#redshift data warehouse
Explore tagged Tumblr posts
Text
Best Practices for a Smooth Data Warehouse Migration to Amazon Redshift
In the era of big data, many organizations find themselves outgrowing traditional on-premise data warehouses. Moving to a scalable, cloud-based solution like Amazon Redshift is an attractive solution for companies looking to improve performance, cut costs, and gain flexibility in their data operations. However, data warehouse migration to AWS, particularly to Amazon Redshift, can be complex, involving careful planning and precise execution to ensure a smooth transition. In this article, we’ll explore best practices for a seamless Redshift migration, covering essential steps from planning to optimization.
1. Establish Clear Objectives for Migration
Before diving into the technical process, it’s essential to define clear objectives for your data warehouse migration to AWS. Are you primarily looking to improve performance, reduce operational costs, or increase scalability? Understanding the ‘why’ behind your migration will help guide the entire process, from the tools you select to the migration approach.
For instance, if your main goal is to reduce costs, you’ll want to explore Amazon Redshift’s pay-as-you-go model or even Reserved Instances for predictable workloads. On the other hand, if performance is your focus, configuring the right nodes and optimizing queries will become a priority.
2. Assess and Prepare Your Data
Data assessment is a critical step in ensuring that your Redshift data warehouse can support your needs post-migration. Start by categorizing your data to determine what should be migrated and what can be archived or discarded. AWS provides tools like the AWS Schema Conversion Tool (SCT), which helps assess and convert your existing data schema for compatibility with Amazon Redshift.
For structured data that fits into Redshift’s SQL-based architecture, SCT can automatically convert schema from various sources, including Oracle and SQL Server, into a Redshift-compatible format. However, data with more complex structures might require custom ETL (Extract, Transform, Load) processes to maintain data integrity.
3. Choose the Right Migration Strategy
Amazon Redshift offers several migration strategies, each suited to different scenarios:
Lift and Shift: This approach involves migrating your data with minimal adjustments. It’s quick but may require optimization post-migration to achieve the best performance.
Re-architecting for Redshift: This strategy involves redesigning data models to leverage Redshift’s capabilities, such as columnar storage and distribution keys. Although more complex, it ensures optimal performance and scalability.
Hybrid Migration: In some cases, you may choose to keep certain workloads on-premises while migrating only specific data to Redshift. This strategy can help reduce risk and maintain critical workloads while testing Redshift’s performance.
Each strategy has its pros and cons, and selecting the best one depends on your unique business needs and resources. For a fast-tracked, low-cost migration, lift-and-shift works well, while those seeking high-performance gains should consider re-architecting.
4. Leverage Amazon’s Native Tools
Amazon Redshift provides a suite of tools that streamline and enhance the migration process:
AWS Database Migration Service (DMS): This service facilitates seamless data migration by enabling continuous data replication with minimal downtime. It’s particularly helpful for organizations that need to keep their data warehouse running during migration.
AWS Glue: Glue is a serverless data integration service that can help you prepare, transform, and load data into Redshift. It’s particularly valuable when dealing with unstructured or semi-structured data that needs to be transformed before migrating.
Using these tools allows for a smoother, more efficient migration while reducing the risk of data inconsistencies and downtime.
5. Optimize for Performance on Amazon Redshift
Once the migration is complete, it’s essential to take advantage of Redshift’s optimization features:
Use Sort and Distribution Keys: Redshift relies on distribution keys to define how data is stored across nodes. Selecting the right key can significantly improve query performance. Sort keys, on the other hand, help speed up query execution by reducing disk I/O.
Analyze and Tune Queries: Post-migration, analyze your queries to identify potential bottlenecks. Redshift’s query optimizer can help tune performance based on your specific workloads, reducing processing time for complex queries.
Compression and Encoding: Amazon Redshift offers automatic compression, reducing the size of your data and enhancing performance. Using columnar storage, Redshift efficiently compresses data, so be sure to implement optimal compression settings to save storage costs and boost query speed.
6. Plan for Security and Compliance
Data security and regulatory compliance are top priorities when migrating sensitive data to the cloud. Amazon Redshift includes various security features such as:
Data Encryption: Use encryption options, including encryption at rest using AWS Key Management Service (KMS) and encryption in transit with SSL, to protect your data during migration and beyond.
Access Control: Amazon Redshift supports AWS Identity and Access Management (IAM) roles, allowing you to define user permissions precisely, ensuring that only authorized personnel can access sensitive data.
Audit Logging: Redshift’s logging features provide transparency and traceability, allowing you to monitor all actions taken on your data warehouse. This helps meet compliance requirements and secures sensitive information.
7. Monitor and Adjust Post-Migration
Once the migration is complete, establish a monitoring routine to track the performance and health of your Redshift data warehouse. Amazon Redshift offers built-in monitoring features through Amazon CloudWatch, which can alert you to anomalies and allow for quick adjustments.
Additionally, be prepared to make adjustments as you observe user patterns and workloads. Regularly review your queries, data loads, and performance metrics, fine-tuning configurations as needed to maintain optimal performance.
Final Thoughts: Migrating to Amazon Redshift with Confidence
Migrating your data warehouse to Amazon Redshift can bring substantial advantages, but it requires careful planning, robust tools, and continuous optimization to unlock its full potential. By defining clear objectives, preparing your data, selecting the right migration strategy, and optimizing for performance, you can ensure a seamless transition to Redshift. Leveraging Amazon’s suite of tools and Redshift’s powerful features will empower your team to harness the full potential of a cloud-based data warehouse, boosting scalability, performance, and cost-efficiency.
Whether your goal is improved analytics or lower operating costs, following these best practices will help you make the most of your Amazon Redshift data warehouse, enabling your organization to thrive in a data-driven world.
#data warehouse migration to aws#redshift data warehouse#amazon redshift data warehouse#redshift migration#data warehouse to aws migration#data warehouse#aws migration
0 notes
Text
Find out which cloud data warehouse is superior—Azure Synapse Analytics or AWS Redshift. Compare features, cost efficiency, and data integration capabilities.
0 notes
Text
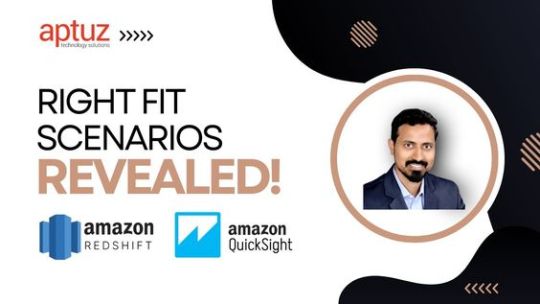
Learn how Amazon Redshift handles massive datasets and complex queries, and when it's best suited for tasks like Mortgage Portfolio Analysis or Real-Time Fraud Detection. Explore AWS QuickSight's integration with AWS data sources and its strengths in Business Intelligence and Data Exploration. Get actionable insights to make informed decisions for your projects and use cases.
#fintech#technology#finance#data analytics#redshift#quicksight#aws#amazon web services#technology videos#learning videos#data management#data warehouse#mortgage services#mortgage finance#data transformation
0 notes
Text
Discover practical strategies and expert tips on optimizing your data warehouse to scale efficiently without spending more money. Learn how to save costs while expanding your data infrastructure, ensuring maximum performance.
0 notes
Text
"Unlocking Business Intelligence with Data Warehouse Solutions"
Data Warehouse Solution: Boosting Business Intelligence
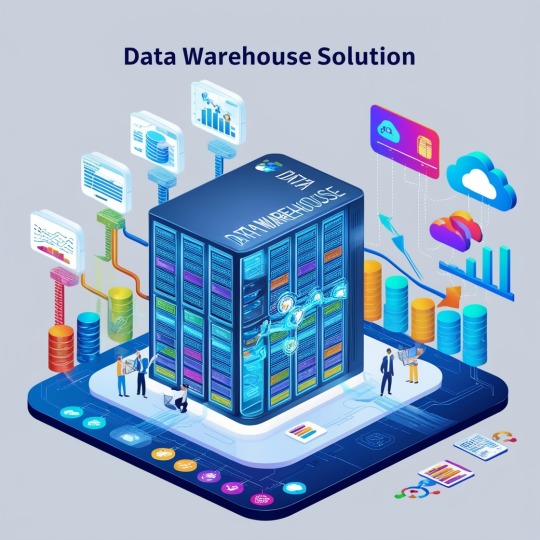
A data warehouse (DW) is an organized space that enables companies to organize and assess large volumes of information through multiple locations in a consistent way. This is intended to assist with tracking, company analytics, and choices. The data warehouse's primary purpose was to render it possible to efficiently analyze past and present information, offering important conclusions for management as a business strategy.
A data warehouse normally employs procedures (Take, convert, load) for combining information coming from several sources, including business tables, operations, and outside data flows.This allows for an advanced level of scrutiny by ensuring data reliability and precision. The information's structure enables complicated searches, which are often achieved using the aid of SQL-based tools, BI (Business Intelligence) software, or information display systems.
Regarding activities requiring extensive research, data storage centers were ideal since they could provide executives with rapid and precise conclusions. Common application cases include accounting, provider direction, customer statistics, and projections of sales. Systems provide connectivity, speed, and easy control of networks, but as cloud computing gained popularity, data warehouses like Amazon's Redshift, Google's Large SEARCH, and Snowflake have remained famous.
In conclusion, managing information systems is essential for companies that want to make the most out of their information. Gathering information collected in one center allows firms to better understand how they operate and introduce decisions that promote inventiveness and originality.
2 notes
·
View notes
Text
I wish I lived in a beautiful world where googling “redshift” returned the wikipedia article on the astronomical phenomenon as the first result instead of amazon’s data warehouse and “ads” redirected me to the astrophysics data system instead of suggesting a linkedin page with careers in advertising
#gonna be real for a moment. the amazon redshift thing is pissing me OFF.#1. it’s a stupid name and 2. it’s a pain in my ass and 3. WHY is it the first result.#astroposting
3 notes
·
View notes
Text
Navigating the Cloud Landscape: Unleashing Amazon Web Services (AWS) Potential
In the ever-evolving tech landscape, businesses are in a constant quest for innovation, scalability, and operational optimization. Enter Amazon Web Services (AWS), a robust cloud computing juggernaut offering a versatile suite of services tailored to diverse business requirements. This blog explores the myriad applications of AWS across various sectors, providing a transformative journey through the cloud.
Harnessing Computational Agility with Amazon EC2
Central to the AWS ecosystem is Amazon EC2 (Elastic Compute Cloud), a pivotal player reshaping the cloud computing paradigm. Offering scalable virtual servers, EC2 empowers users to seamlessly run applications and manage computing resources. This adaptability enables businesses to dynamically adjust computational capacity, ensuring optimal performance and cost-effectiveness.
Redefining Storage Solutions
AWS addresses the critical need for scalable and secure storage through services such as Amazon S3 (Simple Storage Service) and Amazon EBS (Elastic Block Store). S3 acts as a dependable object storage solution for data backup, archiving, and content distribution. Meanwhile, EBS provides persistent block-level storage designed for EC2 instances, guaranteeing data integrity and accessibility.
Streamlined Database Management: Amazon RDS and DynamoDB
Database management undergoes a transformation with Amazon RDS, simplifying the setup, operation, and scaling of relational databases. Be it MySQL, PostgreSQL, or SQL Server, RDS provides a frictionless environment for managing diverse database workloads. For enthusiasts of NoSQL, Amazon DynamoDB steps in as a swift and flexible solution for document and key-value data storage.
Networking Mastery: Amazon VPC and Route 53
AWS empowers users to construct a virtual sanctuary for their resources through Amazon VPC (Virtual Private Cloud). This virtual network facilitates the launch of AWS resources within a user-defined space, enhancing security and control. Simultaneously, Amazon Route 53, a scalable DNS web service, ensures seamless routing of end-user requests to globally distributed endpoints.
Global Content Delivery Excellence with Amazon CloudFront
Amazon CloudFront emerges as a dynamic content delivery network (CDN) service, securely delivering data, videos, applications, and APIs on a global scale. This ensures low latency and high transfer speeds, elevating user experiences across diverse geographical locations.
AI and ML Prowess Unleashed
AWS propels businesses into the future with advanced machine learning and artificial intelligence services. Amazon SageMaker, a fully managed service, enables developers to rapidly build, train, and deploy machine learning models. Additionally, Amazon Rekognition provides sophisticated image and video analysis, supporting applications in facial recognition, object detection, and content moderation.
Big Data Mastery: Amazon Redshift and Athena
For organizations grappling with massive datasets, AWS offers Amazon Redshift, a fully managed data warehouse service. It facilitates the execution of complex queries on large datasets, empowering informed decision-making. Simultaneously, Amazon Athena allows users to analyze data in Amazon S3 using standard SQL queries, unlocking invaluable insights.
In conclusion, Amazon Web Services (AWS) stands as an all-encompassing cloud computing platform, empowering businesses to innovate, scale, and optimize operations. From adaptable compute power and secure storage solutions to cutting-edge AI and ML capabilities, AWS serves as a robust foundation for organizations navigating the digital frontier. Embrace the limitless potential of cloud computing with AWS – where innovation knows no bounds.
3 notes
·
View notes
Text
Data Engineering Concepts, Tools, and Projects
All the associations in the world have large amounts of data. If not worked upon and anatomized, this data does not amount to anything. Data masterminds are the ones. who make this data pure for consideration. Data Engineering can nominate the process of developing, operating, and maintaining software systems that collect, dissect, and store the association’s data. In modern data analytics, data masterminds produce data channels, which are the structure armature.
How to become a data engineer:
While there is no specific degree requirement for data engineering, a bachelor's or master's degree in computer science, software engineering, information systems, or a related field can provide a solid foundation. Courses in databases, programming, data structures, algorithms, and statistics are particularly beneficial. Data engineers should have strong programming skills. Focus on languages commonly used in data engineering, such as Python, SQL, and Scala. Learn the basics of data manipulation, scripting, and querying databases.
Familiarize yourself with various database systems like MySQL, PostgreSQL, and NoSQL databases such as MongoDB or Apache Cassandra.Knowledge of data warehousing concepts, including schema design, indexing, and optimization techniques.
Data engineering tools recommendations:
Data Engineering makes sure to use a variety of languages and tools to negotiate its objects. These tools allow data masterminds to apply tasks like creating channels and algorithms in a much easier as well as effective manner.
1. Amazon Redshift: A widely used cloud data warehouse built by Amazon, Redshift is the go-to choice for many teams and businesses. It is a comprehensive tool that enables the setup and scaling of data warehouses, making it incredibly easy to use.
One of the most popular tools used for businesses purpose is Amazon Redshift, which provides a powerful platform for managing large amounts of data. It allows users to quickly analyze complex datasets, build models that can be used for predictive analytics, and create visualizations that make it easier to interpret results. With its scalability and flexibility, Amazon Redshift has become one of the go-to solutions when it comes to data engineering tasks.
2. Big Query: Just like Redshift, Big Query is a cloud data warehouse fully managed by Google. It's especially favored by companies that have experience with the Google Cloud Platform. BigQuery not only can scale but also has robust machine learning features that make data analysis much easier. 3. Tableau: A powerful BI tool, Tableau is the second most popular one from our survey. It helps extract and gather data stored in multiple locations and comes with an intuitive drag-and-drop interface. Tableau makes data across departments readily available for data engineers and managers to create useful dashboards. 4. Looker: An essential BI software, Looker helps visualize data more effectively. Unlike traditional BI tools, Looker has developed a LookML layer, which is a language for explaining data, aggregates, calculations, and relationships in a SQL database. A spectacle is a newly-released tool that assists in deploying the LookML layer, ensuring non-technical personnel have a much simpler time when utilizing company data.
5. Apache Spark: An open-source unified analytics engine, Apache Spark is excellent for processing large data sets. It also offers great distribution and runs easily alongside other distributed computing programs, making it essential for data mining and machine learning. 6. Airflow: With Airflow, programming, and scheduling can be done quickly and accurately, and users can keep an eye on it through the built-in UI. It is the most used workflow solution, as 25% of data teams reported using it. 7. Apache Hive: Another data warehouse project on Apache Hadoop, Hive simplifies data queries and analysis with its SQL-like interface. This language enables MapReduce tasks to be executed on Hadoop and is mainly used for data summarization, analysis, and query. 8. Segment: An efficient and comprehensive tool, Segment assists in collecting and using data from digital properties. It transforms, sends, and archives customer data, and also makes the entire process much more manageable. 9. Snowflake: This cloud data warehouse has become very popular lately due to its capabilities in storing and computing data. Snowflake’s unique shared data architecture allows for a wide range of applications, making it an ideal choice for large-scale data storage, data engineering, and data science. 10. DBT: A command-line tool that uses SQL to transform data, DBT is the perfect choice for data engineers and analysts. DBT streamlines the entire transformation process and is highly praised by many data engineers.
Data Engineering Projects:
Data engineering is an important process for businesses to understand and utilize to gain insights from their data. It involves designing, constructing, maintaining, and troubleshooting databases to ensure they are running optimally. There are many tools available for data engineers to use in their work such as My SQL, SQL server, oracle RDBMS, Open Refine, TRIFACTA, Data Ladder, Keras, Watson, TensorFlow, etc. Each tool has its strengths and weaknesses so it’s important to research each one thoroughly before making recommendations about which ones should be used for specific tasks or projects.
Smart IoT Infrastructure:
As the IoT continues to develop, the measure of data consumed with high haste is growing at an intimidating rate. It creates challenges for companies regarding storehouses, analysis, and visualization.
Data Ingestion:
Data ingestion is moving data from one or further sources to a target point for further preparation and analysis. This target point is generally a data storehouse, a unique database designed for effective reporting.
Data Quality and Testing:
Understand the importance of data quality and testing in data engineering projects. Learn about techniques and tools to ensure data accuracy and consistency.
Streaming Data:
Familiarize yourself with real-time data processing and streaming frameworks like Apache Kafka and Apache Flink. Develop your problem-solving skills through practical exercises and challenges.
Conclusion:
Data engineers are using these tools for building data systems. My SQL, SQL server and Oracle RDBMS involve collecting, storing, managing, transforming, and analyzing large amounts of data to gain insights. Data engineers are responsible for designing efficient solutions that can handle high volumes of data while ensuring accuracy and reliability. They use a variety of technologies including databases, programming languages, machine learning algorithms, and more to create powerful applications that help businesses make better decisions based on their collected data.
2 notes
·
View notes
Text
Best Informatica Cloud Training in India | Informatica IICS
Cloud Data Integration (CDI) in Informatica IICS
Introduction
Cloud Data Integration (CDI) in Informatica Intelligent Cloud Services (IICS) is a powerful solution that helps organizations efficiently manage, process, and transform data across hybrid and multi-cloud environments. CDI plays a crucial role in modern ETL (Extract, Transform, Load) and ELT (Extract, Load, Transform) operations, enabling businesses to achieve high-performance data processing with minimal complexity. In today’s data-driven world, businesses need seamless integration between various data sources, applications, and cloud platforms. Informatica Training Online
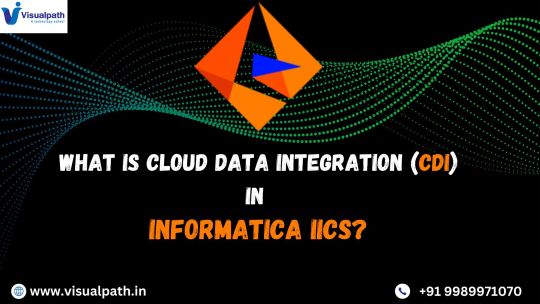
What is Cloud Data Integration (CDI)?
Cloud Data Integration (CDI) is a Software-as-a-Service (SaaS) solution within Informatica IICS that allows users to integrate, transform, and move data across cloud and on-premises systems. CDI provides a low-code/no-code interface, making it accessible for both technical and non-technical users to build complex data pipelines without extensive programming knowledge.
Key Features of CDI in Informatica IICS
Cloud-Native Architecture
CDI is designed to run natively on the cloud, offering scalability, flexibility, and reliability across various cloud platforms like AWS, Azure, and Google Cloud.
Prebuilt Connectors
It provides out-of-the-box connectors for SaaS applications, databases, data warehouses, and enterprise applications such as Salesforce, SAP, Snowflake, and Microsoft Azure.
ETL and ELT Capabilities
Supports ETL for structured data transformation before loading and ELT for transforming data after loading into cloud storage or data warehouses.
Data Quality and Governance
Ensures high data accuracy and compliance with built-in data cleansing, validation, and profiling features. Informatica IICS Training
High Performance and Scalability
CDI optimizes data processing with parallel execution, pushdown optimization, and serverless computing to enhance performance.
AI-Powered Automation
Integrated Informatica CLAIRE, an AI-driven metadata intelligence engine, automates data mapping, lineage tracking, and error detection.
Benefits of Using CDI in Informatica IICS
1. Faster Time to Insights
CDI enables businesses to integrate and analyze data quickly, helping data analysts and business teams make informed decisions in real-time.
2. Cost-Effective Data Integration
With its serverless architecture, businesses can eliminate on-premise infrastructure costs, reducing Total Cost of Ownership (TCO) while ensuring high availability and security.
3. Seamless Hybrid and Multi-Cloud Integration
CDI supports hybrid and multi-cloud environments, ensuring smooth data flow between on-premises systems and various cloud providers without performance issues. Informatica Cloud Training
4. No-Code/Low-Code Development
Organizations can build and deploy data pipelines using a drag-and-drop interface, reducing dependency on specialized developers and improving productivity.
5. Enhanced Security and Compliance
Informatica ensures data encryption, role-based access control (RBAC), and compliance with GDPR, CCPA, and HIPAA standards, ensuring data integrity and security.
Use Cases of CDI in Informatica IICS
1. Cloud Data Warehousing
Companies migrating to cloud-based data warehouses like Snowflake, Amazon Redshift, or Google BigQuery can use CDI for seamless data movement and transformation.
2. Real-Time Data Integration
CDI supports real-time data streaming, enabling enterprises to process data from IoT devices, social media, and APIs in real-time.
3. SaaS Application Integration
Businesses using applications like Salesforce, Workday, and SAP can integrate and synchronize data across platforms to maintain data consistency. IICS Online Training
4. Big Data and AI/ML Workloads
CDI helps enterprises prepare clean and structured datasets for AI/ML model training by automating data ingestion and transformation.
Conclusion
Cloud Data Integration (CDI) in Informatica IICS is a game-changer for enterprises looking to modernize their data integration strategies. CDI empowers businesses to achieve seamless data connectivity across multiple platforms with its cloud-native architecture, advanced automation, AI-powered data transformation, and high scalability. Whether you’re migrating data to the cloud, integrating SaaS applications, or building real-time analytics pipelines, Informatica CDI offers a robust and efficient solution to streamline your data workflows.
For organizations seeking to accelerate digital transformation, adopting Informatics’ Cloud Data Integration (CDI) solution is a strategic step toward achieving agility, cost efficiency, and data-driven innovation.
For More Information about Informatica Cloud Online Training
Contact Call/WhatsApp: +91 7032290546
Visit: https://www.visualpath.in/informatica-cloud-training-in-hyderabad.html
#Informatica Training in Hyderabad#IICS Training in Hyderabad#IICS Online Training#Informatica Cloud Training#Informatica Cloud Online Training#Informatica IICS Training#Informatica Training Online#Informatica Cloud Training in Chennai#Informatica Cloud Training In Bangalore#Best Informatica Cloud Training in India#Informatica Cloud Training Institute#Informatica Cloud Training in Ameerpet
0 notes
Text
A Deep Dive into Amazon Redshift: Your Guide to Cloud Data Warehousing
In the era of big data, organizations are increasingly turning to cloud solutions for efficient data management and analysis. Amazon Redshift, a prominent service from Amazon Web Services (AWS), has become a go-to choice for businesses looking to optimize their data warehousing capabilities. In this blog, we will explore what Amazon Redshift is, its architecture, features, and how it can transform your approach to data analytics.
If you want to advance your career at the AWS Course in Pune, you need to take a systematic approach and join up for a course that best suits your interests and will greatly expand your learning path.
What is Amazon Redshift?
Amazon Redshift is a fully managed, cloud-based data warehouse service designed to handle large-scale data processing and analytics. It enables businesses to analyze vast amounts of structured and semi-structured data quickly and efficiently. With its architecture tailored for high performance, Redshift allows users to run complex queries and generate insights in real time.
The Architecture of Amazon Redshift
Understanding the architecture of Redshift is crucial to appreciating its capabilities. Here are the key components:
1. Columnar Storage
Unlike traditional row-based databases, Redshift uses a columnar storage model. This approach allows for more efficient data retrieval, as only the necessary columns are accessed during queries, significantly speeding up performance.
2. Massively Parallel Processing (MPP)
Redshift employs a massively parallel processing architecture, distributing workloads across multiple nodes. This means that queries can be processed simultaneously, enhancing speed and efficiency.
3. Data Compression
Redshift automatically compresses data to save storage space and improve query performance. By reducing the amount of data that needs to be scanned, it accelerates query execution.
4. Snapshots and Backups
Redshift provides automated snapshots of your data warehouse. This feature ensures data durability and allows for easy restoration in case of failure, enhancing data security.
To master the intricacies of AWS and unlock its full potential, individuals can benefit from enrolling in the AWS Online Training.
Key Features of Amazon Redshift
1. Scalability
Redshift is designed to grow with your data. You can start with a small data warehouse and scale up to petabytes as your data needs expand. This scalability is vital for businesses experiencing rapid growth.
2. Integration with AWS Ecosystem
As part of AWS, Redshift integrates seamlessly with other services like Amazon S3, AWS Glue, and Amazon QuickSight. This integration simplifies data ingestion, transformation, and visualization, creating a cohesive data ecosystem.
3. Advanced Security Features
Redshift offers robust security measures, including data encryption, network isolation with Amazon VPC, and user access controls through AWS IAM. This ensures that your data remains secure and compliant with industry standards.
4. Cost-Effectiveness
With a pay-as-you-go pricing model, Redshift allows businesses to optimize costs based on their usage. Options for reserved instances further enhance cost savings, making it an attractive choice for organizations of all sizes.
Redshift supports a wide range of analytical queries, empowering businesses to explore data trends, customer behavior, and operational efficiencies comprehensively.
Conclusion
Amazon Redshift stands out as a powerful solution for cloud data warehousing and analytics. Its scalable architecture, high performance, and seamless integration with other AWS services make it an ideal choice for businesses looking to leverage their data effectively.
Whether you're a small startup or a large enterprise, Redshift can provide the tools you need to make data-driven decisions and stay competitive in today's data-centric landscape.
0 notes
Text
Seamless integration of PostgreSQL to Amazon Redshift using Zero ETL methodology. Explore the benefits, potential limitations, and essential considerations for optimising data transfer and processing efficiency. Dive into real-time insights and learn how to navigate challenges while maximising the advantages of this innovative data integration approach.
#fintech#technology#finance#data analytics#redshift#PostgreSQL#database#data engineering#data analysis#data warehouse#learning#tech videos
0 notes
Text
"Hands-On Guide to Implementing NoSQL Data Warehousing with Amazon Redshift"
Introduction In this hands-on guide, we will walk you through the process of implementing a NoSQL data warehousing solution using Amazon Redshift. Amazon Redshift is a fully managed data warehouse service that allows you to analyze data across a wide range of data types and sizes. This guide is designed for developers and data analysts who want to learn how to implement a NoSQL data warehousing…
0 notes
Text
Big Data and Data Engineering
Big Data and Data Engineering are essential concepts in modern data science, analytics, and machine learning.
They focus on the processes and technologies used to manage and process large volumes of data.
Here’s an overview:
What is Big Data? Big Data refers to extremely large datasets that cannot be processed or analyzed using traditional data processing tools or methods.
It typically has the following characteristics:
Volume:
Huge amounts of data (petabytes or more).
Variety:
Data comes in different formats (structured, semi-structured, unstructured). Velocity: The speed at which data is generated and processed.
Veracity: The quality and accuracy of data.
Value: Extracting meaningful insights from data.
Big Data is often associated with technologies and tools that allow organizations to store, process, and analyze data at scale.
2. Data Engineering:
Overview Data Engineering is the process of designing, building, and managing the systems and infrastructure required to collect, store, process, and analyze data.
The goal is to make data easily accessible for analytics and decision-making.
Key areas of Data Engineering:
Data Collection:
Gathering data from various sources (e.g., IoT devices, logs, APIs). Data Storage: Storing data in data lakes, databases, or distributed storage systems. Data Processing: Cleaning, transforming, and aggregating raw data into usable formats.
Data Integration:
Combining data from multiple sources to create a unified dataset for analysis.
3. Big Data Technologies and Tools
The following tools and technologies are commonly used in Big Data and Data Engineering to manage and process large datasets:
Data Storage:
Data Lakes: Large storage systems that can handle structured, semi-structured, and unstructured data. Examples include Amazon S3, Azure Data Lake, and Google Cloud Storage.
Distributed File Systems:
Systems that allow data to be stored across multiple machines. Examples include Hadoop HDFS and Apache Cassandra.
Databases:
Relational databases (e.g., MySQL, PostgreSQL) and NoSQL databases (e.g., MongoDB, Cassandra, HBase).
Data Processing:
Batch Processing: Handling large volumes of data in scheduled, discrete chunks.
Common tools:
Apache Hadoop (MapReduce framework). Apache Spark (offers both batch and stream processing).
Stream Processing:
Handling real-time data flows. Common tools: Apache Kafka (message broker). Apache Flink (streaming data processing). Apache Storm (real-time computation).
ETL (Extract, Transform, Load):
Tools like Apache Nifi, Airflow, and AWS Glue are used to automate data extraction, transformation, and loading processes.
Data Orchestration & Workflow Management:
Apache Airflow is a platform for programmatically authoring, scheduling, and monitoring workflows. Kubernetes and Docker are used to deploy and scale applications in data pipelines.
Data Warehousing & Analytics:
Amazon Redshift, Google BigQuery, Snowflake, and Azure Synapse Analytics are popular cloud data warehouses for large-scale data analytics.
Apache Hive is a data warehouse built on top of Hadoop to provide SQL-like querying capabilities.
Data Quality and Governance:
Tools like Great Expectations, Deequ, and AWS Glue DataBrew help ensure data quality by validating, cleaning, and transforming data before it’s analyzed.
4. Data Engineering Lifecycle
The typical lifecycle in Data Engineering involves the following stages: Data Ingestion: Collecting and importing data from various sources into a central storage system.
This could include real-time ingestion using tools like Apache Kafka or batch-based ingestion using Apache Sqoop.
Data Transformation (ETL/ELT): After ingestion, raw data is cleaned and transformed.
This may include:
Data normalization and standardization. Removing duplicates and handling missing data.
Aggregating or merging datasets. Using tools like Apache Spark, AWS Glue, and Talend.
Data Storage:
After transformation, the data is stored in a format that can be easily queried.
This could be in a data warehouse (e.g., Snowflake, Google BigQuery) or a data lake (e.g., Amazon S3).
Data Analytics & Visualization:
After the data is stored, it is ready for analysis. Data scientists and analysts use tools like SQL, Jupyter Notebooks, Tableau, and Power BI to create insights and visualize the data.
Data Deployment & Serving:
In some use cases, data is deployed to serve real-time queries using tools like Apache Druid or Elasticsearch.
5. Challenges in Big Data and Data Engineering
Data Security & Privacy:
Ensuring that data is secure, encrypted, and complies with privacy regulations (e.g., GDPR, CCPA).
Scalability:
As data grows, the infrastructure needs to scale to handle it efficiently.
Data Quality:
Ensuring that the data collected is accurate, complete, and relevant. Data
Integration:
Combining data from multiple systems with differing formats and structures can be complex.
Real-Time Processing:
Managing data that flows continuously and needs to be processed in real-time.
6. Best Practices in Data Engineering Modular Pipelines:
Design data pipelines as modular components that can be reused and updated independently.
Data Versioning: Keep track of versions of datasets and data models to maintain consistency.
Data Lineage: Track how data moves and is transformed across systems.
Automation: Automate repetitive tasks like data collection, transformation, and processing using tools like Apache Airflow or Luigi.
Monitoring: Set up monitoring and alerting to track the health of data pipelines and ensure data accuracy and timeliness.
7. Cloud and Managed Services for Big Data
Many companies are now leveraging cloud-based services to handle Big Data:
AWS:
Offers tools like AWS Glue (ETL), Redshift (data warehousing), S3 (storage), and Kinesis (real-time streaming).
Azure:
Provides Azure Data Lake, Azure Synapse Analytics, and Azure Databricks for Big Data processing.
Google Cloud:
Offers BigQuery, Cloud Storage, and Dataflow for Big Data workloads.
Data Engineering plays a critical role in enabling efficient data processing, analysis, and decision-making in a data-driven world.
0 notes
Text
AWS Data Engineering online training | AWS Data Engineer
AWS Data Engineering: An Overview and Its Importance
Introduction
AWS Data Engineering plays a significant role in handling and transforming raw data into valuable insights using Amazon Web Services (AWS) tools and technologies. This article explores AWS Data Engineering, its components, and why it is essential for modern enterprises. In today's data-driven world, organizations generate vast amounts of data daily. Effectively managing, processing, and analyzing this data is crucial for decision-making and business growth. AWS Data Engineering Training
What is AWS Data Engineering?
AWS Data Engineering refers to the process of designing, building, and managing scalable and secure data pipelines using AWS cloud services. It involves the extraction, transformation, and loading (ETL) of data from various sources into a centralized storage or data warehouse for analysis and reporting. Data engineers leverage AWS tools such as AWS Glue, Amazon Redshift, AWS Lambda, Amazon S3, AWS Data Pipeline, and Amazon EMR to streamline data processing and management.
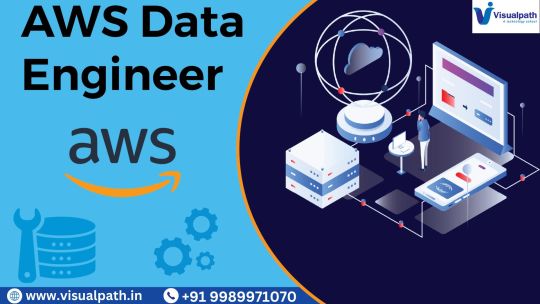
Key Components of AWS Data Engineering
AWS offers a comprehensive set of tools and services to support data engineering. Here are some of the essential components:
Amazon S3 (Simple Storage Service): A scalable object storage service used to store raw and processed data securely.
AWS Glue: A fully managed ETL (Extract, Transform, Load) service that automates data preparation and transformation.
Amazon Redshift: A cloud data warehouse that enables efficient querying and analysis of large datasets. AWS Data Engineering Training
AWS Lambda: A serverless computing service used to run functions in response to events, often used for real-time data processing.
Amazon EMR (Elastic MapReduce): A service for processing big data using frameworks like Apache Spark and Hadoop.
AWS Data Pipeline: A managed service for automating data movement and transformation between AWS services and on-premise data sources.
AWS Kinesis: A real-time data streaming service that allows businesses to collect, process, and analyze data in real time.
Why is AWS Data Engineering Important?
AWS Data Engineering is essential for businesses due to several key reasons: AWS Data Engineering Training Institute
Scalability and Performance AWS provides scalable solutions that allow organizations to handle large volumes of data efficiently. Services like Amazon Redshift and EMR ensure high-performance data processing and analysis.
Cost-Effectiveness AWS offers pay-as-you-go pricing models, eliminating the need for large upfront investments in infrastructure. Businesses can optimize costs by only using the resources they need.
Security and Compliance AWS provides robust security features, including encryption, identity and access management (IAM), and compliance with industry standards like GDPR and HIPAA. AWS Data Engineering online training
Seamless Integration AWS services integrate seamlessly with third-party tools and on-premise data sources, making it easier to build and manage data pipelines.
Real-Time Data Processing AWS supports real-time data processing with services like AWS Kinesis and AWS Lambda, enabling businesses to react to events and insights instantly.
Data-Driven Decision Making With powerful data engineering tools, organizations can transform raw data into actionable insights, leading to improved business strategies and customer experiences.
Conclusion
AWS Data Engineering is a critical discipline for modern enterprises looking to leverage data for growth and innovation. By utilizing AWS's vast array of services, organizations can efficiently manage data pipelines, enhance security, reduce costs, and improve decision-making. As the demand for data engineering continues to rise, businesses investing in AWS Data Engineering gain a competitive edge in the ever-evolving digital landscape.
Visualpath is the Best Software Online Training Institute in Hyderabad. Avail complete AWS Data Engineering Training worldwide. You will get the best course at an affordable cost
Visit: https://www.visualpath.in/online-aws-data-engineering-course.html
Visit Blog: https://visualpathblogs.com/category/aws-data-engineering-with-data-analytics/
WhatsApp: https://www.whatsapp.com/catalog/919989971070/
#AWS Data Engineering Course#AWS Data Engineering Training#AWS Data Engineer Certification#Data Engineering course in Hyderabad#AWS Data Engineering online training#AWS Data Engineering Training Institute#AWS Data Engineering Training in Hyderabad#AWS Data Engineer online course
0 notes
Text
Visualpath: Advance your career with Matillion Online Training.Get an Matillion Training from our industry experts and gain hands-on experience with our interactive programs.We provide to individuals globally in the USA, UK, Canada,Australia
(Course Covered): Snowflake,ETL, Informatica, Data Warehouse, DBT(Data Build Tool), SQL, Talend,POWER BI, Data bricks,Amazon Redshift,Oracle etc. Enroll Today!! Contact us at +91-9989971070
WhatsApp:https://www.whatsapp.com/catalog/919989971070/
Visit: https://www.visualpath.in/matillion-online-training.html
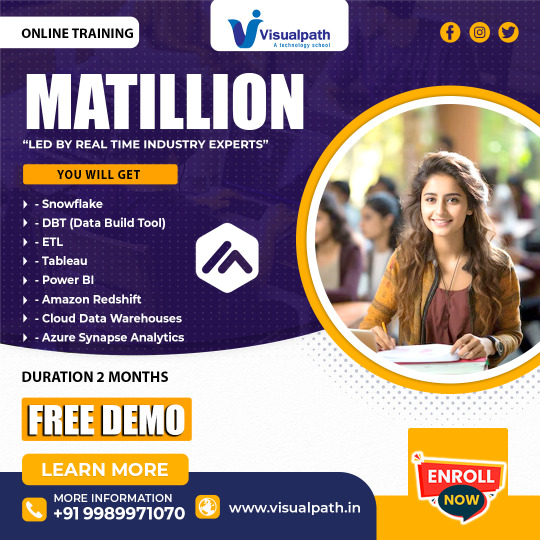
#Matillion Online Training#Matillion Training#Matillion Etl Training#Matillion Training Online#Matillion Training In Hyderabad#Matillion For Snowflake Training#Matillion Online Certification Course#Matillion Online Course In Hyderabad
0 notes