#data engineer jobs
Explore tagged Tumblr posts
Text
Data Engineering Interview Questions and Answers
Summary: Master Data Engineering interview questions & answers. Explore key responsibilities, common topics (Big Data's 4 Vs!), and in-depth explanations. Get interview ready with bonus tips to land your dream Data Engineering job!
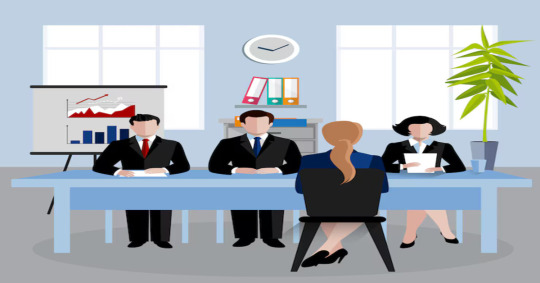
Introduction
The ever-growing volume of data presents exciting opportunities for data engineers. As the architects of data pipelines and custodians of information flow, data engineers are in high demand.
Landing your dream Data Engineering role requires not only technical proficiency but also a clear understanding of the specific challenges and responsibilities involved. This blog equips you with the essential Data Engineering interview questions and answers, helping you showcase your expertise and secure that coveted position.
Understanding the Role of a Data Engineer
Data engineers bridge the gap between raw data and actionable insights. They design, build, and maintain data pipelines that ingest, transform, store, and analyse data. Here are some key responsibilities of a data engineer:
Data Acquisition: Extracting data from various sources like databases, APIs, and log files.
Data Transformation: Cleaning, organizing, and transforming raw data into a usable format for analysis.
Data Warehousing and Storage: Designing and managing data storage solutions like data warehouses and data lakes.
Data Pipelines: Building and maintaining automated processes that move data between systems.
Data Security and Governance: Ensuring data security, access control, and compliance with regulations.
Collaboration: Working closely with data analysts, data scientists, and other stakeholders.
Common Data Engineering Interview Questions
Now that you understand the core responsibilities, let's delve into the most frequently asked Data Engineering interview questions:
What Is the Difference Between A Data Engineer And A Data Scientist?
While both work with data, their roles differ. Data engineers focus on building and maintaining data infrastructure, while data scientists use the prepared data for analysis and building models.
Explain The Concept of Data Warehousing And Data Lakes.
Data warehouses store structured data optimized for querying and reporting. Data lakes store both structured and unstructured data in a raw format, allowing for future exploration.
Can You Describe the ELT (Extract, Load, Transform) And ETL (Extract, Transform, Load) Processes?
Both ELT and ETL are data processing techniques used to move data from various sources to a target system for analysis. While they achieve the same goal, the key difference lies in the order of operations:
ELT (Extract, Load, Transform):
Extract: Data is extracted from its original source (databases, log files, etc.).
Load: The raw data is loaded directly into a data lake, a large storage repository for raw data in various formats.
Transform: Data is transformed and cleaned within the data lake as needed for specific analysis or queries.
ETL (Extract, Transform, Load):
Extract: Similar to ELT, data is extracted from its source.
Transform: The extracted data is cleansed, transformed, and organized into a specific format suitable for analysis before loading.
Load: The transformed data is then loaded into the target system, typically a data warehouse optimized for querying and reporting.
What Are Some Common Data Engineering Tools and Technologies?
Data Engineers wield a powerful toolkit to build and manage data pipelines. Here are some essentials:
Programming Languages: Python (scripting, data manipulation), SQL (database querying).
Big Data Frameworks: Apache Hadoop (distributed storage & processing), Apache Spark (in-memory processing for speed).
Data Streaming: Apache Kafka (real-time data pipelines).
Cloud Platforms: AWS, GCP, Azure (offer data storage, processing, and analytics services).
Data Warehousing: Tools for designing and managing data warehouses (e.g., Redshift, Snowflake).
Explain How You Would Handle a Situation Where A Data Pipeline Fails?
Data pipeline failures are inevitable, but a calm and structured approach can minimize downtime. Here's the key:
Detect & Investigate: Utilize monitoring tools and logs to pinpoint the failure stage and root cause (data issue, code bug, etc.).
Fix & Recover: Implement a solution (data cleaning, code fix, etc.), potentially recover lost data if needed, and thoroughly test the fix.
Communicate & Learn: Keep stakeholders informed and document the incident, including the cause, solution, and lessons learned to prevent future occurrences.
Bonus Tips: Automate retries for specific failures, use version control for code, and integrate data quality checks to prevent issues before they arise.
By following these steps, you can efficiently troubleshoot data pipeline failures and ensure the smooth flow of data for your critical analysis needs.
Detailed Answers and Explanations
Here are some in-depth responses to common Data Engineering interview questions:
Explain The Four Vs of Big Data (Volume, Velocity, Variety, And Veracity).
Volume: The massive amount of data generated today.
Velocity: The speed at which data is created and needs to be processed.
Variety: The diverse types of data, including structured, semi-structured, and unstructured.
Veracity: The accuracy and trustworthiness of the data.
Describe Your Experience with Designing and Developing Data Pipelines.
Explain the specific tools and technologies you've used, the stages involved in your data pipelines (e.g., data ingestion, transformation, storage), and the challenges you faced while designing and implementing them.
How Do You Handle Data Security and Privacy Concerns Within a Data Engineering Project?
Discuss security measures like access control, data encryption, and anonymization techniques you've implemented. Highlight your understanding of relevant data privacy regulations like GDPR (General Data Protection Regulation).
What Are Some Strategies for Optimising Data Pipelines for Performance?
Explain techniques like data partitioning, caching, and using efficient data structures to improve the speed and efficiency of your data pipelines.
Can You Walk us Through a Specific Data Engineering Project You've Worked On?
This is your opportunity to showcase your problem-solving skills and technical expertise. Describe the project goals, the challenges you encountered, the technologies used, and the impact of your work.
Tips for Acing Your Data Engineering Interview
Acing the Data Engineering interview goes beyond technical skills. Here, we unveil powerful tips to boost your confidence, showcase your passion, and leave a lasting impression on recruiters, ensuring you land your dream Data Engineering role!
Practice your answers: Prepare for common questions and rehearse your responses to ensure clarity and conciseness.
Highlight your projects: Showcase your technical skills by discussing real-world Data Engineering projects you've undertaken.
Demonstrate your problem-solving skills: Be prepared to walk through a Data Engineering problem and discuss potential solutions.
Ask insightful questions: Show your genuine interest in the role and the company by asking thoughtful questions about the team, projects, and Data Engineering challenges they face.
Be confident and enthusiastic: Project your passion for Data Engineering and your eagerness to learn and contribute.
Dress professionally: Make a positive first impression with appropriate attire that reflects the company culture.
Follow up: Send a thank-you email to the interviewer(s) reiterating your interest in the position.
Conclusion
Data Engineering is a dynamic and rewarding field. By understanding the role, preparing for common interview questions, and showcasing your skills and passion, you'll be well on your way to landing your dream Data Engineering job.
Remember, the journey to becoming a successful data engineer is a continuous learning process. Embrace challenges, stay updated with the latest technologies, and keep pushing the boundaries of what's possible with data.
#Data Engineering Interview Questions and Answers#data engineering interview#data engineering#engineering#data science#data modeling#data engineer#data engineering career#data engineer interview questions#how to become a data engineer#data engineer jobs
0 notes
Text
Apply Now Automotive Industry Domain careers | KPIT Jobs
Apply for these profiles, Autosar Experts, Data Engineer, C/C++ Simulation Expert, Functional Safety Experts, and RUST-Android AAOS Developer. Shape the future of the automotive and mobility industry with industry leaders. Contribute to technology projects that embrace diverse software skills, playing a crucial role in building a cleaner, smarter, and safer world. Reimagine your career with cutting-edge technologies, best-in-class training, global impact opportunities, and purpose-led work.
#Jobs#job seekers#apply for Jobs#kpit jobs#automotive and mobility industry jobs#automotive industry jobs#automotive domain jobs#Autosar Experts jobs#Data Engineer jobs#C/C++ Simulation Expert#Functional Safety Experts#RUST-Android AAOS Developer
0 notes
Text
Imagine working in Engineering on the Enterprise-D and you're hovering by the door of the Chief Engineer's office, waiting to ask him a question bc the Operations Officer is down here AGAIN and has been talking about a dream he had for the past 20 minutes.
#I know its sometimes implied that Data is like working a shift in Engineering#which makes NO sense#literally WHAT is Data's job supposed to be#but I swear sometimes he's down there JUST bc he came to talk to geordi#star trek next gen#star trek tng#tng#data soong#star trek#geordi la forge
289 notes
·
View notes
Text
behold! the yugioh duel monsters english dub crimes and kill count list, up to episode 78 because that's where dice and I are in the show. I've set the sheet up so that all stats will increment automatically as I add more episodes
some fun facts:
Yami Bakura and Marik are currently tied for most crimes! But Yami Bakura tries to kill people more often (he's made 14 attempts, 6 of which were successful). Marik hasn't successfully killed anyone yet
The Pharaoh has the highest murder success rate (75%!) this number will go up once we watch s0
Joey is the show's punching bag (we already knew this) with 0 crimes committed to 11 crimes committed against him
Kaiba has the most crimes committed against his person (12), the majority of which are murder attempts (6). he also holds the record for the most attempts on his life
despite being a side character that doesn't actively duel, Téa has an alarming lot of crimes committed against her
the most popular crime is attempted murder! followed by actual murder! then it's kidnapping and mind control (shoutout for Marik for single-handedly carrying that stat)
disclaimer: it's a bit hard to separate crimes against Yugi from crimes against the Pharaoh. one could argue most of the crimes committed against Yugi were actually meant for the Pharaoh. it's also hard to gauge how many times people have tried to kill the Pharaoh, because every duel against him could be counted as attempted murder. as such, I'm counting attempted murder as actual, outside of card game murder. hence why Yugi has more attempts against him than the Pharaoh
#yugioh dm#ygo dm#I quit my job to take a gap year due to health reasons#I'm a process engineer#I will DIE if I don't get my daily data analysis enrichment#this is how I spent my first day of retirement
16 notes
·
View notes
Text
not me going to digital tech sector job events and every company rep being like "you can scan our QR code to learn more" and me going "my phone can't do that" and taking a picture of their name instead to google them later like the tech-averse old man that I am
#(phone probably can do it I just refuse to figure out how)#apprenticeship search is going well why do you ask#they don't even have the urls under the QR codes anymore smh#I swear I know how to use a computer and do coding and stuff#I just dislike my phone connecting to the internet and paying with anything other than cash#I'm a normal 26 y/o looking for jobs in data and software stuff I swear#(oh yeah Idr if I posted on here but I'm gonna do a apprenticeship cause media industry can go fuck itself for the time being#I need job that will pay me money that I can rely on so we're becoming like a data analyst or an engineer or smth)
11 notes
·
View notes
Text
i'm sorry if you genuinely think bozzi and leclerc "copied the other driver/engineer's strategy" i canttttttt take you seriously
#do any of you understand how this team shit works. how this pre-race strategy meetings team shit works.#or calling this win 'lucky' be for reallllllll#i dont generally go for the block button but that should be an immediate block#its just fascinating the thought processes required to avoid admitting some of these guys are just good at their jobs#possibly better than others.#there's thoughts in me about the ways fandom 'character analysis' trends intersect with the way people talk about f1 on tumblr/twitter#while just completely forgetting or ignoring not just the competitive sports of it all but the very real ways the teams operate#did you guys know ferrari has a whole 'remote garage' of engineers in italy that tune in every race just to analyse data in real time#and feed back possible strategies to the pit wall that then get discussed and acted on based on drivers feedback?#do you GENUINELY think its just bryan bozzi leaning over fred's shoulder to copy adami's homework#you know ferrari has their very own hannah schmidt? maybe not as good as her but there's a dude in there whose job is 'tell us what to do'#maybe you could learn his name it might be helpful#sorry AND ONE MORE THING#how do you call yourself a leclerc fan and then turn around to call this a lucky win#it required outqualifying his teammate#it required taking advantage of the situation around him to jump lando at la roggia#it required sticking close to both mclarens in dirty air and taking a gamble on the early pit stop#it required 37 LAPS ON HARDS THAT NEVER WENT BELOW OR ABOVE 1:23:000 EXCEPT ONCE#and yes it required required teamwork. as most wins do unless you have a rocket under your ass (and/or don't know how to use it)#the only lucky part was lando once again fumbling the first lap and george taking himself out at turn 1#but you understand he still had to drive the rest of the 52 laps himself right. god#its too early for me to be this mad
3 notes
·
View notes
Text
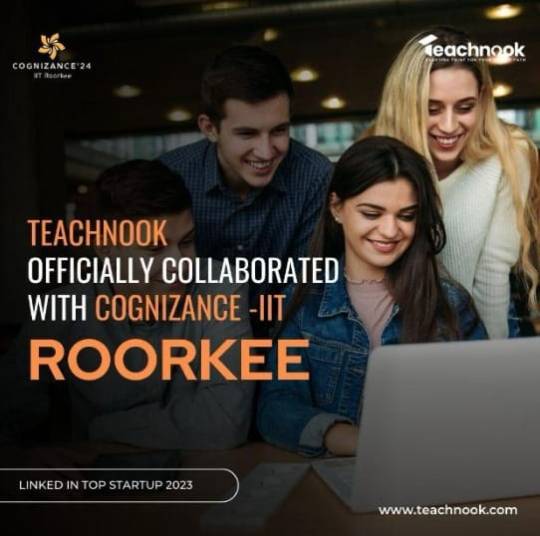
Cognizance IIT Roorkee Internship and Training Program
Registration Link : https://forms.gle/E2cHdnjyzYytKxC39
#engineering#internship#jobs#iit#work from home#student#ai#datascience#data analytics#machinelearning#webde#web development#ui ux development services#graphic design#finance#marketing
3 notes
·
View notes
Text
Listening to one of the engineers in the office talking on the phone and I have never heard this sweet man angry like ever and hearing him get frustrated with a customer on the phone like oh damn... Oh damn! And he isn't even getting that loud, he's keeping pretty calm but I can just TELL he's angry
#and I can *tell* which customer it is just based on the engineers involved#love it when we (my job) make something according to a customer's schematic and then they get mad that it doesn't fit kgjwoe /lh#data log: personal
2 notes
·
View notes
Text
y'all the data scientist job market is so bleak. like 75% of companies are just looking for people to write chat bots 😭
#i'm gonna learn how so i can get a fucking job but idk. i might need to consider a career change in the near future lmao#i'm considering a pivot to software engineering. i'll build a fucking website or something. idk#that was my first Serious Career Plan™ at age 16 so i'm gonna laugh so hard if i end up being a software engineer after all#i've applied to a few jobs that are kinda in between data science and software engineering so we'll see about that lol#m.txt
2 notes
·
View notes
Text
🚀 My Interview Journey: How PrepStat.in Transformed My Placement Experience 🌟

In the vast ocean of placement preparation resources, I found my anchor in PrepStat.in. Navigating through the choppy waters of interviews and tests, PrepStat.in became my lighthouse, guiding me to shore.
When I first started my placement journey, I was overwhelmed by the sheer volume of information available online. There were plenty of resources, but none that offered the clarity and accessibility I needed. That’s when I stumbled upon PrepStat.in — a comprehensive platform designed to help students like me ace their placements.
PrepStat.in isn’t just another educational website; it’s a holistic ecosystem. From detailed tutorials and practice tests to expert tips and real-time problem-solving, PrepStat.in covers all bases. The user-friendly interface and structured content made my study sessions more productive and less stressful.
What truly set PrepStat.in apart was its focus on practical applications. The platform emphasized real-world scenarios, which bridged the gap between theoretical knowledge and practical skills. This approach not only boosted my confidence but also sharpened my problem-solving abilities.
My interview journey was transformative, thanks to PrepStat.in. The mock interviews and personalized feedback helped me refine my responses and improve my overall performance. Each session was a learning experience, bringing me closer to my goal.
Thanks to PrepStat.in, I secured my dream job. The platform’s well-rounded preparation strategy, coupled with its intuitive design, made all the difference. If you’re navigating the placement maze, let PrepStat.in be your guiding star. Trust me, you won’t regret it! 💼🌠
#data analytics#job interview#software#data analysis#software engineering#software development#software testing#software services#developer#applications#development
1 note
·
View note
Text
Data Scientist vs. Data Engineer: Key Differences & Career Insights

Data is everywhere in today's world. Whatever apps we use or whatever websites we visit, everything is based on data. But have you ever thought about who does all this magic behind data? There are two very important roles: Data Scientists and Data Engineers. Though they deal with data, their jobs are completely different.
So, let's break it down in basic terms and go through the main key differences between a Data scientist vs Data engineer to help you decide which path might be right for you.
Difference Between Data Engineer And Data Scientist
In Data engineer vs Data scientist think of a Data Engineer as the person who designs the foundation and building of structures to allow data to flow well. Their job involves making sure data gets collected stored, and kept ready to analyze. A Data Scientist, however, works like a detective to use data to uncover patterns, trends, and insights that help businesses make smart choices.
Educational Requirements
In India, skill sets are more crucial than degree requirements for becoming a successful Data Scientist vs Data Engineer. You may observe many successful Data Scientists and Data Engineers from various educational backgrounds who are excelling in this industry, but if becoming a data scientist or data engineer is your ultimate aim, then studying data analytics courses in college can undoubtedly help you become the greatest.
Educational qualification to become a Data Scientist.
1. You will need a bachelor's degree in Computer Science, Mathematics, Statistics, or Engineering to start a career in data science. To get better opportunities you can also go for a master's and PHD degree.
2. If you have already completed your degree in another stream and then chose data analysis as your career short-duration data analyst course can help you with that.
3. Familiarity with data analysis, SQL, Excel, and software such as Tableau or Power BI are also important to be a good data scientist.
4. A good understanding of statistics and probability is important for analyzing data correctly.
Educational qualification to become a Data Engineer.
1. A bachelor's degree in Computer Science, Information Technology, or Engineering.
2. Strong programming skills in languages like Python, Java, or Scala. You also need to know databases and data processing frameworks.
3. Tools: Experience with cloud platforms (AWS, Google Cloud) and managing data pipelines (such as Apache Kafka or Hadoop) is important for designing efficient data systems.
4. The ability to solve problems and design systems that store and process large amounts of data efficiently.
Career Tips: Getting a Job as a Data Science VS Data Engineering
Here are some tips that can help you get jobs in data science vs data engineering.
Learn the Basics
Start by learning the basics of programming, databases, and math. These are the foundation for both data engineer vs data scientist. Once you understand these, you'll be able to learn more advanced topics easily.
Online Courses
There are many free online courses that teach skills like Python, SQL, and machine learning. Take advantage of these courses to learn at your own pace and build your knowledge step by step.
Hands-On Practice
Theory is important, but nothing beats practical experience. Work on small projects, such as analyzing datasets or building basic machine learning models. Use platforms like Kaggle to practice with real-world datasets.
Internships
Look for internships or part-time jobs related to data. Even a small role can help you gain experience and make your resume stronger in the data engineer vs data scientist vs data analyst field. You’ll also learn a lot by working in a real-world environment.
Stay Updated
The tech world moves quickly, so it’s important to keep learning new tools and techniques related to data engineer vs data analyst. Stay up-to-date to stay competitive in your field.
Networking
Connect with others in the field. Join online communities, attend meetups, or follow experts on social media. Networking can help you learn from others and lead to job opportunities in the data engineer vs data scientist field.
How to Enhance Your Job Application
To enhance your job application in data science vs data engineering, follow these simple tips.
Tailor Your Resume: Customize your resume to match the job you're applying for. Highlight your skills and experience that are most relevant to the role.
Write a Strong Cover Letter: Write a short, personalized cover letter and mention why you want the job and why you’re a great fit.
Showcase Your Achievements: Focus on your accomplishments, not just your responsibilities. Use numbers to show your impact if possible.
Keep it short and simple: Avoid big words or complicated sentences. Make everything easy to read and straightforward.
Proofread: Always look for errors. You may look careless to interviewers if one simple mistake occurs.
Be honest: Never exaggerate and write what you can not explain. Employers value honesty most of all.
Comparison Of Salary: Data Engineer VS Data Scientist
Both Data Engineers and Data Scientists have high-paying jobs, but data scientist vs data engineer salaries can vary a bit. In India, the average data science engineer salary is between ₹9,00,000 and ₹22,00,000, depending on their skills like machine learning and analytics, which are in high demand. If you're a senior Data Scientist, you could earn ₹25,00,000 or even more. Additionally, if you're considering a career as a data analyst, the data analyst job salary typically ranges between ₹4,00,000 and ₹8,00,000, depending on experience and expertise.
On the other hand, a Data Engineer's salary is usually between ₹7,00,000 and ₹15,00,000, depending on the type of work and experience. If you have knowledge of cloud computing and big data tools, your salary can be higher. Also, these are the estimates, it also depends on the company you are working with and the knowledge you have gained.
Career Growth and Pathways
The career growth you can expect as a Data engineer vs Data analyst or scientist.
Career Pathway for Data Engineers.
Starting Out: You might begin as a junior data engineer or a data analyst. This helps you understand how to handle data and learn the tools.
Mid-Level: After gaining some experience, you can become a senior data engineer. At this stage, you’ll take on bigger projects and maybe even manage teams.
Top-Level: With more experience, you could become a lead data engineer or even a chief data officer. These roles involve more decision-making and overseeing larger data systems for the company.
Career Pathway for Data Scientists.
Starting Out: Most people start as junior data scientists or data analysts. This is where you learn how to work with data and do basic analysis.
Mid-Level: As you gain experience, you can become a senior data scientist. You’ll work on bigger problems, analyze more complex data, and might even guide junior team members.
Top-Level: At this level, you can become a lead data scientist or a machine learning engineer. These positions involve using advanced techniques to solve difficult problems and sometimes creating new tools for analysis.
Conclusion
If you're looking to master Python, Analytics Shiksha is the perfect choice. Their Super30 Analytics course, part of their data analytics courses online, offers comprehensive training in data analysis and job preparation. Don’t miss out—secure your spot today, as only 30 seats are available!
The opportunities in both fields are immense, and with the right skills, you can make your mark in the data world.
Be sure to enroll and reserve your spot in our Super30 data analytics course to advance your skills. This program is built around problem-solving techniques and allows you to work with real data.
Frequently Asked Questions: Data Engineer vs. Data Scientist
Can Data Scientists Transition with Data Analytics Courses to Become Data Engineers?
Yes, by increasing knowledge of coding, databases, and system design through data analytics courses, a data scientist can easily become a data engineer.
Which is Better: Data Scientist Data Engineer vs Data Scientist?
It depends on what you enjoy doing to solve problems and make predictions or to build the infrastructure and tools to store and process data. If you love analyzing data and creating models, a data scientist role might be better. If you prefer working on technology and systems, a data engineer job could be the right fit for you.
What is The Difference Between Data Engineer and Data Scientist
A data engineer works on systems that collect, store, and process data. They make sure the data is clean and ready for analysis. A data scientist analyzes the data, creates models, and discovers insights to help businesses solve problems. In a nutshell, engineers prepare the data, while scientists analyze it. To excel in either role, enrolling in data analytics courses can help you build the necessary skills and expertise.
#data analytics courses#data analytics courses in Delhi#data analytics courses online#data analyst job salary#data science vs data engineering
0 notes
Text
Apply Now Automotive Industry Domain careers | KPIT Jobs
Apply for these profiles, Autosar Experts, Data Engineer, C/C++ Simulation Expert, Functional Safety Experts, and RUST-Android AAOS Developer. Shape the future of the automotive and mobility industry with industry leaders. Contribute to technology projects that embrace diverse software skills, playing a crucial role in building a cleaner, smarter, and safer world. Reimagine your career with cutting-edge technologies, best-in-class training, global impact opportunities, and purpose-led work.
#Jobs#job seekers#apply for Jobs#kpit jobs#automotive and mobility industry jobs#automotive industry jobs#automotive domain jobs#Autosar Experts jobs#Data Engineer jobs#C/C++ Simulation Expert#Functional Safety Experts#RUST-Android AAOS Developer
0 notes
Text
Job - Alert 📚
🌍 Join Our Team as a Software Engineer at GFZ Helmholtz-Zentrum für Geoforschung!
GFZ is looking for a Software Engineer (f_m_x) to enhance the operations of our Satellite Laser Ranging (SLR) station in Potsdam. If you have a background in computer science or software engineering and are passionate about contributing to cutting-edge research in Earth monitoring, we want to hear from you!
📅 Application Deadline: February 23, 2025
🖥️ Interested? Apply now through our online application form via the following Link: https://www.academiceurope.com/job/?id=6728
0 notes
Text
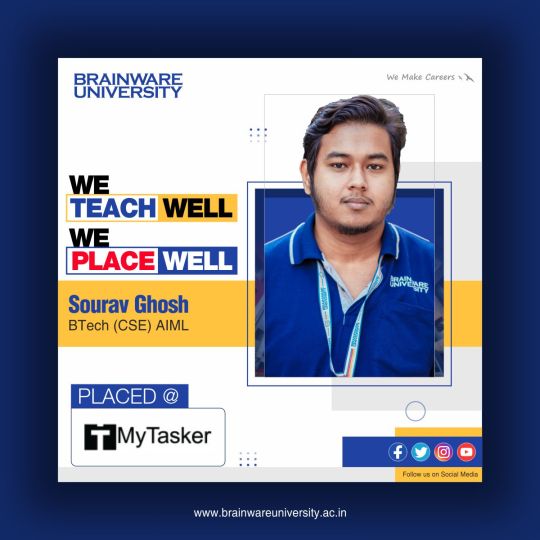
BTech CSE: Your Gateway to High-Demand Tech Careers
Apply now for admission and avail the Early Bird Offer
In the digital age, a BTech in Computer Science & Engineering (CSE) is one of the most sought-after degrees, offering unmatched career opportunities across industries. From software development to artificial intelligence, the possibilities are endless for CSE graduates.
Top Job Opportunities for BTech CSE Graduates
Software Developer: Design and develop innovative applications and systems.
Data Scientist: Analyze big data to drive business decisions.
Cybersecurity Analyst: Safeguard organizations from digital threats.
AI/ML Engineer: Lead the way in artificial intelligence and machine learning.
Cloud Architect: Build and maintain cloud-based infrastructure for global organizations.
Why Choose Brainware University for BTech CSE?
Brainware University provides a cutting-edge curriculum, hands-on training, and access to industry-leading tools. Our dedicated placement cell ensures you’re job-ready, connecting you with top recruiters in tech.
👉 Early Bird Offer: Don’t wait! Enroll now and take the first step toward a high-paying, future-ready career in CSE.
Your journey to becoming a tech leader starts here!
#n the digital age#a BTech in Computer Science & Engineering (CSE) is one of the most sought-after degrees#offering unmatched career opportunities across industries. From software development to artificial intelligence#the possibilities are endless for CSE graduates.#Top Job Opportunities for BTech CSE Graduates#Software Developer: Design and develop innovative applications and systems.#Data Scientist: Analyze big data to drive business decisions.#Cybersecurity Analyst: Safeguard organizations from digital threats.#AI/ML Engineer: Lead the way in artificial intelligence and machine learning.#Cloud Architect: Build and maintain cloud-based infrastructure for global organizations.#Why Choose Brainware University for BTech CSE?#Brainware University provides a cutting-edge curriculum#hands-on training#and access to industry-leading tools. Our dedicated placement cell ensures you’re job-ready#connecting you with top recruiters in tech.#👉 Early Bird Offer: Don’t wait! Enroll now and take the first step toward a high-paying#future-ready career in CSE.#Your journey to becoming a tech leader starts here!#BTechCSE#BrainwareUniversity#TechCareers#SoftwareEngineering#AIJobs#EarlyBirdOffer#DataScience#FutureOfTech#Placements
0 notes
Text
*laughs in still 923 characters in my data set after cleaning up all the duplicate names* 🙃 (down from 975 (unique) old names)
#ship stats#coding#I love csv files they're so satisfying to look at#I'm like THIS 🤏 close to visualising this first version aaaah#I've assigned and completed/corrected (as much as possible) the demographic data#(you need to understand the amount of wikis I had to manually look through for this cleaning process)#now turning everything into csv files again so I can LOOK AT EM BETTER#and then need to look into vis softwares to use o.o#we had bootcamp graduation today also!! 🎉 I am officially a trained data engineer#what will I do with my time now until I have hunted myself a job? hoPEFULLY FINALLY EDIT MY REMAINING MATRIARCHY VIDEOS Q.Q
3 notes
·
View notes
Text

At M.Kumarasamy College of Engineering (MKCE), we emphasize the significance of engineering ethics in shaping responsible engineers. Engineering ethics guide decision-making, foster professionalism, and ensure societal welfare. Our curriculum integrates these principles, teaching students to consider the long-term impacts of their work. Students are trained in truthfulness, transparency, and ethical communication, while also prioritizing public safety and environmental sustainability. We focus on risk management and encourage innovation in sustainable technologies. Our programs also address contemporary challenges like artificial intelligence and cybersecurity, preparing students to tackle these with ethical responsibility. MKCE nurtures future engineers who lead with integrity and contribute to society’s well-being.
To know more : https://mkce.ac.in/blog/engineering-ethics-and-navigating-the-challenges-of-modern-technologies/
#mkce college#top 10 colleges in tn#best engineering college in karur#engineering college in karur#private college#libary#mkce#best engineering college#engineering college#mkce.ac.in#mkce online payment#japanese nat exam date 2025#karur job vacancy#m kumarasamy college of engineering address#m.kumarasamy college of engineering address#MKCE Engineering Curriculum#Engineering Ethics#Collaboration in Engineering Projects#Workplace Ethics in Engineering#Engineering Ethics in AI#Social Responsibility in Engineering#• Technological Innovation and Ethics#• Sustainable Engineering Solutions#• Ethical Engineering Practices#• Data Privacy and Security
0 notes