#Apache beam
Explore tagged Tumblr posts
Text
There was both a book and a movie about the abduction experience.
From the essay:
Travis Walton’s 1975 abduction in Arizona’s Apache-Sitgreaves National Forest is a well-known UFO case. After a beam struck him, Walton woke up on an examination table surrounded by non-human beings. Despite controversy and doubt, UFO experts find the account consistent with other abductions, making it a significant study in UFO phenomena.
14 notes
·
View notes
Text

there are other abduction cases involving the mutilation of animals by beings that don’t look like the cat-eyed beings. In fact, what appear to be the Controllers of smaller entities are often tall humanoids, sometimes seen in long, white robes, even with hoods over their heads. Government documents have described smaller beings referred to as “extraterrestrial biological entities,��� or EBEs, and another group called the “Talls.” Some people in the human abduction syndrome think the EBEs and the Talls are at war with each other — but not with bullets. The impression is that these E. T.s war through deceptive mind control and manipulation of time lines.
Perhaps deception and time warps are why there is so much confusion in the high strangeness of encounters with Other Intelligences, the variety of non-human physical appearances, and lack of consistent communication by the entities about who they are, where they are from, and why they are on planet Earth lifting people from cars and bedrooms, or animals from backyards and pastures in beams of light.
While Judy Doraty’s May 1973 encounter with her teenage daughter near a pasture outside Houston, Texas, involved the cat-eyed beings and mutilation of a calf on board the craft in front of Judy, there was another abduction experience seven years later in the first week of May 1980 near a Cimarron, New Mexico, pasture.Purple map pointer marks Cimarron, New Mexico, northwest of Taos. Santa Fe and Los Alamos are marked by larger red circles in lower left of map while all the other red circles mark places of multiple animal mutilations in the Jicarilla Apache Indian Reservation, Dulce, Chama, Espanola, Questa, Taos, Las Vegas and Raton, New Mexico. Across the northern border into Colorado, other red circles at multiple mutilation sites are in Pagosa Springs, Alamosa, Walsenburg and Trinidad. The first worldwide-reported mutilation case was a mare named Lady found in September 1967, near Alamosa, Colorado, dead and stripped of flesh from the chest up and all the chest organs surgically removed.Lady, a 3-year-old Appaloosa mare, owned by Nellie and Berle Lewis, who had a ranch in the San Luis Valley of southern Colorado near Alamosa. Lady was found September 8, 1967, dead and bloodlessly stripped of flesh from the neck up. All her chest organs had also been “surgically” removed, according to John Altshuler, M. D. who examined the mutilated horse. Lady’s hoof tracks stopped about 100 feet southeast of her body where it looked like she had jumped around in a circle as if trying to escape something. There were no tracks around Lady’s body, but 40 feet south of her was a broken bush. Around the bush was a 3-foot-diameter circle of 6 or 8 holes in the ground about 4 inches across and 3 to 4 inches deep. Photograph taken three weeks after Lady’s death by Don Anderson.
Posted on December 30, 2022 © 2023 by Linda Moulton Howe
Part 2: Hall of Mirrors with A Quicksand Floor
“The brightest, whitest light I’ve ever seen. How can it fly like that? What is it? Oh, I’m scared. How can they be doing that — killing that cow? It’s not even dead! It’s alive!”
– Female abductee at cattle mutilation site, Cimarron, NM, May 1980
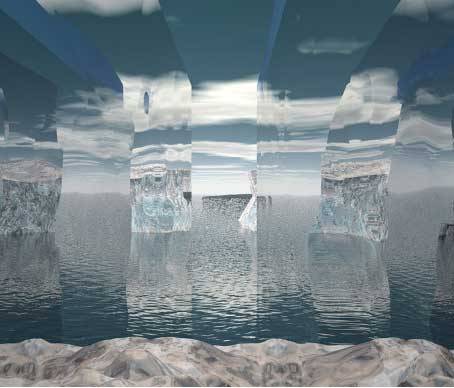
Return to Part 1.

But there are other abduction cases involving the mutilation of animals by beings that don’t look like the cat-eyed beings. In fact, what appear to be the Controllers of smaller entities are often tall humanoids, sometimes seen in long, white robes, even with hoods over their heads. Government documents have described smaller beings referred to as “extraterrestrial biological entities,” or EBEs, and another group called the “Talls.” Some people in the human abduction syndrome think the EBEs and the Talls are at war with each other — but not with bullets. The impression is that these E. T.s war through deceptive mind control and manipulation of time lines.
Perhaps deception and time warps are why there is so much confusion in the high strangeness of encounters with Other Intelligences, the variety of non-human physical appearances, and lack of consistent communication by the entities about who they are, where they are from, and why they are on planet Earth lifting people from cars and bedrooms, or animals from backyards and pastures in beams of light.

The following excerpts are from May 1980 hypnosis sessions with a young boy and his mother who saw humanoids mutilating a cow in a Cimarron pasture followed by an abduction of them both. The hypnosis sessions began on May 11, 1980, when Leo Sprinkle, Director of Counseling and Testing at the University of Wyoming, received a phone call from scientist Paul Bennewitz, who was investigating the mother and son abduction for the Aerial Phenomenon Research Organization (APRO).
3 notes
·
View notes
Text
Why do all BS-4 bikes have a headlight? What is the logic of a daytime running light?
BS-4 (Bharat Stage 4) motorcycles are required to be equipped with headlights that are always on (cannot be turned off). This design is mainly based on the upgrade of safety regulations and the logic of traffic accident prevention. The following is a specific analysis:
1. Reasons for the mandatory turning on of BS-4 motorcycle headlights
1. India's AIS-065 safety regulations
BS-4 was implemented in 2017 and took effect simultaneously: it requires motorcycle headlights to be designed to be automatically turned on (Always On) and the manual off function is cancelled.
Purpose: Improve the visibility of motorcycles during the day through all-weather lighting (motorcycles are small in size and the accident mortality rate is 26 times that of cars).
2. Reduce daytime collision accidents
Data support: According to statistics from the Indian Ministry of Roads, turning on headlights during the day can reduce motorcycle accidents by 27% (pilot data from 2015-2020).
International precedent: The European Union has required motorcycle headlights to be always on since 2003, and Thailand, Brazil and other countries have followed suit.
3. Synergy with BS-4 emission upgrades
BS-4 standards not only limit emissions (CO≤1.0g/km), but also integrate safety performance upgrades (such as OBD-II, ABS and lighting specifications).
II. The logic of daytime running lights (DRL)
1. Core functions
Enhanced visibility: DRL uses a low-energy, high-brightness light source (usually LED) to outline the vehicle during the day, reducing the risk of oncoming vehicles misjudging the distance.
Non-lighting use: Unlike nighttime headlights, DRLs do not need to illuminate the road, but only need to indicate their existence.
2. The particularity of motorcycle DRLs
Integrated design: The BS-4 motorcycle headlights are essentially reused low-beam lights as DRLs, with the brightness reduced to 30% (such as Hero Splendor iSmart) to reduce energy consumption.
Regulatory requirements: AIS-065 stipulates that the power of the headlight in daytime mode must be ≤10W (LED) or ≤15W (halogen).
III. Examples of BS-4 motorcycle lighting systems
Vehicle type Headlight type Daytime mode brightness Regulatory adaptation solution
Bajaj Pulsar 150 Halogen headlight (55W) Power reduction to 15W Resistor current limiting + normally open circuit
TVS Apache RTR 160 LED headlight (10W) Full light (10W) Dedicated DRL module + light sensor automatic switching
IV. Disputes and user responses
1. Common complaints
Battery consumption: Old halogen lamp models (such as Honda CB Shine) may reduce battery life by 20% due to constant light.
Maintenance cost: The replacement cost of a damaged LED module is high (about ₹800–1500 vs ₹100–300 for halogen lamps).
2. Solution
Upgrade LEDs: Use low-power LED headlights (such as Osram Night Breaker LED).
Install DRLs: Add independent LED light strips to the bumper (must comply with AIS-049 standards).
V. Global Trend Comparison
Country Motorcycle Lighting Regulations Accident Reduction Effect
India BS-4 + AIS-065 (headlights always on) 27%
EU ECE R53 (DRL mandatory) 31%
Thailand Daytime Headlights (mandatory in 2014) 22%
Summary
The mandatory headlights for motorcycles under BS-4 are a policy choice that prioritizes safety over convenience, aiming to reduce the daytime accident rate through simple, crude but effective means. Although it sacrifices the freedom of manual control by users, data proves that its social benefits are significant. In the future, with the popularization of LEDs and the development of intelligent lighting systems (such as adaptive DRLs), the contradiction between energy consumption and safety will be further alleviated.
#led lights#car lights#led car light#youtube#led auto light#led headlights#led light#led headlight bulbs#ledlighting#young artist#daytime running light#motorcycles#motorbike#moto#car#cars#headlight bulb#headlamp#headlight
0 notes
Text
VR Project (Blog 06: Modular Pieces Creation) [Assignment set by Apache]
As I am the Environment artist, I have taken upon myself to create the modular pieces for the castle interior.
Figure 1: Interior modular pieces development.
I find several references of European castles as it is a castle located in Poland. I have notice the interior is obviously made of individual stone pieces and the ceiling is wooden with supportive horizontal wooden beams. Due to time constraints, other assignment's demanding due dates and manpower shortage, not much heavily detailed research was carried out.
Figure 2: 19 Individual Modular Pieces for main room (Top). Figure 3: 6 Individual Modular Pieces for the Basilicks' dungeon (Bottom).
After identifying the individual parts that are required to give the feeling of a medieval a castle interior, 19 individual unique pieces were modelled and UV unwrapped. This follows the update no.4 modelling method.
Figure 3: Optimisation modelling step.
Polygon faces that are not visible to the player, such as those facing outward or hidden behind other models, were removed to ensure they are not rendered in Unreal Engine. This helps improve performance by reducing unnecessary processing of unseen geometry.
Figure 4: Environment Blockout
The environment was briefly blocked out in the Blender file to ensure all measurements were accurate. This included importing Akshat's models to check whether they fit well within the environment space. If any dimensional issues were found in his models, I could inform him immediately during this stage. This workflow allowed me to make quick adjustments and reinsert the corrected models into the blockout to verify scale and believability, avoiding the need to import into Unreal Engine only to discover issues that would require reimporting.
Figure 5: Texturing in Substance Painter and Unreal Engine.
An example of the texturing approach can be seen in Figure 4, where the upper and lower sections of the wall were textured in Substance Painter. The central stone wall area was left untextured so that a seamless stone texture from the Fab library could be applied later.
Previously, I was not very efficient in creating modular pieces, often making assets that were only used once or splitting models unnecessarily when they could have been combined. Through this assignment, I had the opportunity to improve my modular modelling skills, ensuring that many of the pieces I created were reused multiple times throughout the project and were further optimised.
0 notes
Text
Mastering Data Pipelines with Apache Beam: A Pragmatic Approach
Introduction Mastering Data Pipelines with Apache Beam: A Pragmatic Approach is a comprehensive guide to building data pipelines using Apache Beam, a Python-based unified programming model for both batch and streaming data processing. In this tutorial, we will cover the core concepts, implementation, and best practices for building efficient and scalable data pipelines using Beam. This tutorial…
0 notes
Text
Building Real-Time Data Pipelines: Key Tools and Best Practices
As the demand for immediate insights grows across industries, real-time data pipelines are essential in modern data engineering. Unlike batch processing, which handles data at scheduled intervals, real-time pipelines process data continuously, enabling organizations to respond instantly to new information and events. Constructing these pipelines effectively requires the right tools, approaches, and industry best practices. Timely insights can be delivered by data engineers who can build robust, real-time data pipelines that deliver the insights effectively.
Choosing the Right Tools for Real-Time Data Processing
Building a real-time pipeline starts with selecting tools that can handle high-speed data ingestion and processing. Apache Kafka, a popular event streaming platform, manages vast amounts of data by distributing messages across multiple brokers, making it scalable. For stream processing, tools like Apache Flink and Spark Structured Streaming process data with low latency. Combining these tools allows data engineers to build flexible, adaptive pipelines that support complex processing requirements. Seamless integration between these tools reduces development time and ensures smooth data flow, allowing engineers to deliver value faster.
Defining Data Transformation and Processing Stages
After data ingestion, the next step is transforming it into a usable format. Real-time pipelines require transformations that clean, filter, and enrich data in motion. Tools like Apache Beam and AWS Lambda offer flexible options for real-time transformation. Apache Beam’s unified model works across systems like Flink and Spark, simplifying scalable transformations. Defining clear processing stages, such as aggregating for analytics or filtering for anomaly detection, ensures data is processed accurately for real-time delivery to users or applications. With these stages in place, engineers can optimize data flow at every step.
Ensuring Data Quality and Reliability
In real-time systems, data quality is critical, as errors can quickly compound. Data engineers should incorporate automated validation and error-handling mechanisms to maintain quality. Tools like Great Expectations enable customizable data validation, while Apache Druid offers real-time data monitoring. Error-handling strategies, such as retries and dead-letter queues, allow the pipeline to continue even if certain inputs fail. Managing data quality prevents errors from affecting downstream applications, ensuring insights remain accurate. These measures are crucial for maintaining trust in the pipeline’s outputs.
Monitoring and Optimizing Pipeline Performance
Monitoring ensures that real-time data pipelines run smoothly. Tools like Prometheus and Grafana track pipeline performance, measuring latency, throughput, and resource use. This helps engineers identify bottlenecks early on, such as ingestion slowdowns or increased processing loads. Optimizing performance may involve adjusting resources, fine-tuning partitioning, or scaling resources based on demand. Proactive monitoring and optimization keep data moving efficiently, reducing delays and improving responsiveness. Continuous performance checks enable data engineers to meet evolving business needs with ease.
Building Effective Real-Time Data Pipelines for Added Efficiency
Creating efficient real-time data pipelines requires a strategic approach to data ingestion, processing, and monitoring. By leveraging tools like Apache Kafka, Flink, and Great Expectations, data engineers can build high-quality pipelines for real-time insights. Web Age Solutions provides specialized real-time data engineering courses, helping professionals build responsive data pipelines and enabling organizations to remain agile and data-driven in today’s fast-paced landscape.
For more information visit: https://www.webagesolutions.com/courses/data-engineering-training
0 notes
Text
Apache Beam For Beginners: Building Scalable Data Pipelines
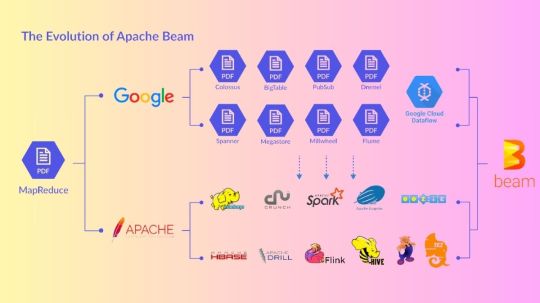
Apache Beam
Apache Beam, the simplest method for streaming and batch data processing. Data processing for mission-critical production workloads can be written once and executed anywhere.
Overview of Apache Beam
An open source, consistent approach for specifying batch and streaming data-parallel processing pipelines is called Apache Beam. To define the pipeline, you create a program using one of the open source Beam SDKs. One of Beam’s supported distributed processing back-ends, such as Google Cloud Dataflow, Apache Flink, or Apache Spark, then runs the pipeline.
Beam is especially helpful for situations involving embarrassingly parallel data processing, where the issue may be broken down into numerous smaller data bundles that can be handled separately and concurrently. Beam can also be used for pure data integration and Extract, Transform, and Load (ETL) activities. These operations are helpful for loading data onto a new system, converting data into a more suitable format, and transferring data between various storage media and data sources.Image credit to Apache Beam
How Does It Operate?
Sources of Data
Whether your data is on-premises or in the cloud, Beam reads it from a wide range of supported sources.
Processing Data
Your business logic is carried out by Beam for both batch and streaming usage cases.
Writing Data
The most widely used data sinks on the market receive the output of your data processing algorithms from Beam.
Features of Apache Beams
Combined
For each member of your data and application teams, a streamlined, unified programming model for batch and streaming use cases.
Transportable
Run pipelines across several execution contexts (runners) to avoid lock-in and provide flexibility.
Wide-ranging
Projects like TensorFlow Extended and Apache Hop are built on top of Apache Beam, demonstrating its extensibility.
Open Source
Open, community-based support and development to help your application grow and adapt to your unique use cases.
Apache Beam Pipeline Runners
The data processing pipeline you specify with your Beam program is converted by the Beam Pipeline Runners into an API that works with the distributed processing back-end of your choosing. You must designate a suitable runner for the back-end where you wish to run your pipeline when you run your Beam program.
Beam currently supports the following runners:
The Direct Runner
Runner for Apache Flink Apache Flink
Nemo Runner for Apache
Samza the Apache A runner Samza the Apache
Spark Runner for Apache Spark by Apache
Dataflow Runner for Google Cloud Dataflow on Google Cloud
Jet Runner Hazelcast Jet Hazelcast
Runner Twister 2
Get Started
Get Beam started on your data processing projects.
Visit our Getting started from Apache Spark page if you are already familiar with Apache Spark.
As an interactive online learning tool, try the Tour of Beam.
For the Go SDK, Python SDK, or Java SDK, follow the Quickstart instructions.
For examples that demonstrate different SDK features, see the WordCount Examples Walkthrough.
Explore our Learning Resources at your own speed.
on detailed explanations and reference materials on the Beam model, SDKs, and runners, explore the Documentation area.
Learn how to run Beam on Dataflow by exploring the cookbook examples.
Contribute
The Apache v2 license governs Beam, a project of the Apache Software Foundation. Contributions are highly valued in the open source community of Beam! Please refer to the Contribute section if you would want to contribute.
Apache Beam SDKs
Whether the input is an infinite data set from a streaming data source or a finite data set from a batch data source, the Beam SDKs offer a uniform programming model that can represent and alter data sets of any size. Both bounded and unbounded data are represented by the same classes in the Beam SDKs, and operations on the data are performed using the same transformations. You create a program that specifies your data processing pipeline using the Beam SDK of your choice.
As of right now, Beam supports the following SDKs for specific languages:
Java SDK for Apache Beam Java
Python’s Apache Beam SDK
SDK Go for Apache Beam Go
Apache Beam Python SDK
A straightforward yet effective API for creating batch and streaming data processing pipelines is offered by the Python SDK for Apache Beam.
Get started with the Python SDK
Set up your Python development environment, download the Beam SDK for Python, and execute an example pipeline by using the Beam Python SDK quickstart. Next, learn the fundamental ideas that are applicable to all of Beam’s SDKs by reading the Beam programming handbook.
For additional details on specific APIs, consult the Python API reference.
Python streaming pipelines
With Beam SDK version 2.5.0, the Python streaming pipeline execution is possible (although with certain restrictions).
Python type safety
Python lacks static type checking and is a dynamically typed language. In an attempt to mimic the consistency assurances provided by real static typing, the Beam SDK for Python makes use of type hints both during pipeline creation and runtime. In order to help you identify possible issues with the Direct Runner early on, Ensuring Python Type Safety explains how to use type hints.
Managing Python pipeline dependencies
Because the packages your pipeline requires are installed on your local computer, they are accessible when you execute your pipeline locally. You must, however, confirm that these requirements are present on the distant computers if you wish to run your pipeline remotely. Managing Python Pipeline Dependencies demonstrates how to enable remote workers to access your dependencies.
Developing new I/O connectors for Python
You can develop new I/O connectors using the flexible API offered by the Beam SDK for Python. For details on creating new I/O connectors and links to implementation guidelines unique to a certain language, see the Developing I/O connectors overview.
Making machine learning inferences with Python
Use the RunInference API for PyTorch and Scikit-learn models to incorporate machine learning models into your inference processes. You can use the tfx_bsl library if you’re working with TensorFlow models.
The RunInference API allows you to generate several kinds of transforms since it accepts different kinds of setup parameters from model handlers, and the type of parameter dictates how the model is implemented.
An end-to-end platform for implementing production machine learning pipelines is called TensorFlow Extended (TFX). Beam has been integrated with TFX. Refer to the TFX user handbook for additional details.
Python multi-language pipelines quickstart
Transforms developed in any supported SDK language can be combined and used in a single multi-language pipeline with Apache Beam. Check out the Python multi-language pipelines quickstart to find out how to build a multi-language pipeline with the Python SDK.
Unrecoverable Errors in Beam Python
During worker startup, a few typical mistakes might happen and stop jobs from commencing. See Unrecoverable faults in Beam Python for more information on these faults and how to fix them in the Python SDK.
Apache Beam Java SDK
A straightforward yet effective API for creating batch and streaming parallel data processing pipelines in Java is offered by the Java SDK for Apache Beam.
Get Started with the Java SDK
Learn the fundamental ideas that apply to all of Beam’s SDKs by beginning with the Beam Programming Model.
Further details on specific APIs can be found in the Java API Reference.
Supported Features
Every feature that the Beam model currently supports is supported by the Java SDK.
Extensions
A list of available I/O transforms may be found on the Beam-provided I/O Transforms page.
The following extensions are included in the Java SDK:
Inner join, outer left join, and outer right join operations are provided by the join-library.
For big iterables, sorter is a scalable and effective sorter.
The benchmark suite Nexmark operates in both batch and streaming modes.
A batch-mode SQL benchmark suite is called TPC-DS.
Euphoria’s Java 8 DSL for BEAM is user-friendly.
There are also a number of third-party Java libraries.
Java multi-language pipelines quickstart
Transforms developed in any supported SDK language can be combined and used in a single multi-language pipeline with Apache Beam. Check out the Java multi-language pipelines quickstart to find out how to build a multi-language pipeline with the Java SDK.
Read more on govindhtech.com
#ApacheBeam#BuildingScalableData#Pipelines#Beginners#ApacheFlink#SourcesData#ProcessingData#WritingData#TensorFlow#OpenSource#GoogleCloud#ApacheSpark#ApacheBeamSDK#technology#technews#Python#machinelearning#news#govindhtech
0 notes
Text
Mastering Data Flow in GCP: A Complete Guide
1. Introduction
Overview of Data Flow in GCP
In the modern digital age, the volume of data generated by businesses and applications is growing at an unprecedented rate. Managing, processing, and analyzing this data in real-time or in batch jobs has become a key factor in driving business insights and competitive advantages. Google Cloud Platform (GCP) offers a suite of tools and services to address these challenges, with Dataflow standing out as one of the most powerful tools for building and managing data pipelines.
Data Flow in GCP refers to the process of collecting, processing, and analyzing large volumes of data in a streamlined and scalable way. This process is critical for businesses that require fast decision-making, accurate data analysis, and the ability to handle both real-time streams and batch processing. GCP Dataflow provides a fully-managed, cloud-based solution that simplifies this entire data processing journey.
As part of the GCP ecosystem, Dataflow integrates seamlessly with other services like Google Cloud Storage, BigQuery, and Cloud Pub/Sub, making it an integral component of GCP's data engineering and analytics workflows. Whether you need to process real-time analytics or manage ETL pipelines, GCP Dataflow enables you to handle large-scale data workloads with efficiency and flexibility.
What is Dataflow?
At its core, Dataflow is a managed service for stream and batch processing of data. It leverages the Apache Beam SDK to provide a unified programming model that allows developers to create robust, efficient, and scalable data pipelines. With its serverless architecture, Dataflow automatically scales up or down depending on the size of the data being processed, making it ideal for dynamic and unpredictable workloads.
Dataflow stands out for several reasons:
It supports streaming data processing, which allows you to handle real-time data in an efficient and low-latency manner.
It also excels in batch data processing, offering powerful tools for running large-scale batch jobs.
It can be used to build ETL pipelines that extract, transform, and load data into various destinations, such as BigQuery or Google Cloud Storage.
Its integration with GCP services ensures that you have a complete ecosystem for building data-driven applications.
The importance of Data Flow in GCP is that it not only provides the infrastructure for building data pipelines but also handles the complexities of scaling, fault tolerance, and performance optimization behind the scenes.
2. What is Dataflow in GCP?
Dataflow Overview
GCP Dataflow is a cloud-based, fully-managed service that allows for the real-time processing and batch processing of data. Whether you're handling massive streaming datasets or processing huge data volumes in batch jobs, Dataflow offers an efficient and scalable way to transform and analyze your data. Built on the power of Apache Beam, Dataflow simplifies the development of data processing pipelines by providing a unified programming model that works across both stream and batch processing modes.
One of the key advantages of Dataflow is its autoscaling capability. When the workload increases, Dataflow automatically provisions additional resources to handle the load. Conversely, when the workload decreases, it scales down resources, ensuring you only pay for what you use. This is a significant cost-saving feature for businesses with fluctuating data processing needs.
Key Features of GCP Dataflow
Unified Programming Model: Dataflow utilizes the Apache Beam SDK, which provides a consistent programming model for stream and batch processing. Developers can write their code once and execute it across different environments, including Dataflow.
Autoscaling: Dataflow automatically scales the number of workers based on the current workload, reducing manual intervention and optimizing resource utilization.
Dynamic Work Rebalancing: This feature ensures that workers are dynamically assigned tasks based on load, helping to maintain efficient pipeline execution, especially during real-time data processing.
Fully Managed: Dataflow is fully managed, meaning you don’t have to worry about infrastructure, maintenance, or performance tuning. GCP handles the heavy lifting of managing resources, freeing up time to focus on building and optimizing pipelines.
Integration with GCP Services: Dataflow integrates seamlessly with other Google Cloud services such as BigQuery for data warehousing, Cloud Pub/Sub for messaging and ingestion, and Cloud Storage for scalable storage. This tight integration ensures that data flows smoothly between different stages of the processing pipeline.
Comparison with Other GCP Services
While Dataflow is primarily used for processing and analyzing streaming and batch data, other GCP services also support similar functionalities. For example, Cloud Dataproc is another option for data processing, but it’s specifically designed for running Apache Hadoop and Apache Spark clusters. BigQuery, on the other hand, is a data warehousing service but can also perform real-time analytics on large datasets.
In comparison, GCP Dataflow is more specialized for streamlining data processing tasks with minimal operational overhead. It provides a superior balance of ease of use, scalability, and performance, making it ideal for both developers and data engineers who need to build ETL pipelines, real-time data analytics solutions, and other complex data processing workflows
3. Data Flow Architecture in GCP
Key Components of Dataflow Architecture
The architecture of GCP Dataflow is optimized for flexibility, scalability, and efficiency in processing both streaming and batch data. The key components in a Dataflow architecture include:
Pipeline: A pipeline represents the entire data processing workflow. It is composed of various steps, such as transformations, filters, and aggregations, that process data from source to destination.
Workers: These are virtual machines provisioned by Dataflow to execute the tasks defined in the pipeline. Workers process the data in parallel, allowing for faster and more efficient data handling. GCP Dataflow automatically scales the number of workers based on the complexity and size of the job.
Sources: The origin of the data being processed. This can be Cloud Pub/Sub for real-time streaming data or Cloud Storage for batch data.
Transforms: These are the steps in the pipeline where data is manipulated. Common transforms include filtering, mapping, grouping, and windowing.
Sinks: The destination for the processed data. This can be BigQuery, Cloud Storage, or any other supported output service. Sinks are where the final processed data is stored for analysis or further use.
How Dataflow Works in GCP
GCP Dataflow simplifies data pipeline management by taking care of the underlying infrastructure, autoscaling, and resource allocation. The process of setting up and running a data pipeline on Dataflow typically follows these steps:
Pipeline Creation: A pipeline is created using the Apache Beam SDK, which provides a unified model for both batch and stream data processing. Developers define a pipeline using a high-level programming interface that abstracts away the complexity of distributed processing.
Ingesting Data: The pipeline starts by ingesting data from sources like Cloud Pub/Sub for streaming data or Cloud Storage for batch data. GCP Dataflow can handle both structured and unstructured data formats, making it versatile for different use cases.
Applying Transformations: Dataflow pipelines apply a series of transformations to the ingested data. These transformations can include data filtering, aggregation, joining datasets, and more. For example, you might filter out irrelevant data or aggregate sales data based on location and time.
Processing the Data: Once the pipeline is set, Dataflow provisions the necessary resources and begins executing the tasks. It automatically scales up resources when data volume increases and scales down when the load decreases, ensuring efficient resource usage.
Outputting Data: After processing, the transformed data is written to its final destination, such as a BigQuery table for analytics, Cloud Storage for long-term storage, or even external databases. Dataflow supports multiple sink types, which makes it easy to integrate with other systems in your architecture.
Understanding Apache Beam in Dataflow
Apache Beam is an open-source, unified programming model for defining both batch and stream data processing pipelines. Beam serves as the foundation for GCP Dataflow, enabling users to write pipelines that can be executed across multiple environments (including Dataflow, Apache Flink, and Apache Spark).
Key concepts of Apache Beam used in GCP Dataflow pipelines:
PCollections: This is a distributed data set that represents the data being processed by the pipeline. PCollections can hold both bounded (batch) and unbounded (stream) data.
Transforms: Operations that modify PCollections, such as filtering or grouping elements.
Windowing: A technique for segmenting unbounded data streams into discrete chunks based on time. This is particularly useful for stream processing, as it allows for timely analysis of real-time data.
Triggers: Controls when windowed results are output based on event time or data arrival.
By leveraging Apache Beam, developers can write pipelines once and execute them in multiple environments, allowing for greater flexibility and easier integration.
4. Stream Processing with GCP Dataflow
What is Stream Processing?
Stream processing refers to the real-time analysis and processing of data as it is generated. Unlike batch processing, which processes data in chunks at scheduled intervals, stream processing analyzes data continuously as it arrives. This capability is particularly useful for applications that require immediate responses to new information, such as real-time analytics, fraud detection, or dynamic pricing models.
Stream Processing in GCP Dataflow allows users to build pipelines that handle unbounded data streams. This means that data flows into the pipeline continuously, and the processing happens in near real-time. GCP Dataflow's ability to manage low-latency processing and dynamically scale resources based on data volume makes it an ideal tool for stream processing applications.
Implementing Stream Processing on Dataflow
Stream processing in GCP Dataflow can be implemented using the Apache Beam SDK, which supports stream data sources like Cloud Pub/Sub. Here's how stream processing works in Dataflow:
Data Ingestion: Data from real-time sources such as IoT devices, social media platforms, or transaction systems is ingested through Cloud Pub/Sub. These sources continuously produce data, which needs to be processed immediately.
Windowing and Aggregation: In stream processing, it’s common to group data into windows based on time. For example, you might group all transactions within a 5-minute window for real-time sales reporting. Windowing allows Dataflow to create discrete chunks of data from an otherwise continuous stream, facilitating easier analysis and aggregation.
Transformation and Filtering: Streamed data is often noisy or contains irrelevant information. Dataflow pipelines apply transformations to clean, filter, and aggregate data in real-time. For example, you can filter out irrelevant logs from a monitoring system or aggregate clicks on a website by geographical location.
Real-Time Analytics: Processed data can be sent to real-time analytics systems like BigQuery. This enables businesses to gain immediate insights, such as detecting fraudulent transactions or generating marketing insights from user behavior on a website.
Advantages of Stream Processing in Dataflow
Real-Time Decision Making: With stream processing, businesses can react to events as they happen. This is crucial for applications like fraud detection, stock market analysis, and IoT monitoring, where quick decisions are essential.
Scalability: Dataflow automatically scales up or down based on the volume of incoming data. This ensures that your pipeline remains performant even as data volumes spike.
Unified Programming Model: Since Dataflow is built on Apache Beam, you can use the same codebase for both stream and batch processing. This simplifies development and reduces maintenance overhead.
5. Batch Processing with GCP Dataflow
What is Batch Processing?
Batch processing is the processing of a large volume of data in a scheduled, defined period. Unlike stream processing, which handles unbounded, continuous data, batch processing deals with bounded data sets that are processed in chunks. This approach is useful for tasks like ETL (Extract, Transform, Load), where data is processed periodically rather than continuously.
Batch processing pipelines in GCP Dataflow allow you to handle large-scale data transformations efficiently, whether for periodic reporting, aggregating data from multiple sources, or building machine learning models. The batch processing mode is especially suited for workloads that do not require real-time processing but need to handle vast amounts of data.
Implementing Batch Jobs on Dataflow
Batch processing in Dataflow involves reading data from sources such as Google Cloud Storage, processing it with the desired transformations, and then outputting the results to a destination like BigQuery or another storage solution. Here's a typical workflow:
Data Ingestion: For batch jobs, data is typically read from static sources such as Cloud Storage or a database. For example, you might pull in a week's worth of sales data for analysis.
Transformation: The batch data is then processed using various transformations defined in the pipeline. These might include filtering out irrelevant data, joining multiple datasets, or performing aggregations such as calculating the total sales for each region.
Batch Execution: Dataflow processes the batch job and automatically provisions the necessary resources based on the size of the dataset. Since batch jobs typically involve processing large datasets at once, Dataflow’s ability to scale workers to meet the workload demands is critical.
Output to Sink: After the data has been processed, the results are written to the designated sink, such as a BigQuery table for analysis or Cloud Storage for long-term storage.
Advantages of Batch Processing in Dataflow
Cost Efficiency: Since batch jobs are processed periodically, resources are only used when necessary, making batch processing a cost-effective solution for tasks like reporting, ETL, and data aggregation.
Scalability: Dataflow handles large-scale batch jobs efficiently by scaling resources to process large volumes of data without impacting performance.
Integration with Other GCP Services: Like stream processing, batch processing in Dataflow integrates seamlessly with BigQuery, Cloud Storage, and other GCP services, enabling you to build robust data pipelines.
6. Key Use Cases for Dataflow in GCP
GCP Dataflow is a versatile service with applications across various industries and use cases. By offering real-time stream processing and scalable batch processing, it provides critical infrastructure for modern data-driven organizations. Here are some key use cases where Dataflow in GCP excels:
Real-Time Analytics
In today's fast-paced business environment, gaining insights from data as soon as it's generated is essential. Real-time analytics enables companies to respond to events and make data-driven decisions immediately. Dataflow's stream processing capabilities make it an ideal choice for real-time analytics pipelines.
Marketing and Customer Engagement: In digital marketing, real-time analytics can be used to track user behavior and engagement in real-time. For example, e-commerce websites can use Dataflow to process clickstream data, track customer interactions, and make instant product recommendations or personalized offers based on user behavior.
Fraud Detection: Financial institutions rely heavily on real-time data processing to detect fraud. Dataflow can process financial transactions as they happen, analyze patterns for anomalies, and trigger alerts if suspicious activities are detected. The low-latency nature of Dataflow stream processing ensures that businesses can act on fraudulent activities in real-time.
IoT Analytics: The Internet of Things (IoT) generates massive amounts of data from connected devices, often requiring real-time analysis. GCP Dataflow can ingest and process this data from devices such as sensors, wearables, and industrial machines, enabling real-time monitoring, predictive maintenance, and anomaly detection.
ETL (Extract, Transform, Load) Pipelines
ETL pipelines are a fundamental part of data engineering, enabling organizations to move data from various sources, transform it into a usable format, and load it into a data warehouse or other destination. GCP Dataflow simplifies the ETL process, making it easy to build pipelines that scale with your data needs.
Data Warehousing: Dataflow can be used to extract data from different sources, transform it by cleansing and aggregating the data, and load it into BigQuery for analysis. For example, an organization might collect sales data from various regional databases and then use Dataflow to aggregate and load this data into a central data warehouse for reporting and analysis.
Data Transformation: As part of the ETL process, GCP Dataflow can perform complex data transformations, such as joining datasets, filtering out irrelevant data, or applying machine learning models to enrich the data before it is loaded into the destination system.
Data Migration: For companies moving to the cloud, GCP Dataflow can be a key tool for migrating large datasets from on-premises systems to the cloud. Whether it's migrating data from legacy databases to Google Cloud Storage or BigQuery, Dataflow ensures smooth and efficient data transfers.
Data Lakes and Warehousing
A data lake is a storage repository that holds vast amounts of raw data in its native format, while a data warehouse stores structured, processed data that can be queried for business insights. Dataflow plays a vital role in the creation and management of both data lakes and data warehouses within GCP.
Data Lakes: Dataflow can process large volumes of raw, unstructured data and store it in Cloud Storage, creating a data lake that can be used for future data exploration and analytics. This allows businesses to store data at scale without the need for immediate structure or format.
Data Warehousing: BigQuery is GCP’s fully-managed, scalable data warehouse, and GCP Dataflow can act as a powerful ETL tool to load structured and transformed data into BigQuery. For example, Dataflow might be used to preprocess transactional data before loading it into BigQuery for real-time analytics.
Machine Learning Pipelines
Machine learning models often require vast amounts of historical data for training and real-time data for continuous learning and inference. GCP Dataflow is ideal for building machine learning data pipelines, whether it’s for preprocessing data for model training or applying real-time models to incoming data.
Preprocessing Data for ML Models: Dataflow can be used to cleanse, transform, and prepare raw data for training machine learning models in AI Platform or Vertex AI. For instance, you might use Dataflow to normalize and structure data before feeding it into a model to predict customer churn.
Real-Time Predictions: Once a machine learning model is deployed, Dataflow can ingest real-time data from Cloud Pub/Sub, run predictions using the trained model, and output the results to BigQuery or another storage system. This enables businesses to make predictions based on incoming data, such as recommending products in real-time or detecting anomalies in IoT sensor data.
7. Best Practices for Using Dataflow in GCP
To get the most out of GCP Dataflow, there are several best practices to consider when building and managing your data pipelines:
Optimizing Dataflow Pipelines
Efficiency is key when designing Dataflow pipelines to minimize costs and ensure optimal performance. Here are some tips for optimizing your pipelines:
Avoid Large Batches in Stream Processing: When processing real-time data streams, it's important to avoid waiting too long before processing data (i.e., accumulating large batches). Use smaller time windows to ensure timely processing and to avoid latency issues.
Use Windowing for Stream Processing: For streaming data, windowing is an essential tool to group unbounded data into discrete chunks. Use appropriate windowing strategies (e.g., fixed windows, sliding windows, or session windows) depending on your use case. For example, session windows are great for tracking user activity on a website over a period of time.
Efficient Data Partitioning: When working with batch jobs, partition your data properly to ensure that each worker processes a reasonable chunk of data. This avoids hotspots where certain workers are overloaded while others are idle.
Security and Compliance
Data security is critical when dealing with sensitive information, and GCP Dataflow provides several features to ensure data privacy and regulatory compliance:
Encryption: All data processed by GCP Dataflow is encrypted at rest and in transit by default. For sensitive data, ensure that you configure custom encryption keys to meet your organization's security standards.
Compliance: GCP Dataflow is compliant with several regulatory standards, including GDPR, HIPAA, and SOC 2. When building data pipelines that process personal data, ensure that your pipeline adheres to these regulations and implements data masking, tokenization, or other privacy-enhancing techniques.
Scaling and Performance Tuning
GCP Dataflow automatically scales to accommodate your data processing needs, but there are a few things you can do to improve performance:
Autoscaling: By default, Dataflow uses autoscaling to adjust the number of workers based on workload. However, in cases where you have a predictable workload, you can manually adjust the number of workers to optimize performance and reduce costs.
Worker Selection: Dataflow allows you to choose different machine types for your workers, depending on your workload. If you're processing large datasets with intensive transformations, consider using higher-tier machine types to improve performance.
Fusion Optimization: Dataflow applies a technique called fusion to combine steps in a pipeline where possible, reducing the overhead of processing multiple steps separately. Make sure that your pipeline is structured in a way that allows Dataflow to apply fusion optimally.
8. Dataflow Pricing in GCP
How GCP Dataflow Pricing Works
GCP Dataflow pricing is based on the resources used by the pipeline, including the number of vCPU, memory, and storage required for the processing tasks. The cost structure involves:
Compute Time: The primary cost comes from the compute resources (i.e., vCPU and memory) used by the workers in your pipeline. You’re charged based on the amount of time your workers are active.
Data Processing Volume: If you are working with large volumes of data, the amount of data processed by the workers also influences the cost.
Autoscaling and Optimization: Since Dataflow supports autoscaling, you only pay for the resources you use, ensuring cost-efficiency for varying workloads. Optimizing pipelines and reducing unnecessary data processing steps can lead to cost savings.
Comparing Costs with Other GCP Services
Compared to other data processing services in GCP, such as Cloud Dataproc or BigQuery, Dataflow offers flexibility for stream and batch processing with real-time autoscaling and advanced data transformations. While BigQuery is more suitable for structured data warehousing tasks, Dataflow excels at building dynamic data pipelines, especially for ETL jobs and real-time streaming applications.
Cost Optimization Strategies
To reduce costs while using GCP Dataflow, consider the following strategies:
Use Preemptible Workers: For batch jobs that can tolerate interruptions, you can use preemptible VMs, which cost significantly less than standard VMs.
Optimize Pipeline Steps: Ensure that your pipeline is optimized to reduce the amount of data that needs to be processed, thereby reducing compute and storage costs.
Batch Processing for Large Jobs: If real-time processing is not required, consider using batch processing instead of streaming. Batch jobs tend to be less resource-intensive and can be scheduled during off-peak hours to further save costs.
9. Alternatives to GCP Dataflow
While GCP Dataflow is a powerful and flexible solution for real-time stream processing and batch data pipelines, other alternatives exist in the data processing landscape. Here, we explore some of the top alternatives to Dataflow, focusing on their features, pros, and cons.
1. Apache Spark on Dataproc
Apache Spark is a popular open-source distributed data processing engine known for its speed and ease of use in big data workloads. When deployed on Google Cloud Dataproc, Spark becomes a compelling alternative to Dataflow.
Key Features:
Provides in-memory data processing, making it suitable for high-performance data analytics.
Supports a wide range of data types, including structured, unstructured, and semi-structured data.
Integrates seamlessly with Hadoop, Hive, and other big data ecosystems.
Supports batch, real-time (through Spark Streaming), and machine learning workflows.
Pros:
In-memory processing offers higher speed than disk-based alternatives.
Broad community support and extensive libraries.
Flexibility to handle diverse workloads, including streaming, batch, machine learning, and SQL queries.
Cons:
Requires more hands-on management, including cluster provisioning and resource optimization.
Lacks the autoscaling capabilities of Dataflow, meaning resource allocation needs to be managed more carefully.
Stream processing in Spark Streaming is often less efficient compared to Dataflow’s native streaming capabilities.
2. Amazon Kinesis
Amazon Kinesis is a fully managed service on AWS designed for real-time data streaming. It is a strong alternative for organizations already using AWS services and looking for real-time data processing capabilities.
Key Features:
Kinesis enables real-time data ingestion from various sources, such as IoT devices, logs, and application events.
Supports integration with other AWS services like Lambda, S3, and Redshift for further data processing and analysis.
Offers Kinesis Data Analytics for real-time analytics on streaming data using SQL queries.
Pros:
Seamless integration with the AWS ecosystem.
Optimized for real-time, low-latency processing.
Managed service, removing the burden of infrastructure management.
Cons:
Less flexibility for complex transformations compared to Dataflow.
Pricing models can become costly for high-throughput data streams.
Lacks a unified framework for handling both batch and streaming pipelines like Dataflow provides with Apache Beam.
3. Azure Stream Analytics
Azure Stream Analytics is a real-time analytics service offered by Microsoft Azure. It is designed for low-latency stream processing and is often used for IoT applications, real-time analytics, and anomaly detection.
Key Features:
Integrates well with Azure IoT Hub, Event Hubs, and other Azure services for real-time data ingestion.
Offers SQL-based query language, allowing users to write real-time queries easily.
Built-in machine learning models for tasks such as predictive analytics and anomaly detection.
Pros:
Easy integration with other Azure services, making it ideal for organizations using the Azure cloud ecosystem.
Managed service with auto-scaling and fault-tolerance built-in.
Streamlined user experience with a simple SQL-like query language for real-time processing.
Cons:
Limited flexibility in terms of complex data transformations and processing compared to Dataflow and Apache Beam.
Batch processing capabilities are not as robust, making it less suitable for workloads that require both batch and stream processing.
4. Apache Flink
Apache Flink is another open-source stream processing framework with advanced features for real-time, stateful computation. Flink is known for its performance in low-latency processing and support for complex event processing (CEP).
Key Features:
Supports true low-latency, real-time stream processing.
Offers event time processing, making it ideal for use cases where the timing of events is critical (e.g., IoT and financial transactions).
Stateful processing capabilities allow for complex event pattern recognition and real-time decision making.
Pros:
Best-in-class stream processing with stateful processing and event time handling.
Flexible support for both batch and stream processing.
High fault tolerance through distributed checkpoints.
Cons:
More complex to set up and manage compared to Dataflow, requiring manual provisioning of infrastructure.
Less user-friendly for developers new to stream processing.
Smaller community compared to Apache Spark and Beam.
5. Apache NiFi
Apache NiFi is a data flow management system that provides an intuitive interface for designing data pipelines. It is especially useful for managing complex, distributed data flows, often across hybrid cloud and on-premise environments.
Key Features:
Provides a visual, drag-and-drop interface for building data pipelines.
Ideal for data ingestion from multiple sources, including IoT devices, web servers, and databases.
Supports both stream and batch processing, with real-time monitoring of data flows.
Pros:
User-friendly, making it accessible to non-developers.
Flexible, allowing for complex routing, transformation, and integration of data across multiple environments.
Well-suited for hybrid cloud and multi-cloud environments.
Cons:
While NiFi is powerful for managing data flows, it is not optimized for high-throughput data processing tasks like Dataflow or Spark.
Stream processing capabilities are limited in comparison to dedicated stream processing systems like Flink or Dataflow.
10. Conclusion
In conclusion, GCP Dataflow is a robust, flexible, and scalable tool for processing both real-time streaming and batch data. With its integration with Apache Beam, Dataflow provides a unified model that allows developers to write pipelines once and execute them across both batch and streaming environments, greatly simplifying the process of managing complex data workflows.
For real-time data processing, Dataflow's stream processing capabilities, combined with tools like Cloud Pub/Sub, offer low-latency, scalable solutions for use cases such as real-time analytics, IoT monitoring, and fraud detection. On the batch processing side, Dataflow provides an efficient way to handle large-scale ETL jobs, data aggregation, and data warehousing tasks, integrating seamlessly with services like BigQuery and Cloud Storage.
While GCP Dataflow excels in many areas, it’s important to weigh it against other tools in the market, such as Apache Spark, Amazon Kinesis, and Azure Stream Analytics. Each of these alternatives has its own strengths and weaknesses, and the choice of tool will depend on your specific use case, cloud provider, and data processing needs.
By following best practices in pipeline optimization, scaling, and security, you can maximize the value of your Dataflow pipelines while keeping costs under control. Additionally, with the built-in autoscaling and fault tolerance features of GCP Dataflow, businesses can ensure that their data pipelines remain resilient and performant even as workloads fluctuate.
In an era where data is increasingly seen as the lifeblood of modern organizations, tools like GCP Dataflow enable companies to harness the power of both real-time and historical data to drive insights, optimize operations, and deliver more value to customers. Whether you are building ETL pipelines, analyzing real-time data streams, or developing machine learning models, GCP Dataflow provides the infrastructure and flexibility needed to meet today’s data challenges. GCP Masters is the best training institute in Hyderabad.
1 note
·
View note
Text
The Sun: A Celestial Beacon in Apache Creation Myths
Image generated by the author
Imagine standing on the edge of a vast desert at dawn. The sky is painted with hues of orange and pink, and a soft breeze carries the promise of a new day. As the first rays of sunlight break over the horizon, the world awakens from its slumber—flowers bloom, animals stir, and the landscape transforms into a vibrant tapestry of life. This daily miracle is not just a spectacle of nature; for the Apache people, it embodies profound spiritual significance. In the rich tapestry of Apache creation myths, the Sun is more than a celestial body; it is the very essence of life, a guiding force that shapes their worldview and spiritual beliefs.
Spiritual Symbolism: The Heartbeat of Apache Culture
At the core of Apache culture lies a deep reverence for the Sun, which symbolizes life, guidance, and renewal. To the Apache, the Sun is akin to a parent, nurturing all that exists on Earth. The imagery of dawn breaking over the desert serves not only as a visual cue for the start of a new day but also as a metaphor for hope and renewal, central themes in Apache narratives. This connection to the Sun infuses their stories with layers of meaning, illustrating how the celestial body is woven into the very fabric of their existence.
Apache creation stories echo with the sentiment that “From the sun, all life is born.” This mantra encapsulates the Sun’s dual role as both creator and nurturer, illuminating both the physical and spiritual realms of Apache life. As the Sun rises, it awakens the earth, instilling warmth and vitality, qualities that the Apache strive to emulate in their daily lives.
Historical Context: The Sun in Apache Cosmology
To fully appreciate the significance of the Sun in Apache culture, it is essential to understand the historical context in which these beliefs developed. The Apache tribes have long inhabited the arid landscapes of the Southwestern United States, where the harsh environment has shaped their relationship with nature. The Sun’s warmth is life-sustaining in this unforgiving terrain, and its influence on the cycles of life—plant growth, animal behavior, and weather patterns—is mirrored in Apache stories.
In these narratives, the Sun is often depicted as a creator who brings order from chaos. The cycles of day and night, the changing seasons, and the rhythms of nature are all seen as manifestations of the Sun's will. Apache rituals and ceremonies frequently revolve around the Sun, underscoring its central place in their cosmology. Whether it’s a harvest ceremony or a rite of passage, the Sun's role is ever-present, guiding the Apache in their quest for balance and harmony.
Cultural Significance: Luminary of Life
When dawn breaks, it signifies more than just the start of a new day; it is a sacred moment that reinforces the Apache identity. The Sun embodies life, warmth, and guidance, acting as a constant reminder of the interconnectedness of all living things. Apache narratives often emphasize the importance of respecting nature, portraying the Sun as a luminous figure that teaches lessons about balance and resilience.
Ceremonies invoking the Sun are common, showcasing the Apache people's gratitude for its life-giving properties. Each sunrise is a call to reflect on the beauty of existence and to honor the natural world. The Sun’s predictable path across the sky serves as a metaphor for navigation, both physically and spiritually. It teaches the Apache about the importance of direction in life, encouraging them to remain grounded in their cultural practices and beliefs.
An Apache Story: The Sun as Creator
Consider a traditional Apache story that beautifully illustrates the Sun's role as a creator. In this narrative, the Sun rises each morning, casting its warm rays across the earth. With each beam of light, life awakens; flowers unfurl, animals leap into action, and the world is vibrant with possibility. The Sun is depicted as a benevolent force, a protector who watches over the earth and its inhabitants.
As the story unfolds, the Sun's descent at dusk invites a moment of reflection. The closing of the day becomes a time to honor the lessons learned and the experiences gained. It teaches resilience through the cycles of life, emphasizing that just as the Sun must set to rise again, so too must individuals endure trials to appreciate the beauty of renewal. This narrative encapsulates the Apache belief in the Sun as a source of hope and sustenance, urging them to honor the celestial body in their daily lives.
Expert Insights: The Sun in Apache Myths
Anthropologists and cultural historians have long studied the Apache relationship with the Sun, noting its personification in myths. The Sun is often depicted as a nurturing parent to the first humans, emphasizing its role in creation and sustenance. Apache stories explore the intricate relationship between the Sun and the Earth, highlighting how its warmth and light are essential for agricultural practices and the rhythms of nature.
Dr. Eliza Cortés, an anthropologist specializing in Native American cultures, notes that “the Apache view of the Sun is deeply intertwined with their understanding of life itself. It is not just a celestial object; it is a vital force that infuses every aspect of their existence.” This perspective underscores the significance of the Sun in Apache cosmology, as a symbol of life, knowledge, and the interconnectedness of all things.
Practical Applications: The Sun's Guidance
Apache narratives extend beyond spiritual symbolism; they offer practical guidance for daily living. The movement of the Sun marks the seasons, providing crucial information for agricultural planning. Apache farmers have relied on the Sun's predictable patterns to determine when to plant and harvest crops, ensuring sustenance for their communities.
Moreover, sunlight plays a significant role in traditional healing practices. The Apache believe that exposure to sunlight enhances vitality and well-being, reinforcing their connection to nature. The teachings of the Sun encourage individuals to seek wisdom from their surroundings, promoting sustainability and respect for all living things. By embracing these principles, Apache culture fosters a sense of community and interconnectedness that enriches both personal growth and collective spirit.
Modern Relevance: Lessons from Apache Wisdom
In a fast-paced world, the teachings of Apache creation myths remain relevant, offering timeless wisdom that transcends generations. The Sun teaches about cycles, balance, and the importance of community. Amid modern challenges—climate change, technological distractions, and social disconnection—Apache stories inspire resilience and remind individuals of their inherent strength and purpose.
By embracing the teachings of the Sun, individuals can cultivate gratitude and mindfulness in their daily lives. This connection fosters a deeper appreciation for the natural world and encourages thoughtful engagement with the environment. The Apache worldview serves as a gentle reminder that we are all part of a greater whole, interconnected with the earth and its cycles.
Conclusion: A Celestial Call to Action
The Sun shines brightly as a central figure in Apache creation myths, shaping cultural identity and spiritual beliefs. Apache narratives reflect a profound connection to the natural world, emphasizing the importance of harmony within nature. As the Sun rises and sets, it calls upon contemporary society to honor the environment and the lessons embedded in these ancient teachings.
As we navigate the complexities of modern life, let us look to the Sun as a guiding force, nurturing our connection to the earth and each other. By embracing the wisdom of the Apache and recognizing the significance of the Sun in our lives, we can find direction and purpose, cultivating a brighter future rooted in respect for nature and its cycles.
In the words of the Apache, may the Sun’s warmth guide us, illuminate our paths, and inspire us to live authentically and thoughtfully in this beautiful world.
AI Disclosure: AI was used for content ideation, spelling and grammar checks, and some modification of this article.
About Black Hawk Visions: We preserve and share timeless Apache wisdom through digital media. Explore nature connection, survival skills, and inner growth at Black Hawk Visions.
0 notes
Text
Yelp Overhauls Its Streaming Architecture with Apache Beam and Apache Flink
https://www.infoq.com/news/2024/04/yelp-streaming-apache-beam-flink/?utm_campaign=infoq_content&utm_source=dlvr.it&utm_medium=tumblr&utm_term=AI%2C%20ML%20%26%20Data%20Engineering-news
0 notes
Text
Eyes in the Sky: How Spy Planes are Keeping Us Safe (and Maybe a Little Too Informed)
Remember that childhood game of "I Spy"? Imagine cranking that up to 11, with high-tech gadgets strapped to flying robots soaring miles above the Earth. That's the world of Airborne Intelligence, Surveillance, and Reconnaissance, or ISR for the alphabet soup aficionados. And guess what? This multi-billion dollar industry is booming, fueled by geopolitical tensions, fancy new sensors, and even a dash of artificial intelligence.
But before you picture James Bond piloting a drone with laser beams (though, wouldn't that be a movie?), let's break it down. The ISR market is all about gathering intel from the air, using things like infrared cameras that see through darkness, radars that map the ground like a 3D printer, and even fancy algorithms that can sniff out a suspicious email from a terrorist base (okay, maybe not exactly like that, but you get the idea).
So, who's buying all these flying snoop machines? Well, Uncle Sam, for one. The US military is a big spender in the ISR game, constantly upgrading its arsenal to keep tabs on potential threats. But it's not just about bombs and bullets. Countries are using ISR tech for all sorts of things, from monitoring borders and tracking illegal fishing boats to mapping disaster zones and even keeping an eye on deforestation.
Of course, with great power comes great responsibility (as Uncle Ben from Spider-Man would say). All this intel gathering raises some eyebrows, especially when it comes to privacy concerns. Who has access to all this data? How is it used? And who's watching the watchers? These are important questions that need to be addressed as the ISR market takes off.
But hey, let's not get too dystopian. The good news is that this technology can also be a force for good. Imagine using these aerial eyes to track down poachers in Africa, deliver medical supplies to remote villages, or even predict natural disasters before they strike. That's a future I can get behind. For more information: https://www.skyquestt.com/report/airborne-isr-market
So, the next time you look up at the clouds, remember there might be more than just fluffy water vapor up there. There could be a high-tech robot eagle, silently keeping watch over the world below. And who knows, maybe it's even using AI to write a blog post about itself (meta, much?).
About Us-
SkyQuest Technology Group is a Global Market Intelligence, Innovation Management & Commercialization organization that connects innovation to new markets, networks & collaborators for achieving Sustainable Development Goals.
Contact Us-
SkyQuest Technology Consulting Pvt. Ltd.
1 Apache Way,
Westford,
Massachusetts 01886
USA (+1) 617–230–0741
Email- [email protected]
Website: https://www.skyquestt.com
0 notes
Text
Essential Components of a Data Pipeline
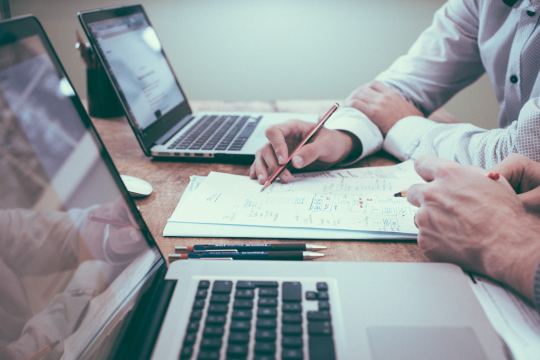
Modern businesses utilize multiple platforms to manage their routine operations. It results in the generation and collection of large volumes of data. With ever-increasing growth and the use of data-driven applications, consolidating data from multiple sources has become a complex process. It is a crucial challenge to use data to make informed decisions effectively.
Data is the foundation for analytics and operational efficiency, but processing this big data requires comprehensive data-driven strategies to enable real-time processing. The variety and velocity of this big data can be overwhelming, and a robust mechanism is needed to merge these data streams. This is where data pipelines come into the picture.
In this blog post, we will define a data pipeline and its key components.
What is a Data Pipeline?
Data can be sourced from databases, files, APIs, SQL, etc. However, this data is often unstructured and not ready for immediate use, and the responsibility of transforming the data into a structured format that can be sent to data pipelines falls on data engineers or data scientists.
A data pipeline is a technique or method of collecting raw, unstructured data from multiple sources and then transferring it to data stores or depositories such as data lakes or data warehouses. But before this data is transferred to a data depository, it usually has to undergo some form of data processing. Data pipelines consist of various interrelated steps that enable data movement from its origin to the destination for storage and analysis. An efficient data pipeline facilitates the management of volume, variety and velocity of data in these applications.
Components Of A Scalable Data Pipeline
Data Sources: Considered as the origins of data. It could be databases, web services, files, sensors, or other systems that generate or store data.
Data Ingestion: Data must be collected and ingested into the pipeline from various sources. It would involve batch processing (periodic updates) or real-time streaming (continuous data flow). The most common tools for ingestion include Apache Kafka, Apache Flume, or cloud-based services like AWS Kinesis or Azure Event Hubs.
Data Transformation: As this data moves through the pipeline, it often needs to be transformed, cleaned, and enriched. Further, it would involve data parsing, filtering, aggregating, joining, and other operations. Tools like Apache Spark and Apache Flink or stream processing frameworks like Kafka Streams or Apache Beam are used.
Data Storage: Data is typically stored in a scalable and durable storage system after transformation. Common choices include data lakes (like Amazon S3 or Hadoop HDFS), relational databases, NoSQL databases (e.g., Cassandra, MongoDB), or cloud-based storage solutions.
Data Processing: This component involves performing specific computations or analytics on the data. It can include batch processing using tools like Hadoop MapReduce or Apache Spark or real-time processing using stream processing engines like Apache Flink or Apache Kafka Streams.
Data Orchestration: Managing data flow through the pipeline often requires orchestration to ensure that various components work together harmoniously. Workflow management tools like Apache Airflow or cloud-based orchestration services like AWS Step Functions can be used.
Data Monitoring and Logging: It's essential to monitor the health and performance of your data pipeline. Logging, metrics, and monitoring solutions like ELK Stack (Elasticsearch, Logstash, Kibana), Prometheus, or cloud-based monitoring services (e.g., AWS CloudWatch) help track and troubleshoot issues.
Data Security: Ensuring data security and compliance with regulations is crucial. Encryption, access controls, and auditing mechanisms are essential to protect sensitive data.
Scalability and Load Balancing: The pipeline should be designed to handle increasing data volumes and traffic. Horizontal scaling, load balancing, and auto-scaling configurations are essential to accommodate growth.
Fault Tolerance and Reliability: Building fault-tolerant components and incorporating redundancy is critical to ensure the pipeline continues to operate in the event of failures.
Data Quality and Validation: Implement data validation checks and quality assurance measures to detect and correct errors in the data as it flows through the pipeline.
Metadata Management: Managing metadata about the data, such as data lineage, schema evolution, and versioning, is essential for data governance and maintaining data integrity.
Data Delivery: After processing, data may need to be delivered to downstream systems, data warehouses, reporting tools, or other consumers. This can involve APIs, message queues, or direct database writes.
Data Retention and Archiving: Define policies for data retention and archiving to ensure data is stored appropriately and complies with data retention requirements and regulations.
Scaling and Optimization: Continuously monitor and optimize the pipeline's performance, cost, and resource utilization as data volumes and requirements change.
Documentation and Collaboration: Maintain documentation that outlines the pipeline's architecture, components, and data flow. Collaboration tools help teams work together on pipeline development and maintenance.
Conclusion
These components of a data pipeline are essential for working with big data. Understanding these components and their role in the data pipeline makes it possible to design and build efficient, scalable, and adaptable systems to the changing needs. You can get the help of a specialist company that offers services for data engineering to help design and build systems for data collection, storage and analysis.
0 notes
Text
0 notes
Text
"Automating Data Processing for Machine Learning Models with Rust and Apache Beam"
Introduction Automating data processing for machine learning models is a crucial step in the machine learning workflow. It involves preparing, transforming, and loading data into a format that can be used by machine learning algorithms. In this tutorial, we will explore how to automate data processing for machine learning models using Rust and Apache Beam. What Readers Will Learn How to use…
0 notes
Text
5 Tips for Aspiring and Junior Data Engineers

Data engineering is a multidisciplinary field that requires a combination of technical and business skills to be successful. When starting a career in data engineering, it can be difficult to know what is necessary to be successful. Some people believe that it is important to learn specific technologies, such as Big Data, while others believe that a high level of software engineering expertise is essential. Still others believe that it is important to focus on the business side of things.
The truth is that all of these skills are important for data engineers. They need to be able to understand and implement complex technical solutions, but they also need to be able to understand the business needs of their clients and how to use data to solve those problems.
In this article, we will provide you with five essential tips to help you succeed as an aspiring or junior data engineer. Whether you’re just starting or already on this exciting career path, these tips will guide you toward excellence in data engineering.
1 Build a Strong Foundation in Data Fundamentals
One of the most critical aspects of becoming a proficient data engineer is establishing a solid foundation in data fundamentals. This includes understanding databases, data modeling, data warehousing, and data processing concepts. Many junior data engineers make the mistake of rushing into complex technologies without mastering these fundamental principles, which can lead to challenges down the road.
Start by learning about relational databases and SQL. Understand how data is structured and organized. Explore different data warehousing solutions and data storage technologies. A strong grasp of these fundamentals will serve as the bedrock of your data engineering career.
2 Master Data Integration and ETL
Efficient data integration and ETL (Extract, Transform, Load) processes are at the heart of data engineering. As a data engineer, you will often be responsible for extracting data from various sources, transforming it into a usable format, and loading it into a data warehouse or data lake. Failing to master ETL processes can lead to inefficiencies and errors in your data pipelines.
Dive into ETL tools and frameworks like Apache NiFi, Talend, or Apache Beam. Learn how to design robust data pipelines that can handle large volumes of data efficiently. Practice transforming and cleaning data to ensure its quality and reliability.
3 Learn Programming and Scripting
Programming and scripting are essential skills for data engineers. Many data engineering tasks require automation and custom code to handle complex data transformations and integration tasks. While you don’t need to be a software developer, having a strong command of programming languages like Python or Scala is highly beneficial.
Take the time to learn a programming language that aligns with your organization’s tech stack. Practice writing scripts to automate repetitive tasks, and explore libraries and frameworks that are commonly used in data engineering, such as Apache Spark for big data processing.
4 Learn Distributed Computing and Big Data Technologies
The data landscape is continually evolving, with organizations handling increasingly large and complex datasets. To stay competitive as a data engineer, you should familiarize yourself with distributed computing and big data technologies. Ignoring these advancements can limit your career growth.
Study distributed computing concepts and technologies like Hadoop and Spark. Explore cloud-based data solutions such as Amazon Web Services (AWS) and Azure, which offer scalable infrastructure for data processing. Understanding these tools will make you more versatile as a data engineer.
5 Cultivate Soft Skills and Collaboration
In addition to technical expertise, soft skills and collaboration are vital for success in data engineering. You’ll often work in multidisciplinary teams, collaborating with data scientists, analysts, and business stakeholders. Effective communication, problem-solving, and teamwork are essential for translating technical solutions into actionable insights.
Practice communication and collaboration by working on cross-functional projects. Attend team meetings, ask questions, and actively participate in discussions. Developing strong soft skills will make you a valuable asset to your organization.
Bonus Tip: Enroll in Datavalley’s Data Engineering Course
If you’re serious about pursuing a career in data engineering or want to enhance your skills as a junior data engineer, consider enrolling in Datavalley’s Data Engineering Course. This comprehensive program is designed to provide you with the knowledge and practical experience needed to excel in the field of data engineering. With experienced instructors, hands-on projects, and a supportive learning community, Datavalley’s course is an excellent way to fast-track your career in data engineering.
Course format:
Subject: Data Engineering Classes: 200 hours of live classes Lectures: 199 lectures Projects: Collaborative projects and mini projects for each module Level: All levels Scholarship: Up to 70% scholarship on all our courses Interactive activities: labs, quizzes, scenario walk-throughs Placement Assistance: Resume preparation, soft skills training, interview preparation For more details on the Big Data Engineer Masters Program visit Datavalley’s official website.
Why choose Datavalley’s Data Engineering Course?
Datavalley offers a beginner-friendly Data Engineering course with a comprehensive curriculum for all levels.
Here are some reasons to consider our course:
Comprehensive Curriculum: Our course teaches you all the essential topics and tools for data engineering. The topics include, big data foundations, Python, data processing, AWS, Snowflake advanced data engineering, data lakes, and DevOps.
Hands-on Experience:We believe in experiential learning, which means you will learn by doing. You will work on hands-on exercises and projects to apply what you have learned.
Project-Ready, Not Just Job-Ready: Upon completion of our program, you will be equipped to begin working right away and carry out projects with self-assurance.
Flexibility: Self-paced learning is a good fit for both full-time students and working professionals because it lets learners learn at their own pace and convenience.
Cutting-Edge Curriculum: Our curriculum is regularly updated to reflect the latest trends and technologies in data engineering.
Career Support: We offer career guidance and support, including job placement assistance, to help you launch your data engineering career.
On-call Project Assistance After Landing Your Dream Job: Our experts can help you with your projects for 3 months. You’ll succeed in your new role and tackle challenges with confidence.
#datavalley#dataexperts#data engineering#data analytics#dataexcellence#data science#data analytics course#data science course#business intelligence#power bi#data engineering roles#data enginner#online data engineering course#datavisualization#data visualization#dataanalytics
0 notes
Text
SQL Pipe Syntax, Now Available In BigQuery And Cloud Logging
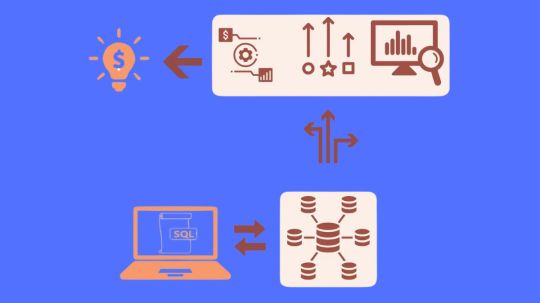
The revolutionary SQL pipe syntax is now accessible in Cloud Logging and BigQuery.
SQL has emerged as the industry standard language for database development. Its well-known syntax and established community have made data access genuinely accessible to everyone. However, SQL isn’t flawless, let’s face it. Several problems with SQL’s syntax make it more difficult to read and write:
Rigid structure: Subqueries or other intricate patterns are needed to accomplish anything else, and a query must adhere to a specific order (SELECT … FROM … WHERE … GROUP BY).
Awkward inside-out data flow: FROM clauses included in subqueries or common table expressions (CTE) are the first step in a query, after which logic is built outward.
Verbose, repetitive syntax: Are you sick of seeing the same columns in every subquery and repeatedly in SELECT, GROUP BY, and ORDER BY?
For novice users, these problems may make SQL more challenging. Reading or writing SQL requires more effort than should be required, even for experienced users. Everyone would benefit from a more practical syntax.
Numerous alternative languages and APIs have been put forth over time, some of which have shown considerable promise in specific applications. Many of these, such as Python DataFrames and Apache Beam, leverage piped data flow, which facilitates the creation of arbitrary queries. Compared to SQL, many users find this syntax to be more understandable and practical.
Presenting SQL pipe syntax
Google Cloud is to simplify and improve the usability of data analysis. It is therefore excited to provide pipe syntax, a ground-breaking invention that enhances SQL in BigQuery and Cloud Logging with the beauty of piped data flow.
Pipe syntax: what is it?
In summary, pipe syntax is an addition to normal SQL syntax that increases the flexibility, conciseness, and simplicity of SQL. Although it permits applying operators in any sequence and in any number of times, it provides the same underlying operators as normal SQL, with the same semantics and essentially the same syntax.
How it operates:
FROM can be used to begin a query.
The |> pipe sign is used to write operators in a consecutive fashion.
Every operator creates an output table after consuming its input table.
Standard SQL syntax is used by the majority of pipe operators:
LIMIT, ORDER BY, JOIN, WHERE, SELECT, and so forth.
It is possible to blend standard and pipe syntax at will, even in the same query.
Impact in the real world at HSBC
After experimenting with a preliminary version in BigQuery and seeing remarkable benefits, the multinational financial behemoth HSBC has already adopted pipe syntax. They observed notable gains in code readability and productivity, particularly when working with sizable JSON collections.
Benefits of integrating SQL pipe syntax
SQL developers benefit from the addition of pipe syntax in several ways. Here are several examples:
Simple to understand
It can be difficult to learn and accept new languages, especially in large organizations where it is preferable for everyone to utilize the same tools and languages. Pipe syntax is a new feature of the already-existing SQL language, not a new language. Because pipe syntax uses many of the same operators and largely uses the same syntax, it is relatively easy for users who are already familiar with SQL to learn.
Learning pipe syntax initially is simpler for users who are new to SQL. They can utilize those operators to express their intended queries directly, avoiding some of the complexities and workarounds needed when writing queries in normal SQL, but they still need to master the operators and some semantics (such as inner and outer joins).
Simple to gradually implement without requiring migrations
As everyone knows, switching to a new language or system may be costly, time-consuming, and prone to mistakes. You don’t need to migrate anything in order to begin using pipe syntax because it is a part of GoogleSQL. All current queries still function, and the new syntax can be used sparingly where it is useful. Existing SQL code is completely compatible with any new SQL. For instance, standard views defined in standard syntax can be called by queries using pipe syntax, and vice versa. Any current SQL does not become outdated or unusable when pipe syntax is used in new SQL code.
No impact on cost or performance
Without any additional layers (such translation proxies), which might increase latency, cost, or reliability issues and make debugging or tweaking more challenging, pipe syntax functions on well-known platforms like BigQuery.
Additionally, there is no extra charge. SQL’s declarative semantics still apply to queries utilizing pipe syntax, therefore the SQL query optimizer will still reorganize the query to run more quickly. Stated otherwise, the performance of queries written in standard or pipe syntax is usually identical.
For what purposes can pipe syntax be used?
Pipe syntax enables you to construct SQL queries that are easier to understand, more effective, and easier to maintain, whether you’re examining data, establishing data pipelines, making dashboards, or examining logs. Additionally, you may use pipe syntax anytime you create queries because it supports the majority of typical SQL operators. A few apps to get you started are as follows:
Debugging queries and ad hoc analysis
When conducting data exploration, you usually begin by examining a table’s rows (beginning with a FROM clause) to determine what is there. After that, you apply filters, aggregations, joins, ordering, and other operations. Because you can begin with a FROM clause and work your way up from there, pipe syntax makes this type of research really simple. You can view the current results at each stage, add a pipe operator, and then rerun the query to view the updated results.
Debugging queries is another benefit of using pipe syntax. It is possible to highlight a query prefix and execute it, displaying the intermediate result up to that point. This is a good feature of queries in pipe syntax: every query prefix up to a pipe symbol is also a legitimate query.
Lifecycle of data engineering
Data processing and transformation become increasingly difficult and time-consuming as data volume increases. Building, modifying, and maintaining a data pipeline typically requires a significant technical effort in contexts with a lot of data. Pipe syntax simplifies data engineering with its more user-friendly syntax and linear query structure. Bid farewell to the CTEs and highly nested queries that tend to appear whenever standard SQL is used. This latest version of GoogleSQL simplifies the process of building and managing data pipelines by reimagining how to parse, extract, and convert data.
Using plain language and LLMs with SQL
For the same reasons that SQL can be difficult for people to read and write, research indicates that it can also be difficult for large language models (LLMs) to comprehend or produce. Pipe syntax, on the other hand, divides inquiries into separate phases that closely match the intended logical data flow. A desired data flow may be expressed more easily by the LLM using pipe syntax, and the generated queries can be made more simpler and easier for humans to understand. This also makes it much easier for humans to validate the created queries.
Because it’s much simpler to comprehend what’s happening and what’s feasible, pipe syntax also enables improved code assistants and auto-completion. Additionally, it allows for suggestions for local modifications to a single pipe operator rather than global edits to an entire query. More natural language-based operators in a query and more intelligent AI-generated code suggestions are excellent ways to increase user productivity.
Discover the potential of pipe syntax right now
Because SQL is so effective, it has been the worldwide language of data for 50 years. When it comes to expressing queries as declarative combinations of relational operators, SQL excels in many things.
However, that does not preclude SQL from being improved. By resolving SQL’s primary usability issues and opening up new possibilities for interacting with and expanding SQL, pipe syntax propels SQL into the future. This has nothing to do with creating a new language or replacing SQL. Although SQL with pipe syntax is still SQL, it is a better version of the language that is more expressive, versatile, and easy to use.
Read more on Govindhtech.com
#SQL#PipeSyntax#BigQuery#SQLpipesyntax#CloudLogging#GoogleSQL#Syntax#LLM#AI#News#Technews#Technology#Technologynews#technologytrends#govindhtech
0 notes