#python numpy
Explore tagged Tumblr posts
Text
Day 1 - 13th April, 2023
A wise someone (@compooter-blob) told me that NumPy, Pandas and Matplotlib are the butter, bread and milk of python development.
I started learning a bit of NumPy today, and I only have one word to describe it - cute.
#dailyfoxposts#studyblr#30dop#30 days of productivity#tumblr challenges#studyblr challenges#study#studyspo#codeblr#coding#python#numpy#python numpy
12 notes
·
View notes
Text
python baby PLEEEEAAAAASEEEEE add generics. it would make my typehinting life SOOOOOO much easier
#programming#python#still haven't found a type checker that doesn't flag my code as “possibly incorrect” because pandas and numpy and stuff love to have like#seventeen different possible return types for a given function#and I don't want them doing static analysis on the whole library because that's why pylyzer has stack overflows out the ass
5 notes
·
View notes
Text
Learning a new computer language is a fresh kind of hell. like what the hell do you mean I don't have the fucking package installed?
#why the hell is numpy not standard anyway#and I fucking have what ever the fuck matplotlib.pyplot as plt is#at least I could read Java#Its not even my code#computer science#python#computer languages#computers#programming
4 notes
·
View notes
Text
Man why is working with web apps so obnoxious. "422 unprocessable entity teehee. Good luck figuring out which entity it is, much less why I refuse to process it"
#i almost went apeshit when fastapi refused to take a numpy int because it WOULDN'T TELL ME WHICH VARIABLE WAS THE PROBLEM#luckily i realized i could put a breakpoint right into my python code before i went entirely off the deep end#programming
10 notes
·
View notes
Text
8 notes
·
View notes
Text
Pandas . . . . for more information and tutorial https://bit.ly/3jqTlRP check the above link
2 notes
·
View notes
Text
Aprende a analizar datos con python!
En este curso aprenderás cómo analizar datos en Python usando matrices multidimensionales en numpy, a manipular DataFrames en pandas, a usar la biblioteca SciPy de rutinas matemáticas y a realizar aprendizaje automático usando scikit-learn. Comienza ya mismo! Pasarás de comprender los conceptos básicos de Python a explorar muchos tipos diferentes de datos a través de clases, laboratorios…
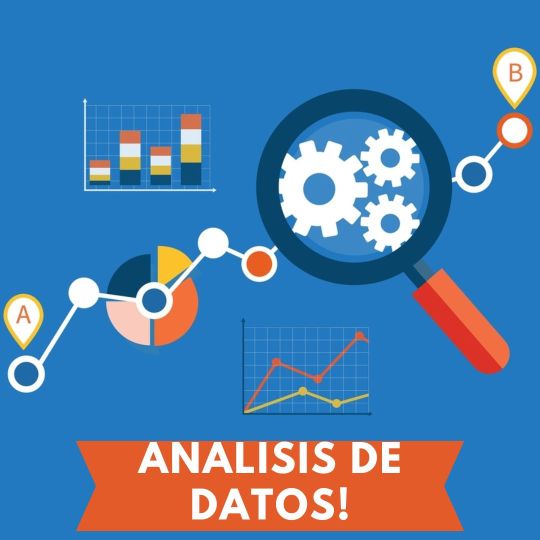
View On WordPress
#analisis de datos#Bibliotecas#curso#curso online#DataFrames#datos#numpy#online#Pandas#programacion#python
3 notes
·
View notes
Text
can someone who's better at coding please tell me what this bug is
(chessgamesarr is a dtype=str numpy array)
why is it cutting the last two characters off why is it doing that i thought it might be a character limit thing but that only is 58->56 long that would be a weird number to cut off at
3 notes
·
View notes
Text
making me code problems to do with matrices without numpy should be illegal im not kidding
#ewwwww this is horrible. i did it with numpy just to see how much cleaner it is and now i need to convert it back to regular python. gross#tbh in my modules im always using np and scikit. so why can't i always use them#finished the num an problem sheets for the first week tho!! the semester hasnt even started im just scared#why is the computer not infallible... i hate floating point errors just do what i tell u to do correctly lol???#joking. but also#.ramblings
1 note
·
View note
Text
What if instead of coding I destroy my computer
#uni talks about the universe#coding#python#numpy#THAT BITCH#WHAT DO YOU MEAN MODULE NOT FOUND#I JUST FUCKING INSTALLED IT
1 note
·
View note
Text
Day 2 - 14th May, 2023
Today I learned about data types in NumPy, and also the different ways of type casting. It was a short study session, because I went out for lunch :P and also had a terrible flare afterwards.
The output of the code above is [ 1 2 3 ] as it converts the floating values into integer values.
🎧 321 blast off - PmBata
#dailyfoxposts#30 days of productivity#30 dop#30doc#studyblr#study#studyspo#codeblr#coding#snappify#python#numpy
19 notes
·
View notes
Text
Not all missing data is the same! 🧩 💡 MCAR: Missing Completely At Random (pure luck). 💡 MAR: Missing At Random (depends on observed data). 💡 MNAR: Missing Not At Random (hidden for a reason).
Understanding these types is the first step to tackling missing data like a pro! 🚀
.
.
.
.
For more details contact Phone: 9948861888 Email: [email protected] Website: datascienceschool.in
#datascience#datascienceschool#ai#machinelearning#data scientist#data#bigdata#learndatascience#database#python#careergrowth#numpy#offlinetraining#online#online training#careersuccess#skilldevelopment
0 notes
Text
youtube
1 note
·
View note
Text
Pandas, NumPy, and Matplotlib are three powerful Python libraries essential for data analysis. Pandas provides high-performance data structures like DataFrames and Series for data manipulation and analysis. NumPy offers efficient numerical computations with multi-dimensional arrays, enabling mathematical operations on large datasets.
0 notes
Text
Python NumPy Basics Made Easy: A Complete Tutorial for Beginners
If you're new to programming or data analysis, learning NumPy is an essential step in your journey. NumPy, short for Numerical Python, is a powerful Python library designed to work with numerical data efficiently. It’s widely used in data science, machine learning, and scientific computing.
What is NumPy?
NumPy is a library that provides support for handling large arrays and matrices of numerical data. It also includes a vast collection of mathematical functions to process and analyze this data easily.
Why Should Beginners Learn NumPy?
Speed and Efficiency: Operations on large datasets are faster and more efficient in NumPy compared to Python lists.
Simplified Operations: It simplifies complex tasks like matrix operations and statistical calculations.
Wide Applications: From finance to physics, it’s used across industries for data analysis and visualization.
How to Start with NumPy
To begin, familiarize yourself with basic concepts like arrays, shapes, and dimensions. Arrays are central to NumPy—they are like advanced lists that allow you to perform mathematical operations directly. Learn how to create and manipulate these arrays as a starting point.
NumPy opens the door to mastering Python-based data analysis and serves as a foundation for more advanced libraries like Pandas and TensorFlow.
For a deeper dive into NumPy concepts, visit Tutorial and Example’s NumPy Tutorial and enhance your learning with practical examples!
0 notes
Video
youtube
Numpy- Scientific Calculation library #datacleaning#python #numpy #dataa...
0 notes