#GeminiPro
Explore tagged Tumblr posts
Text
How many Gemini models are there? Which one is free to use?
There are currently two publicly available versions of Gemini:
Gemini 1.0 Pro: This is the free-to-use version, well-suited for basic tasks like question answering and text summarization.
Gemini Advanced: This requires a Google One AI subscription and offers more capabilities for complex and creative tasks like code generation and translation.
A quick look into the technical differences between Gemini Pro and Advanced.
Gemini 1.0 Pro
Model: 1.0 Pro
Access: Free
Strengths: Factual language understanding, reasoning, summarization
Context window: Smaller
Pricing: Free
Gemini Advanced
Model: Ultra 1.0
Access: Google One AI subscription required
Strengths: Complex tasks, creative text formats, code generation, translation
Context window: Larger
Pricing: $29/month (as part of Google One AI plan)
Understanding Google AI models, their strengths and pricing.
Gemini: Choosing the Right Language Model for Your Needs
Language models are becoming increasingly popular, and with good reason. They can generate text, translate languages, write different kinds of creative content, and answer your questions in an informative way. But with so many models on the market, it can be tough to decide which one is right for you.
In this blog, we’ll take a look at two of Google AI’s language models, Gemini 1.0 Pro and Gemini Advanced, and discuss their strengths and weaknesses to help you make an informed decision.
Gemini 1.0 Pro: The Free and Friendly Option
Gemini 1.0 Pro is a great choice for anyone looking for a free and easy-to-use language model. It excels at understanding factual language, reasoning, and summarizing text. If you need a model to answer your questions in a comprehensive and informative way, or to provide summaries of complex topics, Gemini 1.0 Pro is a solid option.
However, it’s important to remember that Gemini 1.0 Pro is not as powerful as its more advanced counterpart. It may struggle with complex tasks like code generation, writing different kinds of creative text formats, and translating languages. Additionally, its context window is smaller, meaning it can’t remember information from previous interactions as well.
Gemini Advanced: Unleashing the Power of AI
If you need a language model for more complex tasks, Gemini Advanced is the way to go. It’s significantly more powerful than Gemini 1.0 Pro and can handle tasks like code generation, translation, and writing different creative text formats with ease. Its larger context window also allows it to remember more information from previous interactions, making it more adept at understanding complex conversations.
However, Gemini Advanced does come with a price tag. It requires a Google One AI subscription, which can be a barrier for some users. Additionally, its increased power and complexity can make it less user-friendly than Gemini 1.0 Pro.
So, Which Model is Right for You?
The best language model for you depends on your specific needs and preferences. If you’re looking for a free and easy-to-use option for basic tasks, Gemini 1.0 Pro is a great choice. But if you need a more powerful model for complex tasks.
We suggest you start with the free version and if you think the speed and capacity of the free version is not enough, then go for an upgrade.
2 notes
·
View notes
Text
HTTP 429 Errors: Keep Your Users Online And Happy
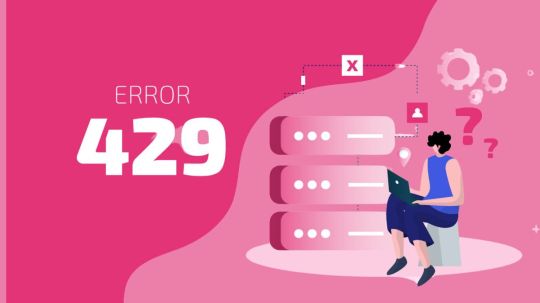
429 Errors
Avoid leaving your visitors waiting when resources run out: How to deal with 429 errors
429 error meaning
When a client sends too many requests to a server in a specified period of time, an HTTP error known as “Too Many Requests” (Error 429) occurs. This error can occur for many reasons:
Rate-limiting
The server limits client requests per time period.
Security
A DDoS attack or brute-force login attempt was detected by the server. In this instance, the server may block the suspect requestor’s IP.
Limits bandwidth
Server bandwidth is maxed out.
Per-user restrictions
The server has hit its maximum on user requests per time period.
The mistake may go away, but you should fix it to avoid losing traffic and rankings. Flushing your DNS cache forces your computer to acquire the latest DNS information, fixing the issue.
Large language models (LLMs) offer developers a great deal of capability and scalability, a seamless user experience depends on resource management. Because LLMs require a lot of processing power, it’s critical to foresee and manage possible resource depletion. Otherwise, 429 “resource exhaustion” errors could occur, which could interfere with users’ ability to interact with your AI application.
Google examines the reasons behind the 429 errors that LLMs make nowadays and provides three useful techniques for dealing with them successfully. Even during periods of high demand, you can contribute to ensuring a seamless and continuous experience by comprehending the underlying causes and implementing the appropriate solutions.
Backoff!
Retry logic and exponential backoff have been used for many years. LLMs can also benefit from these fundamental strategies for managing resource depletion or API unavailability. Backoff and retry logic in the code might be useful when a model’s API is overloaded with calls from generative AI applications or when a system is overloaded with inquiries. Until the overloaded system recovers, the waiting time grows dramatically with each retry.
Backoff logic can be implemented in your application code using decorators in Python. For instance, Tenacity is a helpful Python general-purpose retrying module that makes it easier to incorporate retry behavior into your code. Asynchronous programs and multimodal models with broad context windows, like Gemini, are more prone to 429 errors.
To show how backoff and retry are essential to the success of your gen AI application, Google tested sending a lot of input to Gemini 1.5 Pro. Google is straining the Gemini system by using photos and videoskept in Google Cloud Storage.
The results, where four of five attempts failed, are shown below without backoff and retry enabled.the results without backoff and retry configured
The outcomes with backoff and retry set up are shown below. By using backoff and retry, all five tries were successful. There is a trade-off even when the model responds to a successful API call. A response’s latency increases with the backoff and retry. Performance might be enhanced by modifying the model, adding more code, or moving to a different cloud zone. Backoff and retry, however, is generally better in times of heavy traffic and congestion.The results with backoff and retry configured
Additionally, you could frequently run into problems with the underlying APIs when working with LLMs, including rate-limiting or outages. It becomes increasingly crucial to protect against these when you put your LLM applications into production. For this reason, LangChain presented the idea of a fallback, which is a backup plan that might be employed in an emergency. One fallback option is to switch to a different model or even to a different LLM provider. To make your LLM applications more resilient, you can incorporate fallbacks into your code in addition to backoff and retry techniques.
With Apigee, circuit breaking is an additional strong choice for LLM resilience. You can control traffic distribution and graceful failure management by putting Apigee in between a RAG application and LLM endpoints. Naturally, every model will offer a unique solution, thus it is important to properly test the circuit breaking design and fallbacks to make sure they satisfy your consumers’ expectations.
Dynamic shared quota
For some models, Google Cloud uses dynamic shared quota to control resource allocation in an effort to offer a more adaptable and effective user experience. This is how it operates:
Dynamic shared quota versus Traditional quota
Traditional quota: In a Traditional quota system, you are given a set amount of API requests per day, per minute, or region, for example. You often have to file a request for a quota increase and wait for approval if you need more capacity. This can be inconvenient and slow. Of course, capacity is still on-demand and not dedicated, thus quota allocation alone does not ensure capacity. Dynamic shared quota: Google Cloud offers a pool of available capacity for a service through dynamic shared quota. All of the users submitting requests share this capacity in real-time. You draw from this shared pool according to your needs at any given time, rather than having a set individual limit.
Dynamic shared quota advantages
Removes quota increase requests: For services that employ dynamic shared quota, quota increase requests are no longer required. The system adapts to your usage habits on its own.
Increased efficiency: Because the system can distribute capacity where it is most needed at any given time, resources are used more effectively.
Decreased latency: Google Cloud can reduce latency and respond to your requests more quickly by dynamically allocating resources.
Management made easier: Since you don’t have to worry about reaching set limits, capacity planning is made easier.
Using a dynamic shared quota
429 resource exhaustion errors to Gemini with big multimodal input, like large video files, are more likely to result in resource exhaustion failures. The model performance of Gemini-1.5-pro-001 with a traditional quota and Gemini-1.5-pro-002 with a dynamic shared quota is contrasted below. It can be observed that the second-generation Gemini Pro model performs better than the first-generation model due to dynamic shared quota, even without retrying (which is not advised).model performance of Gemini-1.5-pro-001 with traditional quota versus Gemini-1.5-pro-002 with dynamic shared quotamodel performance of Gemini-1.5-pro-001 with traditional quota versus Gemini-1.5-pro-002 with dynamic shared quota
Dynamic shared quota should be used with backoff and retry systems, particularly as request volume and token size grow. In all of its initial attempts, it ran into 429 errors when testing the -002 model with greater video input. The test results below, however, show that all five subsequent attempts were successful when backoff and retry logic were used. This demonstrates how important this tactic is to the consistent performance of the more recent -002 Gemini model.
A move toward a more adaptable and effective method of resource management in Google Cloud is represented by dynamic shared quota. It seeks to maximize resource consumption while offering users a tightly integrated experience through dynamic capacity allocation. There is no user-configurable dynamic shared quota. Only certain models, such as Gemini-1.5-pro-002 and Gemini-1.5-flash-002, have Google enabled it.
As an alternative, you may occasionally want to set a hard-stop barrier to cease making too many API requests to Gemini. In Vertex AI, intentionally creating a customer-defined quota depends on a number of factors, including abuse, financial constraints and restrictions, or security considerations. The customer quota override capability is useful in this situation. This could be a helpful tool for safeguarding your AI systems and apps. Terraform’s google_service_usage_consumer_quota_override schema can be used to control consumer quota.
Provisioned Throughput
You may reserve specific capacity for generative AI models on the Vertex AI platform with Google Cloud’s Provisioned Throughput feature. This implies that even during periods of high demand, you can rely on consistent and dependable performance for your AI workloads.
Below is a summary of its features and benefits:
Benefits
Predictable performance: Your AI apps will function more smoothly if you eliminate performance fluctuation and receive predictable reaction times.
Reserved capacity: Queuing and resource contention are no longer concerns. For your AI models, you have a specific capacity. The pay-as-you-go charge is automatically applied to extra traffic when Provisioned Throughput capacity is exceeded.
Cost-effective: If you have regular, high-volume AI workloads, it can be less expensive than pay-as-you-go pricing. Use steps one through ten in the order process to determine whether Provisioned Throughput can save you money.
Scalable: As your demands change, you may simply increase or decrease the capacity you have reserved.
Image credit to Google Cloud
This would undoubtedly be helpful if your application has a big user base and you need to give quick response times. This is specifically made for applications like chatbots and interactive content creation that need instantaneous AI processing. Computationally demanding AI operations, including processing large datasets or producing intricate outputs, can also benefit from provisioned throughput.
Stay away with 429 errors
Reliable performance is essential when generative AI is used in production. Think about putting these three tactics into practice to accomplish this. It is great practice to integrate backoff and retry capabilities into all of your gen AI applications since they are made to cooperate.
Read more on Govindhtech.com
#HTTP429errors#ArtificialInteligence#AI#Google#googlecloud#GenerativeAI#Gemini#geminipro#govindhtech#NEWS#TechNews#technology#technologies#technologynews#technologytrends
0 notes
Text
دليلك للحصول على Gemini 1.5 Pro بشكل مجاني والبدء في استخدامه
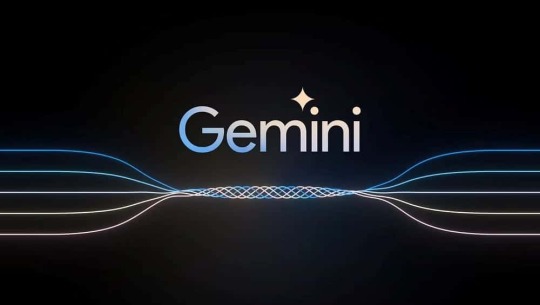
أصدرت Google نموذجها Gemini 1.5 Pro في فبراير 2024، لكنه أصبح مُتاحًا على نطاق واسع فقط في يونيو 2024. ومنذ ذلك الحين، شهد اعتمادًا سريعًا، مما يُوضح مدى قدرته. على الرغم من أنك ستحتاج إلى دفع رسوم اشتراك للوصول إلى مجموعة الميزات الكاملة، إلا أنَّ هناك طريقة يُمكنك من خلالها البدء في استخدام Gemini 1.5 Pro مجانًا اليوم. إذا كنت ترغب في تجربة هذا النموذج دون تكلفة، فهناك بعض الخطوات والنصائح التي يُمكنك اتباعها لتحقيق ذلك. تحقق من طرق جديدة لاستخدام Gmail بشكل أكثر فعاليَّة مع حيل Gemini المُذهلة. <a rel="dofollow" href="https://www.dztechy.com/how-use-gemini-pro-for-free/" data-sce-target="_blank">دليلك للحصول على Gemini 1.5 Pro بشكل مجاني والبدء في استخدامه</a> Read the full article
0 notes
Text
instagram
Google Gemini 1.5 Pro Tops the Charts!
Google's Gemini 1.5 Pro has surpassed OpenAI's GPT-4o and Anthropic’s Claude-3.5 Sonnet to claim the
1 spot on the LMSYS Chatbot Arena leaderboard. With an ELO score of 1300, Gemini 1.5 Pro excels in multi-lingual processing, technical tasks, and multimodal inputs. This significant achievement marks a new milestone in AI, highlighting the need for ethical considerations as these models evolve rapidly.
The competition in the LLM landscape is heating up, with major players pushing the boundaries of what's possible. . . .
For more AI related updates, follow @trillionstech.ai
0 notes
Text
Cách sử dụng Gemini AI của google tại Việt Nam
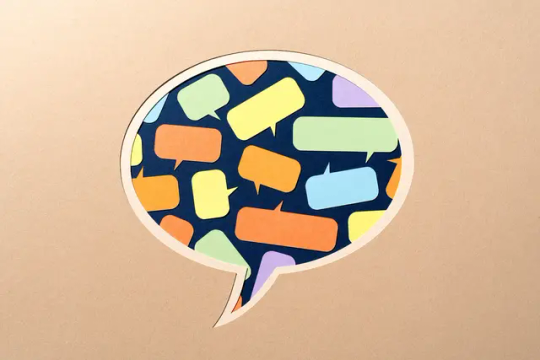
Google đã phát hành một mô hình trí tuệ nhân tạo (AI) mới gọi là Gemini (Google Gemini AI), được cho là tốt hơn các mô hình AI khác về khả năng hiểu, tóm tắt, suy luận, lập trình và lập kế hoạch. Gemini có ba phiên bản: Pro, Ultra và Nano. Phiên bản Pro đã có sẵn, và phiên bản Ultra được phát hành vào đầu năm 2024. Xem thêm: - Tự tạo video chỉ với vài dòng text, trải nghiệm sức mạnh của Sora - Gemini AI là gì? Khám phá tiềm năng Chatbot mới của Google - Galaxy AI làm được những gì? Bùng nổ k�� nguyên điện thoại AI - Làm chủ Prompt AI và nghệ thuật giao tiếp với AI
Gemini đã được tích hợp vào chatbot Bard của Google
Google đã tích hợp Gemini Pro với chatbot Bard của mình, đối thủ cạnh tranh trực tiếp của ChatGPT. Người dùng có thể tương tác bằng văn bản với Bard được hỗ trợ bởi Gemini. Google đã cam kết sẽ hỗ trợ các phương thức tích hợp khác sớm. Bản cập nhật mới có sẵn ở 170 quốc gia và vùng lãnh thổ nhưng giới hạn ở tiếng Anh. Hiện tại có hai cách để sử dụng Google Gemini AI: - Bard Chatbot: Gemini AI phiên bản Pro hiện được tích hợp sẵn vào chatbot Bard . Để sử dụng Gemini AI trong Bard, chỉ cần truy cập trang web Bard và đăng nhập bằng tài khoản Google của bạn. - Pixel 8 Pro: Gemini AI bản Nano cũng có sẵn trên điện thoại thông minh Pixel 8 Pro. Để sử dụng Gemini AI trên Pixel 8 Pro, chỉ cần bật tính năng "Trả lời văn bản được gợi ý bởi AI" trong cài đặt WhatsApp. Người dùng ở Việt Nam cũng đang nhận được bản cập nhật mới. Bạn có thể truy cập sử dụng các tính năng của Gemini qua chatbot Google Bard tại https://gemini.google.com
5 cách để khai thác Gemini AI tốt nhất phục vụ công việc
Google gần đây đã ra mắt Gemini Advanced, phiên bản mạnh mẽ nhất của công cụ thay thế ChatGPT này. Phiên bản mới và được cải tiến này hỗ trợ 40 ngôn ngữ và miễn phí trong 2 tháng. Dưới đây là 5 cách bạn có thể sử dụng nó để phục vụ công việc: 1. Tạo Hình ảnh: Tạo hình ảnh chân thực chỉ bằng các mô tả văn bản. 2. Soạn Thư điện tử và Tài liệu: Bạn có thể dễ dàng soạn thư điện tử, tạo tài liệu và thêm ngữ cảnh hoặc thông tin bổ sung ngay trong Gmail và Google Docs. 3. Trò chuyện bằng giọng nói: Bạn có thể nhấn nút micrô để bật micrô và trò chuyện với Gemini. 4. Tiện ích: Bật các tiện ích mở rộng như YouTube, Drive và Maps để nhận được phản hồi tốt hơn. 5. Lập trình: Bạn có thể yêu cầu Gemini viết, giải thích và gỡ lỗi bất kỳ đoạn mã lập trình nào bạn muốn. Xem thêm tính năng Gemini cho Google Workspace và Google Cloud tại đây: Cách làm chủ Gemini AI: từng bước cài đặt chi tiết
Cách sử dụng Google Gemini trên Pixel 8 Pro
Nếu bạn đang sở hữu chiếc Google Pixel 8 Pro, bạn cũng có thể sử dụng Gemini trên thiết bị của mình mà không cần kết nối Internet. Dòng iện thoại này của Google hỗ trợ sẵn Gemini Nano, phiên bản rút gọn của Gemini mà thiết bị có thể chạy ngay cả khi ngoại tuyến. Gemini Nano tích hợp trong tính năng cao: Trả lời thông minh và Ghi âm. Trả lời thông minh là tính năng gợi ý điều tiếp theo cần nói trong ứng dụng nhắn tin. Trên Pixel 8 Pro, tính năng này được cung cấp bởi Gemini Nano, giúp tạo ra phản hồi tự nhiên và phù hợp hơn trước kia. Để sử dụng tính năng Trả lời thông minh, bạn cần bật AiCore trong Tùy chọn nhà phát triển, sau đó mở WhatsApp để trải nghiệm. Bạn có thể bật tính năng này bằng cách chuyển đến Cài đặt > Tùy chọn nhà phát triển > Cài đặt AiCore > Bật Aicore Persistent .
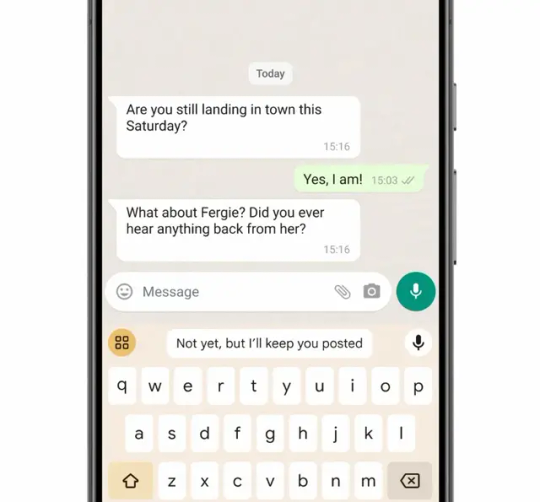
(Hình ảnh: Google) Khi tính năng này được bật, bạn sẽ thấy các đề xuất Trả lời thông minh được hỗ trợ bởi Gemini trong dải đề xuất của bàn phím Gboard. Hiện tại, tính năng này mới hỗ trợ cho tiếng Anh Mỹ trong WhatsApp, Google có kế hoạch mở rộng hỗ trợ cho nhiều ứng dụng và khu vực hơn trong năm tới. Vị trí thứ hai mà bạn có thể sử dụng tốt khả năng của Gemini là ứng dụng Máy ghi âm . Với hỗ trợ của Gemini, giờ đây bạn có thể tạo bản tóm tắt của một cuộc họp đầy đủ chỉ bằng một cú nhấp chuột, cung cấp cho bạn cái nhìn tổng quan nhanh chóng về các điểm chính và điểm nổi bật. Để sử dụng tính năng này, bạn chỉ cần mở ứng dụng và bắt đầu ghi âm. Sau đó, bạn có thể nhấn vào nút tóm tắt để xem bản tóm tắt âm thanh do Gemini Nano tạo ra.
Lịch sử phát hành của Bard AI
Bard AI lần đầu tiên được phát hành dưới dạng phiên bản beta vào ngày 18 tháng 12 năm 2022. Phiên bản beta này chỉ cho phép sử dụng bởi các nhà nghiên cứu và học giả được chọn. Phiên bản chính thức của Bard AI được phát hành vào ngày 1 tháng 7 năm 2023. Lịch sử phát hành của Bard AI theo từng giai đoạn: - Giai đoạn 1 (2022): Phát triển và thử nghiệm Trong giai đoạn này, Bard AI được phát triển và thử nghiệm bởi một nhóm các nhà khoa học và kỹ sư tại Google AI. Nhóm nghiên cứu đã sử dụng một tập dữ liệu khổng lồ gồm văn bản và mã để đào tạo Bard AI. - Giai đoạn 2 (2022-2023): Phát hành beta Trong giai đoạn này, Bard AI được phát hành dưới dạng phiên bản beta cho các nhà nghiên cứu và học giả được chọn. Phiên bản beta này được sử dụng để thu thập phản hồi từ người dùng và cải thiện hiệu suất của Bard AI. - Giai đoạn 3 (2023-nay): Phát hành chính thức Trong giai đoạn này, Bard AI được phát hành dưới dạng phiên bản chính thức cho tất cả mọi người sử dụng. Phiên bản chính thức này có sẵn trên nhiều nền tảng khác nhau, bao gồm web, ứng dụng di động và các sản phẩm của Google. Từ tháng 12/2023 Google tích hợp mô hình Gemini Ai Pro vào Bard AI.
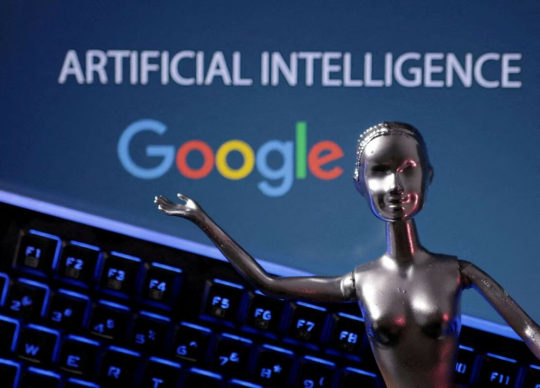
Google tuyên bố Gemini là mô hình AI mạnh mẽ nhất thế giới
Google tuyên bố Gemini là mô hình AI mạnh mẽ nhất thế giới. Một trong những khác biệt lớn nhất so với các mô hình AI phổ biến khác là Gemini được xây dựng như một công cụ đa phương thức từ đầu. Google đã chia sẻ một số bản demo của mô hình AI mới này, cho phép có thể dễ dàng tương tác qua nhiều phương tiện như văn bản, âm thanh, video. Nó cũng có thể kết hợp các phương thức này để làm cho tương tác trở nên giống người hơn.
Gemini đã xuất sắc vượt trội qua nhiều bài kiểm tra AI
Google đã thử nghiệm mô hình AI mới của mình, Gemini, trên nhiều mô hình kiểm thử. Gemini Ultra, phiên bản tiên tiến nhất của Gemini, đã đạt kết quả tốt hơn kết quả tốt nhất hiện tại trên 30/32 bài kiểm tra học thuật phổ biến được sử dụng trong nghiên cứu và phát triển AI. Gemini Ultra đạt 90,0% điểm trong một bài kiểm tra gọi là MMLU (nhận thức ngôn ngữ đa nhiệm lớn). Bài kiểm tra này sử dụng 57 chủ đề như toán học, vật lý, lịch sử, luật pháp, y học và đạo đức để kiểm tra cả kiến thức và khả năng giải quyết vấn đề. Đây là lần đầu tiên một mô hình AI đạt điểm cao hơn các chuyên gia con người trên bài kiểm tra này.
Gemini có khả năng hỗ trợ lập trình mạnh mẽ
Phiên bản đầu tiên của Gemini có thể hiểu, giải thích và tạo mã code chất lượng cao trong các ngôn ngữ lập trình phổ biến như Python, Java, C++, và Go. Google tuyên bố rằng điều này khiến nó trở thành một trong những mô hình AI tốt nhất cho việc lập trình trên thế giới. Gemini Ultra đã rất xuất sắc trong một số bài kiểm tra lập trình tự động trong đó có HumanEval, một tiêu chuẩn công nghiệp quan trọng để kiểm tra hiệu suất trên các tác vụ lập trình, và Natural2Code, một chuẩn kiểm tra việc lập trình do người hay tổng hợp từ nguồn sẵn.
Gemini có thể được sử dụng để cung cấp đầu vào cho các hệ thống lập trình tiên tiến hơn
Hai năm trước, Google đã trình bày AlphaCode, hệ thống AI đầu tiên để tạo mã có thể cạnh tranh trong các cuộc thi lập trình. Sử dụng phiên bản đặc biệt của Gemini, Google đã tạo ra một hệ thống coding tiên tiến hơn, AlphaCode 2. Hệ thống này rất tốt trong việc giải quyết các vấn đề hot trong lập trình liên quan đến toán học phức tạp và khoa học máy tính lý thuyết.
Câu hỏi thường gặp (FAQs) về Gemini AI:
Google Gemini là gì?Gemini là mô hình AI tiên tiến nhất của Google, có thể hiểu và tích hợp nhiều loại thông tin như văn bản, mã, âm thanh, hình ảnh và video. Nó đại diện cho một bước nhảy đáng kể về khả năng AI.Gemini khác với các mô hình AI trước đây như thế nào?Gemini độc đáo với khả năng đa phương thức gốc của nó, có thể xử lý các loại dữ liệu khác nhau ngay từ đầu, nâng cao hiệu quả và hiệu quả trong việc xử lý các tác vụ phức tạp.Có những phiên bản Gemini nào khác nhau?Gemini có ba phiên bản: Ultra cho các tác vụ phức tạp, Pro cho nhiều tác vụ và Nano cho hoạt động hiệu quả trên thiết bị.Điều gì khiến Gemini Ultra trở nên đặc biệt?Gemini Ultra đã vượt qua các chuyên gia con người về hiểu ngôn ngữ đa nhiệm và xuất sắc trong các điểm đánh giá chuẩn lập trình, cho thấy hiệu suất vượt trội trong các tác vụ đa dạng. Gemini đóng góp như thế nào và cho các lĩnh vực gì?Khả năng suy luận đa phương thức tiên tiến của Gemini có thể dẫn đến những đột phá trong các lĩnh vực như khoa học và tài chính bằng cách phân tích và hiểu thông tin phức tạp.Có những biện pháp an toàn nào được áp dụng cho Gemini không?Gemini đã trải qua các đánh giá an toàn toàn diện, bao gồm đánh giá độ lệch và độc tính, và tuân thủ các Nguyên tắc AI của Google về phát triển AI có trách nhiệm.Gemini được tích hợp vào các sản phẩm Google như thế nào?Gemini đang được triển khai trong các sản phẩm Google như Bard, các tính năng nâng cao mở rộng trên Pixel 8 Pro (Gemini Nano) và sẽ sớm mở rộng và tích hợp vào nhiều nền tảng dịch vụ như Tìm kiếm và Chrome.Khả năng mở rộng của Gemini là gì?Gemini được thiết kế để chạy hiệu quả trên nhiều nền tảng, từ trung tâm dữ liệu đến thiết bị di động, điều đó cho phép khả năng mở rộng và thích ứng nhanh với nhiều hệ sinh tháiCác tính năng chính của Gemini là gì?Gemini xuất sắc trong việc hiểu và tạo mã code lập trình, suy luận đa phương thức và vượt qua các điểm chuẩn về hiểu ngôn ngữ, cho thấy khả năng đa dạng của nó.Hiệu suất của Gemini đối với các tác vụ lập trình như thế nào?Gemini đã thể hiện hiệu suất rất cao trong việc tạo ra code lập trình theo tiêu chuẩn và chất lượng cao bằng các ngôn ngữ lập trình phổ biến như Python và Java. Read the full article
#AIBard#bard#BardAI#BardAIlàgì#bardgooglegemini#chatgpt#chatbot#geminiai#geminipro#làmthếnàođểdùnggemini#lịchsửBardAI#sửdụnggeminiaitạiviệtnam
0 notes
Text
Embark on a linguistic adventure with Bard! Explore how Gemini Pro's global access and image generation empower you to create, learn, and express like never before. Discover exciting details, possibilities, and more!
#BardAI#GeminiPro#GlobalAccess#ImageGeneration#AIArt#Creativity#LanguageLearning#FutureofTech#ExploreWithBard
0 notes
Text
GeminiPro Review
Beware of GeminiPro Scams, a dubious entity operating in the financial industry. This suspicious scam broker raises red flags. To delve deeper into the details, read GeminiPro review here. Protect your investments and stay informed to avoid falling victim to potential fraud.
0 notes
Text
【悲報】ワイ、中華スマホが欲しすぎて泣く…
1: 2017/07/03(月) 11:26:51.46 ID:nNhaDPV80livip ulefone Gemini Pro MediaTek Helio X27 Decacore (2.6GHz) RAM 4GB / ROM 64GB 5.5in 1920×1080 IPS 2.5D 13MP Chromatic + 13MP Monochrome Camera NanoSIM+NanoSIMorMicroSD 4G+3G DSDS http://ulefone.com/images/gemini-pro/geminipro-presale/gemini-pro-banner-l.png 2: 2017/07/03(月) 11:27:18.91 ID:SitaAy6/M でもお高いんでしょう? 3: 2017/07/03(月) 11:27:43.55 ID:yK5L1I7mr…
View On WordPress
0 notes
Text
Top 10 Artificial Intelligence (AI) TTS Conversion Tools
1 note
·
View note
Text
Cloud Virtual CISO: 3 Intriguing AI Cybersecurity Use Cases
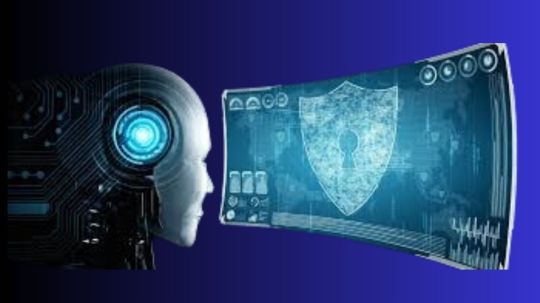
Cloud Virtual CISO Three intriguing AI cybersecurity use cases from a Cloud Virtual CISO intriguing cybersecurity AI use cases
For years, They’ve believed artificial intelligence might transform cybersecurity and help defenders. According to Google Cloud, AI can speed up defences by automating processes that formerly required security experts to labour.
While full automation is still a long way off, AI in cybersecurity is already providing assisting skills. Today’s security operations teams can benefit from malware analysis, summarization, and natural-language searches, and AI can speed up patching.
AI malware analysis Attackers have created new malware varieties at an astonishing rate, despite malware being one of the oldest threats. Defenders and malware analyzers have more varieties, which increases their responsibilities. Automation helps here.
Their Gemini 1.5 Pro was tested for malware analysis. They gave a simple query and code to analyse and requested it to identify dangerous files. It was also required to list compromising symptoms and activities.
Gemini 1.5 Pro’s 1 million token context window allowed it to parse malware code in a single pass and normally in 30 to 40 seconds, unlike previous foundation models that performed less accurately. Decompiled WannaCry malware code was one of the samples They tested Gemini 1.5 Pro on. The model identified the killswitch in 34 seconds in one pass.
They tested decompiled and disassembled code with Gemini 1.5 Pro on multiple malware files. Always correct, it created human-readable summaries.
The experiment report by Google and Mandiant experts stated that Gemini 1.5 Pro was able to accurately identify code that was obtaining zero detections on VirusTotal. As They improve defence outcomes, Gemini 1.5 Pro will allow a 2 million token context frame to transform malware analysis at scale.
Boosting SecOps with AI Security operations teams use a lot of manual labour. They can utilise AI to reduce that labour, train new team members faster, and speed up process-intensive operations like threat intelligence analysis and case investigation noise summarising. Modelling security nuances is also necessary. Their security-focused AI API, SecLM, integrates models, business logic, retrieval, and grounding into a holistic solution. It accesses Google DeepMind’s cutting-edge AI and threat intelligence and security data.
Onboarding new team members is one of AI’s greatest SecOps benefits. Artificial intelligence can construct reliable search queries instead of memorising proprietary SecOps platform query languages.
Natural language inquiries using Gemini in Security Operations are helping Pfizer and Fiserv onboard new team members faster, assist analysts locate answers faster, and increase security operations programme efficiency.
Additionally, AI-generated summaries can save time by integrating threat research and explaining difficult facts in natural language. The director of information security at a leading multinational professional services organisation told Google Cloud that Gemini Threat Intelligence AI summaries can help write an overview of the threat actor, including relevant and associated entities and targeted regions.
The customer remarked the information flows well and helps us obtain intelligence quickly. Investigation summaries can be generated by AI. As security operations centre teams manage more data, they must detect, validate, and respond to events faster. Teams can locate high-risk signals and act with natural-language searches and investigation summaries.
Security solution scaling with AI In January, Google’s Machine Learning for Security team published a free, open-source fuzzing platform to help researchers and developers improve vulnerability-finding. The team told AI foundation models to write project-specific code to boost fuzzing coverage and uncover additional vulnerabilities. This was added to OSS-Fuzz, a free service that runs open-source fuzzers and privately alerts developers of vulnerabilities.
Success in the experiment: With AI-generated, extended fuzzing coverage, OSS-Fuzz covered over 300 projects and uncovered new vulnerabilities in two projects that had been fuzzed for years.
The team noted, “Without the completely LLM-generated code, these two vulnerabilities could have remained undiscovered and unfixed indefinitely.” They patched vulnerabilities with AI. An automated pipeline for foundation models to analyse software for vulnerabilities, develop patches, and test them before picking the best candidates for human review was created.
The potential for AI to find and patch vulnerabilities is expanding. By stacking tiny advances, well-crafted AI solutions can revolutionise security and boost productivity. They think AI foundation models should be regulated by Their Secure AI Framework or a similar risk-management foundation to maximise effect and minimise risk.
Please contact Ask Office of the CISO or attend Their security leader events to learn more. Attend Their June 26 Security Talks event to learn more about Their AI-powered security product vision.
Perhaps you missed it Recent Google Cloud Security Talks on AI and cybersecurity: Google Cloud and Google security professionals will provide insights, best practices, and concrete ways to improve your security on June 26.
Quick decision-making: How AI improves OODA loop cybersecurity: The OODA loop, employed in boardrooms, helps executives make better, faster decisions. AI enhances OODA loops.
Google rated a Leader in Q2 2024 Forrester Wave: Cybersecurity Incident Response Services Report.
From always on to on demand access with Privileged Access Manager: They are pleased to introduce Google Cloud’s built-in Privileged Access Manager to reduce the dangers of excessive privileges and elevated access misuse.
A FedRAMP high compliant network with Assured Workloads: Delivering FedRAMP High-compliant network design securely.
Google Sovereign Cloud gives European clients choice today: Customer, local sovereign partner, government, and regulator collaboration has developed at Google Sovereign Cloud.
Threat Intel news A financially motivated threat operation targets Snowflake customer database instances for data theft and extortion, according to Mandiant.
Brazilian cyber hazards to people and businesses: Threat actors from various reasons will seek opportunities to exploit Brazil’s digital infrastructure, which Brazilians use in all sectors of society, as its economic and geopolitical role grows.
Gold-phishing: Paris 2024 Olympics cyberthreats: The Paris Olympics are at high risk of cyber espionage, disruptive and destructive operations, financially driven behaviour, hacktivism, and information operations, according to Mandiant.Return of ransomware Compared to 2022, data leak site posts and Mandiant-led ransomware investigations increased in 2023.
Read more on Govindhtech.com
#CloudVirtualCISO#CloudVirtual#ai#cybersecurity#CISO#llm#MachineLearning#Gemini#GeminiPro#technology#googlecloud#technews#news#govindhtech
0 notes
Text
Moshi AI Voice chat using artificial intelligence
#ai#Gemini#GPT4o#alkoptantech#GeminiPro#googlechat#OpenAl#ChatGPT5#الذكاء_الاصطناعي_التوليدي#Apps#MoshiAI#blog#seo
1 note
·
View note
Text
How Gemini Model and Workflows Can Summarize Anything
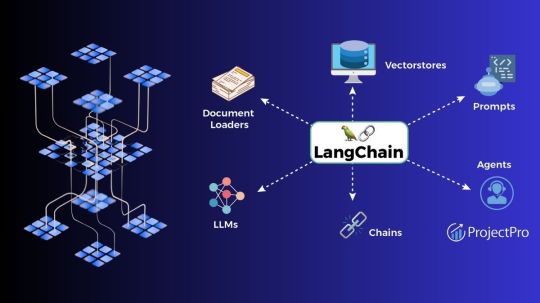
Gemini Model Planes
Given that generative AI is currently a hot topic among developers and business stakeholders, it’s critical to investigate the ways in which server less execution engines such as Google Cloud’s Workflows can automate and coordinate large language model (LLM) use cases. They recently discussed using workflows to orchestrate Vertex AI’s PaLM and Gemini APIs. In this blog post, they demonstrate a specific use case with broad applicability long-document summarization that Workflows can accomplish.
Gemini Large Language Model
To execute complex tasks like document summarising, open-source LLM orchestration frameworks such as LangChain (for Python and TypeScript developers) or LangChain4j (for Java developers) integrate different components including LLMs, document loaders, and vector databases. For this reason, Workflows can likewise be used without requiring a large time investment in an LLM orchestration system.
Techniques for Summarising
Summarising a brief document is as simple as typing its content in its entirety as a prompt into the context window of an LLM. On the other hand, large language model prompts are typically token-count constrained. Extended documents necessitate an other methodology. There are two typical methods:
Map/reduce
To make a lengthy document fit the context window, it is divided into smaller portions. A summary is written for every segment, and as a last step, a summary of all the summaries is written.
Iterative improvement
google cloud assess the document individually, much like the map/reduce method. The first section is summarised, and the LLM iteratively refines it with information from the next section, and so on, all the way to the end of the document.
Both approaches produce excellent outcomes. The map/reduce strategy does, however, have one benefit over the refining technique. Refinement is a progressive process in which the previously refined summary is used to summarise the next section of the document.
You can use map/reduce to construct a summary for each segment in parallel (the “map” operation) and a final summary in the last phase (the “reduce” action), as shown in the picture below. Compared to the sequential method, this is faster.Image to credit google cloud
Google Gemini Models
In an earlier post, they demonstrated how to use Workflows to invoke PaLM and Gemini models and emphasised one of its primary features: concurrent step execution. This function allows us to concurrently produce summaries of the lengthy document parts.
When a new text document is added to a Cloud Storage bucket, the workflow is started.
The text file is divided into “chunks” that are successively summarised.
The smaller summaries are all gathered together and combined into a single summary in the last step of summarising.
Thanks to a subworkflow, all calls to the Gemini 1.0 Pro model are made.
Obtaining the text file and concurrently summarising the relevant portions (“map” component)
A few constants and data structures are prepared in the assign file vars phase. Here, google cloud set the chunk size to 64,000 characters so that the text would fit in the LLM’s context window and adhere to Workflow’s memory constraints. In addition, there are variables for the summaries’ lists and one for the final summary.
The dump file content sub-step loads each section of the document from Cloud Storage before the loop over chunks step removes each text chunk in parallel. The subworkflow in generate chunk summary, which summarises that portion of the document, is then invoked using the Gemini model. The current summary is finally saved in the summaries array.
An overview of summaries (the “reduce” portion)
With all of the chunk summaries now in hand, they can combine the smaller summaries into a final summary of summaries, or aggregate summary as you may call it:They concatenate each chunk summary in concat summaries. To obtain the final summary, they make one final call to google cloud Gemini model summarization subworkflow in the reduce summary phase. They also return the results, including the final summary and the chunk summaries, in return result.
Asking for summaries from the Gemini model
Google cloud “map” and “reduce” procedures both invoke a subworkflow that uses Gemini models to capture the call. Let’s examine this last step of their process in more detail: They set up a few variables for the desired LLM configuration (in this case, Gemini Pro) in init.
They perform an HTTP POST request to the model’s REST API in the call gemini phase. Take note of how merely providing the OAuth2 authentication technique allows us to declaratively authenticate to this API. They pass the prompt for a summary, certain model parameters (like temperature) and the maximum length of the summary to be generated in the body.
The ensuing synopsis
The process is started and completed by saving the text of Jane Austen’s “Pride and Prejudice” into a Cloud Storage bucket. This produces the following preliminary and comprehensive summaries:
Gemini Models
Although they kept the workflow straightforward for the sake of this article, there are a number of ways it might be made even better. For instance, they hard-coded the total character count for each section’s summary, but it could be a process parameter or even calculated based on how long the context-window limit of the model is.
They could deal with situations where an exceptionally long list of document section summaries wouldn’t fit in memory because workflows themselves have a memory limit for the variables and data they store in memory. Not to be overlooked is google cloud more recent large language model, Gemini 1.5, which can summarise a lengthy document in a single run and accept up to one million tokens as input. Of course, you may also utilise an LLM orchestration framework, but Workflows itself can handle certain interesting use cases for LLM orchestration, as this example shows.
In brief
In this paper, they developed a lengthy document summarization exercise without the usage of a specialised LLM framework and examined a new use case for orchestrating LLMs with Workflows. They decreased the time required to construct the entire summary by using Workflows’ parallel step capabilities to create section summaries concurrently
Read more on Govindhtech.com
#geminmodels#generativeai#GoogleCloud#LLM#vertixai#LangChain#CloudStorage#GeminiPro#news#technews#technology#technologynews#technologytrends#govindhtech
0 notes
Text
Leveraging Gemini 1.0 Pro Vision in BigQuery
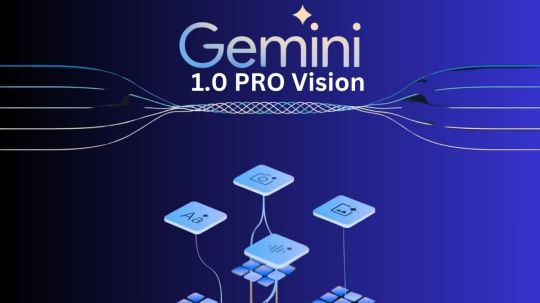
Gemini 1.0 Pro Vision in BigQuery
Organisations are producing more unstructured data in the form of documents, audio files, videos, and photographs as a result of the widespread use of digital devices and platforms, such as social media, mobile devices, and Internet of Things sensors. In order to assist you in interpreting and deriving valuable insights from unstructured data, Google Cloud has introduced BigQuery interfaces with Vertex AI over the last several months. These integrations make use of Gemini 1.0 Pro, PaLM, Vision AI, Speech AI, Doc AI, Natural Language AI, and more.
Although Vision AI can classify images and recognize objects, large language models (LLMs) open up new visual application cases. With Gemini 1.0 Pro Vision, they are extending BigQuery and Vertex AI integrations to provide multimodal generative AI use cases. You may use Gemini 1.0 Pro Vision directly in BigQuery to analyse photos and videos by mixing them with custom text prompts using well-known SQL queries.
Multimodal capabilities in a data warehouse context may improve your unstructured data processing for a range of use cases:
Object recognition: Respond to inquiries pertaining to the precise identification of items in pictures and movies.
Information retrieval: Integrate existing knowledge with data gleaned from pictures and videos.
Captioning and description: Provide varied degrees of depth in your descriptions of pictures and videos.
Understanding digital content: Provide answers by gathering data from web sites, infographics, charts, figures, and tables.
Structured content generation: Using the prompts supplied, create replies in HTML and JSON formats.
Converting unorganised information into an organised form
Gemini 1.0 Pro Vision may provide structured replies in easily consumable forms such as HTML or JSON, with just minor prompt alterations needed for subsequent jobs. Having structured data allows you to leverage the results of SQL operations in a data warehouse like BigQuery and integrate it with other structured datasets for further in-depth analysis.
Consider, for instance, that you have a large dataset that includes pictures of vehicles. Each graphic contains some fundamental information about the automobile that you should be aware of. Gemini 1.0 Pro Vision can be helpful in this use situation!Image credit to Google cloud
As you can see, Gemini has answered with great detail! However, if you’re a data warehouse, the format and additional information aren’t as useful as they are for individuals. You may modify the prompt to instruct the model on how to produce a structured answer, saving unstructured data from becoming even more unstructured.Image credit to Google cloud
You can see how a BigQuery-like setting would make this answer much more helpful.
Let’s now examine how to ask Gemini 1.0 Pro Vision to do this analysis over hundreds of photos straight in BigQuery!
Gemini 1.0 Pro Vision Access via BigQuery ML
BigQuery and Gemini 1.0 Pro Vision are integrated via the ML.GENERATE_TEXT() method. You must build a remote model that reflects a hosted Vertex AI big language model in order to enable this feature in your BigQuery project. Thankfully, it’s just a few SQL lines:
After the model is built, you may produce text by combining your data with the ML.GENERATE_TEXT() method in your SQL queries.
A few observations on the syntax of the ML.GENERATE_TEXT() method when it points to a gemini-pro-vision model endpoint, as this example does:
TABLE: Accepts as input an object table including various unstructured object kinds (e.g. photos, movies).
PROMPT: Applies a single string text prompt to each object, row-by-row, in the object TABLE. This prompt is part of the option STRUCT, which is different from the situation when using the Gemini-Pro model.
To extract the data for the brand, model, and year into new columns for use later, they may add additional SQL to this query.
The answers have now been sorted into brand-new, organised columns.
There you have it, then. A set of unlabeled, raw photos has just been transformed by Google Cloud into structured data suitable for data warehouse analysis. Consider combining this new table with other pertinent business data. For instance, you might get the median or average selling price for comparable automobiles in a recent time frame using a dataset of past auto sales. These are just a few of the opportunities that arise when you include unstructured data into your data operations!
A few things to keep in mind before beginning to use Gemini 1.0 Pro Vision in BigQuery are as follows:
To do Gemini 1.0 Pro Vision model inference over an object table, you need an enterprise or enterprise plus reservation.
Vertex AI large language models (LLMs) and Cloud AI services are subject to limits; thus, it is important to evaluate the current quota for the Gemini 1.0 Pro Vision model.
Next actions
There are several advantages of integrating generative AI straight into BigQuery. You can now write a few lines of SQL to do the same tasks as creating data pipelines and bespoke Python code between BigQuery and the generative AI model APIs! BigQuery scales from one prompt to hundreds while handling infrastructure management.
Read more on Govindhtech.com
#govindhtech#news#BigQuery#Gemini#geminivisionpro#GeminiPro#VertexAI#technologynews#technology#TechnologyTrends
0 notes
Text
Examine Gemini 1.0 Pro with BigQuery and Vertex AI
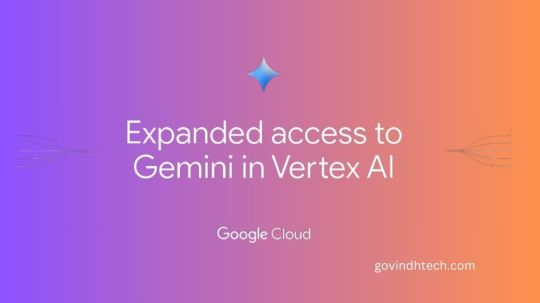
BigQuery and Vertex AI to explore Gemini 1.0 Pro
Innovation may be stifled by conventional partitions separating data and AI teams. These disciplines frequently work independently and with different tools, which can result in data silos, redundant copies of data, overhead associated with data governance, and budgetary issues. This raises security risks, causes ML deployments to fail, and decreases the number of ML models that make it into production from the standpoint of AI implementation.
It can be beneficial to have a single platform that removes these obstacles in order to accelerate data to AI workflows, from data ingestion and preparation to analysis, exploration, and visualization all the way to ML training and inference in order to maximize the value from data and AI investments, particularly around generative AI.
Google is recently announced innovations that use BigQuery and Vertex AI to further connect data and AI to help you achieve this. They will explore some of these innovations in more detail in this blog post, along with instructions on how to use Gemini 1.0 Pro in BigQuery.
What is BigQuery ML?
With Google BigQuery’s BigQuery ML capability, you can develop and apply machine learning models from within your data warehouse. It makes use of BigQuery’s processing and storing capability for data as well as machine learning capabilities, all of which are available via well-known SQL queries or Python code.
Utilize BigQuery ML to integrate AI into your data
With built-in support for linear regression, logistic regression, and deep neural networks; Vertex AI-trained models like PaLM 2 or Gemini Pro 1.0; or imported custom models based on TensorFlow, TensorFlow Lite, and XGBoost, BigQuery ML enables data analysts and engineers to create, train, and execute machine learning models directly in BigQuery using familiar SQL, helping them transcend traditional roles and leverage advanced ML models directly in BigQuery. Furthermore, BigQuery allows ML engineers and data scientists to share their trained models, guaranteeing that data is used responsibly and that datasets are easily accessible.
Every element within the data pipeline may employ distinct tools and technologies. Development and experimentation are slowed down by this complexity, which also places more work on specialized teams. With the help of BigQuery ML, users can create and implement machine learning models using the same SQL syntax inside of BigQuery. They took it a step further and used Vertex AI to integrate Gemini 1.0 Pro into BigQuery in order to further streamline generative AI. Higher input/output scale and improved result quality are key features of the Gemini 1.0 Pro model, which is intended to be used for a variety of tasks such as sentiment analysis and text summarization.
BigQuery ML allows you to integrate generative models directly into your data workflow, which helps you scale and optimize them. By doing this, bottlenecks in data movement are removed, promoting smooth team collaboration and improving security and governance. BigQuery’s tested infrastructure will help you achieve higher efficiency and scale.
There are many advantages to applying generative AI directly to your data:
Reduces the requirement for creating and maintaining data pipelines connecting BigQuery to APIs for generative AI models
Simplifies governance and, by preventing data movement, helps lower the risk of data loss
Lessens the requirement for managing and writing unique Python code to call AI models
Allows petabyte-scale data analysis without sacrificing performance
Can reduce your ownership costs overall by using a more straightforward architecture
In order to perform sentiment analysis on their data, Faraday, a well-known customer prediction platform, had to previously create data pipelines and join multiple datasets. They streamlined the process by giving LLMs direct access to their data, merging it with more first-party customer information, and then feeding it back into the model to produce hyper-personalized content��all inside BigQuery. To find out more, view this sample video.
Gemini 1.0 Pro and BigQuery ML
Create the remote model that reflects a hosted Vertex AI large language model before using Gemini 1.0 Pro in BigQuery. Usually, this process only takes a few seconds. After the model is built, use it to produce text by merging data straight from your BigQuery tables. Then, to access the Gemini 1.0 Pro via Vertex AI and carry out text-generation tasks, use the ML.GENERATE_TEXT construct. The database record and your PROMPT statement are appended by CONCAT. The prompt parameter that controls response randomness is temperature; the lower the temperature, the more relevant the response will be. The boolean flatten_json_output, when set to true, yields a flat, comprehensible text that has been taken from the JSON response.
What your data can achieve with generative AI
They think that the potential of AI technology for your business data is still largely unrealized. Data analysts’ responsibilities are growing with generative AI, going beyond just gathering, processing, and analyzing massive datasets to include proactively influencing data-driven business impact.
Data analysts can, for instance, use generative models to compile past email marketing data (open rates, click-through rates, conversion rates, etc.) and determine whether personalized offers outperform generic promotions or not, as well as which subject line types consistently result in higher open rates. Analysts can use these insights to direct the model to generate a list of interesting options for the subject line that are specific to the identified preferences. With just one platform, they can also use the generative AI model to create interesting email content.
Early adopters have shown a great deal of interest in resolving a variety of use cases from different industries. For example, the following advanced data processing tasks can be made simpler by using ML.GENERATE_TEXT:
Content generation
Without the need for sophisticated tools, analyze user feedback to create customized email content directly within BigQuery. “Create a marketing email using customer sentiment from [table name] “is a prompt
Summarize
Summarize text that is kept in BigQuery columns, like chat transcripts or online reviews. Example prompt “Combine client testimonials in [table name].”
Enhance data
For a given city name, get the name of the country. Example: “Give me the name of the city in column Y for each zip code in column X.”
Rephrasing
Spelling and grammar in written material, including voice-to-text transcriptions, should be done correctly. “Rephrase column X and add results to column Y” is an example of a prompt.
Feature extraction
Feature extraction is the process of removing important details or terms from lengthy text files, like call transcripts and internet reviews. “Extract city names from column X” is the example given.
Sentiment analysis
Recognize how people feel about particular topics in a text. Example prompt: “Incorporate findings into column Y by extracting sentiment from column X.”
Retrieval-augmented generation (RAG)
Utilizing BigQuery vector search, obtain pertinent data related to a task or question and supply it to a model as context. Use a support ticket, for instance, to locate ten related prior cases that you can pass to a model as context so that it can summarize and offer a solution.
Integrating unstructured data into your Data Cloud is made simpler, easier, and more affordable with BigQuery’s expanded support for cutting-edge foundation models like Vertex AI’s Gemini 1.0 Pro.
Come explore the future of generative AI and data with Google
Refer to the documentation to find out more about these new features. With the help of this tutorial, you can operationalize ML workflows, deploy models, and apply Google’s best-in-class AI models to your data without transferring any BigQuery data. Additionally, you can view a demonstration that shows you how to use BigQuery to build an end-to-end data analytics and AI application that fully utilizes the power of sophisticated models like Gemini, as well as a behind-the-scenes look at the development process. View Google’s most recent webcast on product innovation to find out more about the newest features and how BigQuery ML can be used to create and utilize models with just basic SQL.
Read more on govindhtech.com
#gemini#vertexai#bigquery#generativeai#bigqueryml#geminipro#rag#google#technology#technews#news#govindhtech
0 notes
Text
Gemma open models now available on Google Cloud
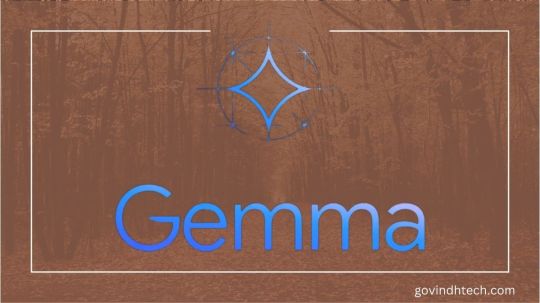
Google today unveiled Gemma, a line of cutting-edge, lightweight open models developed using the same science and technology as the Gemini models. They are happy to announce that Google Cloud users can begin utilizing Gemma open models in Vertex AI for customization and building, as well as for deployment on Google Kubernetes Engine (GKE), right now. Google’s next step in making AI more transparent and available to developers on Google Cloud is the launch of Gemma and their enhanced platform capabilities.
Gemma displays models
The Gemma family of open models is composed of lightweight, cutting-edge models that are constructed using the same technology and research as the Gemini models. Gemma, which was created by Google DeepMind and various other Google teams, was named after the Latin gemma, which means “precious stone.” Gemma was inspired by Gemini AI. Along with their model weights, Google is also making available resources to encourage developer creativity, promote teamwork, and direct the ethical use of Gemma models.
Gemma is currently accessible via Google Cloud
Google-capable Gemini models and Gemma models share infrastructure and technical components. When compared to other open models, this allows Gemma models to achieve best-in-class performance for their sizes. Gemma 2B and Gemma 7B are the two sizes of weights that they are releasing. To facilitate research and development, pre-trained and instruction-tuned variants of each size are released.
Gemma supports frameworks like JAX, PyTorch, Keras 3.0, Hugging Face Transformers, and Colab and Kaggle notebooks tools that Google Cloud developers love and use today. Gemma open models can be used on Google Cloud, a workstation, or a laptop. Developers can now work with and customize Vertex AI and run it on GKE thanks to these new open models. They have worked with NVIDIA to optimize Gemma for NVIDIA GPUs to maximize industry-leading performance.
Gemma is now accessible everywhere in the world. What you should know in particular is this:
The Gemma 2B and Gemma 7B model weights are the two sizes that they are releasing. There are trained and fine-tuned versions available for every size.
Using Gemma, a new Responsible Generative AI Toolkit offers instructions and necessary resources for developing safer AI applications.
Google is offering native Keras 3.0 toolchains for inference and supervised fine-tuning (SFT) across all major frameworks, including PyTorch, TensorFlow, and JAX.
Gemma is simple to get started with thanks to pre-configured Colab and Kaggle notebooks and integration with well-known programs like Hugging Face, MaxText, NVIDIA NeMo, and TensorRT-LLM.
Pre-trained and fine-tuned Gemma open models can be easily deployed on Vertex AI and Google Kubernetes Engine (GKE) and run on your laptop, workstation, or Google Cloud.
Industry-leading performance is ensured through optimization across multiple AI hardware platforms, such as NVIDIA GPUs and Google Cloud TPUs.
All organizations, regardless of size, are permitted to use and distribute the terms of use in a responsible commercial manner.
Unlocking Gemma’s potential in Vertex AI
Gemma has joined more than 130 models in the Vertex AI Model Garden, which now includes the Gemini 1.0 Pro, 1.0 Ultra, and 1.5 Pro models they recently announced expanded access to Gemini.
Developers can benefit from an end-to-end ML platform that makes managing, tuning, and monitoring models easy and intuitive by utilizing Gemma open models on Vertex AI. By utilizing Vertex AI, builders can lower operational costs and concentrate on developing customized Gemma versions that are tailored to their specific use cases.
For instance, developers can do the following with Vertex AI’s Gemma open models:
Create generative AI applications for simple tasks like Q&A, text generation, and summarization.
Utilize lightweight, customized models to facilitate research and development through experimentation and exploration.
Encourage low-latency real-time generative AI use cases, like streaming text
Developers can easily transform their customized models into scalable endpoints that support AI applications of any size with Vertex AI’s assistance.
Utilize Gemma open models on GKE to scale from prototype to production
GKE offers resources for developing unique applications, from basic project prototyping to large-scale enterprise deployment. Developers can now use Gemma to build their generation AI applications for testing model capabilities or constructing prototypes by deploying it directly on GKE:
Use recognizable toolchains to deploy personalized, optimized models alongside apps in portable containers.
Adapt infrastructure configurations and model serving without having to provision or maintain nodes.
Quickly integrate AI infrastructure that can grow to accommodate even the most complex training and inference scenarios.
GKE offers autoscaling, reliable operations environments, and effective resource management. Furthermore, it facilitates the easy orchestration of Google Cloud AI accelerators, such as GPUs and TPUs, to improve these environments and speed up training and inference for generative AI model construction.
Cutting-edge performance at scale
The infrastructure and technical components of Gemma open models are shared with Gemini, their most powerful AI model that is currently accessible to the public. In comparison to other open models, this allows Gemma 2B and 7B to achieve best-in-class performance for their sizes. Additionally, Gemma open models can be used directly on a desktop or laptop computer used by developers. Interestingly, Gemma meets their strict requirements for responsible and safe outputs while outperforming noticeably larger models on important benchmarks. For specifics on modeling techniques, dataset composition, and performance, consult the technical report.
At Google, we think AI should benefit everyone. Google has a long history of developing innovations and releasing them to the public, including JAX, AlphaFold, AlphaCode, Transformers, TensorFlow, BERT, and T5. They are thrilled to present a fresh batch of Google open models today to help researchers and developers create ethical AI.
Begin using Gemma on Google Cloud right now
Working with Gemma models in Vertex AI and GKE on Google Cloud is now possible. Visit ai.google.dev/gemma to access quickstart guides and additional information about Gemma.
Read more on Govindhtech.com
#Gemma#GoogleCloud#vertexai#GoogleKubernetesEngine#GeminiAI#Gemmamodels#NVIDIA#NVIDIAGPUs#GenerativeAIToolkit#geminipro#geminiultra#technews#technology#govindhtech
0 notes
Text
Introducing Gemini 1.5, Google’s next-generation model

Introducing Gemini 1.5 This is an exciting AI time. New advances in AI could help billions of people in the future. Since launching Gemini 1.0, Google has tested, improved, and added features.
Gemini 1.5: Google’s next-generation model Performance is greatly improved in Gemini 1.5. Their approach has changed, incorporating research and engineering innovations into nearly every aspect of foundation model development and infrastructure. A new Mixture-of-Experts (MoE) architecture makes Gemini 1.5 train and serve more efficiently.
First up is Gemini 1.5 Pro, their early testing model. It’s a mid-size multimodal model optimized for scaling across many tasks and performs similarly to Gemini 1.0 Ultra, Google’s largest model. A breakthrough experimental feature in long-context understanding is also introduced.
The standard Gemini 1.5 Pro context window holds 128,000 tokens. AI Studio and Vertex AI are now offering private previews to a select group of developers and enterprise customers with a context window of up to 1 million tokens.
Optimizations to reduce latency, computational requirements, and user experience are underway as they roll out the full 1 million token context window. Google is excited for people to try this breakthrough capability; see below for availability.
More advances in our next-generation models will allow people, developers, and enterprises to create, discover, and build with AI.
A highly efficient architecture, Gemini 1.5 is based on Google’s leading Transformer and MoE research. Instead of one large neural network, MoE models have smaller “expert” neural networks.
MoE models learn to activate only relevant expert pathways in their neural network based on input. This specialization greatly improves model efficiency. Google pioneered deep learning MoE with Sparsely-Gated MoE, GShard-Transformer, Switch-Transformer, M4, and more.
Google’s latest model architecture innovations help Gemini 1.5 learn complex tasks faster, maintain quality, and train and serve more efficiently. These efficiencies are helping their teams iterate, train, and deliver more advanced Gemini faster than ever, and Google is optimizing.
More context, better help Tokens form an AI model’s “context window” for processing information. Tokens can be words, images, videos, audio, or code. A larger context window allows a model to process more information in a prompt, improving its consistency, relevance, and usefulness.
Google’s machine learning innovations have increased 1.5 Pro’s context window capacity beyond Gemini 1.0’s 32,000 tokens. They can now produce 1 million tokens.
This means Gemini 1.5 Pro can process 1 hour of video, 11 hours of audio, 30,000 lines of code, or 700,000 words in one go. Up to 10 million tokens were tested in their research.
Complex reasoning about massive data Gemini 1.5 Pro efficiently analyzes, classifies, and summarizes large amounts of content per prompt. It can reason about conversations, events, and details in the 402-page Apollo 11 transcripts.
Gemini 1.5 Pro can comprehend, reason, and identify intriguing details in Apollo 11’s 402-page transcripts.
Better cross-modal reasoning 1.5 Pro performs advanced understanding and reasoning for video and other modalities. When given a 44-minute silent Buster Keaton film, the model can accurately analyze plot points and events and even reason about small details that could be missed.
Gemini 1.5 Pro can identify a 44-minute silent Buster Keaton film scene from a simple line drawing of a real-life object.
Relevant problem-solving with longer code 1.5 Pro solves more relevant problems across longer code blocks. It can reason across examples, suggest helpful modifications, and explain how different parts of the code work when given a prompt with more than 100,000 lines of code.
Gemini 1.5 Pro can solve, modify, and explain 100,000 lines of code.
Improved performance 1.5 Pro beats 1.0 Pro on 87% of Google’s large language model benchmarks in text, code, image, audio, and video evaluations. It performs similarly to 1.0 Ultra on the same benchmarks.
Gemini 1.5 Pro performs well as its context window grows. 1.5 Pro found a small piece of text containing a fact or statement 99% of the time in Needle In A Haystack (NIAH) evaluations of 1 million data tokens.
Gemini 1.5 Pro also has impressive “in-context learning” skills, learning a new skill from a long prompt without fine-tuning. The Machine Translation from One Book (MTOB) benchmark measures how well the model learns from new information. Given a Kalamang grammar manual, a language with fewer than 200 speakers worldwide, the model learns to translate English to Kalamang similarly to a human.
Since 1.5 Pro’s long context window is unique among large-scale models, Google is constantly creating new evaluations and benchmarks to test its novel capabilities.
Thorough ethics and safety testing Their models undergo rigorous ethics and safety tests following Google’s AI Principles and safety policies. To improve their AI systems, they apply these research findings to governance, model development, and evaluation.
Google teams have refined 1.0 Ultra since December to make it safer for a wider release. They also conducted novel safety risk research and developed red-teaming methods to test for various harms.
Google has prepared 1.5 Pro for responsible deployment by conducting extensive content safety and representational harm testing, as they did for Gemini 1.0. They will continue this testing. Beyond this, they are creating tests for 1.5 Pro’s novel long-context capabilities.
Build and test Gemini models Google aims to responsibly bring new Gemini models to billions of people, developers, and businesses worldwide.
Developers and enterprise customers can preview 1.5 Pro in AI Studio and Vertex AI starting today.
When the model is ready, they will release 1.5 Pro with a 128,000 token context window. Google will soon introduce pricing tiers starting at 128,000 context windows and scaling up to 1 million tokens as they improve the model.
Early testers can try the 1 million token context window for free, but expect longer latency. Significant speed improvements are coming.
Developers can sign up in AI Studio to test 1.5 Pro, while enterprise customers can contact their Vertex AI account team.
FAQ Gemini 1.5 Release Date In reality, Google released the Gemini 1.5 Pro first, then the regular Gemini 1.5 model. This is an explanation: In February 2024, Google reveals and makes available Gemini 1.5 Pro in private preview to select developers and business clients. It boasts performance comparable to the largest model at the time, Gemini 1.0 Ultra, and comes with a context window that holds 128,000 tokens. Google intends to release the standard Gemini 1.5 model for wider use later in 2024 (date to be announced). Its capabilities and the date of release are still unknown.
Read more on Govindhtech.com
0 notes