#table1
Explore tagged Tumblr posts
Text
DON'T STEP ON THE TABLE1!!! People are eating there rn!!!!!!
3 notes
·
View notes
Link
#10-seaterdiningtablecustomsize#10-seaterdiningtableIndia#buydiningtablewithmarbletop#customizablediningtableIndia#diningtablesetforlargefamilies#diningtablesetwithmarbletop#diningtablewith10chairsonline#luxurydiningtablesetfor10#luxuryhomedécordiningtableIndia#luxurymarblediningtableset#marbleandsteeldiningtableset#marbletopdiningtableIndia#moderndiningtablesetIndia#premiumdiningtableonlineIndia#Shopps.indiningtableset#stainlesssteeldiningtableIndia
0 notes
Text
Capstone Milestone Assignment 2: Methods
Full Code: https://github.com/sonarsujit/MSC-Data-Science_Project/blob/main/capstoneprojectcode.py
Methods
1. Sample: The data for this study was drawn from the World Bank's global dataset, which includes comprehensive economic and health indicators from various countries across the world. The final sample consists of N = 248 countries, and I am only looking at the 2012 data for this sample data.
Sample Description: The sample includes countries from various income levels, regions, and development stages. It encompasses both high-income countries with advanced healthcare systems and low-income countries where access to healthcare services might be limited. The sample is diverse in terms of geographic distribution, economic conditions, and public health outcomes, providing a comprehensive view of global health disparities.
2. Measures: The given dataset has 86 variables and form the perspective of answering the research question, I focused on life expectancy and access to healthcare services. The objective is to look into these features statistically and narrow down to relevant and important features that will align to my research problem.
Here's a breakdown of the selected features and how they relate to my research:
Healthcare Access and Infrastructure: Access to electricity, Access to non-solid fuel,Fixed broadband subscriptions, Improved sanitation facilities, Improved water source, Internet users
Key Health and Demographic Indicators: Adolescent fertility rate, Birth rate, Cause of death by communicable diseases and maternal/prenatal/nutrition conditions, Fertility rate, Mortality rate, infant , Mortality rate, neonatal , Mortality rate, under-5, Population ages 0-14 ,Urban population growth
Socioeconomic Factors: Population ages 65 and above, Survival to age 65, female, Survival to age 65, male, Adjusted net national income per capita, Automated teller machines (ATMs), GDP per capita, Health expenditure per capita, Population ages 15-64, Urban population.
Variable Management:
The Life Expectancy variable was used as a continuous variable in the analysis.
All the independent variables were also used as continuous variables.
Out of the 84 in quantitative independent variables, I found that the above 26 features closely describe the health access and infrastructure, key health and demographic indicators and socioeconomic factors based on the literature review.
I run the Lasso regression to get insights on features that will align more closely with my research question
Table1: Lasso regression to find important features that support my research question.
Based on the result from lasso regression, I finalized 8 predictor variables which I believe will potentially help me answer my research question.
To further support the selection of these 8 features, I run Correlation Analysis for these 8 features and found to have both positive and negative correlations with the target variable (Life Expectancy at Birth, Total (Years))
Table 2: Pearson Correlation values and relative p values
The inclusion of both positive and negative correlations provides a balanced view of the factors affecting life expectancy, making these features suitable for your analysis.
Incorporating these variables should allow us to capture the multifaceted relationship between healthcare access and life expectancy across different countries, and effectively address our research question.
Analyses:
The primary goal of the analysis was to explore and understand the factors that influence life expectancy across different countries. This involved using Lasso regression for feature selection and Pearson correlation for assessing the strength of relationships between life expectancy and various predictor variables.
The Lasso model revealed that factors such as survival rates to age 65, health expenditure, broadband access, and mortality rate under 5 were the most significant predictors of life expectancy.
The mean squared error (MSE) = 1.2686 of the model was calculated to assess its predictive performance.
Survival to age 65 (both male and female) had strong positive correlations with life expectancy, indicating that populations with higher survival rates to age 65 tend to have higher overall life expectancy.
Health expenditure per capita showed a moderate positive correlation, suggesting that countries investing more in healthcare tend to have longer life expectancy.
Mortality rate, under-5 (per 1,000) had a strong negative correlation with life expectancy, highlighting that higher child mortality rates are associated with lower life expectancy.
Rural population (% of total) had a negative correlation, indicating that countries with a higher percentage of rural populations might face challenges that reduce life expectancy.
0 notes
Text
Chamorro
Chamorro (pork shank) Méxican Chamorro via Instant Pot pressure cooker Instant Pot Pressure CookerSheet Pan, 1/4Spoon, slotted 2 ea Chamorro (Pork Shank) (without skin)1 tbsp Salt, table1 tbsp Oregano, Méxican4 cups Water Place the chamorro on the sheet pan and cover liberally with the salt and Méxican Oregano. Place the sheet pan in the refrigerator for a minimum of 30 minutes.Place 4 cups…

View On WordPress
0 notes
Text
how is it colonialism to say that the indigenous people that never left the land should have their land back?? rather than people who made homes in other nations for thousands of years getting to take their land and home from them?
the ACTUAL original phrase in arabic, by the way, is not what that westerner wrote nor what this liar claimed! it actually is “we will not accept anything but the freeing of palestine from the river to the sea”. that person in the video wrote the arabic version entirely wrong, it’s not “sea to sea” in the arabic version, and it’s not “palestine will be arab” in the arabic version. these are lies to support the colonisation of palestine, and yes this is colonisation. this reverse uno card does not work when numerous genetic tests have proven over and over again that palestinians are indigenous.

if you search “from the river to the sea palestine will be” in arabic:

ALL the top results say “palestine will be free”, or alternatively “it is palestine, from the river to the sea”. so this person was either misinformed or, what is more likely, intentionally choosing an example of an ignorant westerner who wrote the arabic phrase incorrectly to then mislead others. now for your other lies that you spewed to @chiefmuffinmuncher

interesting how nowhere in your comment here did you include any actual studies into this. you have to rely on sourceless conspiracies because if you looked at the research, you'd see palestinian indigeneity has been proven over and over again. here's some sources:
“Remarkably, AJs exhibit a dominant Iranian (88%) and residual Levantine (3%˜) ancestries, as opposed to Bedouins (14%˜ and 68%˜, respectively) and Palestinians (18%˜ and 58%˜, respectively). Only two AJs exhibit Levantine ancestries typical to Levantine populations… Overall, the combined results are in a strong agreement with the predictions of the Irano-Turko-Slavic hypothesis (Table (Table1)1) and rule out an ancient Levantine origin for AJs, which is predominant among modern-day Levantine populations (e.g., Bedouins and Palestinians).”
so this study found that ashkenazi jewish people to not be levantine people, whereas palestinians are. but this is just one study. what do other studies say?
“Two major differences among the populations in this study were the high degree of European admixture (30%–60%) among the Ashkenazi, Sephardic, Italian, and Syrian Jews and the genetic proximity of these populations to each other compared to their proximity to Iranian and Iraqi Jews. This time of a split between Middle Eastern Iraqi and Iranian Jews and European/Syrian Jews, calculated by simulation and comparison of length distributions of IBD segments, is 100–150 generations, compatible with a historical divide that is reported to have occurred more than 2500 years ago.2,5 The Middle Eastern populations were formed by Jews in the Babylonian and Persian empires who are thought to have remained geographically continuous in those locales…
Besides Southern European groups, the closest genetic neighbors to most Jewish populations are the Palestinians, Bedouins, and Druze. The observed differentiation of these groups reflects their histories of within-group endogamy. Yet, their genetic proximity to one another and to European and Syrian Jews suggests a shared genetic history of related Middle Eastern and non-Semitic Mediterranean ancestors who chose different religious and tribal affiliations. These observations are supported by the significant overlap of Y chromosomal haplogroups between Israeli and Palestinian Arabs with Ashkenazi and non-Ashkenazi Jewish populations that has been described previously. Likewise, a study comparing 20 microsatellite markers in Israeli Jewish, Palestinian, and Druze populations demonstrated the proximity of these two non-Jewish populations to Ashkenazi and Iraqi Jews.”
(to simplify it for you, ashkenazim are more similar to southern europeans. after europeans, they’re closest genetically to palestinians. palestinians are the closest genetically to jewish people overall, indicating a common ancestry.
to spell it out for you, that means palestinians are just the people who never left whereas jewish ppl left palestine and mixed with other populations.)
maybe you'll argue two studies aren't enough to disprove your lies, okay, lets see what even more research shows
“Israeli and Palestinian Arabs share a similar linguistic and geographic background with Jews. (p.631) According to historical records part, or perhaps the majority, of the Moslem Arabs in this country descended from local inhabitants, mainly Christians and Jews, who had converted after the Islamic conquest in the seventh century AD (Shaban 1971; Mc Graw Donner 1981). These local inhabitants, in turn, were descendants of the core population that had lived in the area for several centuries, some even since prehistorical times (Gil 1992). On the other hand, the ancestors of the great majority of present-day Jews lived outside this region for almost two millennia. Thus, our findings are in good agreement with historical evidence and suggest genetic continuity in both populations despite their long separation and the wide geographic dispersal of Jews.(p.637)” (Nebel, 2000)
this study found the same, which is that the people of the levant, including palestinians, are descendants of the canaanites.. an indigenous group to the levant:
were the ancient canaanites perhaps also pesky arab invaders, then? or does converting to islam somehow change you from indigenous to your own country to a coloniser? somehow, i dont think that's how colonisation works at all.
but maybe that's still not enough for you, so lets look at even more
“The closest genetic neighbors to most Jewish groups were the Palestinians, Israeli Bedouins, and Druze in addition to the Southern Europeans, including Cypriots. The genetic clusters formed by each of these non-Jewish Middle Eastern groups reflect their own histories of endogamy. Their proximity to one another and to European and Syrian Jews suggested a shared genetic history of related Semitic and non-Semitic Mediterranean ancestors who followed different religious and tribal affiliations. Earlier studies of Israeli Jewish, Palestinian and Druze populations made a similar observation by demonstrating the proximity of these two non-Jewish populations to Ashkenazi and Iraqi Jews (Rosenberg et al. 2001; Kopelman et al. 2009).”
“The comparison with other Mediterranean populations by using neighbor-joining dendrograms and correspondence analyses reveal that Palestinians are genetically very close to Jews and other Middle East populations, including Turks (Anatolians), Lebanese, Egyptians, Armenians, and Iranians. Archaeologic and genetic data support that both Jews and Palestinians came from the ancient Canaanites, who extensively mixed with Egyptians, Mesopotamian, and Anatolian peoples in ancient times. Thus, Palestinian-Jewish rivalry is based in cultural and religious, but not in genetic, differences.”
Our recent study of high-resolution microsatellite haplotypes demonstrated that a substantial portion of Y chromosomes of Jews (70%) and of Palestinian Muslim Arabs (82%) belonged to the same chromosome pool (Nebel et al. 2000). Of those Palestinian chromosomes, approximately one-third formed a group of very closely related haplotypes that were only rarely found in Jews. Altogether, the findings indicated a remarkable degree of genetic continuity in both Jews and Arabs, despite their long separation and the wide geographic dispersal of Jews.
pretending like palestinians are somehow the Real Colonisers for simply having existed in their own country and their ancestors converting to islam & christianity is beyond bizarre. so this was just zionist lies upon lies to justify genocide and colonialism, while pretending that actually wanting palestinian liberation is somehow wanting genocide and colonialism. disgusting
Posted @withregram • @adielofisrael “From the (Jordan) river to the (Mediterranean) sea, Palestine will be free (of Jews)”.
This is how you should read it because this is what it means. It is just sugarcoated call for genocide of the Jewish people and ethnic cleansing of Jews from our indigenous homeland. It is not de-colonial, it is the epitome of Arab colonialism and a remnant of the Palestinian Arab collaboration with Nazi Germany under the leadership of Hajj Amin Al Husseini, who coined this phrase.
#israel #palestine #gaza #indigenous
[angrybell: but given what we have seen from the “Free Palestine” crowd, I don’t think that they care the Jewish people will be killed off to make way for their Arab utopia. Its not a bug for them, its a feature.]
#israel#palestine#zionism#also checked in arabic all variations of palestine will be free in arabic#vs all variations of 'palestine will be arab'#there were more than twice as many using the term FREE not arab. overall about 699 results for arab.. 30000 for free#the original was free. the common slogan is free. the translation says free. the people say free.#but zionists have to lie so theyll tell u that the actual arabic for it is 'palestine will be arab'
516 notes
·
View notes
Text
1. Управління даними:
1.1 Кодування відсутніх даних:
У наборі даних NESARC пропущені значення позначені -99.
Заміню ці значення на NA (недоступно) у Python.
1.2 Кодування достовірних даних:
Деякі змінні, такі як DRNKQ1, містять нечислові значення, наприклад, 'Refused' або 'Don't Know'.
Заміню ці значення на відповідні коди, наприклад, 99 для 'Refused' та 98 для 'Don't Know'.
1.3 Перекодування змінних:
Деякі змінні, такі як GAD7 та PHQ9, мають шкали, які не відповідають моєму аналізу.
Перекодую ці змінні, щоб створити нові змінні, які відповідають моїм потребам.
1.4 Створення вторинних змінних:
За потреби створю нові змінні, які є комбінацією існуючих змінних.
1.5 Розбиття або групування змінних:
За потреби розбиваю або групую змінні на основі певних критеріїв.
import pandas as pd
#Завантаження набору даних NESARC
nesarc = pd.read_csv("NESARC_Public_Use_Dataset_2013_01_14.csv")
#Кодування відсутніх даних
nesarc.replace(-99, np.NA, inplace=True)
#Кодування достовірних даних
nesarc["DRNKQ1"].replace("Refused", 99, inplace=True) nesarc["DRNKQ1"].replace("Don't Know", 98, inplace=True)
#Перекодування змінних
nesarc["GAD7_binary"] = (nesarc["GAD7"] >= 10).astype(int) nesarc["PHQ9_binary"] = (nesarc["PHQ9"] >= 10).astype(int)
#Створення вторинних змінних
nesarc["overall_mental_health"] = nesarc["GAD7_binary"] + nesarc["PHQ9_binary"]
#Розбиття змінних
nesarc_men = nesarc[nesarc["SEX"] == 1] nesarc_women = nesarc[nesarc["SEX"] == 2]
#Запуск розподілів частот
table1 = pd.crosstab(nesarc["DRNKQ1"], nesarc["GAD7_binary"]) table2 = pd.crosstab(nesarc["DRNKQ1"], nesarc["PHQ9_binary"]) table3 = pd.crosstab(nesarc["DRNKQ1"], nesarc["overall_mental_health"]) table4 = pd.crosstab(nesarc["DRNKQ1"], [nesarc["GAD7_binary"], nesarc["PHQ9_binary"]])
#Відображення результатів
print(table1) print(table2) print(table3) print(table4)
#Аналіз результатів
#… (інтерпретація результатів)
0 notes
Text
[Python] PySpark to M, SQL or Pandas
Hace tiempo escribí un artículo sobre como escribir en pandas algunos códigos de referencia de SQL o M (power query). Si bien en su momento fue de gran utilidad, lo cierto es que hoy existe otro lenguaje que representa un fuerte pie en el análisis de datos.
Spark se convirtió en el jugar principal para lectura de datos en Lakes. Aunque sea cierto que existe SparkSQL, no quise dejar de traer estas analogías de código entre PySpark, M, SQL y Pandas para quienes estén familiarizados con un lenguaje, puedan ver como realizar una acción con el otro.
Lo primero es ponernos de acuerdo en la lectura del post.
Power Query corre en capas. Cada linea llama a la anterior (que devuelve una tabla) generando esta perspectiva o visión en capas. Por ello cuando leamos en el código #“Paso anterior” hablamos de una tabla.
En Python, asumiremos a "df" como un pandas dataframe (pandas.DataFrame) ya cargado y a "spark_frame" a un frame de pyspark cargado (spark.read)
Conozcamos los ejemplos que serán listados en el siguiente orden: SQL, PySpark, Pandas, Power Query.
En SQL:
SELECT TOP 5 * FROM table
En PySpark
spark_frame.limit(5)
En Pandas:
df.head()
En Power Query:
Table.FirstN(#"Paso Anterior",5)
Contar filas
SELECT COUNT(*) FROM table1
spark_frame.count()
df.shape()
Table.RowCount(#"Paso Anterior")
Seleccionar filas
SELECT column1, column2 FROM table1
spark_frame.select("column1", "column2")
df[["column1", "column2"]]
#"Paso Anterior"[[Columna1],[Columna2]] O podría ser: Table.SelectColumns(#"Paso Anterior", {"Columna1", "Columna2"} )
Filtrar filas
SELECT column1, column2 FROM table1 WHERE column1 = 2
spark_frame.filter("column1 = 2") # OR spark_frame.filter(spark_frame['column1'] == 2)
df[['column1', 'column2']].loc[df['column1'] == 2]
Table.SelectRows(#"Paso Anterior", each [column1] == 2 )
Varios filtros de filas
SELECT * FROM table1 WHERE column1 > 1 AND column2 < 25
spark_frame.filter((spark_frame['column1'] > 1) & (spark_frame['column2'] < 25)) O con operadores OR y NOT spark_frame.filter((spark_frame['column1'] > 1) | ~(spark_frame['column2'] < 25))
df.loc[(df['column1'] > 1) & (df['column2'] < 25)] O con operadores OR y NOT df.loc[(df['column1'] > 1) | ~(df['column2'] < 25)]
Table.SelectRows(#"Paso Anterior", each [column1] > 1 and column2 < 25 ) O con operadores OR y NOT Table.SelectRows(#"Paso Anterior", each [column1] > 1 or not ([column1] < 25 ) )
Filtros con operadores complejos
SELECT * FROM table1 WHERE column1 BETWEEN 1 and 5 AND column2 IN (20,30,40,50) AND column3 LIKE '%arcelona%'
from pyspark.sql.functions import col spark_frame.filter( (col('column1').between(1, 5)) & (col('column2').isin(20, 30, 40, 50)) & (col('column3').like('%arcelona%')) ) # O spark_frame.where( (col('column1').between(1, 5)) & (col('column2').isin(20, 30, 40, 50)) & (col('column3').contains('arcelona')) )
df.loc[(df['colum1'].between(1,5)) & (df['column2'].isin([20,30,40,50])) & (df['column3'].str.contains('arcelona'))]
Table.SelectRows(#"Paso Anterior", each ([column1] > 1 and [column1] < 5) and List.Contains({20,30,40,50}, [column2]) and Text.Contains([column3], "arcelona") )
Join tables
SELECT t1.column1, t2.column1 FROM table1 t1 LEFT JOIN table2 t2 ON t1.column_id = t2.column_id
Sería correcto cambiar el alias de columnas de mismo nombre así:
spark_frame1.join(spark_frame2, spark_frame1["column_id"] == spark_frame2["column_id"], "left").select(spark_frame1["column1"].alias("column1_df1"), spark_frame2["column1"].alias("column1_df2"))
Hay dos funciones que pueden ayudarnos en este proceso merge y join.
df_joined = df1.merge(df2, left_on='lkey', right_on='rkey', how='left') df_joined = df1.join(df2, on='column_id', how='left')Luego seleccionamos dos columnas df_joined.loc[['column1_df1', 'column1_df2']]
En Power Query vamos a ir eligiendo una columna de antemano y luego añadiendo la segunda.
#"Origen" = #"Paso Anterior"[[column1_t1]] #"Paso Join" = Table.NestedJoin(#"Origen", {"column_t1_id"}, table2, {"column_t2_id"}, "Prefijo", JoinKind.LeftOuter) #"Expansion" = Table.ExpandTableColumn(#"Paso Join", "Prefijo", {"column1_t2"}, {"Prefijo_column1_t2"})
Group By
SELECT column1, count(*) FROM table1 GROUP BY column1
from pyspark.sql.functions import count spark_frame.groupBy("column1").agg(count("*").alias("count"))
df.groupby('column1')['column1'].count()
Table.Group(#"Paso Anterior", {"column1"}, {{"Alias de count", each Table.RowCount(_), type number}})
Filtrando un agrupado
SELECT store, sum(sales) FROM table1 GROUP BY store HAVING sum(sales) > 1000
from pyspark.sql.functions import sum as spark_sum spark_frame.groupBy("store").agg(spark_sum("sales").alias("total_sales")).filter("total_sales > 1000")
df_grouped = df.groupby('store')['sales'].sum() df_grouped.loc[df_grouped > 1000]
#”Grouping” = Table.Group(#"Paso Anterior", {"store"}, {{"Alias de sum", each List.Sum([sales]), type number}}) #"Final" = Table.SelectRows( #"Grouping" , each [Alias de sum] > 1000 )
Ordenar descendente por columna
SELECT * FROM table1 ORDER BY column1 DESC
spark_frame.orderBy("column1", ascending=False)
df.sort_values(by=['column1'], ascending=False)
Table.Sort(#"Paso Anterior",{{"column1", Order.Descending}})
Unir una tabla con otra de la misma característica
SELECT * FROM table1 UNION SELECT * FROM table2
spark_frame1.union(spark_frame2)
En Pandas tenemos dos opciones conocidas, la función append y concat.
df.append(df2) pd.concat([df1, df2])
Table.Combine({table1, table2})
Transformaciones
Las siguientes transformaciones son directamente entre PySpark, Pandas y Power Query puesto que no son tan comunes en un lenguaje de consulta como SQL. Puede que su resultado no sea idéntico pero si similar para el caso a resolver.
Analizar el contenido de una tabla
spark_frame.summary()
df.describe()
Table.Profile(#"Paso Anterior")
Chequear valores únicos de las columnas
spark_frame.groupBy("column1").count().show()
df.value_counts("columna1")
Table.Profile(#"Paso Anterior")[[Column],[DistinctCount]]
Generar Tabla de prueba con datos cargados a mano
spark_frame = spark.createDataFrame([(1, "Boris Yeltsin"), (2, "Mikhail Gorbachev")], inferSchema=True)
df = pd.DataFrame([[1,2],["Boris Yeltsin", "Mikhail Gorbachev"]], columns=["CustomerID", "Name"])
Table.FromRecords({[CustomerID = 1, Name = "Bob", Phone = "123-4567"]})
Quitar una columna
spark_frame.drop("column1")
df.drop(columns=['column1']) df.drop(['column1'], axis=1)
Table.RemoveColumns(#"Paso Anterior",{"column1"})
Aplicar transformaciones sobre una columna
spark_frame.withColumn("column1", col("column1") + 1)
df.apply(lambda x : x['column1'] + 1 , axis = 1)
Table.TransformColumns(#"Paso Anterior", {{"column1", each _ + 1, type number}})
Hemos terminado el largo camino de consultas y transformaciones que nos ayudarían a tener un mejor tiempo a puro código con PySpark, SQL, Pandas y Power Query para que conociendo uno sepamos usar el otro.
#spark#pyspark#python#pandas#sql#power query#powerquery#notebooks#ladataweb#data engineering#data wrangling#data cleansing
0 notes
Text
SQL Query Tutorial for Beginners: A Step-by-Step Guide
Are you new to the world of databases and eager to learn SQL? SQL (Structured Query Language) is a powerful tool used for managing and manipulating data within relational database management systems (RDBMS). Whether you're a budding programmer, a data analyst, or just someone interested in understanding databases, this SQL query tutorial is tailored just for you. Let's dive into the basics of SQL in this step-by-step guide.
1. Introduction to SQL:
SQL, pronounced as "ess-que-el" or "sequel," is a standard language for interacting with databases. It allows users to perform various operations such as retrieving data, updating records, deleting information, and much more. SQL is used in a wide range of applications, from simple data management tasks to complex database operations in large organizations.
2. Setting Up Your Environment:
Before diving into SQL queries, you need to set up your environment. You can choose from various RDBMS platforms such as MySQL, PostgreSQL, SQLite, or Microsoft SQL Server. Install the software according to your preference and operating system. Many of these platforms offer free versions for beginners to practice and learn.
3. Understanding Database Concepts:
To effectively use SQL, it's essential to understand some basic database concepts. A database is a structured collection of data organized for efficient retrieval. It consists of tables, which store data in rows and columns. Each table represents an entity, and each column represents a specific attribute of that entity. Understanding these concepts will help you design and query databases effectively.
4. Writing Your First SQL Query:
Now that you have your environment set up let's write your first SQL query. Open your chosen RDBMS platform and connect to a database. Start with a simple query to retrieve data from a table. For example:
sql
Copy code
SELECT * FROM table_name;
This query selects all columns from a table named "table_name." Replace "table_name" with the actual name of the table you want to query.
5. Filtering Data with WHERE Clause:
The WHERE clause is used to filter records based on a specified condition. It allows you to extract only the data that meets certain criteria. For instance:
sql
Copy code
SELECT * FROM table_name WHERE column_name = 'value';
This query retrieves all rows from "table_name" where the value in "column_name" matches 'value'. You can use various operators such as "=", "<>", "<", ">", "<=", ">=" to define conditions.
6. Sorting Data with ORDER BY Clause:
The ORDER BY clause is used to sort the result set in ascending or descending order based on one or more columns. For example:
sql
Copy code
SELECT * FROM table_name ORDER BY column_name ASC;
This query retrieves data from "table_name" and sorts it in ascending order based on "column_name." You can use "DESC" keyword to sort in descending order.
7. Aggregating Data with Functions:
SQL provides various aggregate functions to perform calculations on groups of rows and return a single result. Some common aggregate functions include COUNT(), SUM(), AVG(), MIN(), and MAX(). For instance:
sql
Copy code
SELECT COUNT(*) FROM table_name;
This query returns the total number of rows in "table_name." Experiment with other aggregate functions to perform calculations on your data.
8. Joining Tables:
In real-world scenarios, data is often distributed across multiple tables. SQL allows you to combine data from different tables using JOIN operations. There are different types of joins such as INNER JOIN, LEFT JOIN, RIGHT JOIN, and FULL JOIN. For example:
sql
Copy code
SELECT * FROM table1 INNER JOIN table2 ON table1.column_name = table2.column_name;
This query joins "table1" and "table2" based on matching values in "column_name" and retrieves all columns from both tables.
9. Practice and Further Learning:
The key to mastering SQL is practice. Try writing various SQL queries, experiment with different clauses, and explore advanced topics such as subqueries, indexes, and transactions. There are plenty of online resources, tutorials, and exercises available to enhance your SQL skills. Take advantage of them to become proficient in SQL.
0 notes
Text
SQL에서 "조인(Join)"은 두 개 이상의 테이블에서 열을 결합하여 데이터를 조회하는 과정을 말합니다. 조인을 사용하면 관련된 데이터가 여러 테이블에 분산되어 있을 때 이를 통합하여 조회할 수 있습니다. 다양한 종류의 조인이 있으며, 각각 특정한 유형의 결과를 반환합니다.
주요 조인 유형:
SQL에서 "조인(Join)"은 두 개 이상의 테이블에서 열을 결합하여 데이터를 조회하는 과정을 말합니다. 조인을 사용하면 관련된 데이터가 여러 테이블에 분산되어 있을 때 이를 통합하여 조회할 수 있습니다. 다양한 종류의 조인이 있으며, 각각 특정한 유형의 결과를 반환합니다.
주요 조인 유형:
내부 조인 (INNER JOIN):
두 테이블 간에 일치하는 행만 반환합니다. 즉, 양쪽 테이블 모두에서 일치하는 데이터가 있는 경우에만 해당 행들이 결과에 포함됩니다.
구문:sqlCopy codeSELECT columns FROM table1 INNER JOIN table2 ON table1.column_name = table2.column_name;
외부 조인 (OUTER JOIN):
외부 조인은 LEFT OUTER JOIN, RIGHT OUTER JOIN, FULL OUTER JOIN으로 나뉩니다.
LEFT (OUTER) JOIN: 왼쪽 테이블의 모든 행과 오른쪽 테이블에서 일치하는 행을 반환합니다. 오른쪽 테이블에 일치하는 행이 없는 경우 NULL 값으로 반환합니다.
RIGHT (OUTER) JOIN: 오른쪽 테이블의 모든 행과 왼쪽 테이블에서 일치하는 행을 반환합니다. 왼쪽 테이블에 일치하는 행이 없는 경우 NULL 값으로 반환합니다.
FULL (OUTER) JOIN: 왼쪽과 오른쪽 테이블 모두에서 일치하는 행을 반환합니다. 어느 한쪽에만 있는 행도 포함되며, 일치하는 행이 없는 쪽은 NULL 값으로 반환합니다.
크로스 조인 (CROSS JOIN):
두 테이블 간의 모든 가능한 조합을 반환합니다. 이는 두 테이블의 각 행이 다른 테이블의 모든 행과 결합됩니다.
구문:sqlCopy codeSELECT columns FROM table1 CROSS JOIN table2;
자체 조인 (SELF JOIN):
테이블이 자기 ���신과 조인되는 경우입니다. 이는 별칭(Alias)을 사용하여 동일한 테이블을 두 번 참조함으로써 수행됩니다.
표준 조인 (ANSI SQL-92 조인 구문):
표준 조인은 SQL-92 표준에서 도입된 조인 구문으로, 조인을 명확하게 표현할 수 있게 해줍니다. 표준 조인 구문은 조인의 종류를 명시적으로 기술하여 가독성과 관리의 용이성을 높입니다.
예를 들어, INNER JOIN은 다음과 같이 표현됩니다:sqlCopy codeSELECT columns FROM table1 INNER JOIN table2 ON table1.column_name = table2.column_name;
표준 조인 구문은 특히 조인의 유형��� 명확하게 하여 복잡한 쿼리에서 의도를 분명히 드러내는 데 유용합니다.
조인을 사용하면 데이터베이스 내 여러 테이블 간의 관계를 기반으로 복잡한 쿼리를 생성하고, 필요한 데이터를 효율적으로 추출할 수 있습니다.
표준 조인 (ANSI SQL-92 조인 구문):
표준 조인은 SQL-92 표준에서 도입된 조인 구문으로, 조인을 명확하게 표현할 수 있게 해줍니다. 표준 조인 구문은 조인의 종류를 명시적으로 기술하여 가독성과 관리의 용이성을 높입니다.
예를 들어, INNER JOIN은 다음과 같이 표현됩니다:sqlCopy codeSELECT columns FROM table1 INNER JOIN table2 ON table1.column_name = table2.column_name;
표준 조인 구문은 특히 조인의 유형을 명확하게 하여 복잡한 쿼리에서 의도를 분명히 드러내는 데 유용합니다.
조인을 사용하면 데이터베이스 내 여러 테이블 간의 관계를 기반으로 복잡한 쿼리를 생성하고, 필요한 데이터를 효율적으로 추출할 수 있습니다.
0 notes
Link
"STZ TV Room 2" - Extreme quality hi polygonal models for DAZ studio. Included in this Package: 1 TV Room30 Props1 Coffee pot2 Cola2 Drink1 Cup2 Floor lamp3 Pillows (dForce)1 Pillows1 Morphing Plaid2 Popcorn1 Projector1 Projector screen1 Rack1 Saucer3 Shelf1 Sofa1 Speaker2 Table1 Tray1 TV1 TV remote1 Wardrobe Coming soon: https://3d-stuff.net/ #daz3d #dazstudio #3drender #3dart #daz3dstudio #irayrender #3dartwork #blender #blenderrender #blenderart #noaiart #noaiwriting #noai https://3d-stuff.net/
0 notes
Text
Python RuntimeError: generator raised StopIteration
MySQL Connector error generator raised stopiteration error in Python Error: Error cause in execute with multi=true parameter result = cursor.execute(query, params, multi=True) for r in result: # causes the error OR cursors = cur.execute(operation="select * from table1;select * from table2;",multi=True) for cur in cursors: # <--- this line causes error with python 3.7 and deprecation warning…
View On WordPress
0 notes
Text
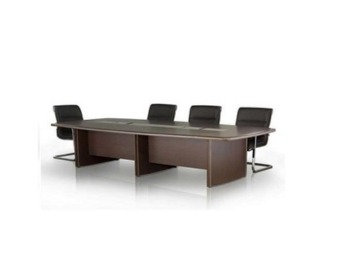
Sleek and Functional Conference Table for Collaborative Meetings: Office Mantra
The conference table is equipped with integrated connectivity options, allowing seamless integration of technology. Built-in power outlets, USB ports, and data ports facilitate effortless device connectivity, enabling participants to share information, present ideas, and collaborate effectively.
Designed for comfort and convenience, the table offers optional features such as ergonomic chairs and cable management systems. These additions promote ergonomic support and ensure a tidy workspace, allowing participants to stay focused and engaged throughout lengthy meetings.
Elevate your conference room experience with Office Mantra sleek and functional conference table. Create an environment that fosters collaboration, inspires creativity, and leaves a lasting impression on clients and team members alike. Transform your meetings into powerful moments of synergy and productivity with our exceptional conference table. Website: https://officemantra.in/conference-table1.php Email: [email protected] Contact: +91 8767458559
#conference table#office mantra#customized office furniture#office supplies#office sofas#office storage#modular office furniture
0 notes
Text
Open interest and volume interpretation
Open interest and volume interpretation:
Open interest (OI) is A number that tells you how many futures or options contracts are currently outstanding (open) in the market. Remember that there are always 2 sides to A trader- A buyer and A seller. Let us say seller sell one contract to the buyer. The buyer is said to be long on the contract and the seller is said to be short on the same contract the open interest in this case is said to be 1.
Open interest information tells us how many contracts are open and live in the market volume on the other hand tells us how many trades were executed on the given day. For every 1buy and 1sell , volume adds up to 1. Hence the volume data always increases on A intraday basis. However, oi not discrete like volumes, oi stacks up or reduces based on the entry and exit of traders.
Tables of trader’s perception-Table1
0 notes
Text
Basic cheat sheet to get you started with SQL
// Creating Tables CREATE TABLE table_name ( column1 datatype, column2 datatype, column3 datatype, … );
// Inserting Data INSERT INTO table_name (column1, column2, column3, …) VALUES (value1, value2, value3, …);
// Updating Data UPDATE table_name SET column1 = value1, column2 = value2, … WHERE condition;
// Deleting Data DELETE FROM table_name WHERE condition;
// Querying Data SELECT column1, column2, … FROM table_name WHERE condition GROUP BY column1 HAVING condition ORDER BY column1 ASC|DESC LIMIT count OFFSET offset;
// Joining Tables SELECT column_name(s) FROM table1 JOIN table2 ON table1.column_name = table2.column_name;
// Aggregating Data SELECT COUNT(column_name) FROM table_name WHERE condition; SELECT AVG(column_name) FROM table_name WHERE condition; SELECT SUM(column_name) FROM table_name WHERE condition; SELECT MIN(column_name) FROM table_name WHERE condition; SELECT MAX(column_name) FROM table_name WHERE condition;
// Creating Indexes CREATE INDEX index_name ON table_name (column_name);
// Creating Views CREATE VIEW view_name AS SELECT column_name(s) FROM table_name WHERE condition;
// Transactions START TRANSACTION; COMMIT; ROLLBACK;
// Granting Permissions GRANT permission ON object TO user;
0 notes