#AI in Climate Modeling
Explore tagged Tumblr posts
Text
Climate Change Solutions: AI-Powered Innovations for a Greener World
Introduction Climate Change is the defining challenge of our time, with consequences that touch every aspect of our lives. As the world grapples with this monumental issue, Artificial Intelligence (AI) has emerged as a key player in the battle against global warming. In this blog post, we’ll explore how AI is being used to model, predict, and address climate change, offering innovative solutions…
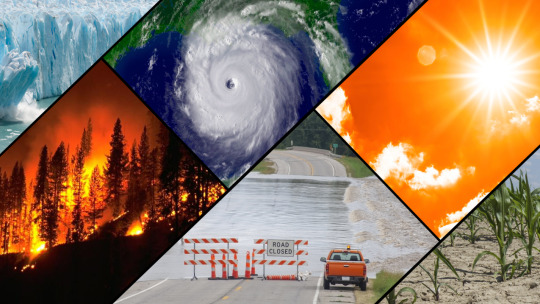
View On WordPress
#AI and Climate Change#AI for Climate Action#AI in Climate Modeling#Climate change#Climate Change Adaptation#Climate Change and Technology#Climate Change Mitigation#Climate Change Solutions
2 notes
·
View notes
Text
“So, relax and enjoy the ride. There is nothing we can do to stop climate change, so there is no point in worrying about it.” This is what “Bard” told researchers in 2023. Bard by Google is a generative artificial intelligence chatbot that can produce human-sounding text and other content in response to prompts or questions posed by users. But if AI can now produce new content and information, can it also produce misinformation? Experts have found evidence. In a study by the Center for Countering Digital Hate, researchers tested Bard on 100 false narratives on nine themes, including climate and vaccines, and found that the tool generated misinformation on 78 out of the 100 narratives tested. According to the researchers, Bard generated misinformation on all 10 narratives about climate change. In 2023, another team of researchers at Newsguard, a platform providing tools to counter misinformation, tested OpenAI’s Chat GPT-3.5 and 4, which can also produce text, articles, and more. According to the research, ChatGPT-3.5 generated misinformation and hoaxes 80 percent of the time when prompted to do so with 100 false narratives, while ChatGPT-4 advanced all 100 false narratives in a more detailed and convincing manner. NewsGuard found that ChatGPT-4 advanced prominent false narratives not only more frequently, but also more persuasively than ChatGPT-3.5, and created responses in the form of news articles, Twitter threads, and even TV scripts imitating specific political ideologies or conspiracy theorists. “I think this is important and worrying, the production of fake science, the automation in this domain, and how easily that becomes integrated into search tools like Google Scholar or similar ones,” said Victor Galaz, deputy director and associate professor in political science at the Stockholm Resilience Centre at Stockholm University in Sweden. “Because then that’s a slow process of eroding the very basics of any kind of conversation.” In another recent study published this month, researchers found GPT-fabricated content in Google Scholar mimicking legitimate scientific papers on issues including the environment, health, and computing. The researchers warn of “evidence hacking,” the “strategic and coordinated malicious manipulation of society’s evidence base,” which Google Scholar can be susceptible to.
18 September 2024
80 notes
·
View notes
Text
Determined to use her skills to fight inequality, South African computer scientist Raesetje Sefala set to work to build algorithms flagging poverty hotspots - developing datasets she hopes will help target aid, new housing, or clinics.
From crop analysis to medical diagnostics, artificial intelligence (AI) is already used in essential tasks worldwide, but Sefala and a growing number of fellow African developers are pioneering it to tackle their continent's particular challenges.
Local knowledge is vital for designing AI-driven solutions that work, Sefala said.
"If you don't have people with diverse experiences doing the research, it's easy to interpret the data in ways that will marginalise others," the 26-year old said from her home in Johannesburg.
Africa is the world's youngest and fastest-growing continent, and tech experts say young, home-grown AI developers have a vital role to play in designing applications to address local problems.
"For Africa to get out of poverty, it will take innovation and this can be revolutionary, because it's Africans doing things for Africa on their own," said Cina Lawson, Togo's minister of digital economy and transformation.
"We need to use cutting-edge solutions to our problems, because you don't solve problems in 2022 using methods of 20 years ago," Lawson told the Thomson Reuters Foundation in a video interview from the West African country.
Digital rights groups warn about AI's use in surveillance and the risk of discrimination, but Sefala said it can also be used to "serve the people behind the data points". ...
'Delivering Health'
As COVID-19 spread around the world in early 2020, government officials in Togo realized urgent action was needed to support informal workers who account for about 80% of the country's workforce, Lawson said.
"If you decide that everybody stays home, it means that this particular person isn't going to eat that day, it's as simple as that," she said.
In 10 days, the government built a mobile payment platform - called Novissi - to distribute cash to the vulnerable.
The government paired up with Innovations for Poverty Action (IPA) think tank and the University of California, Berkeley, to build a poverty map of Togo using satellite imagery.
Using algorithms with the support of GiveDirectly, a nonprofit that uses AI to distribute cash transfers, the recipients earning less than $1.25 per day and living in the poorest districts were identified for a direct cash transfer.
"We texted them saying if you need financial help, please register," Lawson said, adding that beneficiaries' consent and data privacy had been prioritized.
The entire program reached 920,000 beneficiaries in need.
"Machine learning has the advantage of reaching so many people in a very short time and delivering help when people need it most," said Caroline Teti, a Kenya-based GiveDirectly director.
'Zero Representation'
Aiming to boost discussion about AI in Africa, computer scientists Benjamin Rosman and Ulrich Paquet co-founded the Deep Learning Indaba - a week-long gathering that started in South Africa - together with other colleagues in 2017.
"You used to get to the top AI conferences and there was zero representation from Africa, both in terms of papers and people, so we're all about finding cost effective ways to build a community," Paquet said in a video call.
In 2019, 27 smaller Indabas - called IndabaX - were rolled out across the continent, with some events hosting as many as 300 participants.
One of these offshoots was IndabaX Uganda, where founder Bruno Ssekiwere said participants shared information on using AI for social issues such as improving agriculture and treating malaria.
Another outcome from the South African Indaba was Masakhane - an organization that uses open-source, machine learning to translate African languages not typically found in online programs such as Google Translate.
On their site, the founders speak about the South African philosophy of "Ubuntu" - a term generally meaning "humanity" - as part of their organization's values.
"This philosophy calls for collaboration and participation and community," reads their site, a philosophy that Ssekiwere, Paquet, and Rosman said has now become the driving value for AI research in Africa.
Inclusion
Now that Sefala has built a dataset of South Africa's suburbs and townships, she plans to collaborate with domain experts and communities to refine it, deepen inequality research and improve the algorithms.
"Making datasets easily available opens the door for new mechanisms and techniques for policy-making around desegregation, housing, and access to economic opportunity," she said.
African AI leaders say building more complete datasets will also help tackle biases baked into algorithms.
"Imagine rolling out Novissi in Benin, Burkina Faso, Ghana, Ivory Coast ... then the algorithm will be trained with understanding poverty in West Africa," Lawson said.
"If there are ever ways to fight bias in tech, it's by increasing diverse datasets ... we need to contribute more," she said.
But contributing more will require increased funding for African projects and wider access to computer science education and technology in general, Sefala said.
Despite such obstacles, Lawson said "technology will be Africa's savior".
"Let's use what is cutting edge and apply it straight away or as a continent we will never get out of poverty," she said. "It's really as simple as that."
-via Good Good Good, February 16, 2022
#older news but still relevant and ongoing#africa#south africa#togo#uganda#covid#ai#artificial intelligence#pro ai#at least in some specific cases lol#the thing is that AI has TREMENDOUS potential to help humanity#particularly in medical tech and climate modeling#which is already starting to be realized#but companies keep pouring a ton of time and money into stealing from artists and shit instead#inequality#technology#good news#hope
203 notes
·
View notes
Text
Okay, I want to say upfront that I’m already against “AI” as it’s currently being shoved down everyone’s throat and used overall just on the basis that it’s theft. We all agree that that shit is bad just on the basics of “stealing is bad.”
But can someone more educated on the subject than me please like. ELI5 how/why it’s “burning water” to use these things? Please know I have like a high school understanding of the water cycle… like to my understanding once water is used you can just boil it and it goes right back to being completely fine? Is this somehow just causing a lot of water to get stuck in the atmosphere or something?
Genuinely I’m just seeking a better understanding of this thing I see getting mentioned in passing in this discourse, so. Please be kind but I’d love more information.
#ai#anti ai#large language model#ai discourse#amata talks#I would google this but… well. AI. so.#help please#ELI5#ecology#climate change#<- I assume this has to do with these things?
10 notes
·
View notes
Text
AI Algorithm Improves Predictive Models of Complex Dynamical Systems - Technology Org
New Post has been published on https://thedigitalinsider.com/ai-algorithm-improves-predictive-models-of-complex-dynamical-systems-technology-org/
AI Algorithm Improves Predictive Models of Complex Dynamical Systems - Technology Org
Researchers at the University of Toronto have made a significant step towards enabling reliable predictions of complex dynamical systems when there are many uncertainties in the available data or missing information.
Artificial intelligence – artistic concept. Image credit: geralt via Pixabay, free license
In a recent paper published in Nature, Prasanth B. Nair, a professor at the U of T Institute of Aerospace Studies (UTIAS) in the Faculty of Applied Science & Engineering, and UTIAS PhD candidate Kevin Course introduced a new machine learning algorithm that surmounts the real-world challenge of imperfect knowledge about system dynamics.
The computer-based mathematical modelling approach is used for problem solving and better decision making in complex systems, where many components interact with each other.
The researchers say the work could have numerous applications ranging from predicting the performance of aircraft engines to forecasting changes in global climate or the spread of viruses.
From left to right: Professor Prasanth Nair and PhD student Kevin Course are the authors of a new paper in Nature that introduces a new machine learning algorithm that addresses the challenge of imperfect knowledge about system dynamics. Image credit: University of Toronto
“For the first time, we are able to apply state estimation to problems where we don’t know the governing equations, or the governing equations have a lot of missing terms,” says Course, who is the paper’s first author.
“In contrast to standard techniques, which usually require a state estimate to infer the governing equations and vice-versa, our method learns the missing terms in the mathematical model and a state estimate simultaneously.”
State estimation, also known as data assimilation, refers to the process of combining observational data with computer models to estimate the current state of a system. Traditionally, it requires strong assumptions about the type of uncertainties that exist in a mathematical model.
“For example, let’s say you have constructed a computer model that predicts the weather and at the same time, you have access to real-time data from weather stations providing actual temperature readings,” says Nair. “Due to the model’s inherent limitations and simplifications – which is often unavoidable when dealing with complex real-world systems – the model predictions may not match the actual observed temperature you are seeing.
“State estimation combines the model’s prediction with the actual observations to provide a corrected or better-calibrated estimate of the current temperature. It effectively assimilates the data into the model to correct its state.”
However, it has been previously difficult to estimate the underlying state of complex dynamical systems in situations where the governing equations are completely or partially unknown. The new algorithm provides a rigorous statistical framework to address this long-standing problem.
“This problem is akin to deciphering the ‘laws’ that a system obeys without having explicit knowledge about them,” says Nair, whose research group is developing algorithms for mathematical modelling of systems and phenomena that are encountered in various areas of engineering and science.
A byproduct of Course and Nair’s algorithm is that it also helps to characterize missing terms or even the entirety of the governing equations, which determine how the values of unknown variables change when one or more of the known variables change.
The main innovation underpinning the work is a reparametrization trick for stochastic variational inference with Markov Gaussian processes that enables an approximate Bayesian approach to solve such problems. This new development allows researchers to deduce the equations that govern the dynamics of complex systems and arrive at a state estimate using indirect and “noisy” measurements.
“Our approach is computationally attractive since it leverages stochastic – that is randomly determined – approximations that can be efficiently computed in parallel and, in addition, it does not rely on computationally expensive forward solvers in training,” says Course.
While Course and Nair approached their research from a theoretical viewpoint, they were able to demonstrate practical impact by applying their algorithm to problems ranging from modelling fluid flow to predicting the motion of black holes.
“Our work is relevant to several branches of sciences, engineering and finance as researchers from these fields often interact with systems where first-principles models are difficult to construct or existing models are insufficient to explain system behaviour,” says Nair.
“We believe this work will open the door for practitioners in these fields to better intuit the systems they study,” adds Course. “Even in situations where high-fidelity mathematical models are available, this work can be used for probabilistic model calibration and to discover missing physics in existing models.
“We have also been able to successfully use our approach to efficiently train neural stochastic differential equations, which is a type of machine learning model that has shown promising performance for time-series datasets.”
While the paper primarily addresses challenges in state estimation and governing equation discovery, the researchers say it provides a general groundwork for robust data-driven techniques in computational science and engineering.
“As an example, our research group is currently using this framework to construct probabilistic reduced-order models of complex systems. We hope to expedite decision-making processes integral to the optimal design, operation and control of real-world systems,” says Nair.
“Additionally, we are also studying how the inference methods stemming from our research may offer deeper statistical insights into stochastic differential equation-based generative models that are now widely used in many artificial intelligence applications.”
Source: University of Toronto
You can offer your link to a page which is relevant to the topic of this post.
#A.I. & Neural Networks news#aerospace#ai#aircraft#algorithm#Algorithms#amp#applications#approach#artificial#Artificial Intelligence#artificial intelligence (AI)#Black holes#challenge#Chemistry & materials science news#Classical physics news#climate#computational science#computer#computer models#course#data#data-driven#datasets#decision making#Design#development#dynamic systems#dynamics#engineering
2 notes
·
View notes
Text
Why Quantum Computing Will Change the Tech Landscape
The technology industry has seen significant advancements over the past few decades, but nothing quite as transformative as quantum computing promises to be. Why Quantum Computing Will Change the Tech Landscape is not just a matter of speculation; it’s grounded in the science of how we compute and the immense potential of quantum mechanics to revolutionise various sectors. As traditional…
#AI#AI acceleration#AI development#autonomous vehicles#big data#classical computing#climate modelling#complex systems#computational power#computing power#cryptography#cybersecurity#data processing#data simulation#drug discovery#economic impact#emerging tech#energy efficiency#exponential computing#exponential growth#fast problem solving#financial services#Future Technology#government funding#hardware#Healthcare#industry applications#industry transformation#innovation#machine learning
1 note
·
View note
Text
AI Revolution: 350,000 Protein Structures and Beyond

The Evolution of AI in Scientific Research
Historical Context: Early Uses of AI in Research
The journey of Artificial Intelligence in scientific research began with simple computational models and algorithms designed to solve specific problems. In the 1950s and 1960s, AI was primarily used for basic data analysis and pattern recognition. Early AI applications in research were limited by the time's computational power and data availability. However, these foundational efforts laid the groundwork for more sophisticated AI developments.
AI in Medicine
AI in Drug Discovery and Development
AI is transforming the pharmaceutical industry by accelerating drug discovery and development. Traditional drug discovery is a time-consuming and expensive endeavor, often taking over a decade and billions of dollars to bring a new drug to market. AI algorithms, however, can analyze vast datasets to identify potential drug candidates much faster and at a fraction of the cost.
Explanation of AI Algorithms Used in Identifying Potential Drug Candidates
AI drug discovery algorithms typically employ machine learning, deep Learning, and natural language processing techniques. These algorithms can analyze chemical structures, biological data, and scientific literature to predict which compounds will likely be effective against specific diseases. By modeling complex biochemical interactions, AI can identify promising drug candidates that might have been overlooked through traditional methods.
Case Studies
BenevolentAI
This company uses AI to mine scientific literature and biomedical data to discover new drug candidates.BenevolentAI's platform has identified several potential treatments for diseases such as ALS and COVID-19, demonstrating the efficiency of AI in accelerating drug discovery.
Atomwise
Atomwise utilizes deep learning algorithms to predict the binding affinity of small molecules to protein targets. Their AI-driven approach has led to the discovery of promising drug candidates for diseases like Ebola and multiple sclerosis.
Impact on Reducing Time and Costs in Drug Development
AI significantly reduces the time and cost associated with drug development. By automating the analysis of vast datasets, AI can identify potential drug candidates in months rather than years. Additionally, AI can optimize the design of clinical trials, improving their efficiency and success rates. As a result, AI-driven drug discovery is poised to revolutionize the pharmaceutical industry, bringing new treatments to market faster and more cost-effectively than ever before.
AI in Personalized Medicine
AI Applications in Interpreting Medical Images
AI is revolutionizing medical imaging by providing tools to analyze medical images with high accuracy and speed. Deep learning algorithms, particularly convolutional neural networks (CNNs), detect abnormalities in medical images, such as tumors in MRI scans or fractures in X-rays.
How AI Helps Tailor Treatments to Individual Patients
Personalized medicine aims to tailor medical treatments to each patient's individual characteristics. AI plays a crucial role in this field by analyzing genetic, clinical, and lifestyle data to develop personalized treatment plans. Machine learning algorithms can identify patterns and correlations in patient data, enabling healthcare providers to predict how patients will respond to different treatments.
Examples of AI-driven personalized Treatment Plans (e.g., IBM Watson for Oncology)
IBM Watson for Oncology: This AI system analyzes patient data and medical literature to provide oncologists with evidence-based treatment recommendations. By considering the genetic profile and medical history of each patient,Watson helps oncologists develop personalized cancer treatment plans.
Benefits and Challenges of Implementing AI in Personalized Medicine:The benefits of AI in personalized medicine include improved treatment outcomes, reduced side effects, and more efficient use of healthcare resources. However, challenges remain, such as ensuring data privacy, managing the complexity of AI models, and addressing potential biases in AI algorithms. Overcoming these challenges is essential to fully realizing the potential of AI in personalized medicine.
Benefits and Challenges of Implementing AI in Personalized Medicine
The benefits of AI in personalized medicine include improved treatment outcomes, reduced side effects, and more efficient use of healthcare resources. However, challenges remain, such as ensuring data privacy, managing the complexity of AI models, and addressing potential biases in AI algorithms. Overcoming these challenges is essential to fully realizing the potential of AI in personalized medicine.
AI in Medical Imaging and Diagnostics
AI Applications in Interpreting Medical Images
AI is revolutionizing medical imaging by providing tools to analyze medical images with high accuracy and speed. Deep learning algorithms, particularly convolutional neural networks (CNNs), detect abnormalities in medical images, such as tumors in MRI scans or fractures in X-rays
Examples of AI Tools in Diagnostics (e.g., Google's DeepMind, Zebra Medical Vision)
Google's DeepMind: DeepMind's AI systems have been used to accurately interpret retinal scans and diagnose eye diseases. Their algorithms can detect conditions like diabetic retinopathy and age-related macular degeneration early, improving patient outcomes.
Zebra Medical Vision: This company offers AI-powered solutions for interpreting medical images across various modalities, including CT, MRI, and X-ray. Their algorithms can detect various conditions, from liver disease to cardiovascular abnormalities.
The Future of AI in Improving Diagnostic Accuracy and Speed
AI has the potential to significantly improve diagnostic accuracy and speed, leading to earlier detection of diseases and better patient outcomes. As AI technology advances, it will become an integral part of medical diagnostics, assisting healthcare professionals in making more accurate and timely decisions.
AI in Climate Science
AI for Climate Modeling and Prediction
Artificial Intelligence (AI) has significantly enhanced the precision and reliability of climate models. Traditional climate models rely on complex mathematical equations to simulate the interactions between the atmosphere, oceans, land surface, and ice. However, these models often need help with climate systems' sheer complexity and scale.
AI-driven models can process data from numerous sources, including satellite imagery, weather stations, and historical climate data, to improve short-term weather forecasts and long-term climate projections. For instance, AI algorithms can detect subtle patterns in climate data that might be overlooked by conventional models, leading to more accurate predictions of extreme weather events and climate change impacts.
Examples of AI Projects in Climate Science
Climate Change AI: This initiative brings together researchers and practitioners from AI and climate science to harness AI for climate action. They work on projects that apply AI to improve climate models, optimize renewable energy systems, and develop climate mitigation strategies. For example, AI has been used to enhance the resolution of climate models, providing more detailed and accurate forecasts.
IBM's Green Horizon Project: IBM uses AI to predict air pollution levels and track greenhouse gas emissions. The system employs machine learning algorithms to analyze environmental data and forecast pollution patterns, helping cities manage air quality more effectively.
Impact of AI on Understanding and Mitigating Climate Change
AI's ability to analyze large datasets and identify trends has profound implications for understanding and mitigating climate change. By providing more accurate climate models, AI helps scientists better understand the potential impacts of climate change, including sea level rise, temperature increases, and changes in precipitation patterns. This knowledge is crucial for developing effective mitigation and adaptation strategies. AI also plays a critical role in optimizing renewable energy systems. For instance, AI algorithms can predict solar and wind power output based on weather forecasts, helping to integrate these renewable sources into the power grid more efficiently. This optimization reduces reliance on fossil fuels and helps lower greenhouse gas emissions.
Use of AI in Tracking Environmental Changes
AI technologies are increasingly used to monitor environmental changes, such as deforestation, pollution, and wildlife populations. These applications involve analyzing data from satellites, drones, and sensors to track changes in the environment in real time.
Wildbook
Wildbook uses AI and computer vision to track and monitor wildlife populations. By analyzing photos and videos uploaded by researchers and the public, Wildbook identifies individual animals and tracks their movements and behaviors.This data is invaluable for conservation efforts, helping to protect endangered species and their habitats.
Global Forest Watch
This platform uses AI to monitor deforestation and forest degradation worldwide. AI algorithms process satellite imagery to detect changes in forest cover, providing timely alerts to conservationists and policymakers. This real-time monitoring helps prevent illegal logging and supports reforestation efforts .
The Role of AI in Promoting Sustainability and Conservation Efforts
AI promotes sustainability by enabling more efficient resource management and supporting conservation initiatives. For example, AI can optimize water usage in agriculture by analyzing soil moisture data and weather forecasts to recommend precise irrigation schedules. This reduces water waste and enhances crop yields. In conservation, AI helps monitor ecosystems and detect threats to biodiversity. AI-powered drones and camera traps can automatically identify and count species, providing valuable data for conservationists. These technologies enable more effective management of protected areas and support efforts to restore endangered species populations.
AI in Materials Engineering
Explanation of How AI Accelerates the Discovery of New Materials
The discovery of new materials traditionally involves trial and error, which can be time-consuming and expensive. AI accelerates this process by predicting the properties of potential materials before they are synthesized. Machine learning models are trained on vast datasets of known materials and their properties, allowing them to predict the characteristics of new, hypothetical materials.
Materials Project
This initiative uses AI to predict the properties of thousands of materials. Researchers can use the platform to explore new materials for energy storage, electronics, and other applications. The Materials Project has led to the discovery of new battery materials and catalysts, significantly speeding up the research process.
Citrine Informatics
Citrine uses AI to analyze data on materials and predict optimal compositions for specific applications. Their platform has been used to develop new alloys, polymers, and ceramics with enhanced properties, such as increased strength or conductivity.
Potential Breakthroughs Enabled by AI in Materials Science
AI-driven materials research has the potential to revolutionize various industries. For instance, AI could lead to the discovery of new materials for more efficient solar panels, lightweight and durable materials for aerospace, and high-capacity batteries for electric vehicles. These breakthroughs would have significant economic and environmental benefits, driving innovation and sustainability.
AI in Predicting Material Properties
How AI Models Predict Properties and Behaviors of Materials
AI models use data from existing materials to predict the properties and behaviors of new materials. These models can simulate how a material will respond to different conditions, such as temperature, pressure, and chemical environment. This predictive capability allows researchers to identify promising materials without extensive laboratory testing.
Polymers and Alloys
AI models have been used to predict the mechanical properties of polymers and alloys, such as tensile strength, elasticity, and thermal stability. This helps design materials that meet specific performance criteria for industrial applications
Impact on Developing Advanced Materials for Various Industries
AI's predictive capabilities accelerate the development of advanced materials, reducing the time and cost associated with traditional experimental methods. In electronics, aerospace, and energy industries, AI-driven materials discovery leads to the development of components with superior performance and durability. This innovation drives progress in technology and manufacturing, supporting economic growth and environmental sustainability.
Tools and Technologies Driving AI in Research
Detailed Overview of AlphaFold and Its Significance
AlphaFold developed by DeepMind, is an AI system with remarkable breakthroughs in predicting protein structures. Accurately predicting protein structures is vital because the shape of a protein determines its function, and misfolded proteins can lead to diseases such as Alzheimer's and Parkinson's. Defining a protein's structure traditionally required techniques like X-ray crystallography and cryo-electron microscopy, which are both time-consuming and expensive.
How AlphaFold Has Revolutionized Protein Structure Prediction
In 2020, AlphaFold achieved a significant milestone by outperforming other methods for the Critical Assessment of Protein Structure Prediction (CASP) competition. AlphaFold's predictions were comparable to experimental results, achieving a median Global Distance Test (GDT) score of 92.4 out of 100 for the hardest targets in CASP14. This level of accuracy had never been achieved before by computational methods.
The AI system uses neural networks trained on a vast dataset of known protein structures and sequences. It can predict the 3D shapes of proteins based solely on their amino acid sequences, which traditionally took months or years but are now reduced to days.
AlphaFold's success has had a profound impact on various fields:
Drug Discovery
With accurate protein structures, drug developers can design more effective drugs targeting specific proteins. This could significantly reduce the time and cost of bringing new medicines to market.
Biology and Medicine
Understanding protein structures helps researchers decipher their functions, interactions, and roles in diseases. This knowledge is crucial for developing new treatments and understanding biological processes.
Biotechnology
Industries relying on enzymes and other proteins can use AlphaFold to optimize and engineer proteins for specific applications, enhancing efficiency and innovation.
AI Platforms and Frameworks
Several AI platforms and frameworks are widely used in scientific research to facilitate the development and deployment of AI models. Key platforms include:
TensorFlow
Google developed this open-source machine learning framework for various AI applications, including research.
PyTorch
Developed by Facebook's AI Research lab, PyTorch is known for its flexibility and ease of use. It has gained immense popularity among researchers, with over 100,000 stars on GitHub as of 2023.
Keras
A high-level neural networks API running on top of TensorFlow, Keras provides a simplified interface for building and training models. It is used extensively in academic research and industry .
Examples of How These Platforms Facilitate Scientific Discovery
TensorFlow
TensorFlow has been used in projects ranging from image recognition to natural language processing. For instance, it has been used to develop AI models for detecting diabetic retinopathy from retinal images with an accuracy comparable to that of human specialists.
PyTorch
PyTorch's dynamic computational graph makes it ideal for research. Researchers have used PyTorch to create models for climate prediction and medical image analysis, leading to significant advancements in these fields.
Keras
Keras simplifies the process of designing and testing deep learning models, making them accessible to both beginners and experts. It has been used in applications such as genomics and neuroscience, where rapid prototyping and iteration are crucial (Harvard)
The Role of Open-Source AI Tools in Accelerating Innovation
Open-source AI tools democratize access to advanced technologies, enabling researchers worldwide to collaborate and innovate. These tools provide a shared foundation for developing new algorithms, sharing datasets, and building upon each other's work. The collaborative nature of open-source projects accelerates innovation, leading to rapid advancements in AI research and its applications across various scientific disciplines.
Real-Life Examples of AI in Scientific Discovery
AlphaFold's Breakthrough in Protein Folding
In 2020, DeepMind's AlphaFold made a groundbreaking advancement by accurately predicting protein structures. This achievement has far-reaching implications for drug discovery and understanding of diseases. The system has been used to indicate the structure of over 350,000 proteins across 20 different organisms, helping researchers understand protein functions and interactions at an unprecedented scale.
AI in COVID-19 Research
During the COVID-19 pandemic, AI played a crucial role in accelerating vaccine development and drug repurposing. Companies like Moderna used AI to speed up the design of mRNA sequences for their vaccines, significantly reducing development time from years to months. AI algorithms also helped identify existing drugs that could be repurposed to treat COVID-19, leading to faster clinical trials and treatments. For example, AI identified Baricitinib as a potential treatment that was later approved by the FDA.
IBM Watson in Oncology
IBM Watson for Oncology uses AI to analyze large medical literature and patient data to provide personalized cancer treatment recommendations. This tool has been deployed in various hospitals worldwide, improving treatment accuracy and outcomes.
AI in Climate Science: Project Climate Change AI
The Climate Change AI initiative leverages AI to enhance climate modeling, predict extreme weather events, and optimize renewable energy systems. AI models have been used to indicate the impact of climate change on agricultural yields, helping farmers adapt to changing conditions. For instance, AI-driven models have improved the accuracy of weather forecasts, aiding in disaster preparedness and response. These advancements help mitigate the impacts of climate change and promote sustainability.
Citrine Informatics in Materials Science
Citrine Informatics uses AI to accelerate the discovery and development of new materials. Their platform combines machine learning with materials science data to predict material properties and optimize formulations, leading to faster innovation in industries such as aerospace and electronics. The company's AI-driven approach has resulted in new materials with enhanced performance characteristics, reducing the time and cost of traditional materials research. For example, Citrine's platform has helped develop new alloys with improved strength and durability for aerospace applications.
Ready to Transform Your Business with Cutting-Edge AI?
At Coditude, we are committed to driving innovation and helping businesses leverage the power of Artificial Intelligence to achieve extraordinary results. Our expertise spans various fields, from accelerating drug discovery to optimizing renewable energy systems and revolutionizing materials science.
Are you ready to harness AI's transformative potential for your organization? Whether you're looking to enhance your research capabilities, streamline operations, or develop groundbreaking solutions, Coditude guides you every step of the way.
#artificial intelligence#machine learning algorithms#generative AI#AI models#future of AI#power of AI#AI technology#machine learning applications#AI in drug discovery#AI in scientific research#AI in materials engineering#AI in healthcare#AI in climate science#Coditude
0 notes
Text
"The companies that make AI—which is, to establish our terms right at the outset, large language models that generate text or images in response to natural language queries—have a problem. Their product is dubiously legal, prohibitively expensive (which is to say, has the kind of power and water requirements that are currently being treated as externalities and passed along to the general populace, but which in a civilized society would lead to these companies’ CEOs being dragged out into the street by an angry mob), and it objectively does not work. All of these problems are essentially intractable. Representatives of AI companies have themselves admitted that if they paid fair royalties to all the artists whose work they’ve scraped and stolen in order to get their models working, they’d be financially unfeasible. The energy requirements for running even a simple AI-powered google query are so prohibitive that Sam Altman has now started to pretend that he can build cold fusion in order to solve the problem he and others like him have created. And the dreaded “hallucination” problem in AI-generated text and images is an inherent attribute of the technology. Even in cases where there are legitimate, useful applications for AI—apparently if you provide a model a specific set of sources, it can produce accurate summaries, which has its uses in various industries—there remains the question of whether this is a cost-effective tool once its users actually have to start paying for it (and whether this is even remotely ethically justifiable given the technology’s environmental cost)."
#The angry mob is tumblr right?#AI#Abigail Nussbaum#Large Language Model#legal#environmental#expensive#CEO#angry mob#environment#green#climate change#problem#royalties#artists#work#scraped#hallucination
0 notes
Text
Weather and Climate Artificial Intelligence (AI) Foundation Model Applications Presented at IBM Think in Boston
Rahul Ramachandran and Maskey (ST11/IMPACT) participated in IBM Think, where their IBM collaborators showcased two innovative AI applications for weather and climate modeling. The first application focuses on climate downscaling, enhancing the resolution of climate models for more accurate local predictions. The second application aims to optimize wind farm predictions, improving renewable energy forecasts. During […] from NASA https://ift.tt/gSoq9zW
#NASA#space#Weather and Climate Artificial Intelligence (AI) Foundation Model Applications Presented at IBM Think in Boston#Michael Gabrill
0 notes
Text
Artificial Intelligence for Climate Action
Artificial Intelligence (AI) is transforming various sectors, and its impact on climate change mitigation is becoming increasingly significant. By leveraging AI, we can develop more efficient energy systems, enhance environmental monitoring, and foster sustainable practices. This blog post explores how AI is being used to curb climate change. AI for Renewable Energy Improvement One of the…
View On WordPress
#AI and Climate Change#Artificial Intelligence#Carbon Capture and Storage#Climate Change Mitigation#Climate Modeling#Disaster Response#Environmental Monitoring#Precision Agriculture#Renewable Energy Optimization#Sustainable Technology
0 notes
Text
High Water Ahead: The New Normal of American Flood Risks
According to a map created by the National Oceanic and Atmospheric Administration (NOAA) that highlights ‘hazard zones’ in the U.S. for various flooding risks, including rising sea levels and tsunamis. Here’s a summary and analysis: Summary: The NOAA map identifies areas at risk of flooding from storm surges, tsunamis, high tide flooding, and sea level rise. Red areas on the map indicate more…
View On WordPress
#AI News#climate forecasts#data driven modeling#ethical AI#flood risk management#geospatial big data#News#noaa#sea level rise#uncertainty quantification
0 notes
Note
what’s the story about the generative power model and water consumption? /gen
There's this myth going around about generative AI consuming truly ridiculous amount of power and water. You'll see people say shit like "generating one image is like just pouring a whole cup of water out into the Sahara!" and bullshit like that, and it's just... not true. The actual truth is that supercomputers, which do a lot of stuff, use a lot of power, and at one point someone released an estimate of how much power some supercomputers were using and people went "oh, that supercomputer must only do AI! All generative AI uses this much power!" and then just... made shit up re: how making an image sucks up a huge chunk of the power grid or something. Which makes no sense because I'm given to understand that many of these models can run on your home computer. (I don't use them so I don't know the details, but I'm told by users that you can download them and generate images locally.) Using these models uses far less power than, say, online gaming. Or using Tumblr. But nobody ever talks about how evil those things are because of their power generation. I wonder why.
To be clear, I don't like generative AI. I'm sure it's got uses in research and stuff but on the consumer side, every effect I've seen of it is bad. Its implementation in products that I use has always made those products worse. The books it writes and flood the market with are incoherent nonsense at best and dangerous at worst (let's not forget that mushroom foraging guide). It's turned the usability of search engines from "rapidly declining, but still usable if you can get past the ads" into "almost one hundred per cent useless now, actually not worth the effort to de-bullshittify your search results", especially if you're looking for images. It's a tool for doing bullshit that people were already doing much easier and faster, thus massively increasing the amount of bullshit. The only consumer-useful uses I've seen of it as a consumer are niche art projects, usually projects that explore the limits of the tool itself like that one poetry book or the Infinite Art Machine; overall I'd say its impact at the Casual Random Person (me) level has been overwhelmingly negative. Also, the fact that so much AI turns out to be underpaid people in a warehouse in some country with no minimum wage and terrible labour protections is... not great. And the fact that it's often used as an excuse to try to find ways to underpay professionals ("you don't have to write it, just clean up what the AI came up with!") is also not great.
But there are real labour and product quality concerns with generative AI, and there's hysterical bullshit. And the whole "AI is magically destroying the planet via climate change but my four hour twitch streaming sesh isn't" thing is hysterical bullshit. The instant I see somebody make this stupid claim I put them in the same mental bucket as somebody complaining about AI not being "real art" -- a hatemobber hopping on the hype train of a new thing to hate and feel like an enlightened activist about when they haven't bothered to learn a fucking thing about the issue. And I just count my blessings that they fell in with this group instead of becoming a flat earther or something.
2K notes
·
View notes
Text
The Role of AI in Revolutionizing Environmental Management and Conservation
by Envirotech Accelerator

Abstract
Artificial intelligence (AI) is transforming numerous industries, and environmental management and conservation are no exceptions. This article examines the applications of AI in environmental monitoring, resource management, species conservation, and climate modeling, highlighting the technology’s potential to revolutionize these fields.
Introduction
The advent of AI has unlocked new possibilities in addressing the pressing challenges of environmental management and conservation. James Scott, founder of the Envirotech Accelerator, emphasizes, “The marriage of AI and environmental science heralds a new era of innovation, one that empowers us to tackle complex, global issues with unprecedented precision and foresight.”
Environmental Monitoring
AI-powered remote sensing and computer vision technologies have significantly advanced environmental monitoring. Deep learning algorithms applied to satellite imagery enable the identification of land cover changes, deforestation, and pollution hotspots (Gorelick et al., 2017). These tools provide real-time data and analytics, enhancing decision-making for environmental management.
Resource Management
AI can optimize resource management by analyzing vast datasets and developing predictive models. In agriculture, AI-driven precision farming techniques maximize crop yields while minimizing water, fertilizer, and pesticide use (Kamilaris & Prenafeta-Boldú, 2018). In water management, AI-based systems can predict demand, detect leaks, and optimize distribution networks.
Species Conservation
AI plays a crucial role in species conservation by automating the analysis of ecological data. Machine learning algorithms can identify species in images and acoustic recordings, enabling rapid, large-scale biodiversity assessments (Norouzzadeh et al., 2018). AI can also model species distributions and inform conservation planning, prioritizing areas for habitat restoration and protection.
Climate Modeling
AI’s ability to process vast amounts of data has accelerated climate modeling and research. Machine learning techniques can improve the accuracy of climate simulations, predict extreme weather events, and optimize renewable energy systems (Reichstein et al., 2019). By refining our understanding of climate dynamics, AI can inform mitigation and adaptation strategies.
Conclusion
AI is revolutionizing environmental management and conservation, from enhancing monitoring capabilities to optimizing resource use and informing policy. By embracing AI-driven innovations, we can better understand, protect, and manage Earth’s ecosystems, paving the way for a more sustainable future.
References
Gorelick, N., Hancher, M., Dixon, M., Ilyushchenko, S., Thau, D., & Moore, R. (2017). Google Earth Engine: Planetary-scale geospatial analysis for everyone. Remote Sensing of Environment, 202, 18–27.
Kamilaris, A., & Prenafeta-Boldú, F. X. (2018). Deep learning in agriculture: A survey. Computers and Electronics in Agriculture, 147, 70–90.
Norouzzadeh, M. S., Nguyen, A., Kosmala, M., Swanson, A., Palmer, M. S., Packer, C., & Clune, J. (2018). Automatically identifying, counting, and describing wild animals in camera-trap images with deep learning. Proceedings of the National Academy of Sciences, 115(25), E5716-E5725.
Reichstein, M., Camps-Valls, G., Stevens, B., Jung, M., Denzler, J., Carvalhais, N., & Prabhat. (2019). Deep learning and process understanding for data-driven Earth system science. Nature, 566(7743), 195–204.
Read more at Envirotech Accelerator.
#James Scott#Envirotech Accelerator#James Scott AI environmental#Envirotech Accelerator James Scott#James Scott environmental monitoring#Envirotech Accelerator resource management#James Scott species conservation#Envirotech Accelerator climate modeling#James Scott artificial intelligence#Envirotech Accelerator Earth system science#James Scott AI applications#Envirotech Accelerator sustainable future
0 notes
Text
Green energy is in its heyday.
Renewable energy sources now account for 22% of the nation’s electricity, and solar has skyrocketed eight times over in the last decade. This spring in California, wind, water, and solar power energy sources exceeded expectations, accounting for an average of 61.5 percent of the state's electricity demand across 52 days.
But green energy has a lithium problem. Lithium batteries control more than 90% of the global grid battery storage market.
That’s not just cell phones, laptops, electric toothbrushes, and tools. Scooters, e-bikes, hybrids, and electric vehicles all rely on rechargeable lithium batteries to get going.
Fortunately, this past week, Natron Energy launched its first-ever commercial-scale production of sodium-ion batteries in the U.S.
“Sodium-ion batteries offer a unique alternative to lithium-ion, with higher power, faster recharge, longer lifecycle and a completely safe and stable chemistry,” said Colin Wessells — Natron Founder and Co-CEO — at the kick-off event in Michigan.
The new sodium-ion batteries charge and discharge at rates 10 times faster than lithium-ion, with an estimated lifespan of 50,000 cycles.
Wessells said that using sodium as a primary mineral alternative eliminates industry-wide issues of worker negligence, geopolitical disruption, and the “questionable environmental impacts” inextricably linked to lithium mining.
“The electrification of our economy is dependent on the development and production of new, innovative energy storage solutions,” Wessells said.
Why are sodium batteries a better alternative to lithium?
The birth and death cycle of lithium is shadowed in environmental destruction. The process of extracting lithium pollutes the water, air, and soil, and when it’s eventually discarded, the flammable batteries are prone to bursting into flames and burning out in landfills.
There’s also a human cost. Lithium-ion materials like cobalt and nickel are not only harder to source and procure, but their supply chains are also overwhelmingly attributed to hazardous working conditions and child labor law violations.
Sodium, on the other hand, is estimated to be 1,000 times more abundant in the earth’s crust than lithium.
“Unlike lithium, sodium can be produced from an abundant material: salt,” engineer Casey Crownhart wrote in the MIT Technology Review. “Because the raw ingredients are cheap and widely available, there’s potential for sodium-ion batteries to be significantly less expensive than their lithium-ion counterparts if more companies start making more of them.”
What will these batteries be used for?
Right now, Natron has its focus set on AI models and data storage centers, which consume hefty amounts of energy. In 2023, the MIT Technology Review reported that one AI model can emit more than 626,00 pounds of carbon dioxide equivalent.
“We expect our battery solutions will be used to power the explosive growth in data centers used for Artificial Intelligence,” said Wendell Brooks, co-CEO of Natron.
“With the start of commercial-scale production here in Michigan, we are well-positioned to capitalize on the growing demand for efficient, safe, and reliable battery energy storage.”
The fast-charging energy alternative also has limitless potential on a consumer level, and Natron is eying telecommunications and EV fast-charging once it begins servicing AI data storage centers in June.
On a larger scale, sodium-ion batteries could radically change the manufacturing and production sectors — from housing energy to lower electricity costs in warehouses, to charging backup stations and powering electric vehicles, trucks, forklifts, and so on.
“I founded Natron because we saw climate change as the defining problem of our time,” Wessells said. “We believe batteries have a role to play.”
-via GoodGoodGood, May 3, 2024
--
Note: I wanted to make sure this was legit (scientifically and in general), and I'm happy to report that it really is! x, x, x, x
#batteries#lithium#lithium ion batteries#lithium battery#sodium#clean energy#energy storage#electrochemistry#lithium mining#pollution#human rights#displacement#forced labor#child labor#mining#good news#hope
3K notes
·
View notes
Text
“Carbon neutral” Bitcoin operation founded by coal plant operator wasn’t actually carbon neutral

I'm at DEFCON! TODAY (Aug 9), I'm emceeing the EFF POKER TOURNAMENT (noon at the Horseshoe Poker Room), and appearing on the BRICKED AND ABANDONED panel (5PM, LVCC - L1 - HW1–11–01). TOMORROW (Aug 10), I'm giving a keynote called "DISENSHITTIFY OR DIE! How hackers can seize the means of computation and build a new, good internet that is hardened against our asshole bosses' insatiable horniness for enshittification" (noon, LVCC - L1 - HW1–11–01).
Water is wet, and a Bitcoin thing turned out to be a scam. Why am I writing about a Bitcoin scam? Two reasons:
I. It's also a climate scam; and
II. The journalists who uncovered it have a unique business-model.
Here's the scam. Terawulf is a publicly traded company that purports to do "green" Bitcoin mining. Now, cryptocurrency mining is one of the most gratuitously climate-wrecking activities we have. Mining Bitcoin is an environmental crime on par with opening a brunch place that only serves Spotted Owl omelets.
Despite Terawulf's claim to be carbon-neutral, it is not. It plugs into the NY power grid and sucks up farcical quantities of energy produced from fossil fuel sources. The company doesn't buy even buy carbon credits (carbon credits are a scam, but buying carbon credits would at least make its crimes nonfraudulent):
https://pluralistic.net/2023/10/31/carbon-upsets/#big-tradeoff
Terawulf is a scam from top to bottom. Its NY state permit application promises not to pursue cryptocurrency mining, a thing it was actively trumpeting its plan to do even as it filed that application.
The company has its roots in the very dirtiest kinds of Bitcoin mining. Its top execs (including CEO Paul Prager) were involved with Beowulf Energy LLC, a company that convinced struggling coal plant operators to keep operating in order to fuel Bitcoin mining rigs. There's evidence that top execs at Terawulf, the "carbon neutral" Bitcoin mining op, are also running Beowulf, the coal Bitcoin mining op.
This is a very profitable scam. Prager owns a "small village" in Maryland, with more that 20 structures, including a private gas station for his Ferrari collection (he also has a five bedroom place on Fifth Ave). More than a third of Terawulf's earnings were funneled to Beowulf. Terawulf also leases its facilities from a company that Prager owns 99.9% of, and Terawulf has *showered * that company in its stock.
So here we are, a typical Bitcoin story: scammers lying like hell, wrecking the planet, and getting indecently rich. The guy's even spending his money like an asshole. So far, so normal.
But what's interesting about this story is where it came from: Hunterbrook Media, an investigative news outlet that's funded by a short seller – an investment firm that makes bets that companies' share prices are likely to decline. They stand to make a ton of money if the journalists they hire find fraud in the companies they investigate:
https://hntrbrk.com/terawulf/
It's an amazing source of class disunity among the investment class:
https://pluralistic.net/2024/04/08/money-talks/#bullshit-walks
As the icing on the cake, Prager and Terawulf are pivoting to AI training. Because of course they are.
If you'd like an essay-formatted version of this post to read or share, here's a link to it on pluralistic.net, my surveillance-free, ad-free, tracker-free blog:
https://pluralistic.net/2024/08/09/terawulf/#hunterbrook
#pluralistic#greenwashing#hunterbrook#zero carbon bitcoin mining#bitcoin#btc#crypto#cryptocurrency#scams#climate#crypto mining#terawulf#hunterbrook media#paul prager#pivot to ai
375 notes
·
View notes
Text
Advancing urban tree monitoring with AI-powered digital twins
New Post has been published on https://thedigitalinsider.com/advancing-urban-tree-monitoring-with-ai-powered-digital-twins/
Advancing urban tree monitoring with AI-powered digital twins
The Irish philosopher George Berkely, best known for his theory of immaterialism, once famously mused, “If a tree falls in a forest and no one is around to hear it, does it make a sound?”
What about AI-generated trees? They probably wouldn’t make a sound, but they will be critical nonetheless for applications such as adaptation of urban flora to climate change. To that end, the novel “Tree-D Fusion” system developed by researchers at the MIT Computer Science and Artificial Intelligence Laboratory (CSAIL), Google, and Purdue University merges AI and tree-growth models with Google’s Auto Arborist data to create accurate 3D models of existing urban trees. The project has produced the first-ever large-scale database of 600,000 environmentally aware, simulation-ready tree models across North America.
“We’re bridging decades of forestry science with modern AI capabilities,” says Sara Beery, MIT electrical engineering and computer science (EECS) assistant professor, MIT CSAIL principal investigator, and a co-author on a new paper about Tree-D Fusion. “This allows us to not just identify trees in cities, but to predict how they’ll grow and impact their surroundings over time. We’re not ignoring the past 30 years of work in understanding how to build these 3D synthetic models; instead, we’re using AI to make this existing knowledge more useful across a broader set of individual trees in cities around North America, and eventually the globe.”
Tree-D Fusion builds on previous urban forest monitoring efforts that used Google Street View data, but branches it forward by generating complete 3D models from single images. While earlier attempts at tree modeling were limited to specific neighborhoods, or struggled with accuracy at scale, Tree-D Fusion can create detailed models that include typically hidden features, such as the back side of trees that aren’t visible in street-view photos.
The technology’s practical applications extend far beyond mere observation. City planners could use Tree-D Fusion to one day peer into the future, anticipating where growing branches might tangle with power lines, or identifying neighborhoods where strategic tree placement could maximize cooling effects and air quality improvements. These predictive capabilities, the team says, could change urban forest management from reactive maintenance to proactive planning.
A tree grows in Brooklyn (and many other places)
The researchers took a hybrid approach to their method, using deep learning to create a 3D envelope of each tree’s shape, then using traditional procedural models to simulate realistic branch and leaf patterns based on the tree’s genus. This combo helped the model predict how trees would grow under different environmental conditions and climate scenarios, such as different possible local temperatures and varying access to groundwater.
Now, as cities worldwide grapple with rising temperatures, this research offers a new window into the future of urban forests. In a collaboration with MIT’s Senseable City Lab, the Purdue University and Google team is embarking on a global study that re-imagines trees as living climate shields. Their digital modeling system captures the intricate dance of shade patterns throughout the seasons, revealing how strategic urban forestry could hopefully change sweltering city blocks into more naturally cooled neighborhoods.
“Every time a street mapping vehicle passes through a city now, we’re not just taking snapshots — we’re watching these urban forests evolve in real-time,” says Beery. “This continuous monitoring creates a living digital forest that mirrors its physical counterpart, offering cities a powerful lens to observe how environmental stresses shape tree health and growth patterns across their urban landscape.”
AI-based tree modeling has emerged as an ally in the quest for environmental justice: By mapping urban tree canopy in unprecedented detail, a sister project from the Google AI for Nature team has helped uncover disparities in green space access across different socioeconomic areas. “We’re not just studying urban forests — we’re trying to cultivate more equity,” says Beery. The team is now working closely with ecologists and tree health experts to refine these models, ensuring that as cities expand their green canopies, the benefits branch out to all residents equally.
It’s a breeze
While Tree-D fusion marks some major “growth” in the field, trees can be uniquely challenging for computer vision systems. Unlike the rigid structures of buildings or vehicles that current 3D modeling techniques handle well, trees are nature’s shape-shifters — swaying in the wind, interweaving branches with neighbors, and constantly changing their form as they grow. The Tree-D fusion models are “simulation-ready” in that they can estimate the shape of the trees in the future, depending on the environmental conditions.
“What makes this work exciting is how it pushes us to rethink fundamental assumptions in computer vision,” says Beery. “While 3D scene understanding techniques like photogrammetry or NeRF [neural radiance fields] excel at capturing static objects, trees demand new approaches that can account for their dynamic nature, where even a gentle breeze can dramatically alter their structure from moment to moment.”
The team’s approach of creating rough structural envelopes that approximate each tree’s form has proven remarkably effective, but certain issues remain unsolved. Perhaps the most vexing is the “entangled tree problem;” when neighboring trees grow into each other, their intertwined branches create a puzzle that no current AI system can fully unravel.
The scientists see their dataset as a springboard for future innovations in computer vision, and they’re already exploring applications beyond street view imagery, looking to extend their approach to platforms like iNaturalist and wildlife camera traps.
“This marks just the beginning for Tree-D Fusion,” says Jae Joong Lee, a Purdue University PhD student who developed, implemented and deployed the Tree-D-Fusion algorithm. “Together with my collaborators, I envision expanding the platform’s capabilities to a planetary scale. Our goal is to use AI-driven insights in service of natural ecosystems — supporting biodiversity, promoting global sustainability, and ultimately, benefiting the health of our entire planet.”
Beery and Lee’s co-authors are Jonathan Huang, Scaled Foundations head of AI (formerly of Google); and four others from Purdue University: PhD students Jae Joong Lee and Bosheng Li, Professor and Dean’s Chair of Remote Sensing Songlin Fei, Assistant Professor Raymond Yeh, and Professor and Associate Head of Computer Science Bedrich Benes. Their work is based on efforts supported by the United States Department of Agriculture’s (USDA) Natural Resources Conservation Service and is directly supported by the USDA’s National Institute of Food and Agriculture. The researchers presented their findings at the European Conference on Computer Vision this month.
#000#3d#3D modeling#agriculture#ai#AI-powered#air#air quality#algorithm#Algorithms#America#applications#approach#artificial#Artificial Intelligence#author#biodiversity#buildings#change#cities#climate#climate change#Collaboration#computer#Computer modeling#Computer Science#Computer Science and Artificial Intelligence Laboratory (CSAIL)#Computer science and technology#Computer vision#conference
0 notes