#Azure Database
Explore tagged Tumblr posts
Text
SQL Server deadlocks are a common phenomenon, particularly in multi-user environments where concurrency is essential. Let's Explore:
https://madesimplemssql.com/deadlocks-in-sql-server/
Please follow on FB: https://www.facebook.com/profile.php?id=100091338502392
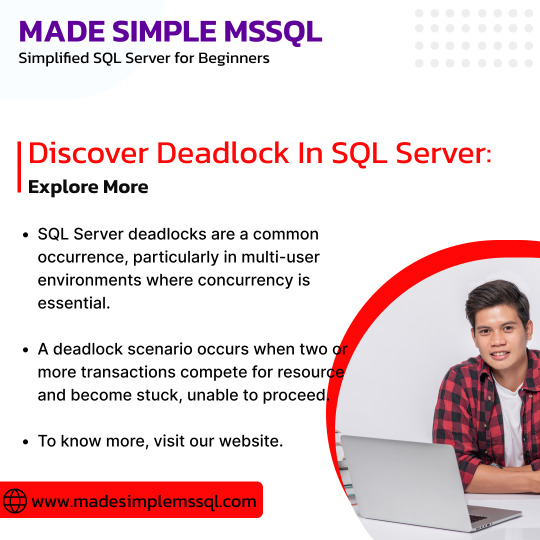
#technews#microsoft#sqlite#sqlserver#database#sql#tumblr milestone#vpn#powerbi#data#madesimplemssql#datascience#data scientist#datascraping#data analytics#dataanalytics#data analysis#dataannotation#dataanalystcourseinbangalore#data analyst training#microsoft azure
5 notes
·
View notes
Text
Introduction to Data Governance in Azure SQL Database
This webinar will cover how having a Data Governance framework in place will support and fortify your efforts to becoming a Data … source
0 notes
Text
What’s New In Databricks? February 2025 Updates & Features Explained!
youtube
What’s New In Databricks? February 2025 Updates & Features Explained! #databricks #spark #dataengineering
Are you ready for the latest Databricks updates in February 2025? 🚀 This month brings game-changing features like SAP integration, Lakehouse Federation for Teradata, Databricks Clean Rooms, SQL Pipe, Serverless on Google Cloud, Predictive Optimization, and more!
✨ Explore Databricks AI insights and workflows—read more: / databrickster
🔔𝐃𝐨𝐧'𝐭 𝐟𝐨𝐫𝐠𝐞𝐭 𝐭𝐨 𝐬𝐮𝐛𝐬𝐜𝐫𝐢𝐛𝐞 𝐭𝐨 𝐦𝐲 𝐜𝐡𝐚𝐧𝐧𝐞𝐥 𝐟𝐨𝐫 𝐦𝐨𝐫𝐞 𝐮𝐩𝐝𝐚𝐭𝐞𝐬. / @hubert_dudek
🔗 Support Me Here! ☕Buy me a coffee: https://ko-fi.com/hubertdudek
🔗 Stay Connected With Me. Medium: / databrickster
==================
#databricks#bigdata#dataengineering#machinelearning#sql#cloudcomputing#dataanalytics#ai#azure#googlecloud#aws#etl#python#data#database#datawarehouse#Youtube
1 note
·
View note
Text
Unlocking Efficient Database Migration with Azure
Learn key strategies and best practices for migrating databases to Microsoft Azure. It covers Azure’s tools, such as Azure Database Migration Service, and guides minimizing downtime, ensuring data integrity, and optimizing performance during migration. For more information, see this article.
0 notes
Text
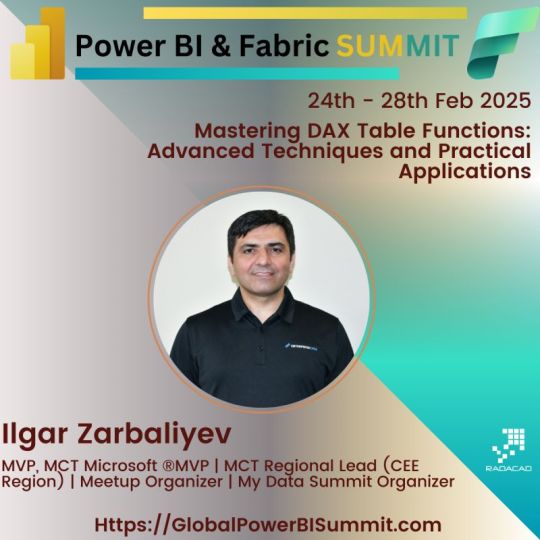
#DataAnalytics#Analytics#Conference#Training#DataWarehousing#Azure#Database#BusinessIntelligence#Realtime#Microsoft#Fabric#DataPlatform#DataEngineering#SQL#Reporting#Insights#Visualization#DAX#PowerQuery#Administration#DBA#DataScience#MachineLearning#AI#MicrosoftAI#Architecture#BestPractices
0 notes
Text
SQL Programming Development Company in the USA
LDS Engineer: Premier SQL Programming Development Company in the USA LDS Engineer stands as one of the leading SQL programming development companies in the USA providing exceptional Information base Answers to Customers across the globe. Our party has a stacked amp sound report for delivering top-tier SQL scheduling services to businesses of all sizes and industries. With a dedicated team of…
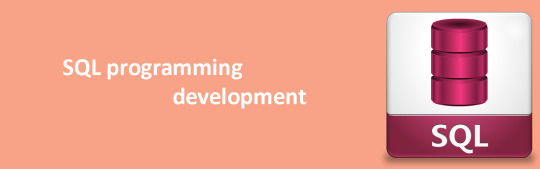
View On WordPress
0 notes
Text
Understanding the Risks of SQL Server NOLOCK
Admittedly, I use NOLOCK all the time in my queries. But in my defense, most of the queries that I write ad-hoc are returning information that I’m not that concerned about. Using the NOLOCK table hint in SQL Server can have significant implications, both positive and negative, depending on the use case. While it is commonly used to improve performance by avoiding locks on a table, it has several…
0 notes
Text
What’s Slowing Down Your Database? Find Out How to Fix It Fast!
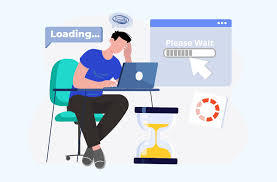
Is your database running slower than it should? A sluggish database can hurt your business in many ways—from delayed decisions to frustrated customers. The good news? You can fix it fast with database performance optimization!
Why Is My Database Slow?
Here are the main reasons your database might be lagging:
Poor Query Performance – Inefficient queries can slow down data retrieval.
Lack of Indexing – Without proper indexing, your database struggles to find data quickly.
Overloaded Servers – Too much traffic can overwhelm your servers and slow performance.
Fragmentation – Over time, data fragmentation can cause slower read and write times.
Outdated Hardware – Old servers may not be able to handle your database's needs.
How to Fix It Fast
Optimize Queries – Rewrite inefficient queries and use proper indexing to speed things up.
Rebuild Indexes – Regular indexing can help improve data retrieval speed.
Monitor Servers – Keep an eye on server performance and upgrade as needed.
Defragment Your Database – Regular maintenance helps avoid slowdowns.
Cloud Migration – Move to the cloud for better scalability and performance.
Don’t let a slow database hold your business back. At KLUSTERFIRST, we specialize in optimizing database performance. Contact us today to make sure your data is working for you at its best!
#azure#databasemanagement#microsoft#cloudcomputing#business#website optimization#infrastructure#cloud migration#all india database provider
0 notes
Text
SQL Server database mail is a key component of good communication. Let’s explore the world of database mail: https://madesimplemssql.com/sql-server-database-mail/
Please follow Us on Facebook: https://www.facebook.com/profile.php?id=100091338502392
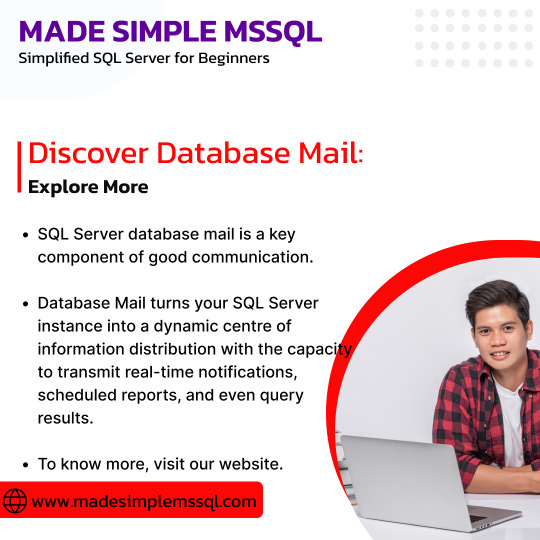
#technews#microsoft#sqlite#sqlserver#database#sql#tumblr milestone#vpn#powerbi#data#madesimplemssql#azure#sqlskills#sql_help
3 notes
·
View notes
Text
Azure SQL Database Tutorial | Relational databases in Azure
Azure SQL Database is one of the key data storage solution for structured data in Azure. If you need to manage structured data via … source
0 notes
Text
Master Data Science, AI, and ChatGPT: Hyderabad's Top Training Destinations
Naresh i Technologies
✍️Enroll Now: https://bit.ly/3xAUmxL
👉Attend a Free Demo On Full Stack Data Science & AI by Mr. Prakash Senapathi.
📅Demo On: 22nd April @ 5:30 PM (IST)
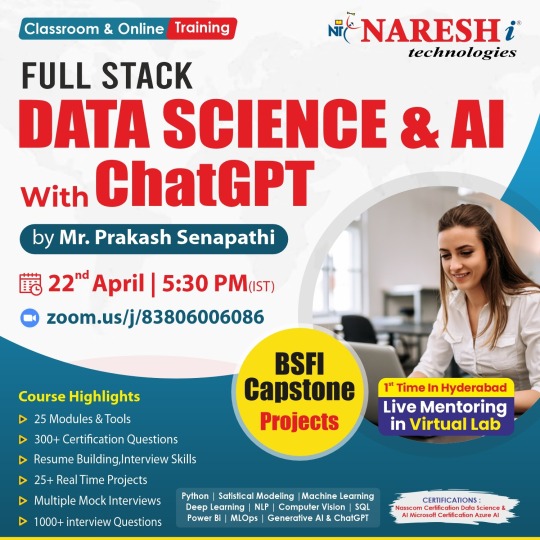
"Explore the Fusion of Data Science, AI, and ChatGPT in Hyderabad's top training programs. Dive into hands-on learning, mastering analytics, machine learning, and natural language processing. Elevate your skills and unlock limitless possibilities in the realm of intelligent technologies."
#Fullstackdatascience#ai#machinelearning#python#chatgpt#sql#Database#Dataanalyst#it#azure#onlinetraining#classroomtraining#education#software#datascience#datasciencetraining#datasciencecourse#datasciencejobs#datascientists#datasciencelearning#datasciencebootcamp#nareshit
1 note
·
View note
Text
Transform your data management experience with Azure Database Services. Harness the scalability, reliability, and flexibility of Azure Cloud databases to streamline operations and organize, access, and optimize your business data. Tap the link to know more: https://www.qservicesit.com/azure-databases/
#microsoftazure#azure software development#azure app development#azure database services#azure database migration service
0 notes
Text
Supercharge Your SQL Server Performance with Premium SSD v2 Storage on Azure VMs
Introduction When it comes to running SQL Server in the cloud, storage performance is key. You want your queries to run lightning fast, but you also need high availability and scalability without breaking the bank. That’s where Azure’s new Premium SSD v2 managed disks come in. In this article, I’ll share what I’ve learned and show you how Premium SSD v2 can take your SQL Server workloads on…
View On WordPress
0 notes
Text
How to Install Azure DevOps Server 2022

View On WordPress
#Azure#Azure CI/CD#Azure DevOps#Azure DevOps Server 2022#Community Edition#Database#Porject Collection#Project#SQL Database#Team Project Collection#Windows#Windows Server
0 notes
Text
Database Migration to Azure: best practices and strategies
Data is growing exponentially, and so are the demands of the modern market. More and more enterprises realize that their present infrastructure is inadequate to fulfil the needs of the modern market. Migrating to modern systems, from on-premises set-ups to cloud-based systems, has become the most preferred choice.
0 notes
Text
I'm Speaking at DataWeekender 6.5!
Unfortunately, the Data TLV summit was delayed. But I still have some good news: I'll be speaking at #DataWeekender 6.5 on November 11, and I will be delivering a brand new session! ✨ It's next week! Register now! #Microsoft #SQLServer #MadeiraData
Unfortunately, the Data TLV summit was delayed. But I still have some good news: I’ll be speaking at #DataWeekender 6.5 on November 11, and I will be delivering a brand new session! ✨ Continue reading Untitled
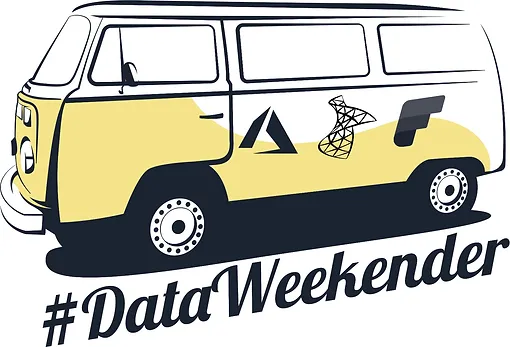
View On WordPress
0 notes