#create kubernetes cluster
Explore tagged Tumblr posts
Text
What is Argo CD? And When Was Argo CD Established?
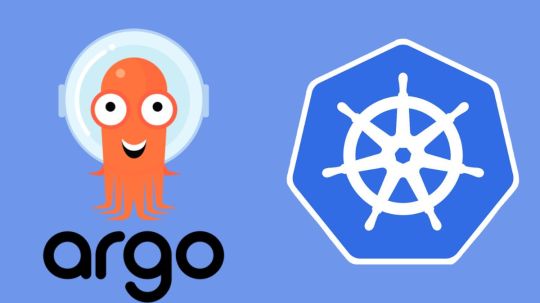
What Is Argo CD?
Argo CD is declarative Kubernetes GitOps continuous delivery.
In DevOps, ArgoCD is a Continuous Delivery (CD) technology that has become well-liked for delivering applications to Kubernetes. It is based on the GitOps deployment methodology.
When was Argo CD Established?
Argo CD was created at Intuit and made publicly available following Applatix’s 2018 acquisition by Intuit. The founding developers of Applatix, Hong Wang, Jesse Suen, and Alexander Matyushentsev, made the Argo project open-source in 2017.
Why Argo CD?
Declarative and version-controlled application definitions, configurations, and environments are ideal. Automated, auditable, and easily comprehensible application deployment and lifecycle management are essential.
Getting Started
Quick Start
kubectl create namespace argocd kubectl apply -n argocd -f https://raw.githubusercontent.com/argoproj/argo-cd/stable/manifests/install.yaml
For some features, more user-friendly documentation is offered. Refer to the upgrade guide if you want to upgrade your Argo CD. Those interested in creating third-party connectors can access developer-oriented resources.
How it works
Argo CD defines the intended application state by employing Git repositories as the source of truth, in accordance with the GitOps pattern. There are various approaches to specify Kubernetes manifests:
Applications for Customization
Helm charts
JSONNET files
Simple YAML/JSON manifest directory
Any custom configuration management tool that is set up as a plugin
The deployment of the intended application states in the designated target settings is automated by Argo CD. Deployments of applications can monitor changes to branches, tags, or pinned to a particular manifest version at a Git commit.
Architecture
The implementation of Argo CD is a Kubernetes controller that continually observes active apps and contrasts their present, live state with the target state (as defined in the Git repository). Out Of Sync is the term used to describe a deployed application whose live state differs from the target state. In addition to reporting and visualizing the differences, Argo CD offers the ability to manually or automatically sync the current state back to the intended goal state. The designated target environments can automatically apply and reflect any changes made to the intended target state in the Git repository.
Components
API Server
The Web UI, CLI, and CI/CD systems use the API, which is exposed by the gRPC/REST server. Its duties include the following:
Status reporting and application management
Launching application functions (such as rollback, sync, and user-defined actions)
Cluster credential management and repository (k8s secrets)
RBAC enforcement
Authentication, and auth delegation to outside identity providers
Git webhook event listener/forwarder
Repository Server
An internal service called the repository server keeps a local cache of the Git repository containing the application manifests. When given the following inputs, it is in charge of creating and returning the Kubernetes manifests:
URL of the repository
Revision (tag, branch, commit)
Path of the application
Template-specific configurations: helm values.yaml, parameters
A Kubernetes controller known as the application controller keeps an eye on all active apps and contrasts their actual, live state with the intended target state as defined in the repository. When it identifies an Out Of Sync application state, it may take remedial action. It is in charge of calling any user-specified hooks for lifecycle events (Sync, PostSync, and PreSync).
Features
Applications are automatically deployed to designated target environments.
Multiple configuration management/templating tools (Kustomize, Helm, Jsonnet, and plain-YAML) are supported.
Capacity to oversee and implement across several clusters
Integration of SSO (OIDC, OAuth2, LDAP, SAML 2.0, Microsoft, LinkedIn, GitHub, GitLab)
RBAC and multi-tenancy authorization policies
Rollback/Roll-anywhere to any Git repository-committed application configuration
Analysis of the application resources’ health state
Automated visualization and detection of configuration drift
Applications can be synced manually or automatically to their desired state.
Web user interface that shows program activity in real time
CLI for CI integration and automation
Integration of webhooks (GitHub, BitBucket, GitLab)
Tokens of access for automation
Hooks for PreSync, Sync, and PostSync to facilitate intricate application rollouts (such as canary and blue/green upgrades)
Application event and API call audit trails
Prometheus measurements
To override helm parameters in Git, use parameter overrides.
Read more on Govindhtech.com
#ArgoCD#CD#GitOps#API#Kubernetes#Git#Argoproject#News#Technews#Technology#Technologynews#Technologytrends#govindhtech
2 notes
·
View notes
Note
Hi!! I'm the anon who sent @/jv the question about how tumblr is handling boops, thanks for answering it in detail i really appreciate it!!! I understand some of it but there's room to learn and I'll look forward to that.
can I ask a follow up question, i don't know if this makes sense but is it possible to use something like k8s containers instead of lots of servers for this purpose?
Hi! Thanks for reaching out.
Yeah my bad, I didn't know what your technical skill level is, so I wasn't writing it in a very approachable level.
The main takeaway is, high scalability has to happen on all levels - feature design, software architecture, networking, hardware, software, and software management.
K8s (an open source software project called Kubernetes, for the normal people) is on the "software management" category. It's like what MS Outlook or Google Calendar is to meetings. It doesn't do the meetings for you, it doesn't give you more time or more meeting rooms, but it gives you a way to say who goes where, and see which rooms are booked.
While I cannot say for Tumblr, I think I've heard they use Kubernetes at least in some parts of the stack, I can't speak for them. I can speak for myself tho! Been using K8s in production since 2015.
Once you want to run more than "1 redis 1 database 1 app" kind of situation, you will likely benefit from using K8s. Whether you have just a small raspberry pi somewhere, a rented consumer-grade server from Hetzner, or a few thousand machines, K8s can likely help you manage software.
So in short: yes, K8s can help with scalability, as long as the overall architecture doesn't fundamentally oppose getting scaled. Meaning, if you would have a central database for a hundred million of your users, and it becomes a bottleneck, then no amount of microservices serving boops, running with or without K8s, will not remove that bottleneck.
"Containers", often called Docker containers (although by default K8s has long stopped using Docker as a runtime, and Docker is mostly just something devs use to build containers) are basically a zip file with some info about what to run on start. K8s cannot be used without containers.
You can run containers without K8s, which might make sense if you're very hardware resource restricted (i.e. a single Raspberry Pi, developer laptop, or single-purpose home server). If you don't need to manage or monitor the cluster (i.e. the set of apps/servers that you run), then you don't benefit a lot from K8s.
Kubernetes is handy because you can basically do this (IRL you'd use some CI/CD pipeline and not do this from console, but conceptually this happens) -
kubectl create -f /stuff/boop_service.yaml kubectl create -f /stuff/boop_ingress.yaml kubectl create -f /stuff/boop_configmap.yaml kubectl create -f /stuff/boop_deploy.yaml
(service is a http endpoint, ingress is how the service will be available from outside of the cluster, configmap is just a bunch of settings and config files, and deploy is the thing that manages the actual stuff running)
At this hypothetical point, Tumblr stuff deploys, updates and tests the boop service before 1st April, generally having some one-click deploy feature in Jenkins or Spinnaker or similar. After it's tested and it's time to bring in the feature to everyone, they'd run
kubectl scale deploy boop --replicas=999
and wait until it downloads and runs the boop server on however many servers. Then they either deploy frontend to use this, or more likely, the frontend code is already live, and just displays boop features based on server time, or some server settings endpoint which just says "ok you can show boop now".
And then when it's over and they disable it in frontend, just again kubectl scale .. --replicas=10 to mop up whichever people haven't refreshed frontend and still are trying to spam boops.
This example, of course, assumes that "boop" is a completely separate software package/server, which is about 85/15% chance that it isn't, and more likely it's just one endpoint that they added to their existing server code, and is already running on hundreds of servers. IDK how Tumblr manages the server side code at all, so it's all just guesses.
Hope this was somewhat interesting and maybe even helpful! Feel free to send more asks.
3 notes
·
View notes
Text
Microsoft Azure Fundamentals AI-900 (Part 5)
Microsoft Azure AI Fundamentals: Explore visual studio tools for machine learning
What is machine learning? A technique that uses math and statistics to create models that predict unknown values
Types of Machine learning
Regression - predict a continuous value, like a price, a sales total, a measure, etc
Classification - determine a class label.
Clustering - determine labels by grouping similar information into label groups
x = features
y = label
Azure Machine Learning Studio
You can use the workspace to develop solutions with the Azure ML service on the web portal or with developer tools
Web portal for ML solutions in Sure
Capabilities for preparing data, training models, publishing and monitoring a service.
First step assign a workspace to a studio.
Compute targets are cloud-based resources which can run model training and data exploration processes
Compute Instances - Development workstations that data scientists can use to work with data and models
Compute Clusters - Scalable clusters of VMs for on demand processing of experiment code
Inference Clusters - Deployment targets for predictive services that use your trained models
Attached Compute - Links to existing Azure compute resources like VMs or Azure data brick clusters
What is Azure Automated Machine Learning
Jobs have multiple settings
Provide information needed to specify your training scripts, compute target and Azure ML environment and run a training job
Understand the AutoML Process
ML model must be trained with existing data
Data scientists spend lots of time pre-processing and selecting data
This is time consuming and often makes inefficient use of expensive compute hardware
In Azure ML data for model training and other operations are encapsulated in a data set.
You create your own dataset.
Classification (predicting categories or classes)
Regression (predicting numeric values)
Time series forecasting (predicting numeric values at a future point in time)
After part of the data is used to train a model, then the rest of the data is used to iteratively test or cross validate the model
The metric is calculated by comparing the actual known label or value with the predicted one
Difference between the actual known and predicted is known as residuals; they indicate amount of error in the model.
Root Mean Squared Error (RMSE) is a performance metric. The smaller the value, the more accurate the model’s prediction is
Normalized root mean squared error (NRMSE) standardizes the metric to be used between models which have different scales.
Shows the frequency of residual value ranges.
Residuals represents variance between predicted and true values that can’t be explained by the model, errors
Most frequently occurring residual values (errors) should be clustered around zero.
You want small errors with fewer errors at the extreme ends of the sale
Should show a diagonal trend where the predicted value correlates closely with the true value
Dotted line shows a perfect model’s performance
The closer to the line of your model’s average predicted value to the dotted, the better.
Services can be deployed as an Azure Container Instance (ACI) or to a Azure Kubernetes Service (AKS) cluster
For production AKS is recommended.
Identify regression machine learning scenarios
Regression is a form of ML
Understands the relationships between variables to predict a desired outcome
Predicts a numeric label or outcome base on variables (features)
Regression is an example of supervised ML
What is Azure Machine Learning designer
Allow you to organize, manage, and reuse complex ML workflows across projects and users
Pipelines start with the dataset you want to use to train the model
Each time you run a pipelines, the context(history) is stored as a pipeline job
Encapsulates one step in a machine learning pipeline.
Like a function in programming
In a pipeline project, you access data assets and components from the Asset Library tab
You can create data assets on the data tab from local files, web files, open at a sets, and a datastore
Data assets appear in the Asset Library
Azure ML job executes a task against a specified compute target.
Jobs allow systematic tracking of your ML experiments and workflows.
Understand steps for regression
To train a regression model, your data set needs to include historic features and known label values.
Use the designer’s Score Model component to generate the predicted class label value
Connect all the components that will run in the experiment
Average difference between predicted and true values
It is based on the same unit as the label
The lower the value is the better the model is predicting
The square root of the mean squared difference between predicted and true values
Metric based on the same unit as the label.
A larger difference indicates greater variance in the individual label errors
Relative metric between 0 and 1 on the square based on the square of the differences between predicted and true values
Closer to 0 means the better the model is performing.
Since the value is relative, it can compare different models with different label units
Relative metric between 0 and 1 on the square based on the absolute of the differences between predicted and true values
Closer to 0 means the better the model is performing.
Can be used to compare models where the labels are in different units
Also known as R-squared
Summarizes how much variance exists between predicted and true values
Closer to 1 means the model is performing better
Remove training components form your data and replace it with a web service inputs and outputs to handle the web requests
It does the same data transformations as the first pipeline for new data
It then uses trained model to infer/predict label values based on the features.
Create a classification model with Azure ML designer
Classification is a form of ML used to predict which category an item belongs to
Like regression this is a supervised ML technique.
Understand steps for classification
True Positive - Model predicts the label and the label is correct
False Positive - Model predicts wrong label and the data has the label
False Negative - Model predicts the wrong label, and the data does have the label
True Negative - Model predicts the label correctly and the data has the label
For multi-class classification, same approach is used. A model with 3 possible results would have a 3x3 matrix.
Diagonal lien of cells were the predicted and actual labels match
Number of cases classified as positive that are actually positive
True positives divided by (true positives + false positives)
Fraction of positive cases correctly identified
Number of true positives divided by (true positives + false negatives)
Overall metric that essentially combines precision and recall
Classification models predict probability for each possible class
For binary classification models, the probability is between 0 and 1
Setting the threshold can define when a value is interpreted as 0 or 1. If its set to 0.5 then 0.5-1.0 is 1 and 0.0-0.4 is 0
Recall also known as True Positive Rate
Has a corresponding False Positive Rate
Plotting these two metrics on a graph for all values between 0 and 1 provides information.
Receiver Operating Characteristic (ROC) is the curve.
In a perfect model, this curve would be high to the top left
Area under the curve (AUC).
Remove training components form your data and replace it with a web service inputs and outputs to handle the web requests
It does the same data transformations as the first pipeline for new data
It then uses trained model to infer/predict label values based on the features.
Create a Clustering model with Azure ML designer
Clustering is used to group similar objects together based on features.
Clustering is an example of unsupervised learning, you train a model to just separate items based on their features.
Understanding steps for clustering
Prebuilt components exist that allow you to clean the data, normalize it, join tables and more
Requires a dataset that includes multiple observations of the items you want to cluster
Requires numeric features that can be used to determine similarities between individual cases
Initializing K coordinates as randomly selected points called centroids in an n-dimensional space (n is the number of dimensions in the feature vectors)
Plotting feature vectors as points in the same space and assigns a value how close they are to the closes centroid
Moving the centroids to the middle points allocated to it (mean distance)
Reassigning to the closes centroids after the move
Repeating the last two steps until tone.
Maximum distances between each point and the centroid of that point’s cluster.
If the value is high it can mean that cluster is widely dispersed.
With the Average Distance to Closer Center, we can determine how spread out the cluster is
Remove training components form your data and replace it with a web service inputs and outputs to handle the web requests
It does the same data transformations as the first pipeline for new data
It then uses trained model to infer/predict label values based on the features.
2 notes
·
View notes
Text
Unlocking the Power of MCC: Simplifying Multi-Cloud Container Management
In the ever-evolving world of cloud technology, managing multi-cloud environments can feel like navigating an intricate maze. Organizations need solutions that not only simplify operations but also maximize efficiency, scalability, and control. Enter MCC (Mirantis Container Cloud)—a powerful platform designed to streamline containerized application management across diverse infrastructures.
What is MCC?
MCC is a comprehensive solution for managing Kubernetes clusters and containerized workloads across multiple cloud providers and on-premises environments. It is purpose-built for flexibility, ensuring that businesses can leverage their existing infrastructure investments while embracing the future of cloud-native applications.
Whether you’re running workloads on public cloud platforms, private clouds, or even bare metal, MCC provides a unified control plane to orchestrate and manage Kubernetes clusters with ease.
Key Features of MCC
Unified Management Across Multi-Cloud Environments: MCC eliminates the silos by offering centralized control over Kubernetes clusters, no matter where they’re deployed—AWS, Azure, Google Cloud, or on-premises.
Self-Service Automation: Developers and operators can create and manage Kubernetes clusters with a few clicks or API calls, speeding up deployment and reducing operational overhead.
Built-In Security and Compliance: With security policies and compliance tools integrated into the platform, MCC ensures that your workloads meet stringent regulatory and organizational requirements.
Scalability with Ease: MCC allows organizations to scale up or down based on demand, ensuring that resources are optimally utilized.
Cluster Lifecycle Management: From provisioning to decommissioning, MCC provides end-to-end lifecycle management for Kubernetes clusters, simplifying the complex processes of updates, backups, and monitoring.
Why Choose MCC?
Simplified Operations: By abstracting away the complexities of managing individual clusters and cloud environments, MCC allows IT teams to focus on innovation rather than infrastructure.
Cost Efficiency: With centralized management and resource optimization, organizations can significantly reduce operational costs and avoid cloud vendor lock-in.
Future-Ready Architecture: As businesses evolve, MCC scales and adapts, making it a long-term solution for organizations on their cloud-native journey.
Real-World Applications
DevOps Empowerment: MCC accelerates DevOps processes by providing developers with self-service cluster provisioning while ensuring that IT maintains control over configurations and security.
Hybrid Cloud Strategies: Organizations can seamlessly manage workloads across on-premises and cloud environments, ensuring high availability and resilience.
Global Deployments: MCC supports distributed architectures, making it an excellent choice for businesses with global operations and diverse infrastructure needs.
Conclusion
MCC is not just a container management tool—it’s a strategic enabler for organizations embracing digital transformation. By providing a unified, efficient, and scalable approach to Kubernetes cluster management, MCC empowers businesses to stay ahead in an increasingly complex and competitive landscape.
If you’re looking to simplify your cloud-native journey, MCC is the bridge between your current infrastructure and your future aspirations. Start exploring its potential today, and see how it transforms your multi-cloud strategy.
For more information visit: https://www.hawkstack.com/
0 notes
Text
Scalable and Reliable High-Availability Clusters with Kubernetes Federation
Building High-Availability Clusters with Kubernetes Federation Introduction In this tutorial, we will explore the concept of building high-availability clusters with Kubernetes Federation. This technology allows us to create a single, unified view of multiple Kubernetes clusters, enabling seamless communication and resource sharing between them. This is crucial for large-scale applications that…
0 notes
Text
Istio Service Mesh Essentials: Simplifying Microservices Management
In today's cloud-native world, microservices architecture has become a standard for building scalable and resilient applications. However, managing the interactions between these microservices introduces challenges such as traffic control, security, and observability. This is where Istio Service Mesh shines.
Istio is a powerful, open-source service mesh platform that addresses these challenges, providing seamless traffic management, enhanced security, and robust observability for microservices. This blog post will dive into the essentials of Istio Service Mesh and explore how it simplifies microservices management, complete with hands-on insights.
What is a Service Mesh?
A service mesh is a dedicated infrastructure layer that facilitates secure, fast, and reliable communication between microservices. It decouples service-to-service communication concerns like routing, load balancing, and security from the application code, enabling developers to focus on business logic.
Istio is one of the most popular service meshes, offering a rich set of features to empower developers and operations teams.
Key Features of Istio Service Mesh
1. Traffic Management
Istio enables dynamic traffic routing and load balancing between services, ensuring optimal performance and reliability. Key traffic management features include:
Intelligent Routing: Use fine-grained traffic control policies for canary deployments, blue-green deployments, and A/B testing.
Load Balancing: Automatically distribute requests across multiple service instances.
Retries and Timeouts: Improve resilience by defining retry policies and request timeouts.
2. Enhanced Security
Security is a cornerstone of Istio, providing built-in features like:
Mutual TLS (mTLS): Encrypt service-to-service communication.
Authentication and Authorization: Define access policies using identity-based and attribute-based rules.
Secure Gateways: Secure ingress and egress traffic with gateways.
3. Observability
Monitoring microservices can be daunting, but Istio offers powerful observability tools:
Telemetry and Metrics: Gain insights into service performance with Prometheus and Grafana integrations.
Distributed Tracing: Trace requests across multiple services using tools like Jaeger or Zipkin.
Service Visualization: Use tools like Kiali to visualize service interactions.
Hands-On with Istio: Setting Up Your Service Mesh
Here’s a quick overview of setting up and using Istio in a Kubernetes environment:
Step 1: Install Istio
Download the Istio CLI (istioctl) and install Istio in your Kubernetes cluster.
Deploy the Istio control plane components, including Pilot, Mixer, and Envoy proxies.
Step 2: Enable Your Services for Istio
Inject Istio's Envoy sidecar proxy into your service pods.
Configure Istio Gateway and VirtualService resources for external traffic management.
Step 3: Define Traffic Rules
Create routing rules for advanced traffic management scenarios.
Test mTLS to secure inter-service communication.
Step 4: Monitor with Observability Tools
Use built-in telemetry to monitor service health.
Visualize the mesh topology with Kiali for better debugging and analysis.
Why Istio Matters for Your Microservices
Istio abstracts complex network-level tasks, enabling your teams to:
Save Time: Automate communication patterns without touching the application code.
Enhance Security: Protect your services with minimal effort.
Improve Performance: Leverage intelligent routing and load balancing.
Gain Insights: Monitor and debug your microservices with ease.
Conclusion
Mastering Istio Service Mesh Essentials opens up new possibilities for managing microservices effectively. By implementing Istio, organizations can ensure their applications are secure, resilient, and performant.
Ready to dive deeper? Explore Istio hands-on labs to experience its features in action. Simplify your microservices management journey with Istio Service Mesh!
Explore More with HawkStack
Interested in modern microservices solutions? HawkStack Technologies offers expert DevOps tools and support, including Istio and other cloud-native services. Reach out today to transform your microservices infrastructure! For more details - www.hawkstack.com
#redhatcourses#information technology#containerorchestration#kubernetes#docker#containersecurity#container#linux#aws#hawkstack#hawkstack technologies
0 notes
Text
GCP DevOps Online Training | GCP DevOps Training in Hyderabad
Mastering Multi-Cloud Strategies with GCP DevOps Training
In today's fast-evolving tech landscape, businesses are increasingly leveraging GCP DevOps Training to streamline workflows and adopt multi-cloud strategies. Google Cloud Platform (GCP) has emerged as a preferred choice for enterprises seeking flexibility, scalability, and innovation in their DevOps pipelines. With the right approach to multi-cloud strategies, organizations can optimize performance, improve resilience, and reduce costs while maintaining a competitive edge.
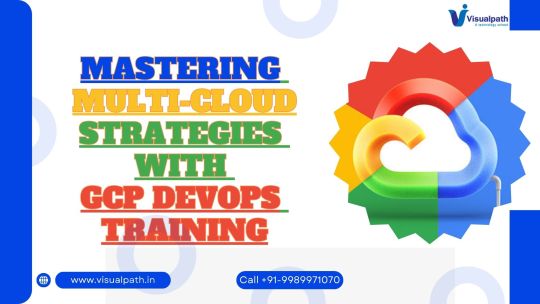
Understanding Multi-Cloud Strategies
A multi-cloud strategy involves the use of services from multiple cloud providers, such as Google Cloud, AWS, and Azure. This approach allows organizations to select the best-in-class services tailored to specific needs, avoid vendor lock-in, and enhance operational resilience. GCP, with its robust tools and seamless DevOps capabilities, plays a pivotal role in helping organizations implement multi-cloud strategies efficiently.
GCP DevOps Training in Hyderabad provides a comprehensive understanding of GCP's role in multi-cloud environments. Through hands-on training, professionals learn how to deploy and manage Kubernetes clusters, implement Infrastructure as Code (IaC), and integrate CI/CD pipelines across multiple platforms. This knowledge is essential for ensuring that applications run smoothly in diverse cloud environments.
Why GCP DevOps is Ideal for Multi-Cloud
Seamless Integration with Other Cloud Platforms GCP supports hybrid and multi-cloud architectures with tools like Anthos, which enable consistent operations across environments. Anthos simplifies managing applications on GCP, AWS, and on-premises infrastructure, making it a cornerstone of multi-cloud strategies.
Unified Monitoring and Management Tools such as Stackdriver (now part of Google Cloud Operations Suite) provide centralized monitoring and logging capabilities, ensuring visibility across all cloud environments. This is a critical aspect covered in GCP DevOps Certification Training, where professionals learn to leverage these tools for effective multi-cloud management.
Enhanced Security Features
Security remains a major concern in multi-cloud setups. GCP addresses this with features like Identity and Access Management (IAM), Shielded VMs, and encryption by default, ensuring robust protection for applications and data. Professionals undergoing GCP DevOps Training in Hyderabad gain hands-on experience in implementing these security features to safeguard multi-cloud deployments
Key Benefits of Multi-Cloud Strategies with GCP DevOps
Cost Optimization: Leverage the pricing advantages of different providers while avoiding over-provisioning.
High Availability: Reduce the risk of downtime by distributing workloads across multiple cloud platforms.
Scalability: Scale applications dynamically across multiple platforms without service interruptions.
Flexibility: Choose the best services from each cloud provider, such as GCP's machine learning tools or AWS's storage solutions.
Through GCP DevOps Certification Training, professionals can master these benefits, enabling businesses to maximize their cloud investments.
Implementing Multi-Cloud Strategies with GCP DevOps
Adopting a multi-cloud approach requires careful planning and the right skill set. The following steps highlight how GCP DevOps supports this process:
Planning and Architecture: Create a strong framework that guarantees seamless interaction between different cloud providers. Training programs, such as GCP DevOps Training, emphasize designing scalable and resilient architectures for multi-cloud environments.
Deployment and Management: Leverage GCP tools like Terraform for IaC and Kubernetes Engine for container orchestration. These tools enable consistent deployments across clouds, reducing complexity.
Continuous Integration and Delivery (CI/CD): Implement CI/CD pipelines with GCP Cloud Build, allowing automated testing and deployments. This ensures rapid and reliable application updates, a core focus in GCP DevOps Training in Hyderabad.
Monitoring and Optimization: Use GCP’s monitoring tools to track performance and identify areas for improvement. Regular monitoring is crucial for maintaining efficiency and avoiding potential issues in multi-cloud setups.
Challenges and Solutions in Multi-Cloud with GCP DevOps
Adopting a multi-cloud strategy is not without challenges. Common issues include interoperability between platforms, increased complexity, and managing security across diverse environments. However, professionals trained through GCP DevOps Certification Training are equipped to tackle these challenges. They learn best practices for configuring seamless integrations, automating processes, and maintaining a unified security posture.
Conclusion
The future of cloud computing lies in multi-cloud strategies, and mastering this approach with GCP DevOps Training is a game-changer for IT professionals and organizations alike. Google Cloud Platform, with its robust tools and innovative features, simplifies the complexities of multi-cloud management.
Whether you're looking to enhance your skills or implement a resilient cloud strategy for your organization, GCP DevOps Training in Hyderabad provides the expertise needed to excel. With proper training and a well-structured plan, businesses can leverage the power of multi-cloud to drive innovation, improve agility, and achieve operational excellence.
Visualpath is the Leading and Best Software Online Training Institute in Hyderabad. Avail complete GCP DevOps Online Training Worldwide. You will get the best course at an affordable cost.
Attend Free Demo
Call on - +91-9989971070.
Visit: https://visualpathblogs.com/
WhatsApp: https://www.whatsapp.com/catalog/919989971070
Visit https://www.visualpath.in/online-gcp-devops-certification-training.html
#GCP DevOps Training#GCP DevOps Training in Hyderabad#GCP DevOps Certification Training#GCP DevOps Online Training#DevOps GCP Online Training in Hyderabad#GCP DevOps Online Training Institute#DevOps on Google Cloud Platform Online Training#DevOps GCP#GCP DevOps#Google#Google Cloud
1 note
·
View note
Text
Assess Application and Cluster Readiness
Review Application Dependencies:
Analyze libraries, JDK versions, frameworks (e.g., Spring Boot, Jakarta EE), and external services (databases, APIs).
Identify any Kubernetes-specific configurations (e.g., ConfigMaps, Secrets, environment variables).
Containerization:
Ensure your Java app is containerized using tools like Docker.
Update the Dockerfile if necessary (e.g., for JDK/JRE updates or changes in base image).
2. Prepare Kubernetes Environment
Target Cluster Validation:
Ensure the target Kubernetes cluster is set up and ready.
Check for compatibility with the current cluster’s API version and resources.
Namespace Setup:
Create equivalent namespaces, RBAC roles, and policies in the target cluster.
Storage:
Verify Persistent Volume Claims (PVCs) and ensure similar storage classes are available.
3. Backup and Export Resources
Kubernetes Resources:
Export all application-related resources using kubectl:bashCopy codekubectl get all -n <namespace> -o yaml > resources.yaml
Persistent Data:
Back up database data or persistent volumes using tools like Velero or rsync.
4. Migrate Application Configurations
ConfigMaps and Secrets:
Export existing configurations:bashCopy codekubectl get configmap -n <namespace> -o yaml > configmap.yaml kubectl get secret -n <namespace> -o yaml > secret.yaml
Reapply them in the new cluster:bashCopy codekubectl apply -f configmap.yaml kubectl apply -f secret.yaml
5. Deploy to Target Cluster
Deploy Application:
Use Helm charts, Kubernetes manifests, or CI/CD pipelines to deploy the Java application to the new cluster.
Update the kubeconfig for the target cluster:bashCopy codekubectl config use-context <target-cluster-context> kubectl apply -f resources.yaml
Persistent Volumes:
Ensure data is restored to the persistent volumes if needed.
6. Test the Migration
Validation:
Ensure the application is running as expected (pods, services, and networking).
Run integration and regression tests.
Connectivity:
Validate external service integrations (e.g., databases, APIs).
Check ingress configurations for public access.
7. Cutover and Cleanup
Switch Traffic:
Update DNS or external load balancer to direct traffic to the new cluster.
Monitor:
Use tools like Prometheus, Grafana, or ELK Stack to monitor application health.
Clean Up Old Environment:
After verifying stability, clean up resources in the old cluster.
Tools to Assist in Migration
Velero: For backup and restore of Kubernetes resources and persistent volumes.
Helm: To manage application deployments and upgrades.
Kubernetes Dashboard: For real-time cluster monitoring.
Kubectl Plugins: To enhance CLI functionality.
If you provide more details about the migration (e.g., specific cloud providers or Kubernetes environments), I can offer more tailored advice!
4o
You said:
Migration of 5000 files per hr fix
ChatGPT said:
ChatGPT
If you're migrating a large number of files (e.g., 5000 files per hour) and encountering performance bottlenecks, you can improve the speed and reliability of your file migration by addressing several key areas. Here's a step-by-step guide to optimize file transfer:
1. Identify and Analyze Bottlenecks
File System Bottlenecks:
Check the source and destination disk I/O performance.
Ensure the files are not locked or being used during migration.
Network Bandwidth:
Measure your available network bandwidth using tools like iperf and ensure it can handle the expected load.
File Metadata Overhead:
Small files can add significant overhead due to metadata operations (e.g., file creation, permissions).
2. Optimize Transfer Mechanisms
Use Parallel Processing:
Transfer multiple files concurrently using multithreaded or parallel tools like:
rsync with --max-size and --parallel options.
GNU Parallel for concurrent file transfers.
bashCopy codefind /source/directory -type f | parallel -j8 rsync -a {} /destination/directory
Use a custom script or a library like Python's concurrent.futures for more control.
Batch File Transfers:
Group files into batches (e.g., tar archives) to reduce the overhead of transferring many small files individually.bashCopy codetar -cvf files.tar /source/files && rsync files.tar /destination Extract the files on the destination:bashCopy codetar -xvf files.tar
3. Ensure Network Optimization
Compression:
Compress files during transfer to reduce data volume:bashCopy codersync -az /source/directory /destination/directory
Increase TCP Window Size:
Tweak TCP settings for large transfers using tools like scp or rsync with buffer options:bashCopy codersync --bwlimit=0 --whole-file /source /destination
Use Faster Protocols:
Use optimized file transfer protocols like Rclone, Aspera, or SFTP for high-speed transfer.
4. Improve Source and Destination Performance
Disk Performance:
Optimize disk reads and writes using SSDs for temporary storage or caches.
Ensure the file system supports efficient file handling (e.g., ext4, XFS).
File System Tuning:
For very large numbers of files, tune parameters like inode_cache to handle metadata efficiently.
5. Error Handling and Retry Logic
Retry Failed Transfers:
Use tools like rsync to retry failed files automatically:bashCopy codersync -avz --partial --progress /source /destination
Logging and Monitoring:
Log all transferred files and
4o
You said:
File trsnsfer to s3 improve
ChatGPT said:
ChatGPT
Improving file transfer to Amazon S3 requires addressing both performance and reliability. Below are strategies and tools to optimize large-scale or high-volume file transfers:
1. Use AWS-Specific Tools
AWS CLI with Parallelism:
Use the --multipart-chunk-size-mb and --parallel flags to upload files in parallel and in chunks:bashCopy codeaws s3 cp /local/path s3://your-bucket-name --recursive --storage-class STANDARD_IA --region <region>
Enable parallel transfers: Update your AWS CLI configuration file (~/.aws/config) to increase concurrency:plaintextCopy code[default] s3 = max_concurrent_requests = 10 max_queue_size = 1000 multipart_threshold = 64MB multipart_chunksize = 64MB
AWS DataSync:
Use AWS DataSync for automated, high-performance, large-scale transfers with minimal manual intervention. DataSync can also manage retry and error handling.
2. Optimize S3 Uploads
Multipart Upload:
Use multipart uploads for files larger than 100 MB to improve transfer speed and reliability.
Example using AWS CLI:bashCopy codeaws s3api create-multipart-upload --bucket your-bucket-name --key file_key
Automate multipart uploads with the AWS SDK or tools like boto3 for Python.
Use the Correct Storage Class:
Choose the appropriate storage class to save costs and improve upload speeds:
STANDARD (default): High performance for frequently accessed data.
INTELLIGENT_TIERING: Automatically optimizes storage costs for infrequently accessed files.
3. Improve Network Performance
Enable Transfer Acceleration:
Use S3 Transfer Acceleration for faster uploads over long distances by leveraging AWS edge locations:bashCopy codeaws s3 cp /local/path s3://your-bucket-name --region <region> --endpoint-url https://<bucket-name>.s3-accelerate.amazonaws.com
Compression:
Compress files before transferring to reduce data size:bashCopy codetar -czvf archive.tar.gz /local/path && aws s3 cp archive.tar.gz s3://your-bucket-name
Optimize Network:
Use AWS Direct Connect or a high-bandwidth internet connection for large-scale transfers.
Monitor latency and bandwidth with tools like iperf.
4. Automate and Parallelize Transfers
Rclone:
Use rclone for high-speed S3-compatible transfers with parallelism and retry capabilities:bashCopy coderclone copy /local/path s3:your-bucket-name --transfers 16 --s3-upload-concurrency 16
GNU Parallel:
Transfer files concurrently by splitting file lists:bashCopy codefind /local/path -type f | parallel -j10 aws s3 cp {} s3://your-bucket-name
5. Error Handling and Retry
Retry Mechanisms:
Use tools or scripts that automatically retry failed uploads.
AWS CLI has built-in retry logic; increase retries if necessary:bashCopy codeaws s3 cp --cli-read-timeout 600 --cli-connect-timeout 600 /local/path s3://your-bucket-name
Chunked Retry:
Break large transfers into smaller, manageable chunks and retry failed chunks only.
6. Monitor and Optimize Transfers
AWS CloudWatch Metrics:
Monitor transfer performance and error rates in real-time.
S3 Inventory:
Use S3 inventory reports to validate uploaded files.
AWS SDKs or Custom Scripts:
Build custom monitoring and retry logic with SDKs like boto3 (Python).
Example Optimized Upload Command
bash
Copy code
aws s3 cp /local/path s3://your-bucket-name --recursive --storage-class STANDARD --region us-east-1 --cli-read-timeout 600 --cli-connect-timeout 600
When to Use Snowball or Snowmobile
For extremely large datasets (terabytes or petabytes), use AWS Snowball or AWS Snowmobile for offline data transfer to S3.
These steps can dramatically improve the efficiency and reliability of your file transfer process to S3. Let me know if you need help implementing any of these!
4o
You said:
Improve fole transfers
ChatGPT said:
ChatGPT
Improving file transfer performance and reliability, whether between servers, local systems, or to cloud storage like Amazon S3, involves a combination of strategies. Here are some best practices for improving file transfers:
1. Optimize the File Transfer Process
Parallelize Transfers
Transfer multiple files concurrently to improve throughput:
Use tools like rsync, scp, or cloud-specific SDKs with multi-threading capabilities.
Example with rsync:bashCopy codersync -azP --info=progress2 --max-size=100M --exclude="*.tmp" /source/ /destination/
Batch Small Files
Small files cause metadata overhead during transfer. Bundle them into archives:bashCopy codetar -czvf archive.tar.gz /source/ Transfer the archive, then extract on the destination:bashCopy codetar -xzvf archive.tar.gz
2. Use High-Performance Transfer Tools
Rclone
Ideal for cloud storage and large-scale transfers with parallelism:bashCopy coderclone copy /source/ remote:bucket-name --transfers=16 --checkers=8
GNU Parallel
Parallelize file uploads/downloads:bashCopy codefind /source/ -type f | parallel -j8 scp {} user@destination:/target/
Multithreaded Tools
Use tools with built-in multithreading, such as bbcp, Aspera, or scp (with parallel scripts).
3. Network Optimization
Compression
Compress files before transfer to reduce the amount of data transmitted:bashCopy codegzip largefile.txt Or combine with the transfer:bashCopy codetar czf - /source/ | ssh user@destination "tar xzf - -C /target/"
Bandwidth Management
Limit bandwidth usage to prevent throttling:
With rsync:bashCopy codersync --bwlimit=1000 -az /source/ /destination/
Use TCP tuning options to optimize network transfer rates:bashCopy codesysctl -w net.ipv4.tcp_window_scaling=1
Use Faster Protocols
Use optimized transfer protocols:
SFTP: Secure but slower for high-volume transfers.
Rsync/SSH: Efficient for incremental and large transfers.
Aspera: High-speed UDP-based protocol.
Enable Transfer Acceleration (Cloud)
For Amazon S3:bashCopy codeaws s3 cp /source/ s3://your-bucket/ --region <region> --endpoint-url https://<bucket-name>.s3-accelerate.amazonaws.com
4. Error Handling and Resilience
Resume Transfers
Use tools that support resuming failed transfers:
Rsync:bashCopy codersync -az --partial /source/ /destination/
AWS CLI:bashCopy codeaws s3 cp --continue /source/ s3://bucket-name/
Retry Logic
Implement retry logic for failed transfers using scripts or tools.
Example using bash:bashCopy codefor i in {1..5}; do scp file user@destination:/target/ && break || sleep 5 done
5. Monitor and Analyze Performance
Real-Time Monitoring
Monitor progress with tools:
Rsync: --progress flag.
SCP: Wrap in pv for progress visualization:bashCopy codetar cf - /source/ | pv | ssh user@destination "tar xf - -C /target/"
Analyze Bottlenecks
Use tools like iperf or nload to check network performance.
Use iotop or dstat to monitor disk I/O on both source and destination.
6. Scale with Advanced Solutions
Distributed File Transfer
Use tools like Globus for efficient distributed data transfers.
Data Transfer Appliances
For extremely large datasets, consider appliances like AWS Snowball or offline methods to ship data physically.
Content Delivery Networks (CDNs)
Use CDNs for faster file distribution to end users.
By combining these strategies—parallelism, compression, protocol optimization, and monitoring—you can significantly enhance file transfer speed and reliability. Let me know if you'd like further customization for a specific use case!
4o
You said:
Threadpool
ChatGPT said:
ChatGPT
A thread pool is a collection of pre-initialized worker threads that stand ready to perform tasks. Instead of creating and destroying threads for each task, a thread pool manages a set of reusable threads, improving performance and resource utilization in multi-threaded applications.
Here’s how to effectively use and optimize thread pools in your application.
Why Use a Thread Pool?
Performance: Reduces the overhead of creating and destroying threads repeatedly.
Resource Management: Controls the number of concurrent threads, preventing system overload.
Scalability: Allows a controlled environment for task execution, making it easier to scale applications.
Key Concepts of Thread Pools
Core Threads: Minimum number of threads that are always kept alive.
**Max Threads
4o
You said:
Improve java spring file transfer
ChatGPT said:
ChatGPT
Improving file transfer in a Java Spring application involves optimizing both the code and the underlying infrastructure. Below are strategies to enhance file transfer performance and reliability.
1. Optimize File Transfer Code
Use Streaming for Large Files
Avoid loading the entire file into memory; instead, use streaming:javaCopy code@PostMapping("/upload") public ResponseEntity<String> uploadFile(@RequestParam("file") MultipartFile file) throws IOException { try (InputStream inputStream = file.getInputStream()) { // Process the input stream directly // Example: Save the file Files.copy(inputStream, Paths.get("target-directory/" + file.getOriginalFilename()), StandardCopyOption.REPLACE_EXISTING); } return ResponseEntity.ok("File uploaded successfully!"); }
Enable Asynchronous File Transfers
Use Spring's @Async annotation for non-blocking file uploads/downloads:javaCopy code@Async public CompletableFuture<String> uploadFileAsync(MultipartFile file) { // File processing logic return CompletableFuture.completedFuture("Upload Complete"); }
Use Chunked Uploads for Large Files
Break large files into smaller chunks for efficient transfer and to handle interruptions gracefully:javaCopy code@PostMapping("/uploadChunk") public ResponseEntity<String> uploadChunk(@RequestParam("chunk") MultipartFile chunk, @RequestParam("fileId") String fileId) { // Append chunk data to the file return ResponseEntity.ok("Chunk uploaded successfully!"); }
2. Optimize for Cloud Storage (e.g., AWS S3, Azure Blob)
Use SDKs for Direct Uploads
Use cloud-specific SDKs (e.g., AWS SDK for S3) to upload files directly to cloud storage instead of saving locally first:javaCopy code@PostMapping("/uploadToS3") public ResponseEntity<String> uploadToS3(@RequestParam("file") MultipartFile file) throws IOException { AmazonS3 s3Client = AmazonS3ClientBuilder.defaultClient(); String bucketName = "your-bucket-name"; s3Client.putObject(bucketName, file.getOriginalFilename(), file.getInputStream(), new ObjectMetadata()); return ResponseEntity.ok("File uploaded to S3!"); }
Enable S3 Transfer Acceleration
Use Transfer Acceleration for faster uploads to Amazon S3:javaCopy codeAmazonS3 s3Client = AmazonS3ClientBuilder.standard() .withAccelerateModeEnabled(true) .build();
3. Optimize File Transfer in REST APIs
Enable Compression
Compress file responses to reduce data size:yamlCopy codespring: servlet: multipart: enabled: true max-file-size: 50MB max-request-size: 50MB gzip: enabled: true min-size: 1024
Use HTTP/2
Switch to HTTP/2 for better concurrency and reduced latency:yamlCopy codeserver: http2: enabled: true
Resume Interrupted Uploads
Use ETag or file metadata to allow resumable file uploads:javaCopy code@PostMapping("/resumeUpload") public ResponseEntity<String> resumeUpload(@RequestParam("file") MultipartFile file, @RequestParam("offset") Long offset) { // Resume logic based on offset return ResponseEntity.ok("Upload resumed!"); }
4. Parallelize File Transfers
Multithreaded File Upload
Use a ThreadPoolExecutor or Spring's TaskExecutor:Copy code
In addition to using @Async, there are other strategies and tools to improve file transfer performance and reliability in a Java Spring application. Here are some approaches:
1. Reactive Programming with Spring WebFlux
For truly non-blocking and asynchronous file transfers, use Spring WebFlux:
Streaming File Upload:javaCopy code@PostMapping(value = "/upload", consumes = MediaType.MULTIPART_FORM_DATA_VALUE) public Mono<Void> uploadFile(Flux<FilePart> filePartFlux) { return filePartFlux.flatMap(filePart -> { Path path = Paths.get("target-directory/" + filePart.filename()); return filePart.transferTo(path); }).then(); }
Streaming File Download:javaCopy code@GetMapping(value = "/download", produces = MediaType.APPLICATION_OCTET_STREAM_VALUE) public Mono<ResponseEntity<Resource>> downloadFile() { Path path = Paths.get("target-directory/large-file.txt"); return Mono.just(ResponseEntity.ok() .header(HttpHeaders.CONTENT_DISPOSITION, "attachment; filename=large-file.txt") .body(new FileSystemResource(path))); }
2. Use CompletableFuture and ExecutorService
Combine CompletableFuture with a custom ExecutorService for better control:javaCopy code@PostMapping("/upload") public CompletableFuture<String> uploadFile(@RequestParam("file") MultipartFile file) { ExecutorService executor = Executors.newFixedThreadPool(4); return CompletableFuture.supplyAsync(() -> { try { // File processing logic Files.copy(file.getInputStream(), Paths.get("target/" + file.getOriginalFilename()), StandardCopyOption.REPLACE_EXISTING); return "File uploaded successfully!"; } catch (IOException e) { throw new RuntimeException("File upload failed!", e); } }, executor); }
3. Apache Kafka for Asynchronous Processing
Use Apache Kafka to decouple file upload from processing:
Producer sends file metadata to Kafka after upload:javaCopy code@PostMapping("/upload") public ResponseEntity<String> uploadFile(@RequestParam("file") MultipartFile file) throws IOException { // Save the file Files.copy(file.getInputStream(), Paths.get("target/" + file.getOriginalFilename())); // Send metadata to Kafka kafkaTemplate.send("file-topic", file.getOriginalFilename()); return ResponseEntity.ok("File uploaded and queued for processing!"); }
Consumer processes files asynchronously:javaCopy code@KafkaListener(topics = "file-topic", groupId = "file-group") public void processFile(String fileName) { // Process the file Path path = Paths.get("target/" + fileName); System.out.println("Processing file: " + path); }
4. Chunked File Transfers
Handle large files by uploading them in chunks:
Frontend sends file chunks with an identifier.
Backend reconstructs the file:javaCopy code@PostMapping("/uploadChunk") public ResponseEntity<String> uploadChunk(@RequestParam("chunk") MultipartFile chunk, @RequestParam("fileId") String fileId, @RequestParam("chunkNumber") int chunkNumber) { Path path = Paths.get("target/" + fileId + "_" + chunkNumber); try { Files.copy(chunk.getInputStream(), path, StandardCopyOption.REPLACE_EXISTING); } catch (IOException e) { return ResponseEntity.status(HttpStatus.INTERNAL_SERVER_ERROR).body("Failed to upload chunk"); } return ResponseEntity.ok("Chunk uploaded successfully!"); }
Reassemble chunks when all are uploaded.
5. WebSockets for Real-Time Progress
Use WebSockets to provide real-time feedback on file transfer progress:javaCopy code@Controller public class WebSocketController { @MessageMapping("/uploadStatus") @SendTo("/topic/progress") public String sendProgress(String status) { return status; } }
Send progress updates from the server while uploading:javaCopy codesimpMessagingTemplate.convertAndSend("/topic/progress", "50% completed");
6. Redis or Database Queues
Use a Redis queue or a database to handle file metadata asynchronously.
Enqueue metadata after upload:javaCopy coderedisTemplate.opsForList().leftPush("fileQueue", fileMetadata);
Process the queue in the background:javaCopy codeString metadata = redisTemplate.opsForList().rightPop("fileQueue");
7. Use MultipartConfig for Large Files
Configure multipart file handling for large files in application.properties:propertiesCopy codespring.servlet.multipart.max-file-size=1GB spring.servlet.multipart.max-request-size=2GB
Custom bean for fine-tuning:javaCopy code@Bean public MultipartConfigElement multipartConfigElement() { MultipartConfigFactory factory = new MultipartConfigFactory(); factory.setMaxFileSize("1GB"); factory.setMaxRequestSize("2GB"); return factory.createMultipartConfig(); }
8. Use AWS S3 for Direct Uploads
Use pre-signed URLs to offload upload work to the client:javaCopy code@GetMapping("/generatePresignedUrl") public ResponseEntity<String> generatePresignedUrl(@RequestParam String fileName) { AmazonS3 s3Client = AmazonS3ClientBuilder.defaultClient(); GeneratePresignedUrlRequest generatePresignedUrlRequest = new GeneratePresignedUrlRequest("bucket-name", fileName) .withMethod(HttpMethod.PUT) .withExpiration(new Date(System.currentTimeMillis() + 3600 * 1000)); URL url = s3Client.generatePresignedUrl(generatePresignedUrlRequest); return ResponseEntity.ok(url.toString()); }
The client uploads directly to S3 using the URL.
9. Optimize File Transfer for High Performance
Compression
Compress files before upload to reduce transfer size:javaCopy codebyte[] compressedData = compress(file.getBytes()); Files.write(Paths.get("compressed-file.gz"), compressedData);
Load Balancing
Use a load balancer (e.g., AWS ALB or NGINX) for scalable upload endpoints.
Retry Logic
Implement retries for failed transfers to improve reliability:javaCopy codeint maxRetries = 3; while (retries < maxRetries) { try { // Attempt file upload break; } catch (IOException e) { retries++; if (retries == maxRetries) { throw e; } } }
These strategies can enhance file transfer performance, scalability, and reliability in Java Spring applications. Let me know if you need code examples for specific use cases!
4o
You said:
S3 relaibility
ChatGPT said:
ChatGPT
Ensuring reliability when transferring files to Amazon S3 involves minimizing failures, handling errors effectively, and ensuring data integrity. Below are best practices and techniques to achieve reliable file transfers to S3:
1. Use Multipart Upload for Large Files
Multipart uploads split large files into smaller parts, upload them in parallel, and reassemble them in S3. This improves reliability and speeds up transfers.
Example Using AWS SDK (Java)
java
Copy code
AmazonS3 s3Client = AmazonS3ClientBuilder.defaultClient(); String bucketName = "your-bucket-name"; String fileName = "large-file.zip"; String keyName = "uploads/" + fileName; File file = new File(fileName); TransferManager tm = TransferManagerBuilder.standard().withS3Client(s3Client).build(); Upload upload = tm.upload(bucketName, keyName, file); try { upload.waitForCompletion(); System.out.println("File uploaded successfully!"); } catch (AmazonClientException e) { System.err.println("Upload failed: " + e.getMessage()); }
2. Use Retry Logic
Add retry mechanisms to handle intermittent network issues or service interruptions.
AWS SDK Retry Settings
The AWS SDK retries failed requests automatically, but you can customize it:
java
Copy code
ClientConfiguration config = new ClientConfiguration(); config.setRetryPolicy(PredefinedRetryPolicies.getDefaultRetryPolicyWithCustomMaxRetries(5)); AmazonS3 s3Client = AmazonS3ClientBuilder.standard() .withClientConfiguration(config) .build();
3. Enable Transfer Acceleration
Transfer Acceleration uses AWS edge locations to improve upload speeds over long distances.
Enable Transfer Acceleration in S3
Enable it in the S3 bucket settings.
Update your client code to use the accelerated endpoint:
java
Copy code
AmazonS3 s3Client = AmazonS3ClientBuilder.standard() .withAccelerateModeEnabled(true) .build();
4. Validate Data Integrity
Use MD5 checksums to ensure the uploaded file matches the original file.
AWS CLI Example
bash
Copy code
aws s3 cp file.txt s3://your-bucket/ --content-md5 <calculated-md5>
Java Example
java
Copy code
String md5 = Base64.getEncoder().encodeToString(DigestUtils.md5(new FileInputStream(file))); ObjectMetadata metadata = new ObjectMetadata(); metadata.setContentMD5(md5); PutObjectRequest request = new PutObjectRequest(bucketName, keyName, new FileInputStream(file), metadata); s3Client.putObject(request);
5. Monitor File Transfers
Use CloudWatch Metrics to monitor S3 request success, failures, and latencies.
Monitor transfer progress with TransferManager in the AWS SDK:
java
Copy code
TransferManager tm = TransferManagerBuilder.standard().withS3Client(s3Client).build(); Upload upload = tm.upload(bucketName, keyName, file); upload.addProgressListener(progressEvent -> { System.out.println("Transferred bytes: " + progressEvent.getBytesTransferred()); }); upload.waitForCompletion();
6. Handle Failures Gracefully
Use Resumable Transfers for interrupted uploads with TransferManager:
java
Copy code
TransferManager tm = TransferManagerBuilder.standard().build(); Upload upload = tm.upload(bucketName, keyName, file); // Add a progress listener upload.addProgressListener((ProgressListener) progressEvent -> { System.out.println("Transferred: " + progressEvent.getBytesTransferred()); }); // Graceful failure handling try { upload.waitForCompletion(); } catch (AmazonClientException e) { System.err.println("Error occurred: " + e.getMessage()); }
7. Use Pre-Signed URLs
Generate pre-signed URLs for reliable, time-limited uploads directly from the client, reducing the load on your server.
Generate Pre-Signed URL
java
Copy code
GeneratePresignedUrlRequest generatePresignedUrlRequest = new GeneratePresignedUrlRequest(bucketName, keyName) .withMethod(HttpMethod.PUT) .withExpiration(new Date(System.currentTimeMillis() + 3600 * 1000)); URL url = s3Client.generatePresignedUrl(generatePresignedUrlRequest); System.out.println("Pre-Signed URL: " + url);
8. Use S3 Event Notifications
Configure S3 Event Notifications to trigger AWS Lambda or SNS for post-upload validation, processing, or alerting on failures.
Example: Notification for Upload Completion
Configure your S3 bucket to send an event to an SQS queue or Lambda function upon successful upload.
9. Leverage Data Replication
Use S3 Cross-Region Replication (CRR) or Same-Region Replication (SRR) for redundancy.
Automatically replicate files to another bucket for disaster recovery.
10. Automate with AWS DataSync
Use AWS DataSync for automated and highly reliable file transfers between on-premises storage and S3. It includes built-in retry and integrity checks.
11. Optimize Network Performance
Compress files before uploading:javaCopy codeFiles.write(Paths.get("compressed-file.gz"), gzipCompress(fileContent));
Use efficient transfer protocols (e.g., HTTP/2 if supported by your client).
12. Secure Transfers
Always use HTTPS for secure communication.
Use S3 Bucket Policies and IAM Roles to restrict access to authorized users.
Example: Complete Reliable Upload Function
java
Copy code
public void reliableUpload(File file, String bucketName, String keyName) { AmazonS3 s3Client = AmazonS3ClientBuilder.defaultClient(); TransferManager tm = TransferManagerBuilder.standard().withS3Client(s3Client).build(); try { // Multipart upload Upload upload = tm.upload(bucketName, keyName, file); upload.addProgressListener((ProgressListener) progressEvent -> { System.out.println("Transferred bytes: " + progressEvent.getBytesTransferred()); }); upload.waitForCompletion(); System.out.println("File uploaded successfully!"); } catch (AmazonClientException e) { System.err.println("Upload failed: " + e.getMessage()); } finally { tm.shutdownNow(); } }
0 notes
Text
Docker Kubernetes: Simplifying Container Management and Scaling with Ease
If you're diving into the world of containerization, you've probably come across terms like Docker and Kubernetes more times than you can count. These two technologies are the backbone of modern software development, especially when it comes to creating scalable, efficient, and manageable applications. Docker Kubernetes are often mentioned together because they complement each other so well. But what exactly do they do, and why are they so essential for developers today?
In this blog, we’ll walk through the essentials of Docker Kubernetes, exploring why they’re a game-changer in managing and scaling applications. By the end, you’ll have a clear understanding of how they work together and how learning about them can elevate your software development journey.
What Is Docker?
Let’s start with Docker. It’s a tool designed to make it easier to create, deploy, and run applications by using containers. Containers package up an application and its dependencies into a single, lightweight unit. Think of it as a portable environment that contains everything your app needs to run, from libraries to settings, without relying on the host’s operating system.
Using Docker means you can run your application consistently across different environments, whether it’s on your local machine, on a virtual server, or in the cloud. This consistency reduces the classic “it works on my machine” issue that developers often face.
Key Benefits of Docker
Portability: Docker containers can run on any environment, making your applications truly cross-platform.
Efficiency: Containers are lightweight and use fewer resources compared to virtual machines.
Isolation: Each container runs in its isolated environment, meaning fewer compatibility issues.
Understanding Kubernetes
Now that we’ve covered Docker, let’s move on to Kubernetes. Developed by Google, Kubernetes is an open-source platform designed to manage containerized applications across a cluster of machines. In simple terms, it takes care of scaling and deploying your Docker containers, making sure they’re always up and running as needed.
Kubernetes simplifies the process of managing multiple containers, balancing loads, and ensuring that your application stays online even if parts of it fail. If Docker helps you create and run containers, Kubernetes helps you manage and scale them across multiple servers seamlessly.
Key Benefits of Kubernetes
Scalability: Easily scale applications up or down based on demand.
Self-Healing: If a container fails, Kubernetes automatically replaces it with a new one.
Load Balancing: Kubernetes distributes traffic evenly to avoid overloading any container.
Why Pair Docker with Kubernetes?
When combined, Docker Kubernetes provide a comprehensive solution for modern application development. Docker handles the packaging and containerization of your application, while Kubernetes manages these containers at scale. For businesses and developers, using these two tools together is often the best way to streamline development, simplify deployment, and manage application workloads effectively.
For example, if you’re building a microservices-based application, you can use Docker to create containers for each service and use Kubernetes to manage those containers. This setup allows for high availability and easier maintenance, as each service can be updated independently without disrupting the rest of the application.
Getting Started with Docker Kubernetes
To get started with Docker Kubernetes, you’ll need to understand the basic architecture of each tool. Here’s a breakdown of some essential components:
1. Docker Images and Containers
Docker Image: The blueprint for your container, containing everything needed to run an application.
Docker Container: The running instance of a Docker Image, isolated and lightweight.
2. Kubernetes Pods and Nodes
Pod: The smallest unit in Kubernetes that can host one or more containers.
Node: A physical or virtual machine that runs Kubernetes Pods.
3. Cluster: A group of nodes working together to run containers managed by Kubernetes.
With this setup, Docker Kubernetes enable seamless deployment, scaling, and management of applications.
Key Use Cases for Docker Kubernetes
Microservices Architecture
By separating each function of an application into individual containers, Docker Kubernetes make it easy to manage, deploy, and scale each service independently.
Continuous Integration and Continuous Deployment (CI/CD)
Docker Kubernetes are often used in CI/CD pipelines, enabling fast, consistent builds, testing, and deployment.
High Availability Applications
Kubernetes ensures your application remains available, balancing traffic and restarting containers as needed.
DevOps and Automation
Docker Kubernetes play a central role in the DevOps process, supporting automation, efficiency, and flexibility.
Key Concepts to Learn in Docker Kubernetes
Container Orchestration: Learning how to manage containers efficiently across a cluster.
Service Discovery and Load Balancing: Ensuring users are directed to the right container.
Scaling and Self-Healing: Automatically adjusting the number of containers and replacing failed ones.
Best Practices for Using Docker Kubernetes
Resource Management: Define resources for each container to prevent overuse.
Security: Use Kubernetes tools like Role-Based Access Control (RBAC) and secrets management.
Monitor and Optimize: Use monitoring tools like Prometheus and Grafana to keep track of performance.
Conclusion: Why Learn Docker Kubernetes?
Whether you’re a developer or a business, adopting Docker Kubernetes can significantly enhance your application’s reliability, scalability, and performance. Learning Docker Kubernetes opens up possibilities for building robust, cloud-native applications that can scale with ease. If you’re aiming to create applications that need to handle high traffic and large-scale deployments, there’s no better combination.
Docker Kubernetes offers a modern, efficient way to develop, deploy, and manage applications in today's fast-paced tech world. By mastering these technologies, you’re setting yourself up for success in a cloud-driven, containerized future.
0 notes
Text
Hyperdisk ML: Integration To Speed Up Loading AI/ML Data

Hyperdisk ML can speed up the loading of AI/ML data. This tutorial explains how to use it to streamline and speed up the loading of AI/ML model weights on Google Kubernetes Engine (GKE). The main method for accessing Hyperdisk ML storage with GKE clusters is through the Compute Engine Persistent Disk CSI driver.
What is Hyperdisk ML?
You can scale up your applications with Hyperdisk ML, a high-performance storage solution. It is perfect for running AI/ML tasks that require access to a lot of data since it offers high aggregate throughput to several virtual machines at once.
Overview
It can speed up model weight loading by up to 11.9X when activated in read-only-many mode, as opposed to loading straight from a model registry. The Google Cloud Hyperdisk design, which enables scalability to 2,500 concurrent nodes at 1.2 TB/s, is responsible for this acceleration. This enables you to decrease pod over-provisioning and improve load times for your AI/ML inference workloads.
The following are the high-level procedures for creating and utilizing Hyperdisk ML:
Pre-cache or hydrate data in a disk image that is persistent: Fill Hyperdisk ML volumes with serving-ready data from an external data source (e.g., Gemma weights fetched from Cloud Storage). The disk image’s persistent disk needs to work with Google Cloud Hyperdisk.
Using an existing Google Cloud Hyperdisk, create a Hyperdisk ML volume: Make a Kubernetes volume that points to the data-loaded Hyperdisk ML volume. To make sure your data is accessible in every zone where your pods will operate, you can optionally establish multi-zone storage classes.
To use it volume, create a Kubernetes deployment: For your applications to use, refer to the Hyperdisk ML volume with rapid data loading.
Multi-zone Hyperdisk ML volumes
There is just one zone where hyperdisk ML disks are accessible. Alternatively, you may dynamically join many zonal disks with identical content under a single logical PersistentVolumeClaim and PersistentVolume by using the Hyperdisk ML multi-zone capability. The multi-zone feature’s referenced zonal disks have to be in the same area. For instance, the multi-zone disks (such as us-central1-a and us-central1-b) must be situated in the same area if your regional cluster is established in us-central1.
Running Pods across zones for increased accelerator availability and cost effectiveness with Spot VMs is a popular use case for AI/ML inference. Because it is zonal, GKE will automatically clone the disks across zones if your inference server runs several pods across zones to make sure your data follows your application.Image Credit To Google Cloud
The limitations of multi-zone Hyperdisk ML volumes are as follows:
There is no support for volume resizing or volume snapshots.
Only read-only mode is available for multi-zone Hyperdisk ML volumes.
GKE does not verify that the disk content is consistent across zones when utilizing pre-existing disks with a multi-zone Hyperdisk ML volume. Make sure your program considers the possibility of inconsistencies between zones if any of the disks have divergent material.
Requirements
The following Requirements must be met by your clusters in order to use it volumes in GKE:
Use Linux clusters with GKE 1.30.2-gke.1394000 or above installed. Make sure the release channel contains the GKE version or above that is necessary for this driver if you want to use one.
A driver for the Compute Engine Persistent Disk (CSI) must be installed. On new Autopilot and Standard clusters, the Compute Engine Persistent Disc driver is on by default and cannot be turned off or changed while Autopilot is in use. See Enabling the Compute Engine Persistent Disk CSI Driver on an Existing Cluster if you need to enable the Cluster’s Compute Engine Persistent Disk CSI driver.
You should use GKE version 1.29.2-gke.1217000 or later if you wish to adjust the readahead value.
You must use GKE version 1.30.2-gke.1394000 or later in order to utilize the multi-zone dynamically provisioned capability.
Only specific node types and zones allow hyperdisk ML.
Conclusion
This source offers a thorough tutorial on how to use Hyperdisk ML to speed up AI/ML data loading on Google Kubernetes Engine (GKE). It explains how to pre-cache data in a disk image, create a it volume that your workload in GKE can read, and create a deployment to use this volume. The article also discusses how to fix problems such a low it throughput quota and provides advice on how to adjust readahead numbers for best results.
Read more on Govindhtech.com
#AI#ML#HyperdiskML#GoogleKubernetesEngine#GKE#VMs#Kubernetes#News#Technews#Technology#Technologynews#Technologytrends#Govindhtech
0 notes
Text
Top 7 Essential DevOps Tools Every Intern Should Know for Success
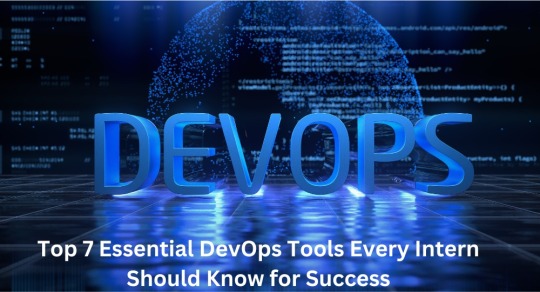
In the fast-paced world of software development, DevOps is a crucial bridge between development and operations. As an intern diving into this field, learning the right tools can give you a competitive edge, boost your productivity, and help you stand out. Whether you're collaborating with teams, automating tasks, or ensuring smooth deployments, understanding DevOps tools is essential.
Here, we’ll break down the top 7 DevOps tools every intern should know. These tools cover everything from continuous integration and deployment to infrastructure management and monitoring. Let's get started!https://internshipgate.com
1. Git: Version Control Done Right
Git is the backbone of version control systems. It allows multiple developers to work on the same project, track changes, and manage code versions efficiently.
Why You Need Git: As an intern, you’ll need to collaborate with others, and Git helps maintain order in a project's history by allowing you to revert to earlier versions when necessary.
Key Features: Branching, merging, commit history, and pull requests.
How to Get Started: Tools like GitHub or GitLab provide a user-friendly interface for Git, offering repositories to store your code, collaborate, and manage projects.
2. Jenkins: Automate Your Workflow
Jenkins is one of the most popular automation tools for continuous integration and continuous delivery (CI/CD). It automates repetitive tasks, such as building, testing, and deploying applications.
Why You Need Jenkins: Automation is at the heart of DevOps. Jenkins allows you to automate the testing and deployment process, reducing human errors and ensuring faster releases.
Key Features: Plugin support, pipeline as code, and easy configuration.
How to Get Started: As an intern, start by setting up simple pipelines and exploring Jenkins plugins to automate various development processes.
3. Docker: Containerization Made Simple
Docker enables developers to package applications into containers—standardized units that contain everything the application needs to run. This ensures consistency across environments, whether it's on your laptop or in production.
Why You Need Docker: Containerization simplifies the deployment process by eliminating the classic "it works on my machine" problem. You'll ensure consistency from development through to production.
Key Features: Lightweight containers, easy scaling, and isolation.
How to Get Started: Experiment by creating a Dockerfile for your project, building containers, and deploying them to services like Docker Hub or Kubernetes.
4. Kubernetes: Orchestrating Containers
Once you understand Docker, the next step is Kubernetes, a powerful orchestration tool that manages containerized applications across multiple hosts.
Why You Need Kubernetes: For large-scale projects, simply running containers isn’t enough. Kubernetes automates the deployment, scaling, and management of containerized applications, ensuring high availability.
Key Features: Load balancing, self-healing, and auto-scaling.
How to Get Started: Start by deploying a small application on a local Kubernetes cluster using Minikube and scaling it as you go.
5. Ansible: Automate Infrastructure Management
Ansible is a popular tool for automating infrastructure tasks. It simplifies complex tasks like application deployment, configuration management, and orchestration.
Why You Need Ansible: Ansible uses a simple, human-readable language (YAML) to automate repetitive tasks. For interns, it’s a great tool to learn how infrastructure is managed.
Key Features: Agentless, idempotent, and easy to learn.
How to Get Started: Set up basic Ansible playbooks to automate tasks like server setup or application deployment.
6. Terraform: Infrastructure as Code (IaC)
Terraform is a tool for creating, managing, and deploying infrastructure resources using a declarative configuration language.
Why You Need Terraform: With Terraform, you can automate infrastructure provisioning, ensuring that environments are consistent, scalable, and repeatable. It’s a key tool in DevOps for managing cloud resources.
Key Features: Cross-platform support, infrastructure state management, and modularity.
How to Get Started: Start by writing simple Terraform scripts to provision cloud resources like virtual machines or storage on platforms like AWS or Google Cloud.
7. Prometheus and Grafana: Monitoring and Visualization
DevOps is not just about deployment; it’s also about maintaining and monitoring the health of your applications and infrastructure. Prometheus and Grafana are the go-to tools for monitoring and visualization.
Why You Need Prometheus and Grafana: Monitoring ensures that you catch issues before they affect users. Prometheus collects metrics from your systems, while Grafana visualizes them, providing insights into system performance.
Key Features: Time-series data collection (Prometheus) and customizable dashboards (Grafana).
How to Get Started: Start with setting up Prometheus to collect basic metrics and use Grafana to create dashboards for visualizing CPU usage, memory, and request rates.
FAQs
What is the difference between Docker and Kubernetes? Docker is used for creating containers, which are lightweight and portable environments for running applications. Kubernetes, on the other hand, manages and orchestrates those containers across multiple machines, handling tasks like load balancing, scaling, and self-healing.
Why is version control important in DevOps? Version control, like Git, allows multiple developers to work on the same codebase without conflicting changes. It tracks changes, facilitates collaboration, and helps revert to previous versions if necessary, ensuring a smooth workflow.
How does Jenkins improve software development? Jenkins automates repetitive tasks such as testing and deployment, reducing manual effort, minimizing errors, and speeding up the release process through continuous integration and delivery (CI/CD).
Is Ansible better than Terraform for infrastructure management? Ansible and Terraform serve different purposes. Ansible is better for configuration management and automation, while Terraform excels in infrastructure provisioning and management. Many DevOps teams use both together.
Can I use Prometheus without Grafana? Yes, Prometheus can be used without Grafana, but it is often paired with Grafana for better visualization. Prometheus collects the metrics, and Grafana helps you analyze them with interactive dashboards.
How can interns start learning these DevOps tools? Start small by experimenting with free tutorials, hands-on labs, and online courses. Use cloud-based platforms like GitHub, AWS, or Google Cloud to practice with these tools in real-world scenarios.
Conclusion
Mastering these essential DevOps tools will set you up for success in your DevOps journey. As an intern, focusing on learning these tools will not only enhance your technical skills but also improve your ability to collaborate with teams and manage complex systems. Whether it's automating workflows with Jenkins or orchestrating containers with Kubernetes, each tool plays a critical role in modern software development and operations.https://internshipgate.com
#career#internship#virtualinternship#internshipgate#internship in india#education#devops#devops tools#interns#job opportunities
0 notes
Text
Transforming Infrastructure with Automation: The Power of Terraform and AWS Elastic Kubernetes Service Training
In the digital age, organizations are modernizing their infrastructure and shifting to cloud-native solutions. Terraform automates infrastructure provisioning across multiple cloud providers, while AWS Elastic Kubernetes Service (EKS) orchestrates containers, enabling businesses to manage scalable, high-availability applications. Together, these technologies form a foundation for managing dynamic systems at scale. To fully leverage them, professionals need practical, hands-on skills. This is where Elastic Kubernetes Services training becomes essential, offering expertise to automate and manage containerized applications efficiently, ensuring smooth operations across complex cloud infrastructures.
Why Automation Matters in Cloud Infrastructure
As businesses scale, manual infrastructure management becomes inefficient and prone to errors, especially in large, multi-cloud environments. Terraform, as an infrastructure-as-code (IaC) tool, automates provisioning, networking, and deployments, eliminating repetitive manual tasks and saving time. When paired with AWS Elastic Kubernetes Service (EKS), automation improves reliability and scalability, optimizes resource use, minimizes downtime, and significantly enhances deployment velocity for businesses operating in a cloud-native ecosystem.
The Role of Terraform in Automating AWS
Terraform simplifies cloud infrastructure by codifying resources into reusable, version-controlled configuration files, ensuring consistency and reducing manual effort across environments. In AWS, Terraform automates critical services such as EC2 instances, VPCs, and RDS databases. Integrated with Elastic Kubernetes Services (EKS), Terraform automates the lifecycle of Kubernetes clusters—from creating clusters to scaling applications across availability zones—allowing seamless cloud deployment and enhancing automation efficiency across diverse environments.
How AWS Elastic Kubernetes Service Elevates Cloud Operations
AWS Elastic Kubernetes Service (EKS) simplifies deploying, managing, and scaling Kubernetes applications by offering a fully managed control plane that takes the complexity out of Kubernetes management. When combined with Terraform, this automation extends even further, allowing infrastructure to be defined, deployed, and updated with minimal manual intervention. Elastic Kubernetes services training equips professionals to master this level of automation, from scaling clusters dynamically to managing workloads and applying security best practices in a cloud environment.
Benefits of Elastic Kubernetes Services Training for Professionals
Investing in Elastic Kubernetes Services training goes beyond managing Kubernetes clusters; it’s about gaining the expertise to automate and streamline cloud infrastructure efficiently. This training enables professionals to:
Increase Operational Efficiency: Automating repetitive tasks allows teams to focus on innovation rather than managing infrastructure manually, improving productivity across the board.
Scale Applications Seamlessly: Understanding how to leverage EKS ensures that applications can scale with demand, handling traffic spikes without sacrificing performance or reliability.
Stay Competitive: With cloud technologies evolving rapidly, staying up-to-date on tools like Terraform and EKS gives professionals a significant edge, allowing them to meet modern business demands effectively.
Driving Innovation with Automation
Automation is essential for businesses seeking to scale and remain agile in a competitive digital landscape. Terraform and AWS Elastic Kubernetes Service (EKS) enable organizations to automate infrastructure management and deploy scalable, resilient applications. Investing in Elastic Kubernetes Services training with Web Age Solutions equips professionals with technical proficiency and practical skills, positioning them as key innovators in their organizations while building scalable cloud environments that support long-term growth and future technological advancements.
For more information visit: https://www.webagesolutions.com/courses/WA3108-automation-with-terraform-and-aws-elastic-kubernetes-service
0 notes
Text
Are you exploring Kubernetes or considering using it? If yes, then you will be thankful you landed on this article about a little pondered but crucial component of Kubernetes: labels and annotations. As we know, Kubernetes is an orchestration tool that is usually used to manage containerized applications. In this article, we will be understanding labels and annotations are so important for managing containerized applications.Introduction to Labels and AnnotationsLabels are used in configuration files to specify attributes of objects that are meaningful and relevant to the user, especially in the grouping, viewing, and performing operations. In addition, labels can be used in the specs and metadata sections.Annotations, on the other hand, help provide a place to store non-identifying metadata, which may be used to elaborate on the context of an object.The following are some Kubernetes labels:name: Name of the applicationinstance: unique name of the instanceversion: semantic version numbercomponent: the component within your logical architecturepart-of: the name of the higher-level application this object is a part of.managed-by: the person who manages it.Note: Labels and selectors work together to identify groups of relevant resources. This procedure must be efficient because selectors are used to querying labels. Labels are always restricted by RFC 1123 to ensure efficient queries. Labels are limited to a maximum of 63 characters by RFC 1123, among other restrictions. When you want Kubernetes to group a set of relevant resources, you should use labels.LabelsLet’s dive in a little further. Labels are value pairs - like pods - that are attached to objects. They’re used to specify identifying attributes of objects which are relevant to users. They do not affect the semantics of the core system in any way - labels are just used to organize subsets of objects. Here, I have created labels using kubectl:Now, the question that arises is this: Why should we use labels in Kubernetes? This is the question most people ask, and so is one of the two main questions of this article.The main benefits of using labels in Kubernetes include help in organizing Kubernetes workloads in the clusters, mapping our own organizational structures into system objects, selecting or querying a specific item, grouping objects and accessing them when required, and finally enabling us to select anything we want and execute it in kubectl.You can use labels and annotations to attach metadata to objects – labels, in particular, can be used to select objects and find objects or collections of objects that satisfy certain criteria. Annotations, however, are not used for identification. Let’s look at annotations a bit more.AnnotationsNow, let us turn to the other question this article wishes to address: Why do we use annotations in Kubernetes?To answer this, let us first look at this photo:As you can see, I have created an image registry and specified the link. Now, in case of anything changes, I can track the changes in the URL specified. This is how we use annotations. One of the most frequently used examples for explaining the need for annotations is comparing it to storing phone numbers. The main benefits of using annotations in Kubernetes include helping us in elaborating the context at hand, the ability to track changes, communicating to the scheduler in Kubernetes about the scheduling policy, and keeping track of the replicates we have created. Kubernetes scheduling is the process of assigning pods to matched nodes in a cluster. The scheduler watches for newly created pods and assigns them to the best possible node based on scheduling principles and configuration options. In addition to that, annotations help us in deployment so that we can track replica sets, they help DevOps teams provide context and keep useful context, and they provide uniqueness, unlike labels.Some examples of information that can be recorded by annotations are fields; build, release, or image
information; pointers to logging; client libraries and tools; user or system provenance information; tool metadata; and directives from end-uses to implementations.ConclusionIn this article, we first went through the basic concept of what labels and annotations are. Then, we used kubectl, the most powerful and easy-to-use command-line tool for Kubernetes. Kubectl helps us query data in our Kubernetes cluster.As you can see, the use of labels and annotations in Kubernetes plays a key role in deployment. They not only help us add more information about our configuration files but also help other teams, especially the DevOps team, understand more about your files and their use in managing these applications.Thanks for reading. Let's keep building stuff together and learn a whole lot more every day! Stay tuned for more on Kubernetes, and happy learning!
0 notes
Text
Achieving Autoscaling Efficiency With EKS Managed Node Groups

Understanding EKS Managed Node Group Autoscaling
As businesses increasingly adopt Kubernetes for their container orchestration needs, managing and scaling node resources efficiently becomes crucial. Amazon Elastic Kubernetes Service (EKS) offers managed node groups that simplify the provisioning and management of worker nodes. One of the standout features of EKS managed node groups is autoscaling, which ensures that your Kubernetes cluster can dynamically adjust to changing workloads. In this blog, we’ll delve into the essentials of EKS managed node group autoscaling, its benefits, and best practices.
What is EKS Managed Node Group Autoscaling?
EKS managed node groups allow users to create and manage groups of EC2 instances that run Kubernetes worker nodes. Autoscaling is the feature that enables these node groups to automatically adjust their size based on the demand placed on your applications. This means adding nodes when your workload increases and removing nodes when the demand decreases, ensuring optimal resource utilization and cost efficiency.
How EKS Managed Node Group Autoscaling Works
EKS managed node group autoscaling leverages the Kubernetes Cluster Autoscaler and the Amazon EC2 Auto Scaling group to manage the scaling of your worker nodes.
Cluster Autoscaler: This Kubernetes component watches for pods that cannot be scheduled due to insufficient resources and automatically adjusts the size of the node group to accommodate the pending pods. Conversely, it also scales down the node group when nodes are underutilized.
EC2 Auto Scaling Group: EKS uses EC2 Auto Scaling groups to manage the underlying EC2 instances. This integration ensures that your Kubernetes worker nodes are automatically registered with the cluster and can be easily scaled in or out based on the metrics provided by the Cluster Autoscaler.
Benefits of EKS Managed Node Group Autoscaling
Cost Efficiency: Autoscaling helps optimize costs by ensuring that you only run the necessary number of nodes to handle your workloads, reducing the number of idle nodes and thus lowering your EC2 costs.
Improved Resource Utilization: By automatically adjusting the number of nodes based on workload, autoscaling ensures that your resources are used efficiently, which improves application performance and reliability.
Simplified Management: EKS managed node groups handle many of the complexities associated with managing Kubernetes worker nodes, including patching, updating, and scaling, allowing you to focus on your applications rather than infrastructure management.
Enhanced Reliability: Autoscaling helps maintain high availability and reliability by ensuring that your cluster can handle workload spikes without manual intervention, thus minimizing the risk of application downtime.
Best Practices for EKS Managed Node Group Autoscaling
Configure Resource Requests and Limits: Ensure that your Kubernetes workloads have properly configured resource requests and limits. This helps the Cluster Autoscaler make informed decisions about when to scale the node group.
Use Multiple Instance Types: Leverage multiple instance types within your managed node group to improve availability and flexibility. This allows the autoscaler to choose from a variety of instance types based on availability and cost.
Set Up Node Group Metrics: Use Amazon CloudWatch to monitor the performance and scaling activities of your node groups. This helps in understanding the scaling behavior and optimizing your configurations for better performance and cost savings.
Tune Autoscaler Parameters: Adjust the parameters of the Cluster Autoscaler to better fit your workload patterns. For example, you can set a maximum and minimum number of nodes to prevent over-provisioning or under-provisioning.
Regularly Update Your Node Groups: Keep your EKS managed node groups up to date with the latest Kubernetes and EC2 AMI versions. This ensures that your cluster benefits from the latest features, performance improvements, and security patches.
Conclusion
EKS managed node group autoscaling is a powerful feature that simplifies the management and scaling of Kubernetes worker nodes, ensuring efficient resource utilization and cost savings. By understanding how autoscaling works and following best practices, you can optimize your EKS clusters for better performance and reliability. Whether you are running a small development environment or a large production system, EKS managed node group autoscaling can help you meet your scaling needs dynamically and efficiently.
0 notes
Text
Ansible and Kubernetes Automation: Simplifying Cluster Management
Kubernetes is the go-to platform for container orchestration, but managing clusters at scale can become complex and time-consuming. This is where Ansible, a powerful IT automation tool, steps in to simplify Kubernetes management. In this blog, we’ll explore how Ansible can automate various Kubernetes tasks, making your operations more efficient and less error-prone.
Why Use Ansible for Kubernetes?
Ansible provides a flexible framework for automating Kubernetes tasks, offering benefits such as:
Centralized Management: Manage Kubernetes clusters alongside other infrastructure components from a single platform.
Consistency: Standardize how deployments, configurations, and updates are handled across multiple clusters.
Ease of Use: Human-readable playbooks make it easy to define and execute tasks without deep programming knowledge.
Integration: Seamlessly connect Kubernetes automation with tools like Helm, Prometheus, or external APIs.
Use Cases for Kubernetes Automation with Ansible
Deploying Applications Across Clusters Ansible can automate the deployment of applications across multiple Kubernetes clusters, ensuring uniformity. This is particularly useful in hybrid or multi-cloud environments.
Streamlining Resource Management From creating namespaces and ConfigMaps to updating deployments and scaling services, Ansible can handle all routine Kubernetes operations effortlessly.
Automating Security and Compliance Tasks like applying Role-Based Access Control (RBAC) policies, configuring network policies, and auditing resource usage can be automated with Ansible, improving security and compliance.
Simplifying Helm Chart Management Helm charts make deploying complex Kubernetes applications easier. With Ansible, you can automate the entire process, from fetching a chart to applying customized configurations.
Integrating with CI/CD Pipelines Ansible bridges the gap between Kubernetes and CI/CD systems, enabling seamless deployment of changes in development, staging, and production environments.
How Ansible Fits into Kubernetes Automation
Declarative vs. Imperative: While Kubernetes is declarative by nature, Ansible complements it with a task-based approach, making it ideal for orchestrating end-to-end workflows.
Cross-Environment Automation: Ansible can connect Kubernetes operations with other systems like load balancers, monitoring tools, and cloud providers, enabling a unified automation strategy.
Simplified Access Control: By integrating with Kubernetes APIs, Ansible ensures secure and controlled access to clusters.
Advantages of Using Ansible for Kubernetes
No Dependency on Agents: Ansible communicates directly with Kubernetes APIs, eliminating the need for agents or additional software on the cluster.
Idempotency: Ansible ensures tasks are performed consistently without unintended consequences, even if playbooks are run multiple times.
Scalability: Handle configurations for multiple clusters simultaneously, whether on-premises, cloud, or hybrid setups.
Improved Productivity: Teams can focus on strategic tasks as repetitive workflows are automated.
Best Practices for Ansible-Kubernetes Integration
Version Control Your Playbooks: Keep all automation scripts in a version control system like Git to maintain transparency and enable collaboration.
Test Before Production: Always test Ansible playbooks in a staging environment before applying them to production clusters.
Use Variables and Templates: Leverage variables to make playbooks reusable across different clusters or environments.
Follow Kubernetes Standards: Ensure your automation aligns with Kubernetes best practices for configurations and resource management.
Conclusion
Combining Ansible with Kubernetes brings together the best of automation and orchestration. By leveraging Ansible’s capabilities, you can simplify Kubernetes management, reduce manual errors, and improve operational efficiency. Whether you’re managing a single cluster or multiple environments, Ansible offers a robust and scalable solution for Kubernetes automation.
Start integrating Ansible into your Kubernetes workflows today, and experience a smoother, more efficient way to manage your clusters!
For more information visit: https://www.hawkstack.com/
0 notes
Text
Real-World Kubernetes Deployments with Azure Kubernetes Service (AKS)
Introduction Real-World Kubernetes Deployments with Azure Kubernetes Service (AKS) is a comprehensive tutorial that covers the implementation of a fully functional Kubernetes cluster on Microsoft Azure. AKS is a managed container service that simplifies the deployment, scaling, and management of containerized applications. What You Will Learn In this tutorial, you will learn: How to create a…
0 notes