organization. Moreover, these very well comply with applicable regulations and put all those who worry about data sovereignty at ease.
Media houses are also cautious about using prompts or APIs connected to public large language models (LLMs), as these could potentially expose confidential data. While cloud solutions are evolving, getting media houses to fully embrace public cloud networks will take time due to their strong comfort with existing on-premise systems.

#gyrus ai#gyrus#ai in mam#intelligent media search#media asset management#AI Media Search#media search#Semantic video search#Media Discovery#Video content indexing#Contextual video search#advanced media search
0 notes
Text
#gyrus#gyrus ai#video anonymization#anonymization software#ai video anonymization software#ai in mam#redaction tool#AI Vision Solutions#Media Asset Management
0 notes
Text
The Need for AI in Media Asset management - Gyrus AI
GYRUS AI provides custom AI models for Media Asset Management Search (MAMS). Built for your use case, be it as a broadcaster, advertiser, or media creator, our solutions are tailored to achieve what you require.
More Information About - The Role of AI-Enabled Media Asset Management in Efficient Content Handling
To learn more about how our AI technologies can help your video content perform better, you may contact us at [email protected] or simply click here: www.gyrus.ai
#video anonymization#anonymization software#gyrus ai#redaction software#gyrus#AI in MAM#AI enabled Media Asset Management#Broadcasting Solutions#AI Vision Analytics#AI Video Anonymization Software
0 notes
Text
#gyrus ai#gyrus#video anonymization#anonymization software#AI in MAM#AI-Enabled Media Asset Management#AI Vision Streaming Analytics#AI Video Anonymization#Gyrus AI Video Streaming Software
0 notes
Text
#anonymization software#video anonymization#redaction software#redaction tool#gyrus ai#gyrus#neural network accelerator#anonymization#data protection#neural networks
0 notes
Text
#video anonymization#anonymization software#redaction software#redaction tool#anonymization#Video Anonymization AI#Gyrus#Gyrus AI Blogs#Gyrus AI
0 notes
Video
youtube
Gyrus AI uses an #AI powered #data #analytics model that helps the #industrial #manufacturing sector in reducing downtime, better design, improve efficiency, increase productivity, better product quality, and the safety of employees. #iot #code #tech #IIoT #MachineLearning
#industry#manufacturingsolution#manufacturing#AI#artificialintelligence#analytics#gyrusai#machinelearning#data#iot#code#videoanalytics#technology#solution#viral
1 note
·
View note
Photo
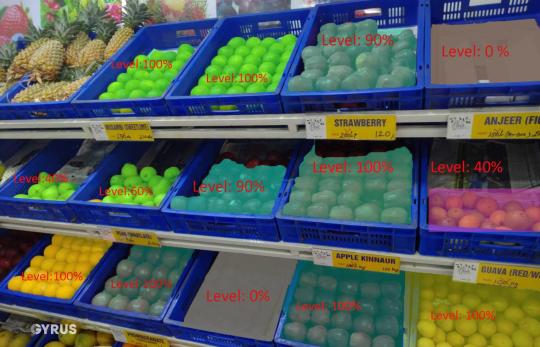
Gyrus AI’s video analytics-based inventory management model.This model can be implemented on a live feed from the surveillance camera's and results can be integrated with Point of Sale for real-time updates on the stock. #smartretail #inventory #videoanalytics
#retail#retail technology#inventory#inventory control#inventory management#video analytics#analytics#data#smartretail
1 note
·
View note
Text
Reduce cost and Monetize Video Streaming with AI/ML
The massive amount of data streaming presents challenges to broadcasters, content distribution networks, such as buffering issues, low resolution, poor quality, high operating cost, monetization, etc. Gyrus AI/ML Models helps to overcome these challenges by providing the highest possible quality of experience and quality of service.
AI/ML models can enable broadcasters and content providers to unearth opportunities to reduce streaming costs, enhance the viewer quality of experience, and improve viewer engagement using smart advertisement & video analytics by using AI/ML models throughout the media workflow.
Video Streaming Solution using Artificial intelligence:
Streaming Cost Optimization
Dynamic Advertisements
Automatic video synthesis for smart advertisement
Compliance to regulatory warnings
We are just at the beginning of the applications of AI to video streaming. Identifying objects, recognizing faces, inserting customized advertisements, complying with regulatory warnings, generating subtitles at high speeds, transmitting the video intelligently at low bandwidth and lower costs are a few of the tasks that can be managed by AI engines very effectively. AI and deep neural network-based enhancements will dramatically improve user Quality of experience and alter video consumption forever. AI solutions will soon become a widespread standard and will continue to redefine the streaming media ecosystem with emerging innovations.
Reference: https://gyrus.ai/
1 note
·
View note