#openwashing
Explore tagged Tumblr posts
Text
10/28/24
Whoops looks like most "open source" ai models aren't actually open source.
77 notes
·
View notes
Text
"Open" "AI" isn’t
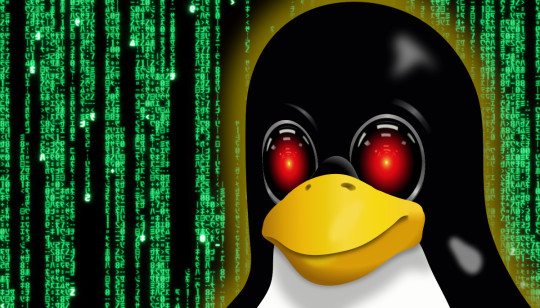
Tomorrow (19 Aug), I'm appearing at the San Diego Union-Tribune Festival of Books. I'm on a 2:30PM panel called "Return From Retirement," followed by a signing:
https://www.sandiegouniontribune.com/festivalofbooks
The crybabies who freak out about The Communist Manifesto appearing on university curriculum clearly never read it – chapter one is basically a long hymn to capitalism's flexibility and inventiveness, its ability to change form and adapt itself to everything the world throws at it and come out on top:
https://www.marxists.org/archive/marx/works/1848/communist-manifesto/ch01.htm#007
Today, leftists signal this protean capacity of capital with the -washing suffix: greenwashing, genderwashing, queerwashing, wokewashing – all the ways capital cloaks itself in liberatory, progressive values, while still serving as a force for extraction, exploitation, and political corruption.
A smart capitalist is someone who, sensing the outrage at a world run by 150 old white guys in boardrooms, proposes replacing half of them with women, queers, and people of color. This is a superficial maneuver, sure, but it's an incredibly effective one.
In "Open (For Business): Big Tech, Concentrated Power, and the Political Economy of Open AI," a new working paper, Meredith Whittaker, David Gray Widder and Sarah B Myers document a new kind of -washing: openwashing:
https://papers.ssrn.com/sol3/papers.cfm?abstract_id=4543807
Openwashing is the trick that large "AI" companies use to evade regulation and neutralizing critics, by casting themselves as forces of ethical capitalism, committed to the virtue of openness. No one should be surprised to learn that the products of the "open" wing of an industry whose products are neither "artificial," nor "intelligent," are also not "open." Every word AI huxters say is a lie; including "and," and "the."
So what work does the "open" in "open AI" do? "Open" here is supposed to invoke the "open" in "open source," a movement that emphasizes a software development methodology that promotes code transparency, reusability and extensibility, which are three important virtues.
But "open source" itself is an offshoot of a more foundational movement, the Free Software movement, whose goal is to promote freedom, and whose method is openness. The point of software freedom was technological self-determination, the right of technology users to decide not just what their technology does, but who it does it to and who it does it for:
https://locusmag.com/2022/01/cory-doctorow-science-fiction-is-a-luddite-literature/
The open source split from free software was ostensibly driven by the need to reassure investors and businesspeople so they would join the movement. The "free" in free software is (deliberately) ambiguous, a bit of wordplay that sometimes misleads people into thinking it means "Free as in Beer" when really it means "Free as in Speech" (in Romance languages, these distinctions are captured by translating "free" as "libre" rather than "gratis").
The idea behind open source was to rebrand free software in a less ambiguous – and more instrumental – package that stressed cost-savings and software quality, as well as "ecosystem benefits" from a co-operative form of development that recruited tinkerers, independents, and rivals to contribute to a robust infrastructural commons.
But "open" doesn't merely resolve the linguistic ambiguity of libre vs gratis – it does so by removing the "liberty" from "libre," the "freedom" from "free." "Open" changes the pole-star that movement participants follow as they set their course. Rather than asking "Which course of action makes us more free?" they ask, "Which course of action makes our software better?"
Thus, by dribs and drabs, the freedom leeches out of openness. Today's tech giants have mobilized "open" to create a two-tier system: the largest tech firms enjoy broad freedom themselves – they alone get to decide how their software stack is configured. But for all of us who rely on that (increasingly unavoidable) software stack, all we have is "open": the ability to peer inside that software and see how it works, and perhaps suggest improvements to it:
https://www.youtube.com/watch?v=vBknF2yUZZ8
In the Big Tech internet, it's freedom for them, openness for us. "Openness" – transparency, reusability and extensibility – is valuable, but it shouldn't be mistaken for technological self-determination. As the tech sector becomes ever-more concentrated, the limits of openness become more apparent.
But even by those standards, the openness of "open AI" is thin gruel indeed (that goes triple for the company that calls itself "OpenAI," which is a particularly egregious openwasher).
The paper's authors start by suggesting that the "open" in "open AI" is meant to imply that an "open AI" can be scratch-built by competitors (or even hobbyists), but that this isn't true. Not only is the material that "open AI" companies publish insufficient for reproducing their products, even if those gaps were plugged, the resource burden required to do so is so intense that only the largest companies could do so.
Beyond this, the "open" parts of "open AI" are insufficient for achieving the other claimed benefits of "open AI": they don't promote auditing, or safety, or competition. Indeed, they often cut against these goals.
"Open AI" is a wordgame that exploits the malleability of "open," but also the ambiguity of the term "AI": "a grab bag of approaches, not… a technical term of art, but more … marketing and a signifier of aspirations." Hitching this vague term to "open" creates all kinds of bait-and-switch opportunities.
That's how you get Meta claiming that LLaMa2 is "open source," despite being licensed in a way that is absolutely incompatible with any widely accepted definition of the term:
https://blog.opensource.org/metas-llama-2-license-is-not-open-source/
LLaMa-2 is a particularly egregious openwashing example, but there are plenty of other ways that "open" is misleadingly applied to AI: sometimes it means you can see the source code, sometimes that you can see the training data, and sometimes that you can tune a model, all to different degrees, alone and in combination.
But even the most "open" systems can't be independently replicated, due to raw computing requirements. This isn't the fault of the AI industry – the computational intensity is a fact, not a choice – but when the AI industry claims that "open" will "democratize" AI, they are hiding the ball. People who hear these "democratization" claims (especially policymakers) are thinking about entrepreneurial kids in garages, but unless these kids have access to multi-billion-dollar data centers, they can't be "disruptors" who topple tech giants with cool new ideas. At best, they can hope to pay rent to those giants for access to their compute grids, in order to create products and services at the margin that rely on existing products, rather than displacing them.
The "open" story, with its claims of democratization, is an especially important one in the context of regulation. In Europe, where a variety of AI regulations have been proposed, the AI industry has co-opted the open source movement's hard-won narrative battles about the harms of ill-considered regulation.
For open source (and free software) advocates, many tech regulations aimed at taming large, abusive companies – such as requirements to surveil and control users to extinguish toxic behavior – wreak collateral damage on the free, open, user-centric systems that we see as superior alternatives to Big Tech. This leads to the paradoxical effect of passing regulation to "punish" Big Tech that end up simply shaving an infinitesimal percentage off the giants' profits, while destroying the small co-ops, nonprofits and startups before they can grow to be a viable alternative.
The years-long fight to get regulators to understand this risk has been waged by principled actors working for subsistence nonprofit wages or for free, and now the AI industry is capitalizing on lawmakers' hard-won consideration for collateral damage by claiming to be "open AI" and thus vulnerable to overbroad regulation.
But the "open" projects that lawmakers have been coached to value are precious because they deliver a level playing field, competition, innovation and democratization – all things that "open AI" fails to deliver. The regulations the AI industry is fighting also don't necessarily implicate the speech implications that are core to protecting free software:
https://www.eff.org/deeplinks/2015/04/remembering-case-established-code-speech
Just think about LLaMa-2. You can download it for free, along with the model weights it relies on – but not detailed specs for the data that was used in its training. And the source-code is licensed under a homebrewed license cooked up by Meta's lawyers, a license that only glancingly resembles anything from the Open Source Definition:
https://opensource.org/osd/
Core to Big Tech companies' "open AI" offerings are tools, like Meta's PyTorch and Google's TensorFlow. These tools are indeed "open source," licensed under real OSS terms. But they are designed and maintained by the companies that sponsor them, and optimize for the proprietary back-ends each company offers in its own cloud. When programmers train themselves to develop in these environments, they are gaining expertise in adding value to a monopolist's ecosystem, locking themselves in with their own expertise. This a classic example of software freedom for tech giants and open source for the rest of us.
One way to understand how "open" can produce a lock-in that "free" might prevent is to think of Android: Android is an open platform in the sense that its sourcecode is freely licensed, but the existence of Android doesn't make it any easier to challenge the mobile OS duopoly with a new mobile OS; nor does it make it easier to switch from Android to iOS and vice versa.
Another example: MongoDB, a free/open database tool that was adopted by Amazon, which subsequently forked the codebase and tuning it to work on their proprietary cloud infrastructure.
The value of open tooling as a stickytrap for creating a pool of developers who end up as sharecroppers who are glued to a specific company's closed infrastructure is well-understood and openly acknowledged by "open AI" companies. Zuckerberg boasts about how PyTorch ropes developers into Meta's stack, "when there are opportunities to make integrations with products, [so] it’s much easier to make sure that developers and other folks are compatible with the things that we need in the way that our systems work."
Tooling is a relatively obscure issue, primarily debated by developers. A much broader debate has raged over training data – how it is acquired, labeled, sorted and used. Many of the biggest "open AI" companies are totally opaque when it comes to training data. Google and OpenAI won't even say how many pieces of data went into their models' training – let alone which data they used.
Other "open AI" companies use publicly available datasets like the Pile and CommonCrawl. But you can't replicate their models by shoveling these datasets into an algorithm. Each one has to be groomed – labeled, sorted, de-duplicated, and otherwise filtered. Many "open" models merge these datasets with other, proprietary sets, in varying (and secret) proportions.
Quality filtering and labeling for training data is incredibly expensive and labor-intensive, and involves some of the most exploitative and traumatizing clickwork in the world, as poorly paid workers in the Global South make pennies for reviewing data that includes graphic violence, rape, and gore.
Not only is the product of this "data pipeline" kept a secret by "open" companies, the very nature of the pipeline is likewise cloaked in mystery, in order to obscure the exploitative labor relations it embodies (the joke that "AI" stands for "absent Indians" comes out of the South Asian clickwork industry).
The most common "open" in "open AI" is a model that arrives built and trained, which is "open" in the sense that end-users can "fine-tune" it – usually while running it on the manufacturer's own proprietary cloud hardware, under that company's supervision and surveillance. These tunable models are undocumented blobs, not the rigorously peer-reviewed transparent tools celebrated by the open source movement.
If "open" was a way to transform "free software" from an ethical proposition to an efficient methodology for developing high-quality software; then "open AI" is a way to transform "open source" into a rent-extracting black box.
Some "open AI" has slipped out of the corporate silo. Meta's LLaMa was leaked by early testers, republished on 4chan, and is now in the wild. Some exciting stuff has emerged from this, but despite this work happening outside of Meta's control, it is not without benefits to Meta. As an infamous leaked Google memo explains:
Paradoxically, the one clear winner in all of this is Meta. Because the leaked model was theirs, they have effectively garnered an entire planet's worth of free labor. Since most open source innovation is happening on top of their architecture, there is nothing stopping them from directly incorporating it into their products.
https://www.searchenginejournal.com/leaked-google-memo-admits-defeat-by-open-source-ai/486290/
Thus, "open AI" is best understood as "as free product development" for large, well-capitalized AI companies, conducted by tinkerers who will not be able to escape these giants' proprietary compute silos and opaque training corpuses, and whose work product is guaranteed to be compatible with the giants' own systems.
The instrumental story about the virtues of "open" often invoke auditability: the fact that anyone can look at the source code makes it easier for bugs to be identified. But as open source projects have learned the hard way, the fact that anyone can audit your widely used, high-stakes code doesn't mean that anyone will.
The Heartbleed vulnerability in OpenSSL was a wake-up call for the open source movement – a bug that endangered every secure webserver connection in the world, which had hidden in plain sight for years. The result was an admirable and successful effort to build institutions whose job it is to actually make use of open source transparency to conduct regular, deep, systemic audits.
In other words, "open" is a necessary, but insufficient, precondition for auditing. But when the "open AI" movement touts its "safety" thanks to its "auditability," it fails to describe any steps it is taking to replicate these auditing institutions – how they'll be constituted, funded and directed. The story starts and ends with "transparency" and then makes the unjustifiable leap to "safety," without any intermediate steps about how the one will turn into the other.
It's a Magic Underpants Gnome story, in other words:
Step One: Transparency
Step Two: ??
Step Three: Safety
https://www.youtube.com/watch?v=a5ih_TQWqCA
Meanwhile, OpenAI itself has gone on record as objecting to "burdensome mechanisms like licenses or audits" as an impediment to "innovation" – all the while arguing that these "burdensome mechanisms" should be mandatory for rival offerings that are more advanced than its own. To call this a "transparent ruse" is to do violence to good, hardworking transparent ruses all the world over:
https://openai.com/blog/governance-of-superintelligence
Some "open AI" is much more open than the industry dominating offerings. There's EleutherAI, a donor-supported nonprofit whose model comes with documentation and code, licensed Apache 2.0. There are also some smaller academic offerings: Vicuna (UCSD/CMU/Berkeley); Koala (Berkeley) and Alpaca (Stanford).
These are indeed more open (though Alpaca – which ran on a laptop – had to be withdrawn because it "hallucinated" so profusely). But to the extent that the "open AI" movement invokes (or cares about) these projects, it is in order to brandish them before hostile policymakers and say, "Won't someone please think of the academics?" These are the poster children for proposals like exempting AI from antitrust enforcement, but they're not significant players in the "open AI" industry, nor are they likely to be for so long as the largest companies are running the show:
https://papers.ssrn.com/sol3/papers.cfm?abstract_id=4493900
I'm kickstarting the audiobook for "The Internet Con: How To Seize the Means of Computation," a Big Tech disassembly manual to disenshittify the web and make a new, good internet to succeed the old, good internet. It's a DRM-free book, which means Audible won't carry it, so this crowdfunder is essential. Back now to get the audio, Verso hardcover and ebook:
http://seizethemeansofcomputation.org
If you'd like an essay-formatted version of this post to read or share, here's a link to it on pluralistic.net, my surveillance-free, ad-free, tracker-free blog:
https://pluralistic.net/2023/08/18/openwashing/#you-keep-using-that-word-i-do-not-think-it-means-what-you-think-it-means
Image: Cryteria (modified) https://commons.wikimedia.org/wiki/File:HAL9000.svg
CC BY 3.0 https://creativecommons.org/licenses/by/3.0/deed.en
#pluralistic#llama-2#meta#openwashing#floss#free software#open ai#open source#osi#open source initiative#osd#open source definition#code is speech
250 notes
·
View notes
Text
Briefing quotidiano: l'"openwashing" affligge la grande intelligenza artificiale Intelligenza Artificiale Open Source: tra nome e realtà La cosiddetta intelligenza artificiale open source suscita interrogativi sulla sua effettiva apertura, dimostrando che il concetto potrebbe essere fuorviante rispetto alla realtà delle pratiche in atto. Stimolazione delle cellule nervose genitali: implicazioni sui topi Uno studio rivela che le vibrazioni a bassa frequenza stimolano le cellule nervose genitali dei topi, aprendo interessanti scenari di ricerca nel campo della neuroscienza. La ricerca sulla schizofrenia e il focus sull’individuo Un approccio centrato sulle persone è fondamentale per la ricerca sulla schizofrenia, ponendo l’individuo al centro delle indagini per migliorare diagnosi e terapie.
0 notes
Text

Result Winter Essentials Team reversible beanie
6 gauge dense knit. Reversible. Stripe knit. Long length. Solid colour inner. Windproof.Decoration Guidelines:Decorating methods: Embroidery. Decorators access point: OpenWashing Instructions40 degree. Iron on low heat. Do not bleach. Do not tumble dry.
0 notes
Text
Openwashing in open data: some recent examples
Post: 11 November 2018
New blog post on my website:
Openwashing in open data: some recent examples
1 note
·
View note
Text
Open Education And Social Justice JIME: A Small Step For Humanity, A Large Step For Academia
Open Education And Social Justice JIME: A Small Step For Humanity, A Large Step For Academia
The May, 2020 issue of the Journal of Interactive Media in Education (JIME) is a special collection on “Open Education and Social Justice.” It is a theoretical look at issues of inclusion, diversity and “participatory parity” in the cavalcade of Open Education, and particularly Open Educational Resources (OER).
It is an open secret of sorts that OER, while an important and well-meaning effort,…
View On WordPress
#amartya sen#john rawls#journal of interactive media in education#laura czerniewicz#nancy fraser#oer#open education#open educational resources#open university#openwashing#sarah lambert#social justice#taskeen adam#textbooks
0 notes
Quote
Key terms in the accountability field are both politically constructed and contested. Accountability – as a “trans-ideological” idea – is up for grabs. Anti-accountability forces have been adept at appropriating accountability ideas (e.g., “fake news,” “drain the swamp” and manufactured online civic engagement). The civic tech field now faces the challenge of communicating accountability ideas more broadly. This involves taking into account the ways in which accountability keywords have different meanings, to different actors, in different contexts – and in different languages. This talk argues that communicating accountability strategies should rely on conceptual and cross-cultural translation rather than awkward attempts at direct linguistic translation. To illustrate how accountability keywords are both politically constructed and contested, this presentation briefly reflects on the origins, circulation, and transformation of six relevant terms: accountability, the right to know, targeted transparency, whistle-blowers, openwashing, and sandwich strategies. The conclusion calls for a two-track approach to communicate public accountability strategies, which involves (1) searching within popular cultures to find existing terms or phrases that can be repurposed, and (2) inventing new discourses that communicate ideas about public accountability that resonate with culturally grounded common-sense understandings.
Political Construction of Accountability Keywords: Lessons From Action-Research – Accountability Research Center
0 notes
Link
Corporations masking themselves as "foundations" for #openwashing by collab https://t.co/9odPugpxOU
— Dr. Roy Schestowitz (罗伊) (@schestowitz) September 29, 2019
via: https://ift.tt/1GAs5mb
0 notes
Photo

¿Listos para el cambio de temporada? Esta semana comienza a bajar las temperaturas. Que no te agarre desprevenida!! Ven a nuestra tienda #openwashleon⚠️ Contamos con lavadora XL de gran capacidad para lavar hasta 2 edredones por tan solo 8 €.!😉 #openwashleon #openwash #sanandresdelrabanedo #cambiodetemporada #bajanlastemperaturas #otoño #edredroneslimpios #fagor #leonesp #lavanderiaautomatica #lavanderiadeautoservicio (en San Andrés del Rabanedo, Spain) https://www.instagram.com/p/BoTq1xCH45-/?utm_source=ig_tumblr_share&igshid=pwbo9z8sdjj3
#openwashleon⚠️#openwashleon#openwash#sanandresdelrabanedo#cambiodetemporada#bajanlastemperaturas#otoño#edredroneslimpios#fagor#leonesp#lavanderiaautomatica#lavanderiadeautoservicio
0 notes
Text
“I’m an open source user/deleloper now because Microsoft declared …. — Dr. Roy Schestowitz (罗伊)
“I’m an open source user/deleloper now because Microsoft declared .NET “core” is “open source” and Visual Studio “code” is now “open source” (with #surveillance right down to the code)” #opencore #openwashing
via “I’m an open source user/deleloper now because Microsoft declared …. — Dr. Roy Schestowitz (罗伊)
View On WordPress
0 notes
Text
What kind of bubble is AI?

My latest column for Locus Magazine is "What Kind of Bubble is AI?" All economic bubbles are hugely destructive, but some of them leave behind wreckage that can be salvaged for useful purposes, while others leave nothing behind but ashes:
https://locusmag.com/2023/12/commentary-cory-doctorow-what-kind-of-bubble-is-ai/
Think about some 21st century bubbles. The dotcom bubble was a terrible tragedy, one that drained the coffers of pension funds and other institutional investors and wiped out retail investors who were gulled by Superbowl Ads. But there was a lot left behind after the dotcoms were wiped out: cheap servers, office furniture and space, but far more importantly, a generation of young people who'd been trained as web makers, leaving nontechnical degree programs to learn HTML, perl and python. This created a whole cohort of technologists from non-technical backgrounds, a first in technological history. Many of these people became the vanguard of a more inclusive and humane tech development movement, and they were able to make interesting and useful services and products in an environment where raw materials – compute, bandwidth, space and talent – were available at firesale prices.
Contrast this with the crypto bubble. It, too, destroyed the fortunes of institutional and individual investors through fraud and Superbowl Ads. It, too, lured in nontechnical people to learn esoteric disciplines at investor expense. But apart from a smattering of Rust programmers, the main residue of crypto is bad digital art and worse Austrian economics.
Or think of Worldcom vs Enron. Both bubbles were built on pure fraud, but Enron's fraud left nothing behind but a string of suspicious deaths. By contrast, Worldcom's fraud was a Big Store con that required laying a ton of fiber that is still in the ground to this day, and is being bought and used at pennies on the dollar.
AI is definitely a bubble. As I write in the column, if you fly into SFO and rent a car and drive north to San Francisco or south to Silicon Valley, every single billboard is advertising an "AI" startup, many of which are not even using anything that can be remotely characterized as AI. That's amazing, considering what a meaningless buzzword AI already is.
So which kind of bubble is AI? When it pops, will something useful be left behind, or will it go away altogether? To be sure, there's a legion of technologists who are learning Tensorflow and Pytorch. These nominally open source tools are bound, respectively, to Google and Facebook's AI environments:
https://pluralistic.net/2023/08/18/openwashing/#you-keep-using-that-word-i-do-not-think-it-means-what-you-think-it-means
But if those environments go away, those programming skills become a lot less useful. Live, large-scale Big Tech AI projects are shockingly expensive to run. Some of their costs are fixed – collecting, labeling and processing training data – but the running costs for each query are prodigious. There's a massive primary energy bill for the servers, a nearly as large energy bill for the chillers, and a titanic wage bill for the specialized technical staff involved.
Once investor subsidies dry up, will the real-world, non-hyperbolic applications for AI be enough to cover these running costs? AI applications can be plotted on a 2X2 grid whose axes are "value" (how much customers will pay for them) and "risk tolerance" (how perfect the product needs to be).
Charging teenaged D&D players $10 month for an image generator that creates epic illustrations of their characters fighting monsters is low value and very risk tolerant (teenagers aren't overly worried about six-fingered swordspeople with three pupils in each eye). Charging scammy spamfarms $500/month for a text generator that spits out dull, search-algorithm-pleasing narratives to appear over recipes is likewise low-value and highly risk tolerant (your customer doesn't care if the text is nonsense). Charging visually impaired people $100 month for an app that plays a text-to-speech description of anything they point their cameras at is low-value and moderately risk tolerant ("that's your blue shirt" when it's green is not a big deal, while "the street is safe to cross" when it's not is a much bigger one).
Morganstanley doesn't talk about the trillions the AI industry will be worth some day because of these applications. These are just spinoffs from the main event, a collection of extremely high-value applications. Think of self-driving cars or radiology bots that analyze chest x-rays and characterize masses as cancerous or noncancerous.
These are high value – but only if they are also risk-tolerant. The pitch for self-driving cars is "fire most drivers and replace them with 'humans in the loop' who intervene at critical junctures." That's the risk-tolerant version of self-driving cars, and it's a failure. More than $100b has been incinerated chasing self-driving cars, and cars are nowhere near driving themselves:
https://pluralistic.net/2022/10/09/herbies-revenge/#100-billion-here-100-billion-there-pretty-soon-youre-talking-real-money
Quite the reverse, in fact. Cruise was just forced to quit the field after one of their cars maimed a woman – a pedestrian who had not opted into being part of a high-risk AI experiment – and dragged her body 20 feet through the streets of San Francisco. Afterwards, it emerged that Cruise had replaced the single low-waged driver who would normally be paid to operate a taxi with 1.5 high-waged skilled technicians who remotely oversaw each of its vehicles:
https://www.nytimes.com/2023/11/03/technology/cruise-general-motors-self-driving-cars.html
The self-driving pitch isn't that your car will correct your own human errors (like an alarm that sounds when you activate your turn signal while someone is in your blind-spot). Self-driving isn't about using automation to augment human skill – it's about replacing humans. There's no business case for spending hundreds of billions on better safety systems for cars (there's a human case for it, though!). The only way the price-tag justifies itself is if paid drivers can be fired and replaced with software that costs less than their wages.
What about radiologists? Radiologists certainly make mistakes from time to time, and if there's a computer vision system that makes different mistakes than the sort that humans make, they could be a cheap way of generating second opinions that trigger re-examination by a human radiologist. But no AI investor thinks their return will come from selling hospitals that reduce the number of X-rays each radiologist processes every day, as a second-opinion-generating system would. Rather, the value of AI radiologists comes from firing most of your human radiologists and replacing them with software whose judgments are cursorily double-checked by a human whose "automation blindness" will turn them into an OK-button-mashing automaton:
https://pluralistic.net/2023/08/23/automation-blindness/#humans-in-the-loop
The profit-generating pitch for high-value AI applications lies in creating "reverse centaurs": humans who serve as appendages for automation that operates at a speed and scale that is unrelated to the capacity or needs of the worker:
https://pluralistic.net/2022/04/17/revenge-of-the-chickenized-reverse-centaurs/
But unless these high-value applications are intrinsically risk-tolerant, they are poor candidates for automation. Cruise was able to nonconsensually enlist the population of San Francisco in an experimental murderbot development program thanks to the vast sums of money sloshing around the industry. Some of this money funds the inevitabilist narrative that self-driving cars are coming, it's only a matter of when, not if, and so SF had better get in the autonomous vehicle or get run over by the forces of history.
Once the bubble pops (all bubbles pop), AI applications will have to rise or fall on their actual merits, not their promise. The odds are stacked against the long-term survival of high-value, risk-intolerant AI applications.
The problem for AI is that while there are a lot of risk-tolerant applications, they're almost all low-value; while nearly all the high-value applications are risk-intolerant. Once AI has to be profitable – once investors withdraw their subsidies from money-losing ventures – the risk-tolerant applications need to be sufficient to run those tremendously expensive servers in those brutally expensive data-centers tended by exceptionally expensive technical workers.
If they aren't, then the business case for running those servers goes away, and so do the servers – and so do all those risk-tolerant, low-value applications. It doesn't matter if helping blind people make sense of their surroundings is socially beneficial. It doesn't matter if teenaged gamers love their epic character art. It doesn't even matter how horny scammers are for generating AI nonsense SEO websites:
https://twitter.com/jakezward/status/1728032634037567509
These applications are all riding on the coattails of the big AI models that are being built and operated at a loss in order to be profitable. If they remain unprofitable long enough, the private sector will no longer pay to operate them.
Now, there are smaller models, models that stand alone and run on commodity hardware. These would persist even after the AI bubble bursts, because most of their costs are setup costs that have already been borne by the well-funded companies who created them. These models are limited, of course, though the communities that have formed around them have pushed those limits in surprising ways, far beyond their original manufacturers' beliefs about their capacity. These communities will continue to push those limits for as long as they find the models useful.
These standalone, "toy" models are derived from the big models, though. When the AI bubble bursts and the private sector no longer subsidizes mass-scale model creation, it will cease to spin out more sophisticated models that run on commodity hardware (it's possible that Federated learning and other techniques for spreading out the work of making large-scale models will fill the gap).
So what kind of bubble is the AI bubble? What will we salvage from its wreckage? Perhaps the communities who've invested in becoming experts in Pytorch and Tensorflow will wrestle them away from their corporate masters and make them generally useful. Certainly, a lot of people will have gained skills in applying statistical techniques.
But there will also be a lot of unsalvageable wreckage. As big AI models get integrated into the processes of the productive economy, AI becomes a source of systemic risk. The only thing worse than having an automated process that is rendered dangerous or erratic based on AI integration is to have that process fail entirely because the AI suddenly disappeared, a collapse that is too precipitous for former AI customers to engineer a soft landing for their systems.
This is a blind spot in our policymakers debates about AI. The smart policymakers are asking questions about fairness, algorithmic bias, and fraud. The foolish policymakers are ensnared in fantasies about "AI safety," AKA "Will the chatbot become a superintelligence that turns the whole human race into paperclips?"
https://pluralistic.net/2023/11/27/10-types-of-people/#taking-up-a-lot-of-space
But no one is asking, "What will we do if" – when – "the AI bubble pops and most of this stuff disappears overnight?"
If you'd like an essay-formatted version of this post to read or share, here's a link to it on pluralistic.net, my surveillance-free, ad-free, tracker-free blog:
https://pluralistic.net/2023/12/19/bubblenomics/#pop
Image: Cryteria (modified) https://commons.wikimedia.org/wiki/File:HAL9000.svg
CC BY 3.0 https://creativecommons.org/licenses/by/3.0/deed.en
--
tom_bullock (modified) https://www.flickr.com/photos/tombullock/25173469495/
CC BY 2.0 https://creativecommons.org/licenses/by/2.0/
4K notes
·
View notes
Text
Key open gov deadline nears with no public action
New Post has been published on https://britishdigitalmarketingnews.com/key-open-gov-deadline-nears-with-no-public-action/
Key open gov deadline nears with no public action
Open Gov
Key open gov deadline nears with no public action
By Alex Howard
Aug 29, 2018
The Open Government Partnership is a global multi-stakeholder initiative that attempts to act as a convening platform for national governments and their publics to create concrete commitments towards democratic reforms. In MSIs, participants voluntarily make collective commitments towards achieving a given goal. The Paris Accord on climate change may be the MSI best known to the public, but there are several other collective governance mechanisms around the world that use the model.
Past and current OGP commitments have included reforms aimed at increasing public access to information, improving good governance, reducing corruption and improving service delivery using new technologies, and engaging the public in public business and processes.
A brief history of OGP
OGP was officially launched by eight founding nations in September 2011, after President Barack Obama outlined a general concept for it in a speech before the United Nations in September 2010. Former U.S. Ambassador to the United Nations Samantha Power once called it Obama’s “signature governance initiative.”
Every two years, participating national governments draft “National Action Plans” in consultation with domestic civil society groups and the public, which the countries then commit to work towards implementing.
OGP has elevated the profile of transparency, accountability issues around the globe, along with elements of participatory democracy, from civic engagement to collaboration. While many participants have continued to engage in anti-democratic behaviors, including passing regressive laws and restricting or even violating various human rights and freedoms, participating in OGP has also contributed to countries enacting new access to information laws and other reforms.
In countries without long traditions of democratic governance, struggling with serious corruption and service delivery problems, OGP can help give the issues of domestic groups and activists more international attention and add pressure on ministers and presidents to follow through on public commitments made before their peers on a public stage.
The existing evidence suggests that while OGP has helped nurture beneficial shared democratic norms among its participants, the reforms associated with it have often been transparency and e-government policies or programs, but not reactive reforms that are responsive to the needs or priorities of civil society actors, or meaningful accountability for bad actors in the public or private sector.
More subtly, participation in OGP can enable ministers, politicians and governments to claim the mantle of openness while they pursue existing agendas for digital transformation and service delivery that are not directly related to transparency, accountability, corruption, or press freedom.
The U.S. role and how it is changing
While the Obama administration had a mixed record on transparency, under President Barack Obama, the United States played a key role as a founding nation, international leader, coalition builder, host and convener.
Obama’s personal participation throughout his second term, combined with his Open Government Directive, signaled that OGP was important to him and an international priority for the nation.
One of the first actions President Donald Trump took in office was to sign a repeal of one of the key initial commitments in the Open Government Partnership — an anti-corruption rule six years in the making regarding disclosure of payments from oil gas and mineral companies to governments. The move was symbolic of broader quiet moves that harm global governance.
Under Trump, the United States government has moved from /open to /closed.Open government has regressed under the president, with well-documented “information darkness” spreading across agencies, further erosion of trust in government and the office of the presidency itself, and the ongoing, unconstitutional corruption of the conflicts of interest that Trump retained in office.
The pivot away from sunshine
For eight months, this White House had little or nothing to say about sunshine laws, open government programs or policies. In September 2017, however, the Trump administration committed to participating in the Open Government Partnership after substantially ignoring or erasing existing open government programs and initiatives, with the notable choice to end disclosure of White House visitor logs.
After the administration announced its intent in a sparsely attended forum in the U.S. General Services administration, it created open.usa.gov to host OGP-related information, set up a Github instance to collect suggested commitments, hosted two workshops in that agency, and a third at the National Archives.
At the end of October, however, the White House informed OGP that it would delay submitting a plan. OGP moved the cohort in which the USA sits, a bureaucratic maneuver that meant the USA was no longer “late.”
In May 2018, the White House quietly announced that it would again pursue co-creating a new national action plan, opened public comment in June, and hosted two more workshops at the GSA headquarters in DC. Administration officials asked participants for concrete, meaningful commitments and suggested initiatives within the President’s Management Agenda.
A timeline published in June put the deadline for the fourth NAP on Aug. 31, 2018, but there’s been no public activity around the plan since the workshops nor disclosure of private development or drafting.
A path forward?
Can stakeholders and activists take this White House at its word that it is sincerely interested in advancing open data and open government policy?
Yes and no.
The Trump administration has demonstrated interest and commitment to supporting open data relevant to economic activity and implementing the DATA Act, improving how spending information is disclosed to the public.
At the same time, Trump administration has actively slowed or prevented disclosures that would be damaging to its own political interests or those of the business interests.
While the “war on data” that federal watchdogs feared has not been declared, political threats to scientific and data integrity have continued to grow, with many continued unannounced changes and reductions to public information on federal websites.
Many of the concerns that open government leaders had in 2016 have been realized over the tumult of the Trump administration and met with letters, complaints, protests and lawsuits.
OpenTheGovernment executive director Lisa Rosenberg said when the White House Office of Management Budget delayed publishing a fourth National Action Plan for the Open Government Partnership on Halloween in 2017, “an administration that has been antagonistic to a free press, withheld the president’s tax returns, kept secret White House Visitors logs, targeted protesters for surveillance and monitoring, and backed out of commitments to disclose information about warrantless surveillance programs, seems unlikely to embrace meaningful commitments under a voluntary, international agreement.”
A year later, the public will see whether that remains true.
As in other nations, if the White House submits a plan, look for it to position existing digital government initiatives and emerging technology programs – like those outlined in the Presidential Management Agenda – in a handful of commitments in a new open government plan. (That’s a maneuver that has been aptly described as “openwashing” in the past.)
It’s also possible that the administration will keep delaying and drawing the consultation process out, given the absence of meaningful consequences for doing so in the court of public opinion, particularly at a historic moment when the presidency itself typified by crisis.
In either case, it’s worth noting that there’s less good faith remaining on these issues in D.C. In the summer of 2018, most of the members of the open government community — as represented by the national coalition of organizations that work on transparency, accountability, ethics and good government — chose not to participate in the workshops in D.C. or propose commitments to the Github forum.
Given the challenges that persisted for organizations that tried to use OGP as an effective platform for achieving their advocacy and reform goals in the past, there’s also growing doubt and skepticism across U.S. civil society regarding how effective OGP can be as a mechanism for advocacy and substantive reforms.
Organizations in this community could file a complaint to OGP about a flawed consultation that was marked by minimal public engagement and participation – but that action isn’t likely to galvanize the U.S. government to change its behavior or make new commitments to a voluntary international organization.
If a new plan does not include some commitments that are responsive to the priorities of open government advocates and watchdogs — like some of the federal ethics reforms that Open Government Partnership researchers highlight as a missing component of the past three plans – organizations can and should highlight the Trump administration’s shadowy record to the public, press and OGP itself, using the initiative as a platform to hold the U.S. government accountable.
Given the well-documented failings of the Trump administration on open government, more criticism will come as little surprise, but it will be important to set the record straight for posterity and the public through trustworthy, nonpartisan institutions.
In the summer of 2018, an increasing proportion of the American public now tells Pew Research that President Trump “has definitely or probably not run “an open and transparent administration.” But there also has been an increase in the proportion of people who think that Trump definitely has done so, likely in part because the president has made that claim repeatedly.
In fact, in 2018 more Republicans now say Trump has run an open and transparent administration, over a time period when his administration’s record on open government if anything, grew worse in its second year: secretive, corrupt, hostile to journalism and whistleblowers, mired in scandal, shadowed by foreign entanglements, and characterized by false and misleading claims made to the public by a president whose tangled relationship with the truth is unprecedented in American history.
About the Author
Alex Howard is an open government advocate and civic tech journalist in Washington, D.C. Follow him on Twitter at @digiphile
Source: https://fcw.com/articles/2018/08/29/howard-open-gov-under-trump.aspx
0 notes
Text

Result Headwear Sport cap
None-crease sport fabric. Recycled PU peak, just unfold and wear. Featherweight. Tear away labels. Quick dry. Unlined. Reflective detail to peak and crown. Low profile jockey style. 2 panel with mesh side panels. Pre-curved peak. Tear release fabric size adjuster.Decoration Guidelines:Decorating methods: Embroidery Transfer print. Decorators access point: OpenWashing InstructionsSponge clean only, do not bleach, do not iron, do not dry clean, do not tumble dry.
0 notes
Text
The 2018 ‘Horizon Report’ Is Late. But It Almost Never Emerged.
The story behind the latest Horizon Report—which ranks tech trends in higher education—is easily more dramatic than the document’s actual conclusions. But both are available as of today.
A panel of 71 experts convened by the New Media Consortium worked for months to dig through research and make recommendations for this year’s report, following a process that had been honed over several years. Then, as the report neared completion, the New Media Consortium abruptly shut down amid mysterious financial troubles, and it was unclear whether the work would ever reach the public.
In February, Educause bought the assets of the New Media Consortium and pledged to continue the Horizon Report series, and to complete and publish the 2018 edition. The report is much later than usual—NMC had planned to release it in March—but Educause officials say they took pains to uphold the traditions of that group.
An Educause official tasked with taking over this effort tries to reassure skeptical observers that the latest version would be faithful to the original. “We’re very committed to following the format of the previous NMC report,” says Noreen Barajas-Murphy, interim director of academic community programs, who led the completion of the document. “It was really important to us that it have not just the look, but that it also read and felt like a Horizon Report.”
And as in past years, the report will be free, and Educause says it does not see the project as a revenue source.
Barajas-Murphy says that Educause is already starting the planning for the 2019 Horizon Report for higher education, which is scheduled for release in February, at the group’s ELI conference.
The plan is to form a new panel of experts—some of them from previous NMC panels, and some from the Educause community—to make recommendations for next year’s report. And the group will continue to use the software developed by NMC to coordinate the research, which was among the assets purchased by Educause.
Not everything will remain exactly the same, though. One possible change will be to incorporate “predictive validity” techniques that Educause applies in some of its other research, to try to make the process of identifying trends more rigorous, says Barajas-Murphy.
What’s In This Year’s Edition
The Horizon Report aims to identify themes and challenges in higher education and technology, and predict which trends may materialize in the near future. And this year’s predictions range from the abstract, such as “advancing cultures of innovation,” to more specific ones like an increasing appearance of new interdisciplinary studies on campus.
At a time when traditional degree paths such as history or the humanities are under greater scrutiny, the report asserts that interdisciplinary studies will be increasingly thought of as a way to maintain “the relevance of traditional academic disciplines by fostering new and creative programs of study.”
These programs are also, in a way, a “response to [funding] scarcity, and how institutions are both making the best use of things they already have on campus and then weaving them together to provide students with an interdisciplinary experience,” says Barajas-Murphy.
Several of the ideas and challenges have landed a spot in the previous editions of the report. Open educational resources (OER) have been included as key trends since 2013, for example. But the report argues that there have been small changes in the trends that have been cited year after year.
With OER, the report notes, “initial advances in the authoring platform or curation method of open resources is now overshadowed by campuswide OER initiatives and sophisticated publishing options that blend adaptive elements into an OER text.”
David Thomas, director of academic technology at the University of Colorado at Denver, says that even repeated trends change over time. “When open educational resources first emerged, it was the wild west. Anyone who wanted to contribute content could,” he says. These days, OER quality frameworks have emerged and the technology is more commonplace on campuses. But new challenges have evolved with the industry, too, such as the concern that some publishers and companies are merely claiming to be open for marketing purposes—a practice sometimes referred to as “openwashing.”
Previous versions of the report, which go back 16 years, have been somewhat hit-or-miss when it comes to their predictions.
The 2014 report claimed that virtual assistants would rise to prominence on college campuses within four to five years. Today, it is becoming increasingly common to see devices like Amazon’s Alexa integrated into dorm rooms and learning spaces. (Hit.)
The 2015 report, on the other hand, predicted that wearable technologies, including Google Glass, would find a place in higher-ed research settings. (Big miss.)
The report does not focus on whether past predictions came to be. “The Horizon Report, at its worst, is future telling or a ouija board and at best, it’s a mirror of the industry,” says Thomas. “It works less as an early-warning system for people who don’t know what’s going on, and more of a lens into what the [academic technology] community is interested in.”
Aware of its fortune-telling limitations, Thomas still thinks “it’s a miracle the Horizon Report is coming out this year.” He adds: “It’s unfortunate what happened to NMC, but the fact that Educause picked it up and is carrying it forward is awesome. Every industry should have some sort of community focal point.”
Meanwhile, at least one new effort has emerged to offer an alternative to the Horizon Report. That effort, called FOEcast, was first proposed by Bryan Alexander, a consultant and self-described “futurist” of edtech who served as one of the expert panelists on several Horizon Reports, including the 2018 edition.
Barajas-Murphy, of Educause, welcomes others to join in. As she put it: “There is plenty of room in the space of forecasting for many voices.”
The 2018 ‘Horizon Report’ Is Late. But It Almost Never Emerged. published first on https://medium.com/@GetNewDLBusiness
0 notes
Link
Linux distros: love, openwashing & the thousand yard stare http://ift.tt/2EcjD1n
0 notes