#dataprep
Explore tagged Tumblr posts
Text
Maximizing Data Potential: The Power of Data Preparation Tools
Data preparation tools play a pivotal role in the realm of big data, catering to structured, unstructured, and semi-structured data environments. These tools come equipped with pre-built functionalities that effectively automate and streamline recurring processes. With collaborative interfaces for report generation and model construction, they ensure seamless operations in data management. Their primary objective is to facilitate the migration of top-quality data for analysis while promptly flagging any instances of data duplication, empowering users to take necessary corrective measures.
Key vendors of data analysis tools offer a plethora of capabilities, ranging from consolidating diverse data sources into cohesive datasets to employing AI-driven mechanisms for data and field identification within multi-structured documents. Automated extraction and classification of data, along with quality assessment, data discovery, and data lineage functionalities, are integral features provided by these leading tools. Moreover, they excel in rectifying imbalanced datasets by amalgamating internal and external data sources, generating new data fields, and eliminating outliers. The evolution of data preparation software is evident in the expansion towards cloud-based solutions and the augmentation of their capabilities to align with DataOps principles, facilitating the automation of data pipeline construction for business intelligence (BI) and analytics purposes.
Quadrant Knowledge Solutions emphasizes the significance of data preparation tools in enabling organizations to identify, cleanse, and transform raw datasets from diverse sources. These tools empower data professionals to conduct comprehensive analysis and derive valuable insights using machine learning (ML) algorithms and analytics tools. By streamlining processes such as data cleansing, validation, and transformation without necessitating human intervention or coding expertise, these tools expedite decision-making processes for businesses. They enable users to devote more time to data mining and analysis, thereby enhancing overall operational efficiency.
Prominent vendors in the data preparation software market include Modak Analytics, Oracle, Precisely, Quest, SAP, SAS, Talend, Tamr, TIBCO, and Yellowfin. These industry leaders offer robust solutions that cater to the diverse needs of modern enterprises. Their continuous innovation and commitment to enhancing data management capabilities contribute significantly to driving efficiency and fostering data-driven decision-making across various sectors.
In conclusion, data preparation tools serve as indispensable assets in the realm of big data, offering a wide array of functionalities to streamline data management processes. Their role in facilitating data migration, cleansing, and transformation cannot be overstated, especially in today's data-driven landscape. With advancements in technology and a growing emphasis on automation, these tools are poised to play an even more significant role in empowering organizations to harness the full potential of their data assets.
#DataAnalysisPreparation#DataPrepTools#DataPreparationSoftware#DataPrep#DataPrepSoftware#DataPreparationTools#CloudBasedDataPreparation#DataOps#BI
1 note
·
View note
Text
Data Cleaning Techniques Every Data Scientist Should Know
Data Cleaning Techniques Every Data Scientist Should Know
Data cleaning is a vital step in any data analysis or machine learning workflow. Raw data is often messy, containing inaccuracies, missing values, and inconsistencies that can impact the quality of insights or the performance of models.
Below, we outline essential data cleaning techniques that every data scientist should know.
Handling Missing Values
Missing data is a common issue in datasets and needs to be addressed carefully.
Common approaches include:
Removing Missing Data:
If a row or column has too many missing values, it might be better to drop it entirely.
python
df.dropna(inplace=True)
# Removes rows with any missing values Imputing Missing Data:
Replace missing values with statistical measures like mean, median, or mode.
python
df[‘column’].fillna(df[‘column’].mean(),
inplace=True)
Using Predictive Imputation:
Leverage machine learning models to predict and fill missing values.
2. Removing Duplicates Duplicates can skew your analysis and result in biased models.
Identify and remove them efficiently:
python
df.drop_duplicates(inplace=True)
3. Handling Outliers
Outliers can distort your analysis and lead to misleading conclusions.
Techniques to handle them include:
Visualization: Use boxplots or scatter plots to identify outliers.
Clipping: Cap values that exceed a specific threshold.
python
df[‘column’] = df[‘column’].clip(lower=lower_limit, upper=upper_limit)
Transformation: Apply logarithmic or other transformations to normalize data.
4. Standardizing and Normalizing
Data To ensure consistency, particularly for machine learning algorithms, data should often be standardized or normalized:
Standardization: Converts data to a mean of 0 and a standard deviation of
python from sklearn.preprocessing
import StandardScaler scaler = StandardScaler()
df_scaled = scaler.fit_transform(df)
Normalization:
Scales values to a range between 0 and 1.
pythonfrom sklearn.preprocessing
import MinMaxScaler scaler = MinMaxScaler()
df_normalized = scaler.fit_transform(df)
5. Fixing Structural Errors
Structural errors include inconsistent naming conventions, typos, or incorrect data types.
Correct these issues by: Renaming columns for uniformity:
python df.rename(columns={‘OldName’: ‘NewName’}, inplace=True)
Correcting data types:
python
df[‘column’] = df[‘column’].astype(‘int’)
6. Encoding Categorical Data
Many algorithms require numeric input, so categorical variables must be encoded:
One-Hot Encoding:
python
pd.get_dummies(df, columns=[‘categorical_column’], drop_first=True)
Label Encoding:
python
from sklearn.preprocessing
import LabelEncoder encoder = LabelEncoder()
df[‘column’] = encoder.fit_transform(df[‘column’])
7. Addressing Multicollinearity
Highly correlated features can confuse models.
Use correlation matrices or Variance Inflation Factor (VIF) to identify and reduce multicollinearity.
8. Scaling Large Datasets For datasets with varying scales, scaling ensures all features contribute equally to the model:
python
from sklearn.preprocessing
import StandardScaler scaler = StandardScaler()
df_scaled = scaler.fit_transform(df)
Tools for Data Cleaning
Python Libraries:
Pandas, NumPy, OpenRefine Automation:
Libraries like dataprep or pyjanitor streamline the cleaning process.
Visual Inspection:
Tools like Tableau and Power BI help spot inconsistencies visually. Conclusion Data cleaning is the foundation of accurate data analysis and successful machine learning projects.
Mastering these techniques ensures that your data is reliable, interpretable, and actionable.
As a data scientist, developing an efficient data cleaning workflow is an investment in producing quality insights and impactful results.
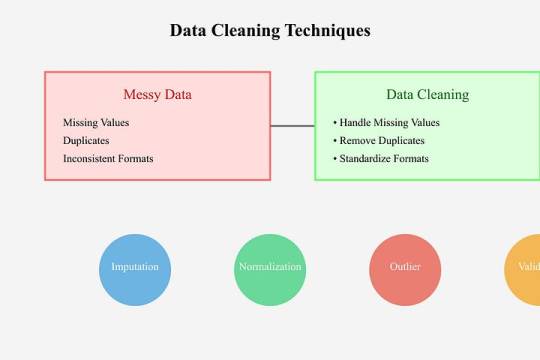
0 notes
Text
Dataset Splitting: Mastering Machine Learning Data Preparation
MachineLearning tip: Master the art of splitting datasets! Learn why it's crucial, how to implement train_test_split, and verify your results. Perfect for #DataScience beginners and pros alike. Boost your ML skills now! #AIEducation #DataPrep
Dataset splitting is a crucial step in machine learning data preparation. By dividing your data into training and testing sets, you ensure your models can generalize well to unseen information. This blog post will guide you through the process of splitting datasets, with a focus on financial data like Tesla’s stock prices. We’ll explore the importance of this technique and provide practical…
0 notes
Text
Practical Python in Power BI: Cleaning constituent data using dataprep.ai
Power BI is a powerful tool. In my consulting work I utilize Power Query for Power BI to transform and prepare constituent data for system migrations. One recent breakthrough in regards to making that even more powerful and efficient was the implementation of Python scripting and the dataprep library.
The following articles were very helpful for figuring out how to do this:
How to Use Python in Power BI - freeCodeCamp
Run Python scripts in Power BI Desktop - Microsoft
There's a major discrepancy between those articles - the freeCodeCamp article provides instructions on how to use a Python environment managed via Anaconda in Power BI; whereas Microsoft's documentation warns that Python distributions requiring an extra step to prepare the environment, such as Conda, might fail to run. They advise to instead use the official Python distribution from python.org.
I've tried both, and as far as I can tell both methods seem to work for this purpose. When installing the official Python distribution, the only pre-packaged installer available is for the current version (currently 3.11.4) which requires a little bit of dataprep debugging post-install to get it working. Anaconda makes it easier to install prior Python versions and to switch between multiple Python environments (I successfully tested this in a Python 3.9 installation running in Anaconda). The following instructions are written for the former method though, using the latest version of Python installed via their Windows executable per Microsoft's recommendation.
To conceptualize how Power BI works with Python, it's important to understand them as entirely separate systems. For the purpose of data transformation, a Power Query Python scripting step loads the previous query step into a pandas dataframe for Python to execute, then loads the output of that back to the next query step.
So with that context, the way we'll approach this install is like so:
Set up a local Python development environment
Install the Dataprep library within that
Utilize a test script to debug and verify that the Python environment is working as expected
Configure Power BI to tap into the Python environment
1. Set up a local Python development environment
The first step is easy, navigate to python.org/downloads, click the Download button, and execute the installer keeping all the default settings.
Once you have Python installed, then open a command prompt and run the following commands:
py -m pip install pandas
py -m pip install matplotlib
After installing these two libraries, you've now got the basics set to use Python in Power BI.
2. Install the Dataprep library
Installing the Dataprep library comes next, and to do that you need Microsoft Visual C++ 14.0 installed as a prerequisite. Navigate on over to the Microsoft Visual Studio downloads page and download the free Community version installer.
Launch the Visual Studio installer, and before you click the install button select the box to install the Python development workload, then also check the box to install optional Python native development tools. Then click install and go get yourself a cup of coffee - it's a large download that'll take a few minutes.
After the Visual Studio installation completes, then head back to your command prompt and run the following command to install Dataprep:
py -m pip install dataprep
3. Utilize a test script to debug and validate the Python environment
With the local Python development environment and Dataprep installed, you can try to execute this test script by running the following command in your command prompt window:
py "C:\{path to script}\Test python pandas script.py"
In practice this script will fail if you try to run it using Python 3.11 (it might work in Python 3.9 via Anaconda). It seems that the reason the script fails is because of a couple of minor incompatibilities in the latest versions of a couple packages used by Dataprep. They're easily debugged and fixed though:
The first error message reads: C:\Users\yourname\AppData\Local\Programs\Python\Python311\Lib\site-packages\dask\dataframe\utils.py:367: FutureWarning: pandas.Int64Index is deprecated and will be removed from pandas in a future version. Use pandas.Index with the appropriate dtype instead.
To fix this error, simply navigate to that file location in Windows Explorer, open the utils.py file, and comment out line 367 by adding a pound sign at the beginning. While you're in there, also comment out lines 409-410 which might produce another error because they reference that function from line 367.
After making that adjustment, if you return to the command line and try to execute the test script you'll encounter another error message. This time it reads: cannot import name 'soft_unicode' from 'markupsafe'
Googling that message turns up a lot of discussion threads from people who encountered the same problem, the upshot of which is that the soft_unicode function was deprecated in markupsafe as of version 2.1.0, and the fix is a simple matter of downgrading that package by running this command in your command line window: py -m pip install markupsafe==2.0.1
After those adjustments have been made, you should be able to run the test script in your command line window and see this successful result:
4. Configure Power BI to tap into the Python environment
You're so close! Now that you have Dataprep working in Python on your local machine, it's time to configure Power BI to leverage it.
In Power BI Desktop - Options - Python scripting, ensure that your Python installation directory is selected as the home directory. Note that if you manage multiple environments via Anaconda, this is where you would instead select Other and paste an environment file path.
Now in Power Query, select Transform - Run Python Script to add a step to your query. The script used here differs in a couple key ways from the test Python script run via your command prompt:
omit import pandas and import numpy
instead of defining df as your pandas dataframe, use the predefined dataset dataframe
My final script, pasted below, leverages the phone and email cleanup functions in Dataprep, as well as leveraging Python to calculate when a proper case cleanup is needed in a slightly more efficient manner than my previous PBI steps to clean that up.
Scripts
Power BI Python script syntax
# 'dataset' holds the input data for this script dataset['FirstName Lower'] = dataset['FirstName'] == dataset['FirstName'].str.lower() dataset['FirstName Upper'] = dataset['FirstName'] == dataset['FirstName'].str.upper() dataset['FirstName Proper'] = dataset['FirstName'].str.title() dataset['LastName Lower'] = dataset['LastName'] == dataset['LastName'].str.lower() dataset['LastName Upper'] = dataset['LastName'] == dataset['LastName'].str.upper() dataset['LastName Proper'] = dataset['LastName'].str.title() from dataprep.clean import validate_phone dataset['Valid Phone'] = validate_phone(dataset["Phone"]) from dataprep.clean import clean_phone dataset = clean_phone(dataset, "Phone") from dataprep.clean import validate_email dataset['Valid Email'] = validate_phone(dataset["Email"]) from dataprep.clean import clean_email dataset = clean_email(dataset, "Email", remove_whitespace=True, fix_domain=True)
Python test script syntax
import pandas as pd import numpy as np df = pd.DataFrame({ "phone": [ "555-234-5678", "(555) 234-5678", "555.234.5678", "555/234/5678", 15551234567, "(1) 555-234-5678", "+1 (234) 567-8901 x. 1234", "2345678901 extension 1234" ], "email": [ "[email protected]", "[email protected]", "y [email protected]", "[email protected]", "H [email protected]", "hello", np.nan, "NULL" ] }) from dataprep.clean import validate_phone df["valid phone"] = validate_phone(df["phone"]) from dataprep.clean import clean_phone df = clean_phone(df, "phone") from dataprep.clean import validate_email df["valid email"] = validate_phone(df["email"]) from dataprep.clean import clean_email df = clean_email(df, "email", remove_whitespace=True, fix_domain=True) print(df)
0 notes
Text
BigQuery for data analytics in GCP
GCP Taining and Certification, Google BigQuery is a fully managed, serverless data warehousing and analytics platform offered by Google Cloud Platform (GCP). It enables organizations to analyze large datasets quickly and efficiently. Here's an overview in 250 words:
1. Scalable Data Warehousing:
BigQuery can handle petabytes of data, providing a scalable solution for data warehousing and analytics.
2. Serverless and Managed:
It's serverless, meaning users don't need to manage infrastructure, and Google takes care of performance optimization and scaling automatically.
3. SQL Query Language:
BigQuery uses standard SQL for querying data, making it accessible to users familiar with SQL.
4. Real-time Analysis:
It supports real-time analysis with streaming data ingestion, enabling immediate insights from live data sources.
5. Integration with GCP Services:
BigQuery seamlessly integrates with other GCP services like Cloud Storage, Dataflow, and Dataprep, allowing data import, transformation, and visualization.
6. Data Security and Governance:
It provides robust security features, including fine-grained access control, encryption at rest and in transit, and audit logging.
7. Cost-Effective Pricing:
Users are billed for the amount of data processed by queries and storage used. BigQuery's pricing model is cost-effective, especially for on-demand, ad-hoc querying.
8. Machine Learning Integration:
It offers integration with Google's AI and machine learning tools, allowing data scientists to train models on BigQuery data.
9. Geospatial Analytics:
BigQuery supports geospatial data types and functions for location-based analysis.
10. Data Export:
Users can export query results to various formats or directly into other GCP services for further analysis or visualization.
11. Data Studio Integration:
Connect BigQuery with Google Data Studio for creating interactive, customizable reports and dashboards.
BigQuery is widely used for various data analytics tasks, including business intelligence, data exploration, machine learning, and real-time data analysis. Its simplicity, scalability, and integration with the broader GCP ecosystem make it a powerful tool for deriving insights from large and complex datasets.
0 notes
Photo
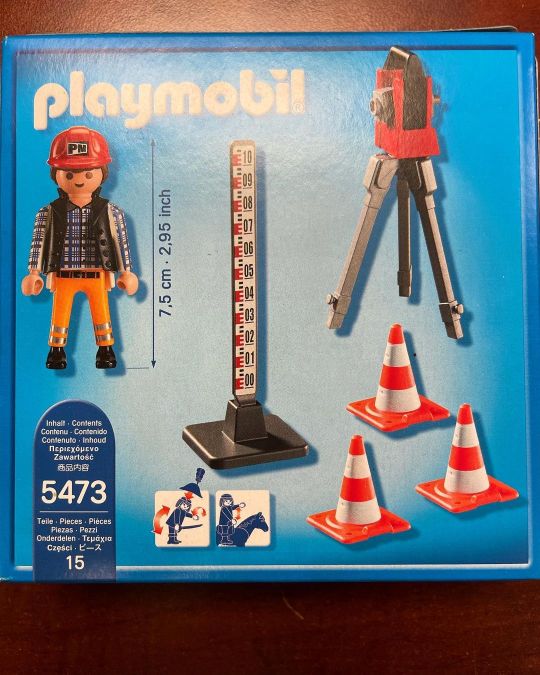
Playmobil surveyor is what I got from my daughter yesterday! So great full she know I love the profession! #survey #takeoff #3dmodeling #surveying #landsurveying #trimble #drafting #surveyinglife #buildingsurveying #lovesurveying #dataprep #earthworks #trimblebusinesscenter #sitemodz #prositeconsulting #surveyworld #geospatial #geodesy #mixedreality #sitemodz #Trimble geospatial #leicageosystems (at Spruce Grove, Alberta) https://www.instagram.com/p/ChH1NSXr-2I/?igshid=NGJjMDIxMWI=
#survey#takeoff#3dmodeling#surveying#landsurveying#trimble#drafting#surveyinglife#buildingsurveying#lovesurveying#dataprep#earthworks#trimblebusinesscenter#sitemodz#prositeconsulting#surveyworld#geospatial#geodesy#mixedreality#leicageosystems
5 notes
·
View notes
Photo
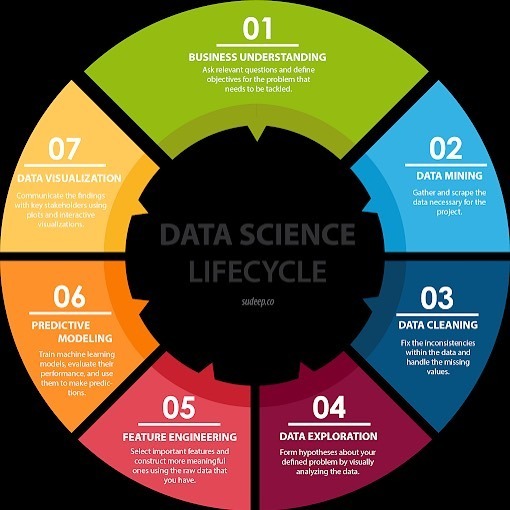
Interesting piece, the #DataScience Lifecycle #infographic, read more at: datastandard.io —————— #BigData #DataScientists #AI #MachineLearning #DataLiteracy #DataMining #DataClearning #DataPrep #FeatureEngineering #PredictiveModeling #DataViz #DataStorytelling #tensorflow #googleai #nvidia #datascience (at Mambo Microsystems Ltd) https://www.instagram.com/p/CAPDnb4BCNr/?igshid=67fhyew7nrmd
#datascience#infographic#bigdata#datascientists#ai#machinelearning#dataliteracy#datamining#dataclearning#dataprep#featureengineering#predictivemodeling#dataviz#datastorytelling#tensorflow#googleai#nvidia
0 notes
Link
Doppelt so viele Händler und Produkte online nehmen. Onedot hilft beim Onboarding. Erfahren Sie mehr.
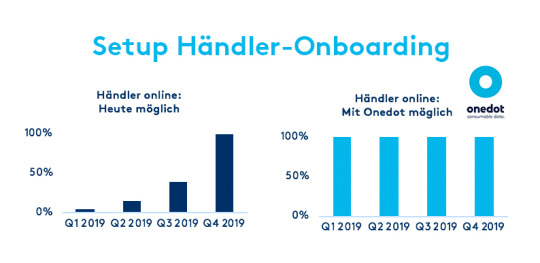
#onedot#consumabledata#ai#artificialintelligence#ml#dataprep#pdm#pim#erp#produktdaten#zukunft#datacleaning
0 notes
Text
Tableau unveils high-scale Hyper engine, previews self-service data-prep and 'smart' capabilities
Video: The data ownership land grab is on Tableau Software is the Apple of the analytics market, with a huge fan base and enthusiastic customers who are willing to stand in long lines for a glimpse at what’s next. Last week’s Tableau Conference in Las Vegas proved that once again with record attendance of more than 14,000. The Tableau fan boys and fan girls were not disappointed, as the company…
View On WordPress
#&039smart&039#Capabilities#Dataprep#Engine#highscale#Hyper#Previews#selfservice#Tableau#unveils
0 notes
Text
RT IBMAnalytics "RT Trifacta: Announcing our partnership with IBM and the launch of a new jointly developed data preparation tool – IBM InfoSphere Advanced Data Preparation! See why IBM believes #dataprep is critical to #DataOps & #AI in a_adam_wilson blog: …
RT IBMAnalytics "RT Trifacta: Announcing our partnership with IBM and the launch of a new jointly developed data preparation tool – IBM InfoSphere Advanced Data Preparation! See why IBM believes #dataprep is critical to #DataOps & #AI in a_adam_wilson blog: …
— Ross Radev (@Ross_Radev) June 28, 2019
from Twitter https://twitter.com/Ross_Radev
2 notes
·
View notes
Photo

KirkDBorne https://twitter.com/KirkDBorne/status/1581817494142332928 https://t.co/mUCO99QqB6 October 17, 2022 at 10:20AM
Excellent book from @PacktPublishing now available >> "Hands-On Data Preprocessing in #Python" at https://t.co/mUCO99QqB6 by @JafariRoy ————— #BigData #Analytics #DataScience #AI #MachineLearning #DataScientists #DataPrep #DataWranging #DataLiteracy #100DaysOfCode https://t.co/xNJPB3SoTN
— Kirk Borne (@KirkDBorne) Oct 17, 2022
0 notes
Text
Bookmark: Power Query like ETL Tools
Why Power Query?
Power Query enables easy -
Pivoting
Merges
Filtering
Grouping
Splitting
Replacing
An advantage of Power Query is that it enables previews, that is not usually available with other ETL tools.
Alternatives to Power Query
Knime
Alteryx
Great tool but big bump in pricing from desktop to servers; same goes for the automation add-on
ADF or Azure Synapse
Has good PQ integration
Also check out “Azure Data Factory”; but you need to have the data in Azure PAAS.
Other Tools
Pentaho
Apache hop
Matillion ETL
Sisense
dbt
Domo
Quicktable
Zoho Dataprep
🔗 Source
0 notes
Text
Challenges in data preparation and how Zoho DataPrep helps
Challenges in data preparation and how Zoho DataPrep helps
With the rising emphasis on data-driven models and algorithms in business, large volumes of data are being generated—but rarely put to proper use. This is primarily due to challenges in understanding data, high costs involved in managing data, low quality and inaccurate data, data compliance issues, the need for complex systems to manage and cleanse data, and more. What is data…
View On WordPress
0 notes
Photo
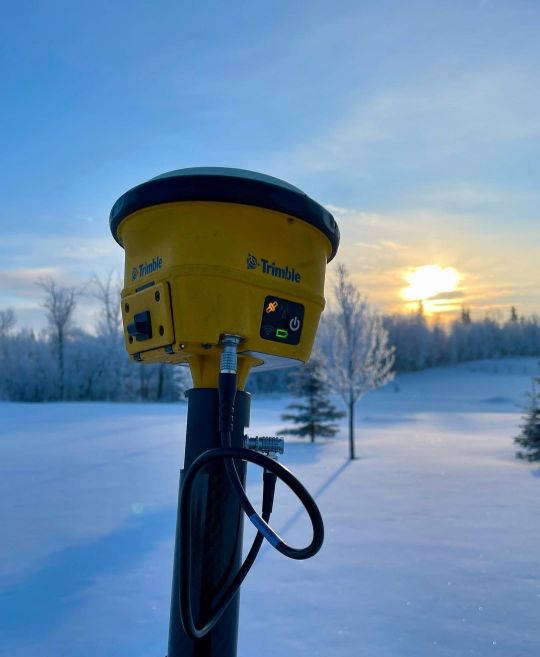
TGIF last one of 2022 ! Beautifully day outside, just a bit chilly ! Everybody have a great day! SPS985 in winter. #survey #takeoff #3dmodeling #surveying #landsurveying #trimble #drafting #surveyinglife #buildingsurveying #lovesurveying #dataprep #earthworks #trimblebusinesscenter #prositeconsulting #surveyworld #geospatial #geodesy #mixedreality #sitemodz #Trimblegeospatial #leicageosystems #gps #tgif #2022 (at Spruce Grove, Alberta) https://www.instagram.com/p/CmzL6qVp6Bb/?igshid=NGJjMDIxMWI=
#survey#takeoff#3dmodeling#surveying#landsurveying#trimble#drafting#surveyinglife#buildingsurveying#lovesurveying#dataprep#earthworks#trimblebusinesscenter#prositeconsulting#surveyworld#geospatial#geodesy#mixedreality#sitemodz#trimblegeospatial#leicageosystems#gps#tgif#2022
0 notes
Link
The core of Apache Spark is not only the cluster-computing framework, but also the amazing Spark community that shares, teaches, and learns from each other. Yesterday, at the Impact Hub Zürich, Philipp Brunenberg talked about Spark: onedot.com/spark
#philippbrunenberg #apachespark #spark #zürich #machinelearning #structuredstreaming #code #impacthub #viadukt #sparktechnical #corespark #shuffle #bigdata #technicaldeep-dive #onedot
0 notes
Text
Google launches Cloud Dataprep in public beta to help companies clean their data before analysis
Google launches Cloud Dataprep in public beta to help companies clean their data before analysis
At its Google Cloud Next conference in San Francisco back in March, Google unveiled Cloud Dataprep, a service for companies to clean their structured and unstructured data sets for analysis in Google’s BigQuery, for example, or even for use in training machine learning models. Over the past six months, Cloud Dataprep has been in private beta, but Google is now officially graduating the service…
View On WordPress
0 notes