#they will use those datasets to inform their decisions. you must do it.
Explore tagged Tumblr posts
Text
first thing: people won't know that you're upset with them and why you're upset with them if you don't tell them.
second thing (very controversial): politicians are still technically people.
third thing: in the USA you can contact politicians about stuff. it's very easy actually.
okay! now synthesize the information.
#don't forget we can actually do that here in the states of america#you can contact their offices and occasionally even the Guys directly.#write letters and emails and shit. copy one off the internet#read a statement into an answering machine. you can copy one of those off the internet as well#leave public comments on bills. you can do that#i have done all of these. fucking do it.#right now. copy an email you agree with and send it to someone.#plagiarize the whole thing who fucking cares. they won't read it but whatever you say in it will be aggregated into datasets and shit.#they will use those datasets to inform their decisions. you must do it.
3 notes
·
View notes
Text
Ethics in Data Science: Challenges and Responsibilities
In today’s data-driven world, data science plays a crucial role in shaping decisions across industries. With the exponential growth of data and the rise of artificial intelligence, ethical concerns are becoming more significant than ever. For those aspiring to enter this field, it's essential to not only gain technical knowledge but also understand the ethical responsibilities that come with it. That’s why enrolling in the best data science training in Hyderabad can equip you with both skills and awareness to navigate this complex domain.
Why Ethics Matter in Data Science
The principle of data ethics is the practice of collecting, analyzing, and using data responsibly. As data scientists develop algorithms that influence healthcare, finance, law enforcement, and more, the implications of biased or unfair systems can be profound. A data-driven system that is honest, ethical, and prevents discrimination promotes trust in the system and protects individuals' privacy.
Key Ethical Challenges in Data Science
1. Data Privacy
With access to personal and sensitive data, data scientists must ensure they do not misuse or expose this information. Ethical data handling involves secure storage, anonymization, and clear user consent.
2. Bias and Fairness
Algorithms can unintentionally carry human bias if trained on skewed or incomplete datasets. This can lead to unfair outcomes in areas like job recruitment or loan approvals. Addressing bias is a key ethical responsibility.
3. Transparency and Accountability
Many data science models, especially deep learning algorithms, are often seen as "black boxes." When decisions affect people’s lives, it's important to provide explanations and be accountable for outcomes.
4. Informed Consent
Data should only be collected with the informed consent of users. Ethical issues arise when companies collect user data without clear disclosure or for purposes not agreed upon.
5. Dual Use of Technology
Some data applications may serve beneficial or harmful purposes depending on their use. For instance, facial recognition can enhance security but also invade privacy or enable surveillance.
The Responsibilities of a Data Scientist
Ethical responsibility falls on data scientists to question the impact of their work. Before developing models, they must ask: Is this data collected ethically? Is there a risk of harm? Are the outputs fair and transparent?
Organizations and professionals must work hand-in-hand to establish ethical guidelines and best practices. Ethics should not be an afterthought but a core component of every data project.
The Role of Education in Ethical Data Science
Integrating ethical training in data science education is essential. Students and professionals must understand that their models and decisions affect real people. Along with programming and analytical skills, a strong moral compass is now a non-negotiable trait for any data science professional.
Conclusion: Building Ethical Data Scientists
As data continues to influence the way our society functions, ethical practices in data science are no longer optional—they are a responsibility. By understanding the challenges and upholding ethical standards, data scientists can ensure their work is fair, respectful, and impactful. If you’re aspiring to become a responsible and skilled data professional, consider learning from SSSIT Computer Education, a trusted name for comprehensive and ethics-focused training in the field of data science.
#best data science training in hyderabad#best data science training in kukatpally#best data science training in KPHB
0 notes
Text
Why Do Companies Outsource Text Annotation Services?
Building AI models for real-world use requires both the quality and volume of annotated data. For example, marking names, dates, or emotions in a sentence helps machines learn what those words represent and how to interpret them.
At its core, different applications of AI models require different types of annotations. For example, natural language processing (NLP) models require annotated text, whereas computer vision models need labeled images.
While some data engineers attempt to build annotation teams internally, many are now outsourcing text annotation to specialized providers. This approach speeds up the process and ensures accuracy, scalability, and access to professional text annotation services for efficient, cost-effective AI development.
In this blog, we will delve into why companies like Cogito Tech offer the best, most reliable, and compliant-ready text annotation training data for the successful deployment of your AI project. What are the industries we serve, and why is outsourcing the best option so that you can make an informed decision!
What is the Need for Text Annotation Training Datasets?
A dataset is a collection of learning information for the AI models. It can include numbers, images, sounds, videos, or words to teach machines to identify patterns and make decisions. For example, a text dataset may consist of thousands of customer reviews. An audio dataset might contain hours of speech. A video dataset could have recordings of people crossing the street.
Text annotation services are crucial for developing language-specific or NLP models, chatbots, applying sentiment analysis, and machine translation applications. These datasets label parts of text, such as named entities, sentiments, or intent, so algorithms can learn patterns and make accurate predictions. Industries such as healthcare, finance, e-commerce, and customer service rely on annotated data to build and refine AI systems.
At Cogito Tech, we understand that high-quality reference datasets are critical for model deployment. We also understand that these datasets must be large enough to cover a specific use case for which the model is being built and clean enough to avoid confusion. A poor dataset can lead to a poor AI model.
How Do Text Annotation Companies Ensure Scalability?
Data scientists, NLP engineers, and AI researchers need text annotation training datasets for teaching machine learning models to understand and interpret human language. Producing and labeling this data in-house is not easy, but it is a serious challenge. The solution to this is seeking professional help from text annotation companies.
The reason for this is that as data volumes increase, in-house annotation becomes more challenging to scale without a strong infrastructure. Data scientists focusing on labeling are not able to focus on higher-level tasks like model development. Some datasets (e.g., medical, legal, or technical data) need expert annotators with specialized knowledge, which can be hard to find and expensive to employ.
Diverting engineering and product teams to handle annotation would have slowed down core development efforts and compromised strategic focus. This is where specialized agencies like ours come into play to help data engineers support their need for training data. We also provide fine-tuning, quality checks, and compliant-labeled training data, anything and everything that your model needs.
Fundamentally, data labeling services are needed to teach computers the importance of structured data. For instance, labeling might involve tagging spam emails in a text dataset. In a video, it could mean labeling people or vehicles in each frame. For audio, it might include tagging voice commands like “play” or “pause.”
Why is Text Annotation Services in Demand?
Text is one of the most common data types used in AI model training. From chatbots to language translation, text annotation companies offer labeled text datasets to help machines understand human language.
For example, a retail company might use text annotation to determine whether customers are happy or unhappy with a product. By labeling thousands of reviews as positive, negative, or neutral, AI learns to do this autonomously.
As stated in Grand View Research, “Text annotation will dominate the global market owing to the need to fine-tune the capacity of AI so that it can help recognize patterns in the text, voices, and semantic connections of the annotated data”.
Types of Text Annotation Services for AI Models
Annotated textual data is needed to help NLP models understand and process human language. Text labeling companies utilize different types of text annotation methods, including:
Named Entity Recognition (NER) NER is used to extract key information in text. It identifies and categorizes raw data into defined entities such as person names, dates, locations, organizations, and more. NER is crucial for bringing structured information from unstructured text.
Sentiment Analysis It means identifying and tagging the emotional tone expressed in a piece of textual information, typically as positive, negative, or neutral. This is commonly used to analyze customer reviews and social media posts to review public opinion.
Part-of-Speech (POS) Tagging It refers to adding metadata like assigning grammatical categories, such as nouns, pronouns, verbs, adjectives, and adverbs, to each word in a sentence. It is needed for comprehending sentence structure so that the machines can learn to perform downstream tasks such as parsing and syntactic analysis.
Intent Classification Intent classification in text refers to identifying the purpose behind a user’s input or prompt. It is generally used in the context of conversational models so that the model can classify inputs like “book a train,” “check flight,” or “change password” into intents and enable appropriate responses for them.
Importance of Training Data for NLP and Machine Learning Models
Organizations must extract meaning from unstructured text data to automate complex language-related tasks and make data-driven decisions to gain a competitive edge.
The proliferation of unstructured data, including text, images, and videos, necessitates text annotation to make this data usable as it powers your machine learning and NLP systems.
The demand for such capabilities is rapidly expanding across multiple industries:
Healthcare: Medical professionals employed by text annotation companies perform this annotation task to automate clinical documentation, extract insights from patient records, and improve diagnostic support.
Legal: Streamlining contract analysis, legal research, and e-discovery by identifying relevant entities and summarizing case law.
E-commerce: Enhancing customer experience through personalized recommendations, automated customer service, and sentiment tracking.
Finance: In order to identify fraud detection, risk assessment, and regulatory compliance, text annotation services are needed to analyze large volumes of financial text data.
By investing in developing and training high-quality NLP models, businesses unlock operational efficiencies, improve customer engagement, gain deeper insights, and achieve long-term growth.
Now that we have covered the importance, we shall also discuss the roadblocks that may come in the way of data scientists and necessitate outsourcing text annotation services.
Challenges Faced by an In-house Text Annotation Team
Cost of hiring and training the teams: Having an in-house team can demand a large upfront investment. This refers to hiring, recruiting, and onboarding skilled annotators. Every project is different and requires a different strategy to create quality training data, and therefore, any extra expenses can undermine large-scale projects.
Time-consuming and resource-draining: Managing annotation workflows in-house often demands substantial time and operational oversight. The process can divert focus from core business operations, such as task assignments, to quality checks and revisions.
Requires domain expertise and consistent QA: Though it may look simple, in actual, text annotation requires deep domain knowledge. This is especially valid for developing task-specific healthcare, legal, or finance models. Therefore, ensuring consistency and accuracy across annotations necessitates a rigorous quality assurance process, which is quite a challenge in terms of maintaining consistent checks via experienced reviewers.
Scalability problems during high-volume annotation tasks: As annotation needs grow, scaling an internal team becomes increasingly tough. Expanding capacity to handle large influx of data volume often means getting stuck because it leads to bottlenecks, delays, and inconsistency in quality of output.
Outsource Text Annotation: Top Reasons and ROI Benefits
The deployment and success of any model depend on the quality of labeling and annotation. Poorly labeled information leads to poor results. This is why many businesses choose to partner with Cogito Tech because our experienced teams validate that the datasets are tagged with the right information in an accurate manner.
Outsourcing text annotation services has become a strategic move for organizations developing AI and NLP solutions. Rather than spending time managing expenses, businesses can benefit a lot from seeking experienced service providers. Mentioned below explains why data scientists must consider outsourcing:
Cost Efficiency: Outsourcing is an economical way that can significantly reduce labor and infrastructure expenses compared to hiring internal workforce. Saving costs every month in terms of salary and infrastructure maintenance costs makes outsourcing a financially sustainable solution, especially for startups and scaling enterprises.
Scalability: Outsourcing partners provide access to a flexible and scalable workforce capable of handling large volumes of text data. So, when the project grows, the annotation capacity can increase in line with the needs.
Speed to Market: Experienced labeling partners bring pre-trained annotators, which helps projects complete faster and means streamlined workflows. This speed helps businesses bring AI models to market more quickly and efficiently.
Quality Assurance: Annotation providers have worked on multiple projects and are thus professional and experienced. They utilize multi-tiered QA systems, benchmarking tools, and performance monitoring to ensure consistent, high-quality data output. This advantage can be hard to replicate internally.
Focus on Core Competencies: Delegating annotation to experts has one simple advantage. It implies that the in-house teams have more time refining algorithms and concentrate on other aspects of model development such as product innovation, and strategic growth, than managing manual tasks.
Compliance & Security: A professional data labeling partner does not compromise on following security protocols. They adhere to data protection standards such as GDPR and HIPAA. This means that sensitive data is handled with the highest level of compliance and confidentiality. There is a growing need for compliance so that organizations are responsible for utilizing technology for the greater good of the community and not to gain personal monetary gains.
For organizations looking to streamline AI development, the benefits of outsourcing with us are clear, i.e., improved quality, faster project completion, and cost-effectiveness, all while maintaining compliance with trusted text data labeling services.
Use Cases Where Outsourcing Makes Sense
Outsourcing to a third party rather than performing it in-house can have several benefits. The foremost advantage is that our text annotation services cater to the needs of businesses at multiple stages of AI/ML development, which include agile startups to large-scale enterprise teams. Here’s how:
Startups & AI Labs Quality and reliable text training data must comply with regulations to be usable. This is why early-stage startups and AI research labs often need compliant labeled data. When startups choose top text annotation companies, they save money on building an internal team, helping them accelerate development while staying lean and focused on innovation.
Enterprise AI Projects Big enterprises working on production-grade AI systems need scalable training datasets. However, annotating millions of text records at scale is challenging. Outsourcing allows enterprises to ramp up quickly, maintain annotation throughput, and ensure consistent quality across large datasets.
Industry-specific AI Models Sectors such as legal and healthcare need precise and compliant training data because they deal with personal data that may violate individual rights while training models. However, experienced vendors offer industry-trained professionals who understand the context and sensitivity of the data because they adhere to regulatory compliance, which benefits in the long-term and model deployment stages.
Conclusion
There is a rising demand for data-driven solutions to support this innovation, and quality-annotated data is a must for developing AI and NLP models. From startups building their prototypes to enterprises deploying AI at scale, the demand for accurate, consistent, and domain-specific training data remains.
However, managing annotation in-house has significant limitations, as discussed above. Analyzing return on investment is necessary because each project has unique requirements. We have mentioned that outsourcing is a strategic choice that allows businesses to accelerate project deadlines and save money.
Choose Cogito Tech because our expertise spans Computer Vision, Natural Language Processing, Content Moderation, Data and Document Processing, and a comprehensive spectrum of Generative AI solutions, including Supervised Fine-Tuning, RLHF, Model Safety, Evaluation, and Red Teaming.
Our workforce is experienced, certified, and platform agnostic to accomplish tasks efficiently to give optimum results, thus reducing the cost and time of segregating and categorizing textual data for businesses building AI models. Original Article : Why Do Companies Outsource Text Annotation Services?
#text annotation#text annotation service#text annotation service company#cogitotech#Ai#ai data annotation#Outsource Text Annotation Services
0 notes
Text
AI and the Future of Work - The New Era of Productivity

Generative AI has evolved rapidly from novelty to necessity. But despite its explosive adoption, most professionals are still underutilizing its real potential. Why?
Because they treat AI like a search engine, not a strategy partner.
Tools like ChatGPT, DeepSeek, Claude, and Gemini can do far more than answer questions—they can elevate thinking, accelerate decision-making, and automate complex processes. In this new era of Productivity 4.0, the key differentiator isn’t access to AI—it’s how well you use it.
The Productivity Paradox: Everyone Has AI, But Few Get Results

McKinsey’s 2023 report on the economic potential of generative AI estimated that it could add up to $4.4 trillion annually to the global economy. But here’s the paradox: while adoption is widespread, impact is uneven.
Most professionals report using AI for surface-level tasks—like writing emails or summarizing documents. These are helpful, but not transformative. The real gains come when AI is integrated into core workflows, especially in areas like:
Strategic planning
Data interpretation
Team management and training
Problem-solving and innovation cycles
The question is: How do you get there?
AI Is Only as Good as the User: Why Prompting Is the New Power Skill

We’ve entered a new skill economy where prompt engineering—the art of giving AI the right instructions—defines how useful the tool becomes. According to MIT Sloan, professionals who use iterative prompting (refining AI responses step-by-step) can generate outcomes 40% more accurate and relevant than those who rely on single queries.
Example Use Case: Strategic Brainstorming
Instead of asking:
“Give me marketing ideas for a new product.”
A skilled professional might guide AI through a layered prompt path:
“Identify customer segments based on this product feature set.”
“Based on Segment A, generate emotional messaging that resonates with early adopters.”
“What platforms are most aligned with their behavior?”
The output becomes customized strategy, not generic advice.
From Information Overload to Insight Extraction

Professionals are drowning in data. According to IDC, data creation will reach 180 zettabytes by 2025, and yet, less than 3% of it is analyzed. That’s a staggering waste of potential insight.
Generative AI can help bridge this gap—but only if guided properly. When used intentionally, AI can:
Cluster and summarize large reports
Identify trends from unstructured datasets
Extract key actions or red flags from customer feedback or performance logs
Case Study:
A Fortune 500 HR department used GPT-based tools to analyze over 10,000 open-ended exit interview comments. Through advanced prompting and sentiment analysis, they uncovered a pattern of dissatisfaction tied to a specific managerial policy—insight that had been missed for years using traditional analysis.
Result? Policy change led to a 12% reduction in employee churn within 6 months.
Training, Upskilling, and Knowledge Transfer at Scale
AI can also reshape learning and development. By turning internal documents and expert knowledge into adaptive AI tutors, companies are accelerating onboarding and reskilling.
Imagine giving new hires access to an AI agent that understands your company’s SOPs, tone of voice, and workflows—and can answer questions 24/7 with consistency. According to Deloitte, firms using AI-enhanced learning solutions are seeing 30–50% reductions in training time while improving retention.
This is no longer the future. It’s happening now.
The Real Opportunity: AI as a Co-Creator of Value

To lead in the Productivity 4.0 era, professionals must move beyond passive use. They need to actively partner with AI to:
Design better strategies
Refine customer experiences
Accelerate innovation pipelines
Improve cross-functional collaboration
This shift isn’t about replacing humans—it’s about amplifying them.
Action Framework: From Tool to Transformation
Adopt: Experiment with different tools and models.
Adapt: Integrate AI into day-to-day workflows (not just occasional tasks).
Advance: Develop internal use cases that align with team goals and KPIs.
Augment: Combine AI outputs with human judgment to make decisions faster and better.
Conclusion: The Leaders of Tomorrow Are Learning AI Today
Those who unlock the deeper use cases of AI will not only outperform—they’ll redefine what performance looks like.
Whether you're in operations, marketing, HR, or executive leadership, now is the time to explore how AI can integrate into your strategic stack, not just your task list.
The difference between professionals who keep up—and those who leap forward—comes down to this: Are you learning how to use AI effectively, or just using it occasionally?
Your Blueprint for Success: Transform Your Productivity and Master Stress-Free Leadership
The good news is that these principles don’t have to be learned through trial and error. Productivity 4.0 offers a proven blueprint to help executives master these strategies in a clear and structured way.
By applying the tools and techniques from the Productivity 4.0 Premium Course, you’ll learn how to streamline your email workflow, optimize your task management, and organize your information—all in a way that reduces stress and boosts your decision-making capacity.
Are you ready to take control of your time, reduce stress, and become a more effective executive? The tools and strategies are at your fingertips. It’s time to transform your productivity and unlock your full leadership potential.
Scheduling more—it’s in managing your energy smarter. 🚀
📺 Watch this video to learn how top performers structure their day around peak energy levels, maximize deep work, and avoid burnout. Discover science-backed strategies to work smarter, not harder! 💡
How to setup a new office for your startup with high productivity
Besides, these are useful tips if you want to cultivate your skills as a leader:
Leadership Development Guide: Watch Strategy (Yes, it's Free)
Process Improvement Toolkit: Download PDF (Yes, it's Free)
Workforce Flywheel Framework Training: Watch here (Yes, it's Free)
Tools for HR Leaders Access Here (Yes, it's Free)
References:
David Allen. Getting Things Done: The Art of Stress-Free Productivity. Penguin Books, 2001.
Tony Schwartz. The Power of Full Engagement: Managing Energy, Not Time, Is the Key to High Performance and Personal Renewal. Free Press, 2003.
Cal Newport. Deep Work: Rules for Focused Success in a Distracted World. Grand Central Publishing, 2016.
Author information: My HoaPassionate Learning & Program Officer VSHR Pro Academy
0 notes
Text
Data Analysis Tools and Techniques in DY Patil Distance MBA
Business decisions are driven by data analysis All those who are pursuing their distance MBA from top distance MBA colleges including the DY Patil Distance Learning MBA program must learn how to use some of these data analysis tools and techniques. In the blog below, we explore what Data Analysis is as a subject per se and how to practically learn about it through tools similar covered under the DY Patil Distance MBA so that students can ease their transition into corporates.
Data Analysis in Business Response
Data is the lifeblood of all business — for a long time now there have been data-driven organizations and enterprises.
Data Analysis is the science of examining raw data to draw conclusions and insights that help in decision-making. Data mining is collecting, cleaning, and analyzing data to discover patterns, trends, or correlations. It is not only about doing the math: it's about having that data be meaningful to your business. By using data analysis, companies can:
Integrity customer needs and behaviors
Renovate operations to reduce costs
Stay Ahead of the Competition by Predicting Future Trends
These days, data has become the key player in this chess game called business and there is no way we can command one anymore without knowing how to make sense of it. It is by studying the Distance MBA program from DY Patil that you will be equipped with these skills to stride into this data-driven world assertively.
DY Patil Distance MBA Tools Covered
One of the Distance MBAs by DY Patil is teaching their students with a few main data analysis tools. They have globally employed industry-standard tools used by professionals in multiple industries.
1. Excel: It seems quite normal since Excel is commonly associated with spreadsheets. Data Analysis - Powerhouse Students learn how to use it for basic data manipulation, calculations, and creating pivot tables to quickly summarize a large amount of data.
2. SQL (Sequel Query Language): SQL Rip and replace the only way to maintain your databases It is crucial for the effective extracting of data from big datasets. In the DY Patil Distance MBA course, you will learn to write SQL queries to fetch and analyze data from complexly designed databases.
3. Tableau: Produces easy data visualization in Tableau This tool supports interactive, shareable dashboards. The program focuses on how to prep and shape raw data into visualizeable, actionable insights you can make sense of using Tableau.
4. Python: This has made Python quite popular with data analysts. DY Patil Distance MBA includes Python from Data Cleaning to Advanced Statistical Analysis. It introduces Python libraries such as Pandas and NumPy, which make difficult data analysis tasks easier.
Advanced Techniques
Teaching the tools will only take you so far, this is not where top distance MBA colleges like DY Patil Distance MBA stops. Students will learn advanced data analysis techniques, to be competent and beyond.
1. Predictive Analytics: Predictive analytics employs historical data to indicate possible future outcomes. This approach is vital for being able to make informed decisions. Students in the program build predictive models to help businesses predict trends and behaviors.
2. Data Visualization: Data visualization is a top skill, even beyond Tableau. Students learn how to display their data, making it easily accessible and apparent through graphs or charts with the help of other visual aids.
3. Statistical Modeling: Data models are often used as a tool to understand the data and Relationships between variables that operate from statistical perspectives. Statistical Modeling A Part Of DY Patil Distance MBA To Help Students Learn Application Of Models In Business Contexts
4. Favor Practical Learning: While theory is very important, most learning will happen where the rubber meets the road. DY Patil further says that students would not only read about tools and techniques but also use them in their ward classes.
5. Hands-On Projects: Curriculum with real-world projects The students are provided with data sets and required to analyze them using the tools that they have learned. The idea behind these projects is to do the work of business so that students will look at a practical experience supercharging their theoretical skill sets.
Conclusion
If you are planning to take the business route, data analytics is a must-know in today's world which recognizes people from their database. The course includes the essentials of data analysis like Excel, SQL, and Tableau and also explores more advanced methods such as predictive analytics covered by Python & statistical modeling. By balancing theoretical lectures with an elaborate series of hands-on projects & real-world case study-based practical learning, the students are adequately geared up for corporate success. Be it aiming for a role in data analysis or simply wanting to have something strong under their MBA toolkit, the DY Patil Online MBA is amongst the top distance MBA colleges and provides you both with applicable relevant as well comprehensive knowledge and hands-on experience that will help fulfill these goals.
#DY Patil Distance MBA#Data Analysis Tools#Data Analysis Techniques#Distance Learning#Business Intelligence#MBA Skills
0 notes
Text
The Must-Have Tools and Techniques for Data Science training courses in Pune Success
Pune, also known as the "Oxford of the East," has emerged as a major hub for technology and innovation in India with many prestigious universities and top-notch educational training institutes, Pune attracts students from all over the country who are looking to excel in fields such as data science, big data analytics and machine learning in this blog post, we will explore some of the must-have tools and techniques that can lead you to success in these rapidly growing fields.
Firstly, let's talk about data analytics training in Pune the city offers a wide range of courses for aspiring data analysts, from basic introductory classes to advanced certification programs One of the most popular options is the Big Data Analytics course offered by Savitribai Phule Pune University This intensive program covers topics like database management, predictive modelling, and statistical analysis – equipping students with a strong foundation for pursuing a career in data analytics.
For those interested specifically in big data training in Pune, there are several specialized institutes that offer comprehensive courses to meet industry demands These courses cover key concepts like Hadoop framework implementation and programming languages such as R or Python – which are essential skills for working with large datasets Institutes like Techno geeks also provide hands-on experience through live projects where students can apply their learning directly.
When it comes to machine learning training in Pune, one cannot ignore Symbiosis International University (SIU - rated among one of India's best universities for computer science education SIU offers a Master's degree program specializing in artificial intelligence that combines theoretical knowledge with practical application through case studies and project work – making it an ideal choice for those looking to build expertise in machine learning.
Last but certainly not least is the highly sought-after field of Data Science course offered by institutions like MIT School of Distance Education (MIT-SDE Their full-time Masters' degree focuses on developing analytical thinking combined with knowledge about cutting-edge technologies used in data science This course equips candidates with the necessary skills to handle complex data sets, design predictive models and make informed decisions based on statistical analysis. Pune has emerged as a leading hub for data science and analytics training in recent years, with a growing number of professionals flocking to the city to enhance their skills and career opportunities This is not surprising, considering the abundant job prospects that come with expertise in these fields However, what sets Pune apart from other cities is its focus on real-world experience and hands-on learning in its data science training courses in Pune.
Data analytics training in Pune offers students a comprehensive curriculum that emphasizes practical implementation over theoretical knowledge The course structure ensures that students are equipped with the necessary skills to tackle real-world challenges faced by organizations today Unlike traditional classroom-based teaching methods, this approach allows students to learn by doing, gaining valuable experience through projects and case studies.
#SAP training in Pune#Data Science training in Pune#best SAP training in Pune#SAP training institute Pune#Data Science training institute in Pune#SAP training center in Pune#Best Data Science training in Pune#data science training center in Pune
0 notes
Text
5 Features To Look For In A Data Cleansing Tool
Since data has become the fuel of machine learning and artificial intelligence, most businesses have become data-intensive. While most data providers and tools can assist companies in obtaining data in large quantities, they do not assure data quality. Therefore, organizations must realize the importance of data cleansing to eradicate errors in datasets. Leveraging the expertise of data cleansing companies is the best way to remove and fix corrupt, poorly formatted, inaccurate, erroneous, duplicate, and incomplete data points within datasets.
Even the most sophisticated algorithms are beaten by high-quality data. You will get misleading results without clean data, jeopardizing your decision-making processes.
According to Gartner’s research, Measuring the Business Value of Data Quality, 40% of companies fail to meet their goals due to poor data quality.
So, it has become a necessity to have a solid data management strategy.
While deleting unnecessary data is vital, the ultimate purpose of data cleansing is to make data as accurate as possible. With this process, you can make datasets as accurate as possible. It helps correct spelling and syntax errors, identifies and deletes duplicate data points, and fills mislabeled or empty fields.
Importance Of Data Cleansing
According to a Gartner report, companies believe that poor data costs them roughly $13 million yearly. More importantly, the research company discovered that 60% of organizations do not know how much incorrect data costs them since they do not track the effect.
It is believed that when it comes to data, your insights and analyses are only as good as the data you use, which directly means junk data equals rubbish analysis. Data cleaning, also known as data cleansing and scrubbing, is critical for your business if you want to foster a culture of quality data decision-making.
The datasets are more likely to be erroneous, disorganized, and incomplete if it is not cleaned beforehand. As a result, data analysis will be more difficult, unclear, and inaccurate – so will the decision based on that data analysis. To avoid the effects of poor data on your business, cleanse datasets as soon as you collect them. Not only will this reduce mistakes, but it will also reduce your staff’s frustration, boost productivity, and improve data analysis and decision-making.
How To Cleanse Data?
Data cleansing is the process of preparing data for analysis by weeding out extraneous or erroneous information. Going through zillions of data points manually for cleansing is a time taking and error-prone process. So, data cleaning technologies are crucial in making data ready for usage.
Data cleansing tools improve the quality, applicability, and value of your data by eliminating errors, reducing inconsistencies, and removing duplicates. This allows organizations to trust their data, make sound decisions, and provide better customer experiences. Data cleaning tools, also known as data scrubbing or data cleaning tools, find and eliminate incorrect or unnecessary data points and make the database precise for analysis. Employing automation to cleanse your data means that your talented resources can focus on what they do best while the tool takes care of the rest.
Many data cleansing service providers globally offer hassle-free data cleansing services to those who don’t have the time or resources to use a tool for making datasets relevant for quick and precise analysis. Choosing a tool is always a more cost-effective and hassle-free option for data cleansing. With a data cleaning tool, things that can be easily removed from datasets to make them more relevant for analysis are –
Missing fields
Outdated information
Data entered in the wrong field
Duplicate entries
Misspellings, typing errors, spelling variations
And other flaws
What Features To Look For When Choosing The Best Data Cleansing Tool?
If you don’t trust the data used in your daily work, it’s high time you start cleaning it using a cutting-edge tool with the power of AI.
An AI-powered tool delivers a whole host of specific benefits. It provides better quality data that is accurate, valid, properly formatted, and complete in a timely manner. Even top data cleansing companies today employ data cleansers to weed out erroneous, unstructured data from the datasets.
But the question is, what features to look for when finding the right tool to get the work done? Here is the list of the top 7 features that the best data cleansing software must have.
1. Data Profiling
Data profiling is the process of evaluating, analyzing, and synthesizing data into meaningful summaries. The approach produces a high-level overview that can be used to identify data quality concerns, hazards, and general trends. It translates numbers into terms and generates key insights that ordinary people can understand and may subsequently use to their advantage. Charts. Trends. Statistics. Data profiling allows for the creation of bird’s-eye summaries of tabular files. It gives extensive information and descriptive statistics for each dataset variable. Data profiling and cleansing features, which can automate metadata identification and provide clear visibility into the source data to detect any anomalies, should be included in an end-to-end data cleansing solution.
2. Excellent Connectivity
A data cleansing tool should handle standard source data formats and destination data structures, such as XML, JSON, and EDI. Thanks to connectivity to popular destination formats, you can export clean data to various destinations, including Oracle, SQL Server, PostgreSQL, and BI applications like Tableau and PowerBI. So, choose the best data cleansing software that offers excellent connectivity. This will help your company to gain faster access to high-quality data for rapid decision-making. Being data-driven in today’s world has become necessary since it helps businesses to be profitable.
The data-driven company is not only 23 times more likely to attract consumers, but they are also six times more likely to retain customers and 19 times more likely to be profitable, states McKinsey Global Institute.
3. Data Mapping
The best data cleansing software should have a data mapping feature since it bridges the gap between two systems or data models so that when data is transported from one location to another, it is accurate and usable at the same time. Each of the best data cleansing companies uses easy data mapping tools. The usability of a data cleansing tool is improved by the data mapping feature. It’s critical to correctly map or match data from source to transformation and then to the destination to ensure that your data is cleansed accurately. Such functionality can be supported by tools with a code-free, drag-and-drop graphical user interface. Always check the data mapping features when you choose the data cleansing tool.
4. Quality Checks
47% of new data collected by companies has one or more critical mistakes.
When collected data fails to match the company’s standards for accuracy, validity, completeness, and consistency, it can seriously affect customer service, staff productivity, and critical strategy-making. Data used for business purposes should have accuracy, completeness, reasonability, timeliness, uniqueness/deduplication, validity, and accessibility. So when you choose the data cleansing tool, make sure it offers advanced profiling and cleansing capabilities along with data transformation functionality. Many data cleansing companies and data cleansing service providers use such advanced data cleaning tools to deliver accurate data for business intelligence.
5. Friendly Interface
Choose a data cleansing tool that has a highly intuitive and friendly user interface. It should be easy to use and yet powerful to handle large-scale data cleaning. An ideal data cleansing tool should be used by anyone, not just IT people. When you use a data cleansing tool with a friendly user interface, you don’t need any expertise or expert IT professionals to operate it. The data cleansing process also becomes super fast with the best data cleansing software having a simple and friendly UI.
5 Benefits Of Automating The Data Cleansing Process For Your Company
According to Kissmetrics, companies might lose up to 20% of their revenue due to poor data quality.
Cleansing data and making it usable has become a necessity today. Data cleansing is frequently a task of data scientists and business analysts, whether they are new to the field or have been doing it for years. It isn’t the most enjoyable aspect of the work, but ensuring that your data is useful and accurate in the long run is required.
If data errors and the process of their eradication creeps you out, it’s best to put data cleansing on auto-pilot mode. Automation eliminates the need to manually search through each data piece to identify problems. Automating the data cleansing process has some unexpected benefits that only data cleansing companies have considered. And it’s time for you to automate your data cleansing process and enjoy its benefits like –
1. Increased Productivity
78% of business leaders agree that automating workplace tasks boosts all stakeholders’ productivity.
Automation impacts your business operations and workflow in a positive way. Discussing data cleansing automation, it eliminates the need to manually comb through data pieces to identify errors, duplicates, and other flaws. Instead of spending hours manually altering data or doing it in Excel, use data cleansing tools. They will perform the heavy lifting for you. More and more datasets will be cleansed when you put the process on autopilot mode.
2. Saved Time
Imagine yourself cleaning datasets one by one. Isn’t it scary? If you clean every piece of data one by one from your large datasets, it is going to take an eternity.
According to MIT Sloan research, employees squander over half of their time doing mundane data quality activities.
Automating the process saves you a lot of time which you can simply use on other important tasks. The most significant benefit of automation is the ability to do repeated tasks fast and without mistakes. You’ll save not only a lot of time but also eliminate time-consuming tasks like exporting and importing tables to keep your system up to date.
3. Reduced Cost
Automating data cleansing reduces the need for a specialist data cleansing team. There is no need to spend excessive money on training staff and providing them with a well-equipped working space.
74% of surveyed marketers believe that business owners and marketers use automation to save time and money.
With a little guidance, a non-tech person can easily use a data cleansing tool. You are going to reduce the cost of data cleansing by introducing automation.
4. Improved Accuracy
Accurate data is critical to the success of any business and project. However, checking for data accuracy manually can be difficult and time-consuming. That is why automation is so beneficial. You’ll never have to worry about manually checking for mistakes or dealing with the intricacies of your database again with automated data management.
5. Improved Focus On Core Tasks
The data cleansing process can be effectively automated using a cutting-edge tool. Users get more time to focus on strategic business-related core activities, while automation software takes care of repetitive tasks.
In fact, 85% of business leaders believe that automation improves their focus on strategic goals.
Manual data cleansing is a time-consuming and tedious procedure that might take days to complete. That is why it is critical to automate it. While maintaining data quality is a problem for every new organization, you can avoid being lost at sea with the correct data cleansing methods and technologies.
If you don’t have time to clean the datasets, even using a tool, you can simply choose a data cleansing company. Many data cleansing service providers outsource data cleansing services to their customers and make their valuable datasets error-free and ready to use for instant analysis. They reduce the hassle of finding an ideal tool for data cleansing.
Choose A Team, Not Just A Tool
When you’re searching for a solution to clean up your entire data system, you’re looking for more than simply a tool. You’re looking for an expert team to help you solve your data problems. Why? Because cleaning big data systems requires more than merely comparing rows and columns to find problems. It is a business practice that necessitates a full grasp of your company’s surroundings, difficulties, and data objectives. Only an expert team capable of doing everything can help you get the most out of the tool.
One of the best data cleansing companies that you can choose for adding accuracy to your datasets is Outsource BigData. We have trained professionals to provide cutting-edge data cleansing services to customers having large-scale databases. Along with data management, collection, and cleansing services, we offer our customers round-the-clock IT support.
0 notes
Text
Cutting carbon emissions on the US power grid
New Post has been published on https://sunalei.org/news/cutting-carbon-emissions-on-the-us-power-grid/
Cutting carbon emissions on the US power grid
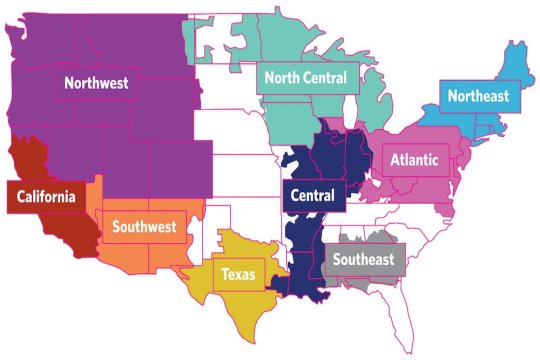
To help curb climate change, the United States is working to reduce carbon emissions from all sectors of the energy economy. Much of the current effort involves electrification — switching to electric cars for transportation, electric heat pumps for home heating, and so on. But in the United States, the electric power sector already generates about a quarter of all carbon emissions. “Unless we decarbonize our electric power grids, we’ll just be shifting carbon emissions from one source to another,” says Amanda Farnsworth, a PhD candidate in chemical engineering and research assistant at the MIT Energy Initiative (MITEI).
But decarbonizing the nation’s electric power grids will be challenging. The availability of renewable energy resources such as solar and wind varies in different regions of the country. Likewise, patterns of energy demand differ from region to region. As a result, the least-cost pathway to a decarbonized grid will differ from one region to another.
Over the past two years, Farnsworth and Emre Gençer, a principal research scientist at MITEI, developed a power system model that would allow them to investigate the importance of regional differences — and would enable experts and laypeople alike to explore their own regions and make informed decisions about the best way to decarbonize. “With this modeling capability you can really understand regional resources and patterns of demand, and use them to do a ‘bespoke’ analysis of the least-cost approach to decarbonizing the grid in your particular region,” says Gençer.
To demonstrate the model’s capabilities, Gençer and Farnsworth performed a series of case studies. Their analyses confirmed that strategies must be designed for specific regions and that all the costs and carbon emissions associated with manufacturing and installing solar and wind generators must be included for accurate accounting. But the analyses also yielded some unexpected insights, including a correlation between a region’s wind energy and the ease of decarbonizing, and the important role of nuclear power in decarbonizing the California grid.
A novel model
For many decades, researchers have been developing “capacity expansion models” to help electric utility planners tackle the problem of designing power grids that are efficient, reliable, and low-cost. More recently, many of those models also factor in the goal of reducing or eliminating carbon emissions. While those models can provide interesting insights relating to decarbonization, Gençer and Farnsworth believe they leave some gaps that need to be addressed.
For example, most focus on conditions and needs in a single U.S. region without highlighting the unique peculiarities of their chosen area of focus. Hardly any consider the carbon emitted in fabricating and installing such “zero-carbon” technologies as wind turbines and solar panels. And finally, most of the models are challenging to use. Even experts in the field must search out and assemble various complex datasets in order to perform a study of interest.
Gençer and Farnsworth’s capacity expansion model — called Ideal Grid, or IG — addresses those and other shortcomings. IG is built within the framework of MITEI’s Sustainable Energy System Analysis Modeling Environment (SESAME), an energy system modeling platform that Gençer and his colleagues at MITEI have been developing since 2017. SESAME models the levels of greenhouse gas emissions from multiple, interacting energy sectors in future scenarios.
Importantly, SESAME includes both techno-economic analyses and life-cycle assessments of various electricity generation and storage technologies. It thus considers costs and emissions incurred at each stage of the life cycle (manufacture, installation, operation, and retirement) for all generators. Most capacity expansion models only account for emissions from operation of fossil fuel-powered generators. As Farnsworth notes, “While this is a good approximation for our current grid, emissions from the full life cycle of all generating technologies become non-negligible as we transition to a highly renewable grid.”
Through its connection with SESAME, the IG model has access to data on costs and emissions associated with many technologies critical to power grid operation. To explore regional differences in the cost-optimized decarbonization strategies, the IG model also includes conditions within each region, notably details on demand profiles and resource availability.
In one recent study, Gençer and Farnsworth selected nine of the standard North American Electric Reliability Corporation (NERC) regions. For each region, they incorporated hourly electricity demand into the IG model. Farnsworth also gathered meteorological data for the nine U.S. regions for seven years — 2007 to 2013 — and calculated hourly power output profiles for the renewable energy sources, including solar and wind, taking into account the geography-limited maximum capacity of each technology.
The availability of wind and solar resources differs widely from region to region. To permit a quick comparison, the researchers use a measure called “annual capacity factor,” which is the ratio between the electricity produced by a generating unit in a year and the electricity that could have been produced if that unit operated continuously at full power for that year. Values for the capacity factors in the nine U.S. regions vary between 20 percent and 30 percent for solar power and for between 25 percent and 45 percent for wind.
Calculating optimized grids for different regions
For their first case study, Gençer and Farnsworth used the IG model to calculate cost-optimized regional grids to meet defined caps on carbon dioxide (CO2) emissions. The analyses were based on cost and emissions data for 10 technologies: nuclear, wind, solar, three types of natural gas, three types of coal, and energy storage using lithium-ion batteries. Hydroelectric was not considered in this study because there was no comprehensive study outlining potential expansion sites with their respective costs and expected power output levels.
To make region-to-region comparisons easy, the researchers used several simplifying assumptions. Their focus was on electricity generation, so the model calculations assume the same transmission and distribution costs and efficiencies for all regions. Also, the calculations did not consider the generator fleet currently in place. The goal was to investigate what happens if each region were to start from scratch and generate an “ideal” grid.
To begin, Gençer and Farnsworth calculated the most economic combination of technologies for each region if it limits its total carbon emissions to 100, 50, and 25 grams of CO2 per kilowatt-hour (kWh) generated. For context, the current U.S. average emissions intensity is 386 grams of CO2 emissions per kWh.
Given the wide variation in regional demand, the researchers needed to use a new metric to normalize their results and permit a one-to-one comparison between regions. Accordingly, the model calculates the required generating capacity divided by the average demand for each region. The required capacity accounts for both the variation in demand and the inability of generating systems — particularly solar and wind — to operate at full capacity all of the time.
The analysis was based on regional demand data for 2021 — the most recent data available. And for each region, the model calculated the cost-optimized power grid seven times, using weather data from seven years. This discussion focuses on mean values for cost and total capacity installed and also total values for coal and for natural gas, although the analysis considered three separate technologies for each fuel.
The results of the analyses confirm that there’s a wide variation in the cost-optimized system from one region to another. Most notable is that some regions require a lot of energy storage while others don’t require any at all. The availability of wind resources turns out to play an important role, while the use of nuclear is limited: the carbon intensity of nuclear (including uranium mining and transportation) is lower than that of either solar or wind, but nuclear is the most expensive technology option, so it’s added only when necessary. Finally, the change in the CO2 emissions cap brings some interesting responses.
Under the most lenient limit on emissions — 100 grams of CO2 per kWh — there’s no coal in the mix anywhere. It’s the first to go, in general being replaced by the lower-carbon-emitting natural gas. Texas, Central, and North Central — the regions with the most wind — don’t need energy storage, while the other six regions do. The regions with the least wind — California and the Southwest — have the highest energy storage requirements. Unlike the other regions modeled, California begins installing nuclear, even at the most lenient limit.
As the model plays out, under the moderate cap — 50 grams of CO2 per kWh — most regions bring in nuclear power. California and the Southeast — regions with low wind capacity factors — rely on nuclear the most. In contrast, wind-rich Texas, Central, and North Central don’t incorporate nuclear yet but instead add energy storage — a less-expensive option — to their mix. There’s still a bit of natural gas everywhere, in spite of its CO2 emissions.
Under the most restrictive cap — 25 grams of CO2 per kWh — nuclear is in the mix everywhere. The highest use of nuclear is again correlated with low wind capacity factor. Central and North Central depend on nuclear the least. All regions continue to rely on a little natural gas to keep prices from skyrocketing due to the necessary but costly nuclear component. With nuclear in the mix, the need for storage declines in most regions.
Results of the cost analysis are also interesting. Texas, Central, and North Central all have abundant wind resources, and they can delay incorporating the costly nuclear option, so the cost of their optimized system tends to be lower than costs for the other regions. In addition, their total capacity deployment — including all sources — tends to be lower than for the other regions. California and the Southwest both rely heavily on solar, and in both regions, costs and total deployment are relatively high.
Lessons learned
One unexpected result is the benefit of combining solar and wind resources. The problem with relying on solar alone is obvious: “Solar energy is available only five or six hours a day, so you need to build a lot of other generating sources and abundant storage capacity,” says Gençer. But an analysis of unit-by-unit operations at an hourly resolution yielded a less-intuitive trend: While solar installations only produce power in the midday hours, wind turbines generate the most power in the nighttime hours. As a result, solar and wind power are complementary. Having both resources available is far more valuable than having either one or the other. And having both impacts the need for storage, says Gençer: “Storage really plays a role either when you’re targeting a very low carbon intensity or where your resources are mostly solar and they’re not complemented by wind.”
Gençer notes that the target for the U.S. electricity grid is to reach net zero by 2035. But the analysis showed that reaching just 100 grams of CO2 per kWh would require at least 50 percent of system capacity to be wind and solar. “And we’re nowhere near that yet,” he says.
Indeed, Gençer and Farnsworth’s analysis doesn’t even include a zero emissions case. Why not? As Gençer says, “We cannot reach zero.” Wind and solar are usually considered to be net zero, but that’s not true. Wind, solar, and even storage have embedded carbon emissions due to materials, manufacturing, and so on. “To go to true net zero, you’d need negative emission technologies,” explains Gençer, referring to techniques that remove carbon from the air or ocean. That observation confirms the importance of performing life-cycle assessments.
Farnsworth voices another concern: Coal quickly disappears in all regions because natural gas is an easy substitute for coal and has lower carbon emissions. “People say they’ve decreased their carbon emissions by a lot, but most have done it by transitioning from coal to natural gas power plants,” says Farnsworth. “But with that pathway for decarbonization, you hit a wall. Once you’ve transitioned from coal to natural gas, you’ve got to do something else. You need a new strategy — a new trajectory to actually reach your decarbonization target, which most likely will involve replacing the newly installed natural gas plants.”
Gençer makes one final point: The availability of cheap nuclear — whether fission or fusion — would completely change the picture. When the tighter caps require the use of nuclear, the cost of electricity goes up. “The impact is quite significant,” says Gençer. “When we go from 100 grams down to 25 grams of CO2 per kWh, we see a 20 percent to 30 percent increase in the cost of electricity.” If it were available, a less-expensive nuclear option would likely be included in the technology mix under more lenient caps, significantly reducing the cost of decarbonizing power grids in all regions.
The special case of California
In another analysis, Gençer and Farnsworth took a closer look at California. In California, about 10 percent of total demand is now met with nuclear power. Yet current power plants are scheduled for retirement very soon, and a 1976 law forbids the construction of new nuclear plants. (The state recently extended the lifetime of one nuclear plant to prevent the grid from becoming unstable.) “California is very motivated to decarbonize their grid,” says Farnsworth. “So how difficult will that be without nuclear power?”
To find out, the researchers performed a series of analyses to investigate the challenge of decarbonizing in California with nuclear power versus without it. At 200 grams of CO2 per kWh — about a 50 percent reduction — the optimized mix and cost look the same with and without nuclear. Nuclear doesn’t appear due to its high cost. At 100 grams of CO2 per kWh — about a 75 percent reduction — nuclear does appear in the cost-optimized system, reducing the total system capacity while having little impact on the cost.
But at 50 grams of CO2 per kWh, the ban on nuclear makes a significant difference. “Without nuclear, there’s about a 45 percent increase in total system size, which is really quite substantial,” says Farnsworth. “It’s a vastly different system, and it’s more expensive.” Indeed, the cost of electricity would increase by 7 percent.
Going one step further, the researchers performed an analysis to determine the most decarbonized system possible in California. Without nuclear, the state could reach 40 grams of CO2 per kWh. “But when you allow for nuclear, you can get all the way down to 16 grams of CO2 per kWh,” says Farnsworth. “We found that California needs nuclear more than any other region due to its poor wind resources.”
Impacts of a carbon tax
One more case study examined a policy approach to incentivizing decarbonization. Instead of imposing a ceiling on carbon emissions, this strategy would tax every ton of carbon that’s emitted. Proposed taxes range from zero to $100 per ton.
To investigate the effectiveness of different levels of carbon tax, Farnsworth and Gençer used the IG model to calculate the minimum-cost system for each region, assuming a certain cost for emitting each ton of carbon. The analyses show that a low carbon tax — just $10 per ton — significantly reduces emissions in all regions by phasing out all coal generation. In the Northwest region, for example, a carbon tax of $10 per ton decreases system emissions by 65 percent while increasing system cost by just 2.8 percent (relative to an untaxed system).
After coal has been phased out of all regions, every increase in the carbon tax brings a slow but steady linear decrease in emissions and a linear increase in cost. But the rates of those changes vary from region to region. For example, the rate of decrease in emissions for each added tax dollar is far lower in the Central region than in the Northwest, largely due to the Central region’s already low emissions intensity without a carbon tax. Indeed, the Central region without a carbon tax has a lower emissions intensity than the Northwest region with a tax of $100 per ton.
As Farnsworth summarizes, “A low carbon tax — just $10 per ton — is very effective in quickly incentivizing the replacement of coal with natural gas. After that, it really just incentivizes the replacement of natural gas technologies with more renewables and more energy storage.” She concludes, “If you’re looking to get rid of coal, I would recommend a carbon tax.”
Future extensions of IG
The researchers have already added hydroelectric to the generating options in the IG model, and they are now planning further extensions. For example, they will include additional regions for analysis, add other long-term energy storage options, and make changes that allow analyses to take into account the generating infrastructure that already exists. Also, they will use the model to examine the cost and value of interregional transmission to take advantage of the diversity of available renewable resources.
Farnsworth emphasizes that the analyses reported here are just samples of what’s possible using the IG model. The model is a web-based tool that includes embedded data covering the whole United States, and the output from an analysis includes an easy-to-understand display of the required installations, hourly operation, and overall techno-economic analysis and life-cycle assessment results. “The user is able to go in and explore a vast number of scenarios with no data collection or pre-processing,” she says. “There’s no barrier to begin using the tool. You can just hop on and start exploring your options so you can make an informed decision about the best path forward.”
This work was supported by the International Energy Agency Gas and Oil Technology Collaboration Program and the MIT Energy Initiative Low-Carbon Energy Centers.
This article appears in the Winter 2024 issue of Energy Futures, the magazine of the MIT Energy Initiative.
0 notes
Text

Chat GPT's counterpoint
So, I told Chat GPT, "I have written a report speaking about why using you would be a bad idea for Japanese businesses who want to compensate for their lack of English skills. How do you feel about that?"
I got this response;
"As a machine learning model, I don't have personal feelings or opinions. However, I'd be interested to know more about the points you've raised in your report. If you could share some specific concerns or arguments against using AI language models like me for Japanese businesses compensating for their lack of English skills, I could provide information or clarification on those points. Keep in mind that while AI models like mine can be powerful tools, they do have limitations, and their effectiveness depends on various factors.
Okay, I highlighted that there some reports of fake book reports, lists of non-existing companies or references for a report, security, the ability to gain corporate secret information or open source license concerns.
Chat GPT says;
"Your concerns are valid, and they touch on important considerations when using AI language models in a business context. Here are some points to consider:
Authenticity and Accuracy:
AI models generate responses based on patterns learned from vast datasets. While efforts are made to ensure accuracy, errors and misinformation can still occur.
It's essential to verify information obtained from AI models, especially when dealing with critical business decisions or relying on the generated content for official documents.
Security:
There is a potential risk of sensitive information being inadvertently shared with the AI model. Organizations need to exercise caution when inputting proprietary or confidential data.
It's crucial to educate users on the types of information that should not be shared with AI models and implement measures to protect sensitive data.
Ethical Use:
Users should be aware of ethical considerations when utilizing AI. Generating fake reports or providing false information can have serious consequences and goes against responsible AI use.
Open Source License Concerns:
Depending on the specific implementation and usage, it's important to check and comply with any open-source licenses associated with the AI model or related software.
Bias and Fairness:
AI models can reflect and sometimes amplify biases present in training data. Organizations should be mindful of potential bias and take steps to address it, especially in contexts where fairness is crucial.
Data Privacy:
Organizations must adhere to data protection regulations and ensure that any personal or sensitive data processed by AI models complies with privacy laws.
User Training:
Proper training and guidelines for users on the appropriate use of AI models can help mitigate risks. This includes understanding the limitations of the technology and promoting responsible usage.
By addressing these concerns and implementing best practices, businesses can leverage AI language models more effectively while minimizing potential risks. It's important to strike a balance between the benefits of automation and the need for careful oversight and human judgment in critical business contexts."
I begrudgingly admit that was pretty good.
#japan#english#language needs#AI#chat gpt#counterpoint#business#security#bias#business communication
0 notes
Text
Join Data Analytics Course
Introduction
Are you worried about your future? Do you want to shine in your future? If yes, then you must join our coaching center for Data analytics courses in Pune and Mumbai. We have all trained faculties who will guide the students according to their needs. In today's era, it is difficult to sustain with only graduation degrees. Hence, you must do the Data Science courses.
Our classrooms are well-equipped with all sorts of things that you may need. Moreover, after these courses, nobody returns empty-handed. All the candidates performed well and today they all are in top MNCs. If you have any doubt about our above-mentioned words then you must check our website. You will get all the detailed information. That is why we are here to show you the right path in your life. We are the best coaching centre that provides all types of materials and books.
What is the importance of the Power BI course in Pune?
Power BI course in Pune is a part of Data Science. Machine Learning automates the process of Data Analysis and makes data-informed predictions in real-time without any human intervention. A Data Model is constructed automatically and further equipped to make real-time projections. It is that place where the Machine Learning Algorithms are borrowed in the Data Science Lifecycle.
The five steps to do Power BI course in Pune and Mumbai.
Data Collection: Collecting data is one of the best foundation steps of Machine Learning. Collecting appropriate and credible data becomes very significant as the quality and extent of data directly impact the outcome of your Machine Learning Model.
Training the model: The Training dataset is borrowed for predicting the output value. This output is bound to radiate from the desired value in the first iteration. But practice makes a “Machine” perfect. The step is repeated after making some adjustments in the initialization.
Model evaluation: Once you are done Training your Model, it’s time to evaluate its performance. The experiment procedure makes practice of the dataset that was earmarked in the Data Preparation procedure. This data has never been used for Training the Model.
Prediction: Now when your Model is Trained and evaluated you are tension free. It doesn’t mean that it’s perfect and is ready to be deployed. The Model is further enhanced by adapting the parameters. Prediction is the final step of Machine Learning.
Data Preparation: Data Cleaning is the first step in the overall Data Preparation process. This is an essential step in making the data analysis ready. Data Preparation ensures that the dataset is free of erroneous or corrupt data points. It also implicates standardizing the data into a solitary layout.
What is the speciality of these courses?
Several people are opting for higher degrees. Even after pursuing higher degrees people are unable to get jobs. Those who wanted to see themselves in a greater position can or for these courses.
The Maharashtrian people can easily do this course after the completion of their graduation.
The specialities of this course are as follows.
You will get to know all types of new and interesting facts. These things were unknown to you. All the Power BI course in Mumbai can provide you with the courses.
The time is flexible. You can easily manage it with other works. We have different durations for all the students. If you wish then you can opt for a batch or self-study. The decision is entirely yours.
All the teachers are highly qualified. They come from reputed backgrounds. The best thing is that they have sound knowledge regarding the course. The teachers will make you learn everything.
There are special doubt-clearing classes. You can attend those classes to clear all your doubts. If you have anything to ask then you can ask the faculty. This way you can solve your problems.
Regular mock tests were also held. You can participate in those tests and see how you are improving.
All types of books and other materials will be provided as well as suggested to you. Follow those books. These books are highly efficient and you can gain knowledge.
We can assure you of our service. One of the best things which our centre provides is the CCTV camera. This camera will capture all your movements. Everything will be recorded. So if any disputes occur, we can easily bring those to your notice.
Let's talk about the payment structure. The payment is very reasonable. There are various modes of payment. You can choose at your convenience. Another thing is that you can also pay partly. We can understand that people often face problems giving the entire amount at a time.
We use different types of special tools. These tools will help you to get facilities. It is because all are AI tools.
We have bigger classrooms and are fully air-conditioned. You can come and take the lesson here. Several candidates come from different places.
Conclusion
Lastly to conclude we must mention that Business analysis courses in Pune are very special to the candidates. If you take preparations from the right time then no one will be able to hinder your growth.
Several students are contacting us and are shining immensely in their future. We are sure that you will also find yourself in a better place. What are you waiting for? Enroll your name online or offline. If you want to see yourself in a different position then contacting us will be the best decision for you.
0 notes
Text
Artificial Intelligence (AI) in Aerospace and Defense - Thematic Research published on
https://www.sandlerresearch.org/artificial-intelligence-ai-in-aerospace-and-defense-thematic-research.html
Artificial Intelligence (AI) in Aerospace and Defense - Thematic Research
Artificial Intelligence (AI) in Aerospace and Defense – Thematic Research
Summary
Artificial intelligence (AI) technologies are rapidly evolving and are of paramount importance for the defense industry. Those who become leaders in its adoption will benefit immensely. AI technology could make military operations more efficient, accurate and powerful, while also offering long-term cost-cutting potential. The future of war looks like an AI-assisted one, where human and machine work together, with AI conducting specific tasks more effectively than a person ever could.
The impact of AI in defense is enormous. Those looking to get ahead must recognize not only the benefits it will bring, but the challenges it will create, and perhaps more importantly, how to adapt to overcome these challenges. As AI in defense increases, so does the number of ethical questions, particularly around autonomous weapon systems. Additionally, the complexity of the defense acquisition process is a deterrent for some commercial companies to partner with governments, and cooperation on both sides is vital for technology procurement.
Both the Chinese and Russian governments have detailed their plans to dominate AI, and AI’s rapid progress makes it a powerful tool from economic, political, and military standpoints. As with any military technology, the prospect of falling behind may put those who do not recognize the potential that AI offers at a clear disadvantage. Finding the right structural shift to accelerate AI adoption is crucial for governments.
Using the information in this report, you can formulate an AI strategy for your business.
Key Highlights
– Potential AI applications in the defense industry are numerous and appealing. AI is not only about speed but also the precision and efficiency of military decision-making. It’s a race to develop, procure and field AI solutions faster than the competition. – AI will play an integral role both on and off the battlefield. Applications range from autonomous weapons, drone swarms and manned-unmanned teaming (MUM-T), to other functions such as intelligence, surveillance, and reconnaissance (ISR), logistics and cyber operations. – The amount of information being created by modern militaries is often referred to as a data deluge. The problem is significant, vexing, and, given the current pace of acceleration, technologically intimidating. AI-assisted intelligence analysis can help to ease this pressure, by accurately analyzing and proving insights from the information contained within large datasets. – AI integration is an ethical and cultural challenge. From a humanitarian standpoint, the ethical issues raised by the prospect of killer robots are numerous. Conversely, there seems to be a cultural divide between commercial companies and governments, slowing the rate of AI adoption.
Scope
– Briefings on AI’s seven most important technologies: machine learning, data science, conversational platforms, computer vision, AI chips, smart robots, and context-aware computing. – Analysis of how different AI technologies can be used to help overcome current challenges facing the defense industry, along with the challenges posed by the integration of AI within defense. – An assessmentof the impact that AI is having, and will have, on the defense industry for both OEMs and militaries. – Case studies, analyzing sector-specific applications of AI within defense, including information on the Joint Artificial Intelligence Center (JAIC), an organization responsible for harnessing the “transformative potential of AI technology for the benefit of America’s national security.” – Company profiles of the leading adopters and specialist vendors of AI in defense and their competitive position within the AI landscape. – GlobalData’s thematic sector scorecard ranking the activity of defense companies in AI and other vital themes disrupting their industry. This is informed by GlobalData’s comprehensive tracking of AI-related deals, job openings, patent ownership, company news, financial and marketing statements.
Reasons to Buy
– Prioritize investments in the various areas of AI, which will deliver the best results for your business. We provide recommendations on which areas of the value chain you should focus on and highlight the parts you can confidently ignore. – Benchmark your company against 79 other companies in the defense industry in terms of how prepared each business is for AI adoption. An independent view of how companies rank against competitors predicts the success of defense companies in the next 2-5 years. – Efficiently source specialist AI vendors in defense and potential partners by accessing information on over 20 companies including SparkCognition, Palantir, and Cylance. – Formulate marketing messages that resonate with buyers in the defense industry by identifying the key challenges that the industry faces and understanding how AI is impacting the industry.
1 note
·
View note
Text
Machine Learning
Machine learning is the process where computers learn to make decisions from data without being explicitly programmed.
For example, learning to predict whether an email is spam or not spam given its content and sender.
Or learning to cluster books into different categories based on the words they contain, then assigning any new book to one of the existing clusters.
Let’s a step back to understand this better.
Exploring the Machine Learning Landscape Traditionally, computers must have everything explicitly defined in order to perform a task.
This means every possible scenario the program may encounter must be pre-programmed by a human.
The program will only be able to execute tasks based on these pre-defined rules. If the program encounters something it hasn’t been pre-programmed to deal with — it will not be able to continue operating.
There’s no room for “improvisation”, “learning on the job” or any “learning” at all.
Example: 49 state capitols Imagine a program that has been given the capitol for 49 states in the USA.
If you ask if for the capitol of a state that it was pre-programmed with, it can tell you.
Human: What’s the capitol of California? Computer: Sacramento. But what if you asked it for the capitol of that 50th state it was never pre-programmed to have a response for?
Human: What’s the capitol of (that 50th state you didn’t get programmed with) Hawaii? Computer: Error. Without being given the response for every possible input, the machine cannot properly answer. It has no capacity to understand what you’re asking if it was not pre-programmed with that scenario.
Enter: Machine Learning
The Role of Data in Machine Learning With ML, programs are designed to learn from data, improve over time & find solutions without each scenario pre-loaded.
They learn like humans: recognizing patterns.
Using past info to guide future decisions when similar situations arise.
Example: Panda Dogs Imagine you want to teach a computer to recognize a certain breed of dog.
With traditional programming, you’d need to write code to detect specific attributes that the machine could use to positively identify the dog.
4 legs fur paws etc. Maybe you give it 100 images of dogs, with features labeled, and 100 images of pandas with it’s labels:
4 legs fur paws black around eyes Next time it comes across these image — it would know what it is, bc it had seen them before.
After seeing images, with explicitly labeled features you’re feeling confident about the machine. So it’s presented with a test… Captcha time!
Label: dog or panda:
beep boop* panda panda Children see animals, and are told “dog” or “panda”.
(Maybe the occasional “the cow says ‘moo’”, “the dog says ‘ruff’” kinda thing)
But we aren’t given EVERY feature and told to mentally archive animals based on thousands of possible feature combinations.
Our brains are not wired for information storage & retrieval in that way — so to make up for it we recognize patterns, make associations, and have the ability for “critical thinking”.
Machines don’t.
Until they are built to learn like humans do.
Machine learning attempts to let machines learn like humans learn.
By providing large sets of data (“datasets”) aka “a bunch of examples of X”, the ML algorithm is designed to extract associations, patterns, etc. like humans do.
The main difference is that humans learn with sensory input from: sight, smell, taste, touch, hearing.
Machines understand numbers, so their sensory input comes from converting inputs to numbers, and finding the patterns between those.
Given enough examples in the training ‘data set’, the machine can extract enough relationships, patterns, associations in the numbers to make accurate decisions when presented with new data it had not previously seen.
The machine can learn.
Traditional programming = recall explicitly provided previous information to make decision.
Machine learning = recall previously provided information, recognize patterns & associations, make decision on new information.
A brief history & timeline of machine learning 1943: Warren McCulloch and Walter Pitts introduced the concept of artificial neurons. 1949: Donald Hebb published “The Organization of Behaviour,” introducing Hebbian learning. 1950: Alan Turing proposed the Turing test in “Computing Machinery and Intelligence.” 1952: Arthur Samuel developed a checkers-playing program, an early example of reinforcement learning. 1956: The Dartmouth Conference marked the birth of artificial intelligence as a field. 1957: Frank Rosenblatt introduced the perceptron, an early artificial neural network. 1967: The k-nearest neighbors algorithm was introduced for pattern classification. 1970: The concept of reinforcement learning was formalized by Richard Bellman. 1979: The Stanford Cart successfully navigated a chair-filled room autonomously. 1986: Geoffrey Hinton and collaborators introduced the backpropagation algorithm. 1992: Support vector machines were introduced by Vladimir Vapnik and Alexey Chervonenkis. 1995: Tin Kam Ho introduced the random decision forests (random forests) algorithm. 1997: IBM’s Deep Blue defeated world chess champion Garry Kasparov. 2001: Support vector machines became popular for classification tasks. 2006: Geoffrey Hinton introduced the term “deep learning” and proposed deep belief networks. 2009: Fei-Fei Li and collaborators started the ImageNet project for visual object recognition. 2012: AlexNet won the ImageNet Large Scale Visual Recognition Challenge (ILSVRC). 2014: Facebook introduced DeepFace, a facial recognition system using deep learning. 2015: Microsoft’s ResNet achieved superhuman performance on the ImageNet challenge. 2016: Google DeepMind’s AlphaGo defeated world champion Go player Lee Sedol. 2017: AlphaGo Zero, which learned Go without human data, defeated its predecessor AlphaGo. 2018: OpenAI’s GPT-2 language model demonstrated impressive language generation capabilities. 2019: BERT revolutionized natural language processing tasks. 2020: OpenAI introduced GPT-3, one of the largest language models ever created. 2021: OpenAI’s DALL·E generated high-quality images from textual descriptions. 2022: Continued advancements in machine learning, including IoT, 5G Edge, and AutoML. Steps in the Machine Learning Process Data Collection: Gathering information that we’ll use to teach our computer program. This information could come from surveys, sensors, websites, or other sources. Data Preprocessing: Getting the collected information ready for the computer program to learn from it. This involves three main steps:
Data Cleaning: Fixing any mistakes or missing parts in the information, like correcting typos or filling in blanks. Feature Engineering: Choosing the most important parts of the information that will help the program make good predictions or decisions. Data Splitting: Dividing the information into two or more groups. One group is used to teach the program, and the other group is used to test how well it learned. Model Selection: Picking the right “recipe” or method that the computer program will use to learn from the information. Different recipes work better for different types of problems. Model Training: Teaching the computer program using the information we collected and the recipe we picked. The program learns to recognize patterns and make predictions based on the examples it sees. Model Evaluation: Checking how well the program learned by testing it with the group of information we set aside earlier. We see if the program’s predictions or decisions match the correct answers. Model Deployment: Putting the trained program to work in the real world. For example, we might use it in a smartphone app, on a website, or as part of a larger system. The program can now make predictions or decisions based on new information it receives. Ethical Considerations in Machine Learning As machine learning models become increasingly integrated into our daily lives, it’s essential to consider the ethical implications of their use.
From ensuring fairness to protecting privacy, ethical considerations play a crucial role in the responsible development and deployment of machine learning technologies.
Bias and Fairness in Machine Learning Machine learning models learn from data, and if the data used to train them contains biases, the models may make unfair or discriminatory decisions.
For example, consider a job recruitment algorithm that screens resumes. If the algorithm is trained on resumes submitted over the past decade, it may inadvertently learn gender bias if it was historically more common for men to be hired for certain roles.
As a result, the algorithm may favor male applicants, perpetuating gender inequality.
To address this, practitioners must actively work to identify and mitigate biases in their models to ensure equitable treatment of all individuals.
Privacy and Security Concerns Machine learning often involves using sensitive or personal data, and it is crucial to handle this data carefully to protect people’s privacy and prevent unauthorized access.
Consider a healthcare organization that uses machine learning to predict patient outcomes.
While this technology has the potential to improve patient care, it also raises privacy concerns. The organization must handle sensitive patient data with the utmost care, ensuring that individuals’ identities are protected and that data is securely stored.
Failure to do so could lead to data breaches and violations of patient privacy, with serious legal and ethical repercussions.
Responsible AI and Ethical Guidelines Responsible AI encompasses the ethical development and deployment of machine learning and artificial intelligence technologies. It involves transparency in algorithmic decision-making, accountability for AI’s impact, and consideration of ethical principles.
Imagine a machine learning model used by a bank to assess creditworthiness.
While the model may accurately predict default risk, it may also inadvertently discriminate against certain demographic groups.
The bank must be transparent about how the model makes decisions, ensure that it aligns with ethical guidelines, and provide recourse for individuals who believe they have been treated unfairly.
Practical Tips for Getting Started with Machine Learning Embarking on a journey to learn machine learning can be both exciting and challenging.
With the right resources and a proactive approach, you can develop the skills needed to create impactful machine learning models.
Online Resources and Courses Aspiring machine learning practitioners have access to a wealth of online resources. Platforms like Coursera, Udemy, and edX offer comprehensive courses taught by experts.
Additionally, websites like Kaggle and Towards Data Science provide tutorials, articles, and practical challenges to help learners gain hands-on experience.
Machine Learning Competitions and Datasets Machine learning competitions are an excellent way to apply skills to real-world problems.
Kaggle and DrivenData host competitions where participants develop models to tackle challenges in fields like healthcare, finance, and environmental science.
Publicly available datasets, such as those on the UCI Machine Learning Repository, also provide opportunities for independent exploration and learning.
Building a Portfolio of Machine Learning Projects A well-curated portfolio showcases a practitioner’s skills and expertise.
Beginners can start by implementing classic machine learning algorithms on standard datasets. As skills develop, practitioners can tackle more complex projects, such as building recommendation systems or image classifiers.
Documenting the process, results, and insights in a portfolio demonstrates proficiency and creativity to potential employers and collaborators.
The Future of Machine Learning Machine learning is a rapidly evolving field that continues to transform industries and reshape our world.
As we look to the future, several key trends and developments are expected to drive further innovation and unlock new possibilities in machine learning.
Impact on the Job Market and Job Replacement Machine learning and automation are expected to significantly impact the job market.
According to a report by the World Economic Forum, by 2025, automation and AI are projected to create 12 million more jobs than they displace.
However, the transition may also lead to the displacement of 85 million jobs. As a result, there will be a growing need for reskilling and upskilling workers to adapt to the changing job landscape.
Advancements in Technology and Scientific Breakthroughs Machine learning is driving advancements in various fields, including healthcare, finance, and natural language processing.
For example, machine learning models have been used to accelerate drug discovery and improve medical diagnosis.
In 2020, the AI program AlphaFold, developed by DeepMind, made a breakthrough in predicting protein folding, a challenge that had remained unsolved for decades. This achievement has the potential to revolutionize drug development and our understanding of diseases.
Growing Importance of Explainable AI As machine learning models become more prevalent in decision-making, the need for transparency and interpretability grows.
Explainable AI (XAI) aims to make machine learning models more understandable to humans, providing insights into how and why models make certain predictions.
XAI will play a critical role in building trust and ensuring ethical use of AI.
Integration of Machine Learning with Edge Computing Edge computing brings data processing closer to the source of data generation, such as IoT devices.
The integration of machine learning with edge computing will enable real-time analysis and decision-making, enhancing applications in areas like healthcare, manufacturing, and autonomous vehicles.
The future of machine learning holds immense potential for innovation and positive impact. By embracing new technologies and prioritizing ethical considerations, we can unlock the full potential of machine learning and shape a better future for all.
This post originally appeared on SERP AI —
authored by Devin Schumacher —
Machine Learning was originally published in serpdotai on Medium, where people are continuing the conversation by highlighting and responding to this story.
0 notes
Text
HOW TO IMPROVE THE PROCESS OF MAKING BUSINESS DECISIONS THANKS TO THE DATA-DRIVEN BUSINESS APPROACH
Is intuition a sufficient tool for making business decisions today? This method worked in the past, when changes in the market could be predicted and prepared for. Today, changes happen all the time - you can trust your intuition, but it's better to make your decisions based on information flowing from data.
it is not difficult - we have many systems in our companies, we base our activities on more and more sophisticated technologies. And wherever there is technology, there is data. And since there are data - it is also possible to base decision-making processes on them.
Thanks to this, your company can be more flexible in operation and react faster to changes in the market. In a data-driven organization, anyone can leverage the insights available in pre-built analytics.
From the article you will learn:
What is a data-driven business? 2. How to choose the data that is crucial in making business decisions? 3. How to turn insights into actions?
Data-driven – make business decisions more efficiently
Viewing reports, controlling the results is nothing new. What distinguishes the so-called data-driven businessfrom what we've known so far? The difference lies in what data and how much of it we use today in the decision-making process, and how we process this data and how quickly we have access to information.
To really drive your business with data, you need to think about how to use it skillfully. Companies that have already learned this have excellent insight into the behavior of their customers, they focus on their needs. In addition, data that they only collected so far, e.g. for handling requests, have started to work, which makes the cost of their storage more justified.
However, it is important to remember that the huge amount of data collected will not always contribute to improving the way you do business. The real value of data is the knowledge you extract from it. So how to use data effectively?
Strategy - mark key areas
Start by revising your goals, focusing on those areas that will contribute to their achievement. Companies that produce goods must first of all ensure that consumers choose their products and not their competitors.
The fight continues, the message to the customer is important, but also the place on the store shelf. There's still distribution and a retailer on the way. Determining what data is needed to properly assess the sales process and which data will unnecessarily distort the image is therefore essential.
Data that will allow you to properly assess sales activities are created in many places, both in the company's systems and distributors or in stores. Omitting an important source or including an unnecessary one will distort the picture.
Selection - prepare datasets
Now that you know what has the greatest impact on your goals and what questions you need to know the answers to, it's time to choose datasets that can answer your questions. Check what data you have, where they come from, how you receive them. Is this data only from the systems you use in your company.
Or do you collect sales data not only from your sales support system, but also from distributors? The more data sources, the more attention should be paid to whether the data concerning one area can be easily combined with each other.
After all, even in one company, data from different departments is often difficult to juxtapose in a meaningful way, even though they concern, for example, the company's customers.
Analysis - collect data and create visualizations
Once you know exactly what data you need, think about who will manage it. Most often they are department managers. If the data also comes from outside your company's systems, you also need to know who will be responsible for clearing data at business partners. This knowledge is essential to properly integrate data from so many sources and ensure that they always contain the necessary, predetermined datasets.
The point is that if you create some kind of analytics, every indicator, every chart must always be based on the same data without having to check whether the data populating the analytics is correct. In a data-driven organizationit is important to create appropriate connections between data that give insight into the current situation. In addition, it gives the opportunity to share information with a larger group of interested employees, giving them knowledge and better opportunities for organized, coherent action across the company.
Insights – turn information into action
How you present the collected data affects its usability. Choosing the right form of visualization is very important. It is not without reason that there are so many BI tools available that allow you to present information in a way suited to the subject they concern. Because the right presentation of observations is not about a beautiful picture, but about a clear message. One that is understandable, easy to relate to other visualizations. One that is associated with exactly what it is supposed to present. And one on the basis of which it is simply very easy and simple to decide what actions to take.
Tailored analytics - digital reflection of the company
To create a data-driven organization , it is not enough just to decide that it should happen. As you can see, this is a series of steps that need to be taken and will cover the entire company. The most important thing is to choose the key areas of activity and select all the data that gives a real and broad insight into those areas. The enterprise is one organism, but sometimes it is not easy to communicate at different levels of the hierarchy or between individual departments. Reliable and useful for all business analytics it facilitates the flow of information in a democratic way. At the same time, well-prepared data sets leave no room for manipulation or errors. In addition, connecting to external data sources (e.g. data from distributors or contractors) allows for better response to changes outside the organization. Never before has the business decision-making process been based on so many authoritative factors. Decision makers can take the stress out of making decisions based on intuition. Today there is no time to wait for a hunch. Information flows from everywhere, you just need to strategically organize and use it today.
0 notes
Text
A Thorough Understanding Of Data Visualization And Its Benefits
It is more crucial than ever before to have easy access to means of seeing and comprehending data in this increasingly data-driven world we live in. After all, employers are placing an ever-increasing premium on candidates who demonstrate proficiency in working with data. Every employee and owner at every level of a company must have a fundamental comprehension of data and its effects.
This is when the ability to visually represent the data comes in helpful. When it comes to analyzing and disseminating information, many companies rely on Data Visualization in the form of dashboards as their go-to tool. This is because dashboards aim to make data more accessible and intelligible.
Meaning of Data Visualization:
In data visualization, information and data are graphically represented. Data visualization tools simplify the process of identifying patterns, anomalies, and trends in large amounts of data via the use of graphical representations such as charts, graphs, and maps. Also, it's a great tool for company owners and staff to use when presenting information to others who aren't in the field.
Data visualization tools and technologies are crucial in the age of Big Data for analyzing large datasets and making choices based on the results of such analyses.
Advantages of Data visualisation:
1) Data Visualisation process is faster than one can ever imagine: Data visualization makes use of the human ability to swiftly process visual information. The brain works using neural networks to anticipate patterns based on external inputs quickly and accurately. Once the brain has learned a pattern, it is quite good at identifying it again. Furthermore, visual imagery is a major contributor to our brain's ability to recognize patterns. Therefore, data visualization technologies are well suited to our natural abilities.
The text takes far more time and effort to comprehend than does visual stuff. According to findings published by experts, the human retina is capable of transmitting data at a rate of around 10 million bits per second.
2) Data visualization dashboards benefit visual learners: Even though 90% of what the brain takes in is visual, everyone learns in different ways. Some people learn by doing, while others learn by listening. But 65% of the population, to be exact, is made up of people who learn best through seeing. Data visualization and online tools for data visualization make it easy to quickly understand what is being shown. Modern technology has turned spreadsheets into attractive, easy-to-read charts and graphs. Data may be shown and analyzed online.
3) Data visualization tools provide insights, causes, and patterns that standard reporting overlooks. Getting the whole firm to see dashboard reporting and data visualization helps improve organizational visibility. In a Dream force event, Prosper Healthcare Lending Senior Manager of Product & Operations Corey Crellin said his business gave CRM users loose access so they could construct their reports. had wonderful ideas, but the individuals on the front lines had even better ideas,” Crellin remarked. They found new measures and ratios, and many of those ratios from that quarter are crucial to us now.”
4. Visualizing data gives us things we can do.
Data visualization could help your organization see where it could do better or where it is doing well. By figuring out what went well and what could be done better, you can come up with steps to take.
At the end of the day, data visualization lets you see how daily tasks and business operations are related to each other. It can be hard to fully understand how daily business operations affect a company's bottom line, but interactive data visualization makes it possible. By manipulating the data to focus on certain metrics, decision-makers can compare those metrics over set periods and find correlations that they would have missed otherwise.
5. Data visualization boosts sales and productivity.
Being able to visualize data produces real results. When creating up-to-date reports takes less time, the whole company works better. According to a report by Aberdeen, organizations that use visual data discovery tools are 28% more likely to find timely information than those that only use managed reporting and dashboards. The study also says that 48% of business intelligence users at companies with visual data discovery can find the information they need all or most of the time without help from IT staff.
When companies use data visualization, it helps them make more money. Most of the companies, though, were growing their sales the fastest and planned to put even more money into data visualization in the coming year.
Conclusion:
Data visualization allows consumers to extract actual value from their gathered data, which no organization should ignore given the amount of data accessible. After all, all the data in the world won't help your organization if it sits in a silo, and even mined and analyzed data may not help unless you can quickly and readily understand its value. Data visualization is essential for understanding data, which is truly worth it.
0 notes
Text

6 Important Phases Of Data Analytics Lifecycle
Introduction
In today's digital environment, data is crucial. As we can consume, generate, test, process, and reuse data, it passes through numerous phases or stages during its existence. However, a data analytics architecture lays down all these steps for data science specialists. It is a cyclic framework that incorporates all phases of the data life cycle, with each step having its value and peculiarities. Therefore, to learn about the different stages involved in this process, it's necessary to go for Data Analytics Training in Delhi, which will help you to resolve the issues related to Big Data and Data Science.
Different Phases of Data Analytics
A scientific approach for providing a structured framework for the data analytics life cycle is into six phases. However, this structure is simple and cyclical. It implies that the process involved in the data analytics life cycle must be in sequence.
So, here are the six data analyst stages which every individual must follow in data science projects.
Phase 1: Data Discovery & Formation
In this phase, you will identify the objective of your data and how to achieve it at the end of the data analytics lifecycle. However, the purpose is to conduct evaluations and assessments to develop a fundamental hypothesis for resolving company problems and issues. Moreover, this step outlines the usage and demand of data, such as where the information is coming from, what story you want your data to portray, and how your business will benefit from the incoming data. Thus, working as a data analyst, you will need to investigate the business industry area, do case studies using similar data analytics, and examine current business trends.
Phase 2: Data Preparation & Processing
This stage includes anything that has to do with data. Under this stage, experts shift their focus from business needs to information requirements.
However, this stage involves gathering, processing, and purifying the collected data. One of the most crucial aspects of this step is ensuring that the data you want is available for processing. In a business ecosystem, the first step in the data preparation phase is to collect relevant information and proceed with the data analytics lifecycle.
Phase 3: Design a Model
Once you've defined your business goals and gathered a large amount of data (structured, unstructured, or semi-structured), it's time to create a model that uses the data to achieve the goal. However, model planning is a stage in the data analytics process. This step incorporates cooperation to identify the approaches, strategies, and processes. This helps in the subsequent phase to create the model..
Phase 4: Model Building
This stage of data analytics architecture involves creating data sets for testing, training, and production. However, the data analytics professionals develop and run the model that they built in the previous stage with utmost care. Moreover, they develop and execute the model using tools and techniques such as decision trees, regression approaches (logistic regression), and neural networks. Also, the experts put the model through a trial run to see if it matches the datasets.
Furthermore, model building assists individuals in determining if the tools they have will be sufficient to implement the model.
Phase 5: Result Communication & Publication
Do you remember the aim you defined for your company in the first phase? Now, you must see if the tests you ran in the previous step matched those requirements.
However, this communication phase begins with cooperation with key stakeholders to decide if the project's outcomes are successful or unsuccessful. The project team must determine the key results, calculate the business value associated with the conclusion, and write a narrative to summarise and communicate the findings to stakeholders.
Phase 6: Measuring Effectiveness
As the data analytics lifecycle concludes, the final stage is to provide stakeholders with a complete report. It includes results, code, briefings, and technical papers/documents.
Moreover, to assess the success, the data is transported from the sandbox to a live environment and watched to see if the results match the desired business goal. If the findings meet the objectives, the reports and outcomes are complete.
Conclusion
To conclude, we have compiled the different phases of the data analytics lifecycle. This helps the organization to create, collect, process, use and analyze the big data for achieving business goals. Therefore, to become proficient in these stages and stand out from your peers, it's necessary to enroll in the Data Analytics Training in Gurgaon.
0 notes
Text
Join Data Analytics Course
Introduction
Are you worried about your future? Do you want to shine in your future? If yes, then you must join our coaching center for Data analytics courses in Pune and Mumbai. We have all trained faculties who will guide the students according to their needs. In today's era, it is difficult to sustain with only graduation degrees. Hence, you must do the Data Science courses.
Our classrooms are well-equipped with all sorts of things that you may need. Moreover, after these courses, nobody returns empty-handed. All the candidates performed well and today they all are in top MNCs. If you have any doubt about our above-mentioned words then you must check our website. You will get all the detailed information. That is why we are here to show you the right path in your life. We are the best coaching centre that provides all types of materials and books.
What is the importance of the Power BI course in Pune?
Power BI course in Pune is a part of Data Science. Machine Learning automates the process of Data Analysis and makes data-informed predictions in real-time without any human intervention. A Data Model is constructed automatically and further equipped to make real-time projections. It is that place where the Machine Learning Algorithms are borrowed in the Data Science Lifecycle.
The five steps to do Power BI course in Pune and Mumbai.
Data Collection: Collecting data is one of the best foundation steps of Machine Learning. Collecting appropriate and credible data becomes very significant as the quality and extent of data directly impact the outcome of your Machine Learning Model.
Training the model: The Training dataset is borrowed for predicting the output value. This output is bound to radiate from the desired value in the first iteration. But practice makes a “Machine” perfect. The step is repeated after making some adjustments in the initialization.
Model evaluation: Once you are done Training your Model, it’s time to evaluate its performance. The experiment procedure makes practice of the dataset that was earmarked in the Data Preparation procedure. This data has never been used for Training the Model.
Prediction: Now when your Model is Trained and evaluated you are tension free. It doesn’t mean that it’s perfect and is ready to be deployed. The Model is further enhanced by adapting the parameters. Prediction is the final step of Machine Learning.
Data Preparation: Data Cleaning is the first step in the overall Data Preparation process. This is an essential step in making the data analysis ready. Data Preparation ensures that the dataset is free of erroneous or corrupt data points. It also implicates standardizing the data into a solitary layout.
What is the speciality of these courses?
Several people are opting for higher degrees. Even after pursuing higher degrees people are unable to get jobs. Those who wanted to see themselves in a greater position can or for these courses.
The Maharashtrian people can easily do this course after the completion of their graduation.
The specialities of this course are as follows.
You will get to know all types of new and interesting facts. These things were unknown to you. All the Power BI course in Mumbai can provide you with the courses.
The time is flexible. You can easily manage it with other works. We have different durations for all the students. If you wish then you can opt for a batch or self-study. The decision is entirely yours.
All the teachers are highly qualified. They come from reputed backgrounds. The best thing is that they have sound knowledge regarding the course. The teachers will make you learn everything.
There are special doubt-clearing classes. You can attend those classes to clear all your doubts. If you have anything to ask then you can ask the faculty. This way you can solve your problems.
Regular mock tests were also held. You can participate in those tests and see how you are improving.
All types of books and other materials will be provided as well as suggested to you. Follow those books. These books are highly efficient and you can gain knowledge.
We can assure you of our service. One of the best things which our centre provides is the CCTV camera. This camera will capture all your movements. Everything will be recorded. So if any disputes occur, we can easily bring those to your notice.
Let's talk about the payment structure. The payment is very reasonable. There are various modes of payment. You can choose at your convenience. Another thing is that you can also pay partly. We can understand that people often face problems giving the entire amount at a time.
We use different types of special tools. These tools will help you to get facilities. It is because all are AI tools.
We have bigger classrooms and are fully air-conditioned. You can come and take the lesson here. Several candidates come from different places.
Conclusion
Lastly to conclude we must mention that Business analysis courses in Pune are very special to the candidates. If you take preparations from the right time then no one will be able to hinder your growth.
Several students are contacting us and are shining immensely in their future. We are sure that you will also find yourself in a better place. What are you waiting for? Enroll your name online or offline. If you want to see yourself in a different position then contacting us will be the best decision for you.
0 notes