#azure ai and ml services
Explore tagged Tumblr posts
Text
Everything you wanted to know about Azure OpenAI Pricing
Azure OpenAI is a powerful tool for businesses, integrating seamlessly with Azure for advanced AI capabilities. It automates processes like natural language processing and image recognition, enhancing efficiency. By leveraging these advances, businesses can automate tasks, improve operations, and unleash limitless potential.
In this blog, we provide detailed insights into Azure OpenAI pricing, helping you understand the costs associated with each service. Our expert guidance ensures you can navigate these options effectively, optimizing your AI investment.
Feel free to reach out if you have questions or need clarification on using Azure OpenAI services, including their use cases. Contact us for detailed pricing information and personalized support.
📞 Phone: +1(702) 780-7900
✉️ Email: [email protected]
1 note
·
View note
Text
Artificial Intellligence & Machine Learning Solutions | AI/ML Services
As we step into a new era of AI/ML, businesses can unlock unprecedented advantages, leveraging the power of data-driven insights, automated processes, intelligent decision-making, and transformative innovation to gain a competitive edge and drive exponential growth.
#Artificial Intellligence & Machine Learning Solutions#AI/ML Services#cloud based quantum machine learning services#cloud machine learning services#google machine learning services#ai and ml development company#aws nlp services#nlp services#azure nlp services
0 notes
Text
The Future of Jobs in IT: Which Skills You Should Learn.
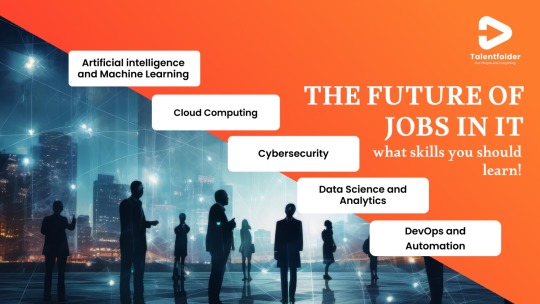
With changes in the industries due to technological changes, the demand for IT professionals will be in a constant evolution mode. New technologies such as automation, artificial intelligence, and cloud computing are increasingly being integrated into core business operations, which will soon make jobs in IT not just about coding but about mastering new technologies and developing versatile skills. Here, we cover what is waiting to take over the IT landscape and how you can prepare for this future.
1. Artificial Intelligence (AI) and Machine Learning (ML):
AI and ML are the things that are currently revolutionizing industries by making machines learn from data, automate processes, and predict outcomes. Thus, jobs for the future will be very much centered around these fields of AI and ML, and the professionals can expect to get work as AI engineers, data scientists, and automation specialists.
2. Cloud Computing:
With all operations now moving online, architects, developers, and security experts are in high demand for cloud work. It is very important to have skills on platforms such as AWS, Microsoft Azure, and Google Cloud for those who wish to work on cloud infrastructure and services.
3. Cybersecurity:
As dependence on digital mediums continues to increase, so must cybersecurity measures. Cybersecurity, ethical hacking, and network security would be skills everyone must use to protect data and systems from all the continuous threats.
4. Data Science and Analytics:
As they say, the new oil in this era is data. Therefore, organisations require professionals who would be able to analyze humongous datasets and infer actionable insights. Data science, data engineering, as well as advanced analytics tools, will be your cornucopia for thriving industries in the near future.
5. DevOps and Automation:
DevOps engineers are the ones who ensure that continuous integration and deployment work as smoothly and automatically as possible. Your knowledge of the business/operations will orient you well on that terrain, depending on how that applies to your needs.
Conclusion
IT job prospects rely heavily on AI, cloud computing, cybersecurity, and automation. It means that IT professionals must constantly innovate and update their skills to stay in competition. Whether an expert with years of experience or a newcomer, focusing on the following in-demand skills will gather success in this diverse land of IT evolution.
You might also like: How to crack interview in MNC IT
2 notes
·
View notes
Text
How can you optimize the performance of machine learning models in the cloud?
Optimizing machine learning models in the cloud involves several strategies to enhance performance and efficiency. Here’s a detailed approach:
Choose the Right Cloud Services:
Managed ML Services:
Use managed services like AWS SageMaker, Google AI Platform, or Azure Machine Learning, which offer built-in tools for training, tuning, and deploying models.
Auto-scaling:
Enable auto-scaling features to adjust resources based on demand, which helps manage costs and performance.
Optimize Data Handling:
Data Storage:
Use scalable cloud storage solutions like Amazon S3, Google Cloud Storage, or Azure Blob Storage for storing large datasets efficiently.
Data Pipeline:
Implement efficient data pipelines with tools like Apache Kafka or AWS Glue to manage and process large volumes of data.
Select Appropriate Computational Resources:
Instance Types:
Choose the right instance types based on your model’s requirements. For example, use GPU or TPU instances for deep learning tasks to accelerate training.
Spot Instances:
Utilize spot instances or preemptible VMs to reduce costs for non-time-sensitive tasks.
Optimize Model Training:
Hyperparameter Tuning:
Use cloud-based hyperparameter tuning services to automate the search for optimal model parameters. Services like Google Cloud AI Platform’s HyperTune or AWS SageMaker’s Automatic Model Tuning can help.
Distributed Training:
Distribute model training across multiple instances or nodes to speed up the process. Frameworks like TensorFlow and PyTorch support distributed training and can take advantage of cloud resources.
Monitoring and Logging:
Monitoring Tools:
Implement monitoring tools to track performance metrics and resource usage. AWS CloudWatch, Google Cloud Monitoring, and Azure Monitor offer real-time insights.
Logging:
Maintain detailed logs for debugging and performance analysis, using tools like AWS CloudTrail or Google Cloud Logging.
Model Deployment:
Serverless Deployment:
Use serverless options to simplify scaling and reduce infrastructure management. Services like AWS Lambda or Google Cloud Functions can handle inference tasks without managing servers.
Model Optimization:
Optimize models by compressing them or using model distillation techniques to reduce inference time and improve latency.
Cost Management:
Cost Analysis:
Regularly analyze and optimize cloud costs to avoid overspending. Tools like AWS Cost Explorer, Google Cloud’s Cost Management, and Azure Cost Management can help monitor and manage expenses.
By carefully selecting cloud services, optimizing data handling and training processes, and monitoring performance, you can efficiently manage and improve machine learning models in the cloud.
2 notes
·
View notes
Text
2 notes
·
View notes
Text
Navigating the Future as Azure Certifications in 2024
In the ever-evolving landscape of cloud technology, Azure certifications continue to be instrumental in shaping the careers of IT professionals and influencing the strategies of organizations worldwide. As we step into 2024, it's essential to explore the current trends, advancements, and the transformative impact that Azure certifications are poised to have in the coming year.
The Continued Relevance of Azure Certifications
Azure certifications are not mere credentials; they are gateways to expertise and recognition in the dynamic world of cloud computing. As businesses increasingly rely on cloud solutions, the demand for skilled Azure professionals continues to grow. In 2024, Azure certifications stand as key assets, ensuring that professionals possess the knowledge and skills needed to navigate the complexities of cloud technology effectively.
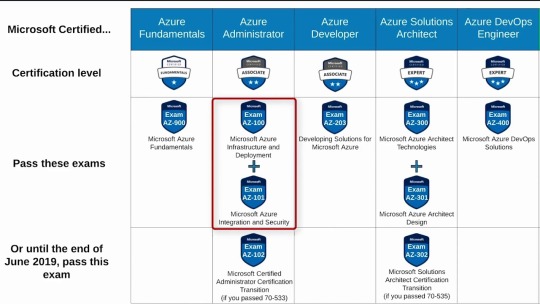
Azure Certification Paths in 2024
Azure certifications are structured into comprehensive paths, catering to individuals at various stages of their cloud journey. From foundational certifications for beginners to advanced tracks tailored for specialized roles like Azure Solutions Architect or Azure DevOps Engineer, the certification paths have evolved to align with the diverse needs of IT professionals. The year 2024 sees an increased focus on role-based certifications, allowing individuals to hone specific skills relevant to their job roles.
Key Benefits of Azure Certifications in 2024
Career Advancement:
Azure certifications are a proven catalyst for career growth. In 2024, as businesses seek skilled professionals, holding an Azure certification becomes a valuable asset for those aiming to advance their careers in cloud technology.
Industry Recognition:
Globally recognized, Azure certifications validate one's expertise in Microsoft's cloud services. Employers across industries acknowledge and value these certifications as a mark of proficiency in handling Azure-based solutions.
Continuous Learning Culture:
In 2024, Azure certifications are not just about achieving a one-time qualification; they embody a commitment to continuous learning. Microsoft regularly updates its certification paths to align with emerging technologies, encouraging professionals to stay abreast of the latest industry trends.
Increased Employability:
As the job market becomes more competitive, possessing Azure certifications enhances employability. In 2024, businesses are actively seeking candidates with practical Azure skills, making certification holders highly sought after.
Emerging Trends in Azure Certifications for 2024
Micro-Certifications:
2024 witnesses a rise in micro-certifications, focusing on specific, targeted skills. These bite-sized certifications allow professionals to demonstrate expertise in niche areas, providing a more granular approach to skill validation.
Scenario-Based Assessments:
Azure certification exams in 2024 are increasingly incorporating scenario-based questions. This shift aims to evaluate not just theoretical knowledge but the ability to apply that knowledge in practical, real-world situations.
Integration of AI and ML:
With the growing importance of artificial intelligence (AI) and machine learning (ML), Azure certifications in 2024 are placing a greater emphasis on these technologies. Certification tracks dedicated to AI and ML applications within Azure are gaining prominence.
Focus on Security:
In response to the heightened concern for cybersecurity, Azure certifications in 2024 place a significant focus on security-related tracks. Azure Security Engineer certifications are expected to be in high demand as organizations prioritize securing their cloud environments.
Tips for Success in Azure Certifications 2024
Stay Updated: Given the evolving nature of technology, staying updated with the latest Azure services and features is crucial. Regularly check Microsoft's official documentation and announcements for any updates.
Hands-On Experience: Practical experience is invaluable. Utilize Azure's sandbox environments, participate in real-world projects, and engage with the Azure portal to reinforce your theoretical knowledge.
Leverage Learning Resources: Microsoft provides a wealth of learning resources, including online courses, documentation, and practice exams. Take advantage of these resources to supplement your preparation.
Join the Azure Community: Engage with the Azure community through forums, webinars, and social media. Networking with professionals in the field can provide insights, tips, and support during your certification journey.
Conclusion
As we venture into 2024, Azure certifications stand as pivotal tools for IT professionals aiming to thrive in the dynamic world of cloud technology. Whether you are starting your journey with foundational certifications or advancing your skills with specialized tracks, Azure certifications in 2024 represent more than just qualifications – they symbolize a commitment to excellence, continuous learning, and a future shaped by innovation in the cloud. Embrace the opportunities, stay ahead of the curve, and let Azure certifications be your guide to success in the ever-evolving realm of cloud proficiency.
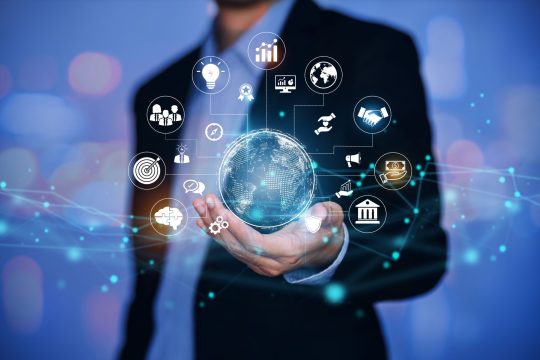
Frequently Asked Questions (FAQs)
What are the new Azure certifications introduced in 2024?
As of 2024, Microsoft has introduced several new certifications to align with emerging technologies. Notable additions include specialized tracks focusing on AI, ML, and advanced security.
How has the exam format changed for 2024?
The exam format in 2024 has evolved to include more scenario-based questions. This change is aimed at assessing practical application skills in addition to theoretical knowledge.
Are there any prerequisites for Azure certifications in 2024?
Prerequisites vary based on the specific certification. While some foundational certifications may have no prerequisites, advanced certifications often require prior experience or the completion of specific lower-level certifications.
Can I still take exams for older Azure certifications in 2024?
Microsoft often provides a transition period for older certifications, allowing candidates to complete them even as new certifications are introduced. However, it's advisable to check Microsoft's official documentation for specific details.
How frequently are Azure certifications updated?
Azure certifications are regularly updated to stay aligned with the latest technologies and industry trends. Microsoft recommends that candidates stay informed about updates through official communication channels.
4 notes
·
View notes
Text
How To Get An Online Internship In the IT Sector (Skills And Tips)
Internships provide invaluable opportunities to gain practical skills, build professional networks, and get your foot in the door with top tech companies.
With remote tech internships exploding in IT, online internships are now more accessible than ever. Whether a college student or career changer seeking hands-on IT experience, virtual internships allow you to work from anywhere.
However, competition can be fierce, and simply applying is often insufficient. Follow this comprehensive guide to develop the right technical abilities.
After reading this, you can effectively showcase your potential, and maximize your chances of securing a remote tech internship.
Understand In-Demand IT Skills
The first step is gaining a solid grasp of the most in-demand technical and soft skills. While specific requirements vary by company and role, these competencies form a strong foundation:
Technical Skills:
Proficiency in programming languages like Python, JavaScript, Java, and C++
Experience with front-end frameworks like React, Angular, and Vue.js
Back-end development skills - APIs, microservices, SQL databases Cloud platforms such as AWS, Azure, Google Cloud
IT infrastructure skills - servers, networks, security
Data science abilities like SQL, R, Python
Web development and design
Mobile app development - Android, iOS, hybrid
Soft Skills:
Communication and collaboration
Analytical thinking and problem-solving
Leadership and teamwork
Creativity and innovation
Fast learning ability
Detail and deadline-oriented
Flexibility and adaptability
Obtain Relevant Credentials
While hands-on skills hold more weight, relevant academic credentials and professional IT certifications can strengthen your profile. Consider pursuing:
Bachelor’s degree in Computer Science, IT, or related engineering fields
Internship-specific courses teaching technical and soft skills
Certificates like CompTIA, AWS, Cisco, Microsoft, Google, etc.
Accredited boot camp programs focusing on applied skills
MOOCs to build expertise in trending technologies like AI/ML, cybersecurity
Open source contributions on GitHub to demonstrate coding skills
The right credentials display a work ethic and supplement practical abilities gained through projects.
Build An Impressive Project Portfolio
Nothing showcases skills better than real-world examples of your work. Develop a portfolio of strong coding, design, and analytical projects related to your target internship field.
Mobile apps - publish on app stores or use GitHub project pages
Websites - deploy online via hosting services
Data science - showcase Jupyter notebooks, visualizations
Open source code - contribute to public projects on GitHub
Technical writing - blog posts explaining key concepts
Automation and scripts - record demo videos
Choose projects demonstrating both breadth and depth. Align them to skills required for your desired internship roles.
Master Technical Interview Skills
IT internship interviews often include challenging technical questions and assessments. Be prepared to:
Explain your code and projects clearly. Review them beforehand.
Discuss concepts related to key technologies on your resume. Ramp up on fundamentals.
Solve coding challenges focused on algorithms, data structures, etc. Practice online judges like LeetCode.
Address system design and analytical problems. Read case interview guides.
Show communication and collaboration skills through pair programming tests.
Ask smart, well-researched questions about the company’s tech stack, projects, etc.
Schedule dedicated time for technical interview practice daily. Learn to think aloud while coding and get feedback from peers.
Show Passion and Curiosity
Beyond raw skills, demonstrating genuine passion and curiosity for technology goes a long way.
Take online courses and certifications beyond the college curriculum
Build side projects and engage in hackathons for self-learning
Stay updated on industry news, trends, and innovations
Be active on forums like StackOverflow to exchange knowledge
Attend tech events and conferences
Participate in groups like coding clubs and prior internship programs
Follow tech leaders on social mediaListen to tech podcasts while commuting
Show interest in the company’s mission, products, and culture
This passion shines through in interviews and applications, distinguishing you from other candidates.
Promote Your Personal Brand
In the digital age, your online presence and personal brand are make-or-break. Craft a strong brand image across:
LinkedIn profile - showcase achievements, skills, recommendations
GitHub - displays coding activity and quality through clean repositories
Portfolio website - highlight projects and share valuable content
Social media - post career updates and useful insights, but avoid oversharing
Blogs/videos - demonstrate communication abilities and thought leadership
Online communities - actively engage and build relationships
Ensure your profiles are professional and consistent. Let your technical abilities and potential speak for themselves.
Optimize Your Internship Applications
Applying isn’t enough. You must optimize your internship applications to get a reply:
Ensure you apply to openings that strongly match your profile Customize your resume and cover letters using keywords in the job description
Speak to skills gained from coursework, online learning, and personal projects
Quantify achievements rather than just listing responsibilities
Emphasize passion for technology and fast learning abilities
Ask insightful questions that show business understanding
Follow up respectfully if you don’t hear back in 1-2 weeks
Show interest in full-time conversion early and often
Apply early since competitive openings close quickly
Leverage referrals from your network if possible
This is how you do apply meaningfully. If you want a good internship, focus on the quality of applications. The hard work will pay off.
Succeed in Your Remote Internship
The hard work pays off when you secure that long-awaited internship! Continue standing out through the actual internship by:
Over Communicating in remote settings - proactively collaborate
Asking smart questions and owning your learning
Finding mentors and building connections remotely
Absorbing constructive criticism with maturity
Shipping quality work on or before deadlines
Clarifying expectations frequently
Going above and beyond prescribed responsibilities sometimes
Getting regular feedback and asking for more work
Leaving with letters of recommendation and job referrals
When you follow these tips, you are sure to succeed in your remote internship. Remember, soft skills can get you long ahead in the company, sometimes core skills can’t.
Conclusion
With careful preparation, tenacity, and a passion for technology, you will be able to get internships jobs in USA that suit your needs in the thriving IT sector.
Use this guide to build the right skills, create an impressive personal brand, ace the applications, and excel in your internship.
Additionally, you can browse some good job portals. For instance, GrandSiren can help you get remote tech internships. The portal has the best internship jobs in India and USA you’ll find. The investment will pay dividends throughout your career in this digital age. Wishing you the best of luck! Let me know in the comments about your internship hunt journey.
#itjobs#internship opportunities#internships#interns#entryleveljobs#gradsiren#opportunities#jobsearch#careeropportunities#jobseekers#ineffable interns#jobs#employment#career
4 notes
·
View notes
Text
How To Get An Online Internship In the IT Sector (Skills And Tips)
Internships provide invaluable opportunities to gain practical skills, build professional networks, and get your foot in the door with top tech companies.
With remote tech internships exploding in IT, online internships are now more accessible than ever. Whether a college student or career changer seeking hands-on IT experience, virtual internships allow you to work from anywhere.
However, competition can be fierce, and simply applying is often insufficient. Follow this comprehensive guide to develop the right technical abilities.
After reading this, you can effectively showcase your potential, and maximize your chances of securing a remote tech internship.
Understand In-Demand IT Skills
The first step is gaining a solid grasp of the most in-demand technical and soft skills. While specific requirements vary by company and role, these competencies form a strong foundation:
Technical Skills:
>> Proficiency in programming languages like Python, JavaScript, Java, and C++ >> Experience with front-end frameworks like React, Angular, and Vue.js >> Back-end development skills - APIs, microservices, SQL databases >> Cloud platforms such as AWS, Azure, Google Cloud >> IT infrastructure skills - servers, networks, security >> Data science abilities like SQL, R, Python >> Web development and design >> Mobile app development - Android, iOS, hybrid
Soft Skills:
>> Communication and collaboration >> Analytical thinking and problem-solving >> Leadership and teamwork >> Creativity and innovation >> Fast learning ability >> Detail and deadline-oriented >> Flexibility and adaptability
Obtain Relevant Credentials
While hands-on skills hold more weight, relevant academic credentials and professional IT certifications can strengthen your profile. Consider pursuing:
>> Bachelor’s degree in Computer Science, IT, or related engineering fields. >> Internship-specific courses teaching technical and soft skills. >> Certificates like CompTIA, AWS, Cisco, Microsoft, Google, etc. >> Accredited boot camp programs focusing on applied skills. >> MOOCs to build expertise in trending technologies like AI/ML, cybersecurity. >> Open source contributions on GitHub to demonstrate coding skills.
The right credentials display a work ethic and supplement practical abilities gained through projects.
Build An Impressive Project Portfolio
Nothing showcases skills better than real-world examples of your work. Develop a portfolio of strong coding, design, and analytical projects related to your target internship field.
>> Mobile apps - publish on app stores or use GitHub project pages >> Websites - deploy online via hosting services >> Data science - showcase Jupyter notebooks, visualizations >> Open source code - contribute to public projects on GitHub >> Technical writing - blog posts explaining key concepts >> Automation and scripts - record demo videos
Choose projects demonstrating both breadth and depth. Align them to skills required for your desired internship roles.
Master Technical Interview Skills
IT internship interviews often include challenging technical questions and assessments. Be prepared to:
>> Explain your code and projects clearly. Review them beforehand. >> Discuss concepts related to key technologies on your resume. Ramp up on fundamentals. >> Solve coding challenges focused on algorithms, data structures, etc. Practice online judges like LeetCode. >> Address system design and analytical problems. Read case interview guides. >> Show communication and collaboration skills through pair programming tests. >> Ask smart, well-researched questions about the company’s tech stack, projects, etc.
Schedule dedicated time for technical interview practice daily. Learn to think aloud while coding and get feedback from peers.
Show Passion and Curiosity
Beyond raw skills, demonstrating genuine passion and curiosity for technology goes a long way.
>> Take online courses and certifications beyond the college curriculum >> Build side projects and engage in hackathons for self-learning >> Stay updated on industry news, trends, and innovations >> Be active on forums like StackOverflow to exchange knowledge >> Attend tech events and conferences >> Participate in groups like coding clubs and prior internship programs >> Follow tech leaders on social media >> Listen to tech podcasts while commuting >> Show interest in the company’s mission, products, and culture
This passion shines through in interviews and applications, distinguishing you from other candidates.
Promote Your Personal Brand
In the digital age, your online presence and personal brand are make-or-break. Craft a strong brand image across:
>> LinkedIn profile - showcase achievements, skills, recommendations >> GitHub - displays coding activity and quality through clean repositories >> Portfolio website - highlight projects and share valuable content >> Social media - post career updates and useful insights, but avoid oversharing >> Blogs/videos - demonstrate communication abilities and thought leadership >> Online communities - actively engage and build relationships
Ensure your profiles are professional and consistent. Let your technical abilities and potential speak for themselves.
Optimize Your Internship Applications
Applying isn’t enough. You must optimize your internship applications to get a reply:
>> Ensure you apply to openings that strongly match your profile >> Customize your resume and cover letters using keywords in the job description >> Speak to skills gained from coursework, online learning, and personal projects >> Quantify achievements rather than just listing responsibilities >> Emphasize passion for technology and fast learning abilities >> Ask insightful questions that show business understanding >> Follow up respectfully if you don’t hear back in 1-2 weeks >> Show interest in full-time conversion early and often >> Apply early since competitive openings close quickly >> Leverage referrals from your network if possible
This is how you do apply meaningfully. If you want a good internship, focus on the quality of applications. The hard work will pay off.
Succeed in Your Remote Internship
The hard work pays off when you secure that long-awaited internship! Continue standing out through the actual internship by:
>> Over Communicating in remote settings - proactively collaborate >> Asking smart questions and owning your learning >> Finding mentors and building connections remotely >> Absorbing constructive criticism with maturity >> Shipping quality work on or before deadlines >> Clarifying expectations frequently >> Going above and beyond prescribed responsibilities sometimes >> Getting regular feedback and asking for more work >> Leaving with letters of recommendation and job referrals
When you follow these tips, you are sure to succeed in your remote internship. Remember, soft skills can get you long ahead in the company, sometimes core skills can’t.
Conclusion
With careful preparation, tenacity, and a passion for technology, you will be able to get internships jobs in USA that suit your needs in the thriving IT sector.
Use this guide to build the right skills, create an impressive personal brand, ace the applications, and excel in your internship.
Additionally, you can browse some good job portals. For instance, GrandSiren can help you get remote tech internships. The portal has the best internship jobs in India and USA you’ll find.
The investment will pay dividends throughout your career in this digital age. Wishing you the best of luck! Let me know in the comments about your internship hunt journey.
#internship#internshipopportunity#it job opportunities#it jobs#IT internships#jobseekers#jobsearch#entryleveljobs#employment#gradsiren#graduation#computer science#technology#engineering#innovation#information technology#remote jobs#remote work#IT Remote jobs
5 notes
·
View notes
Text
Microsoft Azure Fundamentals AI-900 (Part 5)
Microsoft Azure AI Fundamentals: Explore visual studio tools for machine learning
What is machine learning? A technique that uses math and statistics to create models that predict unknown values
Types of Machine learning
Regression - predict a continuous value, like a price, a sales total, a measure, etc
Classification - determine a class label.
Clustering - determine labels by grouping similar information into label groups
x = features
y = label
Azure Machine Learning Studio
You can use the workspace to develop solutions with the Azure ML service on the web portal or with developer tools
Web portal for ML solutions in Sure
Capabilities for preparing data, training models, publishing and monitoring a service.
First step assign a workspace to a studio.
Compute targets are cloud-based resources which can run model training and data exploration processes
Compute Instances - Development workstations that data scientists can use to work with data and models
Compute Clusters - Scalable clusters of VMs for on demand processing of experiment code
Inference Clusters - Deployment targets for predictive services that use your trained models
Attached Compute - Links to existing Azure compute resources like VMs or Azure data brick clusters
What is Azure Automated Machine Learning
Jobs have multiple settings
Provide information needed to specify your training scripts, compute target and Azure ML environment and run a training job
Understand the AutoML Process
ML model must be trained with existing data
Data scientists spend lots of time pre-processing and selecting data
This is time consuming and often makes inefficient use of expensive compute hardware
In Azure ML data for model training and other operations are encapsulated in a data set.
You create your own dataset.
Classification (predicting categories or classes)
Regression (predicting numeric values)
Time series forecasting (predicting numeric values at a future point in time)
After part of the data is used to train a model, then the rest of the data is used to iteratively test or cross validate the model
The metric is calculated by comparing the actual known label or value with the predicted one
Difference between the actual known and predicted is known as residuals; they indicate amount of error in the model.
Root Mean Squared Error (RMSE) is a performance metric. The smaller the value, the more accurate the model’s prediction is
Normalized root mean squared error (NRMSE) standardizes the metric to be used between models which have different scales.
Shows the frequency of residual value ranges.
Residuals represents variance between predicted and true values that can’t be explained by the model, errors
Most frequently occurring residual values (errors) should be clustered around zero.
You want small errors with fewer errors at the extreme ends of the sale
Should show a diagonal trend where the predicted value correlates closely with the true value
Dotted line shows a perfect model’s performance
The closer to the line of your model’s average predicted value to the dotted, the better.
Services can be deployed as an Azure Container Instance (ACI) or to a Azure Kubernetes Service (AKS) cluster
For production AKS is recommended.
Identify regression machine learning scenarios
Regression is a form of ML
Understands the relationships between variables to predict a desired outcome
Predicts a numeric label or outcome base on variables (features)
Regression is an example of supervised ML
What is Azure Machine Learning designer
Allow you to organize, manage, and reuse complex ML workflows across projects and users
Pipelines start with the dataset you want to use to train the model
Each time you run a pipelines, the context(history) is stored as a pipeline job
Encapsulates one step in a machine learning pipeline.
Like a function in programming
In a pipeline project, you access data assets and components from the Asset Library tab
You can create data assets on the data tab from local files, web files, open at a sets, and a datastore
Data assets appear in the Asset Library
Azure ML job executes a task against a specified compute target.
Jobs allow systematic tracking of your ML experiments and workflows.
Understand steps for regression
To train a regression model, your data set needs to include historic features and known label values.
Use the designer’s Score Model component to generate the predicted class label value
Connect all the components that will run in the experiment
Average difference between predicted and true values
It is based on the same unit as the label
The lower the value is the better the model is predicting
The square root of the mean squared difference between predicted and true values
Metric based on the same unit as the label.
A larger difference indicates greater variance in the individual label errors
Relative metric between 0 and 1 on the square based on the square of the differences between predicted and true values
Closer to 0 means the better the model is performing.
Since the value is relative, it can compare different models with different label units
Relative metric between 0 and 1 on the square based on the absolute of the differences between predicted and true values
Closer to 0 means the better the model is performing.
Can be used to compare models where the labels are in different units
Also known as R-squared
Summarizes how much variance exists between predicted and true values
Closer to 1 means the model is performing better
Remove training components form your data and replace it with a web service inputs and outputs to handle the web requests
It does the same data transformations as the first pipeline for new data
It then uses trained model to infer/predict label values based on the features.
Create a classification model with Azure ML designer
Classification is a form of ML used to predict which category an item belongs to
Like regression this is a supervised ML technique.
Understand steps for classification
True Positive - Model predicts the label and the label is correct
False Positive - Model predicts wrong label and the data has the label
False Negative - Model predicts the wrong label, and the data does have the label
True Negative - Model predicts the label correctly and the data has the label
For multi-class classification, same approach is used. A model with 3 possible results would have a 3x3 matrix.
Diagonal lien of cells were the predicted and actual labels match
Number of cases classified as positive that are actually positive
True positives divided by (true positives + false positives)
Fraction of positive cases correctly identified
Number of true positives divided by (true positives + false negatives)
Overall metric that essentially combines precision and recall
Classification models predict probability for each possible class
For binary classification models, the probability is between 0 and 1
Setting the threshold can define when a value is interpreted as 0 or 1. If its set to 0.5 then 0.5-1.0 is 1 and 0.0-0.4 is 0
Recall also known as True Positive Rate
Has a corresponding False Positive Rate
Plotting these two metrics on a graph for all values between 0 and 1 provides information.
Receiver Operating Characteristic (ROC) is the curve.
In a perfect model, this curve would be high to the top left
Area under the curve (AUC).
Remove training components form your data and replace it with a web service inputs and outputs to handle the web requests
It does the same data transformations as the first pipeline for new data
It then uses trained model to infer/predict label values based on the features.
Create a Clustering model with Azure ML designer
Clustering is used to group similar objects together based on features.
Clustering is an example of unsupervised learning, you train a model to just separate items based on their features.
Understanding steps for clustering
Prebuilt components exist that allow you to clean the data, normalize it, join tables and more
Requires a dataset that includes multiple observations of the items you want to cluster
Requires numeric features that can be used to determine similarities between individual cases
Initializing K coordinates as randomly selected points called centroids in an n-dimensional space (n is the number of dimensions in the feature vectors)
Plotting feature vectors as points in the same space and assigns a value how close they are to the closes centroid
Moving the centroids to the middle points allocated to it (mean distance)
Reassigning to the closes centroids after the move
Repeating the last two steps until tone.
Maximum distances between each point and the centroid of that point’s cluster.
If the value is high it can mean that cluster is widely dispersed.
With the Average Distance to Closer Center, we can determine how spread out the cluster is
Remove training components form your data and replace it with a web service inputs and outputs to handle the web requests
It does the same data transformations as the first pipeline for new data
It then uses trained model to infer/predict label values based on the features.
2 notes
·
View notes
Text
Accelerating transformation with SAP on Azure
Microsoft continues to expand its presence in the cloud by building more data centers globally, with over 61 Azure regions in 140 countries. They are expanding their reach and capabilities to meet all the customer needs. The transition from a cloudless domain like DRDC to the entire cloud platform is possible within no time, and a serverless future awaits. Microsoft gives the platform to build and innovate at a rapid speed. Microsoft is enhancing new capabilities to meet cloud services' demands and needs, from IaaS to PaaS Data, AI, ML, and IoT. There are over 600 services available on Azure with a cloud adoption framework and enterprise-scale landing zone. Many companies look at Microsoft Azure security compliance as a significant migration driver. Microsoft Azure has an extensive list of compliance certifications across the globe. The Microsoft services have several beneficial characteristics; capabilities are broad, deep, and suited to any industry, along with a global network of skilled professionals and partners. Expertise in the Microsoft portfolio includes both technology integration and digital transformation. Accountability for the long term, addressing complex challenges while mitigating risk. Flexibility to engage in the way that works for you with the global reach to satisfy the target business audience.
SAP and Microsoft Azure
SAP and Microsoft bring together the power of industry-specific best practices, reference architectures, and professional services and support to simplify and safeguard your migration to SAP in the cloud and help manage the ongoing business operations now and in the future. SAP and Microsoft have collaborated to design and deliver a seamless, optimized experience to help manage migration and business operations as you move from on-premises editions of SAP solutions to SAP S/4 HANA on Microsoft Azure. It reduces complexity, minimizes costs, and supports end-to-end SAP migration and operations strategy, platform, and services. As a result, one can safeguard the cloud migration with out-of-box functionality and industry-specific best practices while immaculately handling the risk and optimizing the IT environment. Furthermore, the migration assimilates best-in-class technologies from SAP and Microsoft, packed with a unified business cloud platform.
SAP Deployment Options on Azure
SAP system is deployed on-premises or in Azure. One can deploy different systems into different landscapes either on Azure or on-premises. SAP HANA on Azure large instances intend to host the SAP application layer of SAP systems in Virtual Machines and the related SAP HANA instance on the unit in the 'SAP HANA Azure Large Instance Stamp.' 'A Large Instance Stamp' is a hardware infrastructure stack that is SAP HANA TDI certified and dedicated to running SAP HANA instances within Azure. 'SAP HANA Large Instances' is the official name for the solution in Azure to run HANA instances on SAP HANA TDI certified hardware that gets deployed in ‘Large Instance Stamps’ in different Azure regions. SAP or HANA Large Instances or HLI are physical servers meaning bare metal servers. HLI does not reside in the same data center as Azure services but is in close proximity and connected through high throughput links to satisfy SAP HANA network latency requirements. HLI comes in two flavors- Type 1 and 2. IaaS can install SAP HANA on a virtual machine running on Azure. Running SAP HANA on IaaS supports more Linux versions than HLI. For example, you can install SAP Netweaver on Windows and Linux IaaS Virtual Machines on Azure. SAP HANA can only run on RedHat and SUSE, while NetWeaver can run on windows SQL and Linux.
Azure Virtual Network
Azure Virtual Network or VNET is a core foundation of the infrastructure implementation on Azure. The VNET can be a communication boundary for those resources that need to communicate. You can have multiple VNETs in your subscription. If they weren't connected, we could call them Pierre in Azure wall; there will be no traffic flow in between. They can also share the same IP range. Understanding the requirements and proper setup is essential as changing them later, especially with the running production workloads, could cause downtime. When you provision a VNET, The private blocks must allocate address space. If you plan to connect multiple VNETs, you cannot have an overlapping address space. The IP range should not clash or overlap with the IP addressing in Azure while connecting on-premise to Azure via express route or site-site VPN. Configuring VNET to the IP address space becomes a DHP service. You can configure VNET with the DNS server's IP addresses to resolve services on-premise.VNETS can be split into different subnets and communicate freely with each other. Network security groups or NSGs are the control planes we use to filter traffic. NSGs are stateful but simple firewall rules based on the source and destination IP and ports.
Azure Virtual Gateway
For extensive connectivity, you must create a virtual gateway subnet. When you create a virtual gateway, you will get prompted for two options: VPN or Express Route Gateway; with VPN, you cannot connect to the Express Route Circuit. If you choose the Express Route Virtual Gateway, you can combine both.
There are two types of VPN;
1) The point-to-site VPN is used for testing and gives the lowest throughput.
2) The site-site VPN connection can offer better benefits by bridging networks.
This VPN offers zero support for SLA and uses this connection as a backup for the recommended connection on Azure, called the express route. Express route is a dedicated circuit using hardware installed on your data center, with a constant link to ‘Microsoft Azure Edge’ devices. Express route is inevitable for maintaining the communication between application VNET running in Azure and on-premise systems to HLI servers. The express route is safer and more resilient than VPN as it provides a connection through a single circuit and facilitates second redundancy; this helps route traffic between SAP application servers inside Azure and enables low latency. Furthermore, the fast path allows routine traffic between SAP application servers inside Azure VNET and HLI through an optimized route that bypasses the virtual network gateway and directly hops through edge routers to HLA servers. Therefore, an ultra-performance express route gateway must have a Fast Path feature.
SAP HANA Architecture (VM)
This design gets centered on the SAP HANA backend on the Linux Suse or RedHat distributions. Even though the Linux OS implementation is the same, the vendor licensing differs. It incorporates always-on replication and utilizes synchronous and asynchronous replication to meet the HANA DB requirements. We have also introduced NetApp file share for DFS volumes used by each SAP component using Azure site recovery and building a DR plan for App ASCs and the web dispatches servers. Azure Active directory is used in synchronization with on-premises active directory, as SAP application user authenticates from on-premises to SAP landscape on Azure with Single Sign-On credentials. Azure high-speed express route gateway securely connects on-premises networks to Azure virtual machines and other resources. The request flows into highly available SAP central, SAP ABAP services ASCS and through SAP application servers running on Azure virtual machines. The on-demand request moves from the SAP App server to the SAP HANA server running on a high-performance Azure VM. Primary active and secondary standby servers run on SAP-certified virtual machines with a cluster availability of 99.95 at the OS level. Data replication is handled through HSR in synchronous mode from primary to secondary enabling zero recovery point objective. SAP HANA data is replicated through a disaster recovery VM in another Azure region through the Azure high-speed backbone network and using HSR in asynchronous mode. The disaster recovery VM can be smaller than the production VM to save costs.
SAP systems are network sensitive, so the network system must factor the design decisions into segmenting the VNETs and NSGs. To ensure network reliability, we must use low latency cross-connections with sufficient bandwidth and no packet loss. SAP is very sensitive to these metrics, and you could experience significant issues if traffic suffers latency or packet loss between the application and the SAP system. We can use proximity placement groups called PGS to force the grouping of different VM types into a single Azure data center to optimize the network latency between the different VM types to the best possible.
Security Considerations
Security is another core pillar of any design. Role-based Access control (RBAC) gets accessed through the Azure management bay. RBAC is backed up through AD using cloud-only synchronized identities. Azure AD can back up the RBAC through cloud-only or synchronized identities. RBAC will tie in those cloud or sync identities to Azure tenants, where you can give personal access to Azure for operational purposes. Network security groups are vital for securing the network traffic both within and outside the network environment. The NSGs are stateful firewalls that preserve session information. You can have a single NSG per subnet, and multiple subnets can share the same energy. Application security group or ASG handles functions such as web servers, application servers, or backend database servers combined to perform a meaningful service. Resource encryption brings the best of security with encryption in transit. SAP recommends using encryption at rest, so for the Azure storage account, we can use storage service encryption, which would use either Microsoft or customer-managed keys to manage encryption. Azure storage also adds encryption in transit, with SSL using HTTPS traffic. You can use Azure Disk Encryption (ADE) for OS and DBA encryption for SQL.
Migration of SAP Workloads to Azure
The most critical part of the migration is understanding what you are planning to migrate and accounting for dependencies, limitations, or even blockers that might stop your migration. Following an appropriate inventory process will ensure that your migration completes successfully. You can use in-hand tools to understand the current SAP landscape in the migration scope. For example, looking at your service now or CMDB catalog might reveal some of the data that expresses your SAP system. Then take that information to start drawing out your sizing in Azure. It is essential to ensure that we have a record of the current environment configuration, such as the number of servers and their names, server roles, and data about CPU and memory. It is essential to pick up the disk sizes, configuration, and throughput to ensure that your design delivers a better experience in Azure. It is also necessary to understand database replication and throughput requirements around replicas. When performing a migration, the sizing for large HANA instances is no different from sizing for HANA in general. For existing and deployment systems you want to move from other RDBMS to HANA, SAP provides several reports that run on your existing SAP systems. If migrating the database to HANA, these reports need to check the data and calculate memory requirements for the HANA instances.
When evaluating high availability and disaster recovery requirements, it is essential to consider the implications of choosing between two-tier and three-tier architectures. To avoid network contention in a two-tier arrangement, install database and Netweaver components on the same Azure VM. The database and application components get installed in three-tier configurations on separate Azure Virtual Machines. This choice has other implications regarding sizing since two-tier, and three-tier SAP ratings for a given VM differs. The high availability option is not mandatory for the SAP application servers.
You can achieve high availability by employing redundancy. To implement it, you can install individual application servers on separate Azure VMs. For example, you can achieve high availability for ASCS and SCS servers running on windows using windows failover clustering with SIOS data keeper. We can also achieve high availability with Linux clustering using Azure NetApp files. For DBMS servers, you should use DB replication technology using redundant nodes. Azure offers high availability through redundancy of its infrastructure and capabilities, such as Azure VM restarts, which play an essential role in a single VM deployment. In addition, Azure offers different SLAs depending on your configuration. For example, SAP landscapes organize SABC servers into different tiers; there are three diverse landscapes: deployment, quality assurance, and production.
Migration Strategies:- SAP landscapes to Azure
Enterprises have SAP systems for business functions like Enterprise Resource Planning(ERP), global trade, business intelligence(BI), and others. Within those systems, there are different environments like sandbox developments, tests, and production. Each horizontal row is an environment, and each vertical dimension is the SAP system for a business function. The layers at the bottom are lower-risk environments and are less critical. Those towards the top are in high-risk environments and are more critical. As you move up the stack, there is more risk in the migration process. Production is the more critical environment. The use of test environments for business continuity is of concern. The systems at the bottom are smaller and have fewer computing resources, lower availability, size requirements, and less throughput. They have the same amount of storage as the production database with a horizontal migration strategy. To gain experience with production systems on Azure, you can use a vertical approach with low-risk factors in parallel to the horizontal design.
Horizontal Migration Strategy
To limit risk, start with low-impact sandboxes or training systems. Then, if something goes wrong, there is little danger associated with users or mission-critical business functions. After gaining experience in hosting, running, and administering SAP systems in Azure, apply to the next layer of systems up the stack. Then, estimate costs, limiting expenditures, performance, and optimization potential for each layer and adjust if needed.
Vertical Migration Strategy
The cost must be on guard along with legal requirements. Move systems from the sandbox to production with the lowest risk. First, the governance, risk, compliance system, and the object Event Repository gets driven towards production. Then the higher risk elements like BI and DRP. When you have a new system, it's better to start in Azure default mode rather than putting it on-premises and moving it later. The last system you move is the highest risk, mission-critical system, usually the ERP production system. Having the most performance virtual machines, SQL, and extensive storage would be best. Consider the earliest migration of standalone systems. If you have different SAP systems, always look for upstream and downstream dependencies from one SAP system to another.
Journey to SAP on Azure
Consider two main factors for the migration of SAP HANA to the cloud. The first is the end-of-life first-generation HANA appliance, causing customers to reevaluate their platform. The second is the desire to take advantage of the early value proposition of SAP business warehouse BW on HANA in a flexible DDA model over traditional databases and later BW for HANA. As a result, numerous initial migrations of SAP HANA to Microsoft Azure have focused on SAP BW to take advantage of SAP HANA's in-memory capability for the BW workloads. In addition, using the SAP database migration option DMO with the System Migration option of SUM facilitates single-step migration from the source system on-premises to the target system residing in Azure. As a result, it minimizes the overall downtime. In general, when initiating a project to deploy SAP workloads to Azure, you should divide it into the following phases. Project preparation and planning, pilot, non-production, production preparation, go-live, and post-production.
Use Cases for SAP Implementation in Microsoft Azure
Use cases
How does Microsoft Azure help?
How do organizations benefit?
Deliver automated disaster recovery with low RPO and RTO
Azure recovery services replicate on-premises virtual machines to Azure and orchestrate failover and failback
RPO and RTO get reduced, and the cost of ownership of disaster recovery (DR) infrastructure diminishes. While the DR systems replicate, the only cost incurred is storage
Make timely changes to SAP workloads by development teams
200-300 times faster infrastructure provisioning and rollout compared to on-premises, more rapid changes by SAP application teams
Increased agility and the ability to provision instances within 20 minutes
Fund intermittently used development and test infrastructure for SAP workloads
Supports the potential to stop development and test systems at the end of business day
Savings as much as 40-75 percent in hosting costs by exercising the ability to control instances when not in use
Increase data center capacity to serve updated SAP project requests
Frees on-premises data center capacity by moving development and test for SAP workloads to Microsoft Azure without upfront investments
Flexibility to shift from capital to operational expenditures
Provide consistent training environments based on templates
Ability to store and use pre-defined images of the training environment for updated virtual machines
Cost savings by provisioning only the instances needed for training and then deleting them when the event is complete
Archive historical systems for auditing and governance
Supports migration of physical machines to virtual machines that get activated when needed
Savings of as much as 60 percent due to cheaper storage and the ability to quickly spin up systems based on need.
References
n.d. Microsoft Azure: Cloud Computing Services. Accessed June 13, 2022. http://azure.microsoft.com.
n.d. All Blog Posts. Accessed June 13, 2022. https://blogs.sap.com.
n.d. Cloud4C: Managed Cloud Services for Enterprises. Accessed June 13, 2022. https://www.cloud4c.com.
n.d. NetApp Cloud Solutions | Optimized Storage In Any Cloud. Accessed June 13, 2022. http://cloud.netapp.com.
4 notes
·
View notes
Text
Maximize Success: Transform Your Life with Azure OpenAI
Artificial Intelligence (AI) has evolved significantly. It transitioned from fiction to an integral part of our daily lives and business operations. In business, AI has shifted from a luxury to an essential tool. It helps analyze data, automate tasks, improve customer experiences, and strategize decisions.
McKinsey’s report suggests AI could contribute $13 trillion to the global economy by 2030. Amidst the ever-changing tech landscape, Azure Open AI stands out as an unstoppable force.
In this blog, we’ll delve into the life-changing impact of Azure Open AI features. We’ll explore how its integration can better workflows, enhance decision-making, and drive unparalleled innovation. Join us on a journey to uncover Azure Open AI to reshape business operations in the modern era.
#azure ai#azure open ai desktop#azure open ai pricing#Azure open ai services#azure ai and ml services
1 note
·
View note
Text
Transform Your Business with Custom #Development Using #Azure #AI
1 note
·
View note
Text
Azure AI-102 Training in Hyderabad | Visualpath
Creating and Managing Machine Learning Experiments in Azure AI
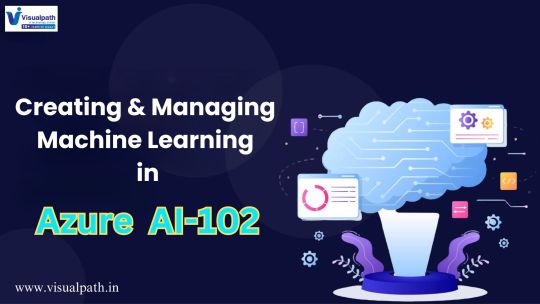
Introduction:
AI 102 Certification is a significant milestone for professionals aiming to design and implement intelligent AI solutions using Azure AI services. This certification demonstrates proficiency in key Azure AI functionalities, including building and managing machine learning models, automating model training, and deploying scalable AI solutions. A critical area covered in the Azure AI Engineer Training is creating and managing machine learning experiments. Understanding how to streamline experiments using Azure's tools ensures AI engineers can develop models efficiently, manage their iterations, and deploy them in real-world scenarios.
Introduction to Azure Machine Learning
Azure AI is a cloud-based platform that provides comprehensive tools for developing, training, and deploying machine learning models. It simplifies the process of building AI applications by offering pre-built services and flexible APIs. Azure Machine Learning (AML), a core component of Azure AI, plays a vital role in managing the entire machine learning lifecycle, from data preparation to model monitoring.
Creating machine learning experiments in Azure involves designing workflows, training models, and tuning hyper parameters. The platform offers both no-code and code-first experiences, allowing users of various expertise levels to build AI models. For those preparing for the AI 102 Certification, learning to navigate Azure Machine Learning Studio and its features is essential. The Studio's drag-and-drop interface enables users to build models without writing extensive code, while more advanced users can take advantage of Python and R programming support for greater flexibility.
Setting Up Machine Learning Experiments in Azure AI
The process of setting up machine learning experiments in Azure begins with defining the experiment's objective, whether it's classification, regression, clustering, or another machine learning task. After identifying the problem, the next step is gathering and preparing the data. Azure AI supports various data formats, including structured, unstructured, and time-series data. Azure’s integration with services like Azure Data Lake and Azure Synapse Analytics provides scalable data storage and processing capabilities, allowing engineers to work with large datasets effectively.
Once the data is ready, it can be imported into Azure Machine Learning Studio. This environment offers several tools for pre-processing data, such as cleaning, normalization, and feature engineering. Pre-processing is a critical step in any machine learning experiment because the quality of the input data significantly affects the performance of the resulting model. Through Azure AI Engineer Training, professionals learn the importance of preparing data effectively and how to use Azure's tools to automate and optimize this process.
Training Machine Learning Models in Azure
Training models is the heart of any machine learning experiment. Azure Machine Learning provides multiple options for training models, including automated machine learning (Auto ML) and custom model training using frameworks like Tensor Flow, PyTorch, and Scikit-learn. Auto ML is particularly useful for users who are new to machine learning, as it automates many of the tasks involved in training a model, such as algorithm selection, feature selection, and hyper parameter tuning. This capability is emphasized in the AI 102 Certification as it allows professionals to efficiently create high-quality models without deep coding expertise.
For those pursuing the AI 102 Certification, it's crucial to understand how to configure training environments and choose appropriate compute resources. Azure offers scalable compute options, such as Azure Kubernetes Service (AKS), Azure Machine Learning Compute, and even GPUs for deep learning models. Engineers can scale their compute resources up or down based on the complexity of the experiment, optimizing both cost and performance.
Managing and Monitoring Machine Learning Experiments
After training a machine learning model, managing the experiment's lifecycle is essential for ensuring the model performs as expected. Azure Machine Learning provides robust experiment management features, including experiment tracking, version control, and model monitoring. These capabilities are crucial for professionals undergoing Azure AI Engineer Training, as they ensure transparency, reproducibility, and scalability in AI projects.
Experiment tracking in Azure allows data scientists to log metrics, parameters, and outputs from their experiments. This feature is particularly important when running multiple experiments simultaneously or iterating on the same model over time. With experiment tracking, engineers can compare different models and configurations, ultimately selecting the model that offers the best performance.
Version control in Azure Machine Learning enables data scientists to manage different versions of their datasets, code, and models. This feature ensures that teams can collaborate on experiments while maintaining a history of changes. It is also crucial for auditability and compliance, especially in industries such as healthcare and finance where regulations require a detailed history of AI model development. For those pursuing the AI 102 Certification, mastering version control in Azure is vital for managing complex AI projects efficiently.
Deploying and Monitoring Models
Once a model has been trained and selected, the next step is deployment. Azure AI simplifies the process of deploying models to various environments, including cloud, edge, and on-premises infrastructure. Through Azure AI Engineer Training, professionals learn how to deploy models using Azure Kubernetes Service (AKS), Azure Container Instances (ACI), and Azure IoT Edge, ensuring that models can be used in a variety of scenarios.
Monitoring also allows engineers to set up automated alerts when a model's performance falls below a certain threshold, ensuring that corrective actions can be taken promptly. For example, engineers can retrain a model with new data to ensure that it continues to perform well in production environments. The ability to manage model deployment and monitoring is a key skill covered in Azure AI Engineer Training, and it is a critical area of focus for the AI 102 Certification.
Best Practices for Managing Machine Learning Experiments
To succeed in creating and managing machine learning experiments, Azure AI engineers must follow best practices that ensure efficiency and scalability. One such practice is implementing continuous integration and continuous deployment (CI/CD) for machine learning models. Azure AI integrates with DevOps tools, enabling teams to automate the deployment of models, manage experiment lifecycles, and streamline collaboration.
Moreover, engineers should optimize the use of computer resources. Azure provides a wide range of virtual machine sizes and configurations, and choosing the right one for each experiment can significantly reduce costs while maintaining performance. Through Azure AI Engineer Training, individuals gain the skills to select the best compute resources for their specific use cases, ensuring cost-effective machine learning experiments.
Conclusion
In conclusion, creating and managing machine learning experiments in Azure AI is a key skill for professionals pursuing the AI 102 Certification. Azure provides a robust platform for building, training, and deploying models, with tools designed to streamline the entire process. From defining the problem and preparing data to training models and monitoring their performance, Azure AI covers every aspect of the machine learning lifecycle.
By mastering these skills through Azure AI Engineer Training, professionals can efficiently manage their AI workflows, optimize model performance, and ensure the scalability of their AI solutions. With the right training and certification, AI engineers are well-equipped to drive innovation in the rapidly growing field of artificial intelligence, delivering value across various industries and solving complex business challenges with cutting-edge technology.
Visualpath is the Best Software Online Training Institute in Hyderabad. Avail complete Azure AI (AI-102) worldwide. You will get the best course at an affordable cost.
Attend Free Demo
Call on - +91-9989971070.
WhatsApp: https://www.whatsapp.com/catalog/919989971070/
Visit: https://www.visualpath.in/online-ai-102-certification.html
#Ai 102 Certification#Azure AI Engineer Certification#Azure AI Engineer Training#Azure AI-102 Course in Hyderabad#Azure AI Engineer Online Training#Microsoft Azure AI Engineer Training#AI-102 Microsoft Azure AI Training
0 notes
Text
The Future of Intelligence: Exploring the Transformative Power of Cloud AI
The world is now a global village, and this is where artificial intelligence (AI) comes in; the intelligent tools that run our daily activities from voice commands to recommendation. Yet, there are numerous organisations and individuals using AI and wishing to do it in future experience various problems such as high levels of infrastructure requirements, need to have specialists in this area, and problems with scaling the processes. And that is where Cloud AI enters a game — a new paradigm of AI as a service that doesn’t require the scale of investments like it used to. Well, what is Cloud AI and why is it revolutionising the way we can regard intelligence? Let’s dive in.
Understanding Cloud AI
In its basic definition, cloud AI is the means of obtaining AI solutions and tools through the usage of cloud solutions. This means that Cloud AI doesn’t need dedicated on-site hardware or a group of data scientists to train models and make AI available to everyone with internet access. Some of the readily available powerful AI tools available in the market are Google Cloud AI, Amazon Web Service AI, Microsoft Azure AI and IBM AI which have simpler forms as API that can be integrated to the systems irrespective of the technologist level of the organization.
Why Cloud AI is a Game Changer
There are three key reasons why Cloud AI is transforming the landscape:
Cost-Effectiveness: Classic AI systems are tremendously computationally intensive which in turn requires large investments in hardware. With Cloud AI, one only pays for the service they employ thus making it affordable. Cloud providers take care of all the issues regarding the hardware and software—no need to worry about it, security and system upgrades included.
Scalability: Whether the user is a small, scrappy startup testing the waters with deep learning, or an established enterprise with millions of users to manage, Cloud AI can be easily scaled up or down to meet the particular user’s needs. It enables organizations to introduce products and services to the market with a level of efficiency that does not consider infrastructure bottlenecks.
Accessibility: One of the major challenges that the adoption of AI has faced is that, it has been realized that it requires expertise. Cloud AI provides ways for adopting complex and powerful AI solutions and pre-configured AI solutions for individuals who do not have programming skills.
Key Applications of Cloud AI
The versatility of Cloud AI is vast, touching numerous industries and transforming business processes. Here are some of the most impactful applications:
Machine Learning (ML) Models
Most AI technologies rely on some form of machine learning, yet constructing and training our models is challenging. Various services that work in the cloud have built-in pre-trained AI models that can be returned as necessary. For instance, when using the Google Cloud, AutoML gives clients an opportunity to create new models for different tasks such as image and text classification through interface, and not through coding. This has ensured that new and small businesses seeking to adopt ML for operations such as customer classification, risk management, and recommendation, can easily do so.
Natural Language Processing (NLP)
It involves Text analysis and Speaking and understanding the language of Humans by The machines. At present, cloud AI services provide dependable NLP tools; thus business solutions enable the components for language translation, sentiment analysis, and text summarization. As applied to customer service this is really helpful – one can think of self-learning chatbots that can handle questions or even analyze customer feedback in the hope of enhancing user satisfaction.
Computer Vision
That way, with Cloud AI, companies can take advantage of such technologies, like computer vision, with less expenses in infrastructure. The main areas of use are face identification, object recognition, as well as video analysis at a higher level. For instance, AWS has an AI service named Rekognition that deals with Images and videos to determine objects, text within images among others. Some of the applications of computer vision include in the retail business, the healthcare business, security firms and even in the creation of concepts that make more personalized customers’ touch points.
Speech Recognition
Speech to text has really evolved and thanks to Cloud AI, it is provided as a service which means its more accessible. Speech recognition can make information from voice and audio transcribed and written text which is helpful for the disabled user and new interfaces. Google Cloud Speech-to-Text for instance is popular for converting audio to text enabling many, from voice assistants to a customer care transcribing service.
Data Analysis and Business Insights
The real-time capability of Cloud AI allows data of large quantities to be run through and analyzed. This capability assists businesses to analyze their data in ways that make it easier to know trends, make analyses on the abnormalities, and make concrete decisions based on the outcome. For example, Cognitive Services of the Microsoft Azure offer analytical tools that may help companies to analyze customer actions, define better prices for their goods and services, or avoid possible inefficiencies.
Benefits of Cloud AI for Businesses
Cloud AI bears several significant benefits that many enterprises will find particularly appealing as the world becomes increasingly reliant on technology.
Speed and Agility: Using Cloud AI, organizations can put in place intelligent solutions faster and are able to follow shifting market demands faster than before. That is why, such sectors as retail companies can employ Cloud AI to analyze customer data and to start precise marketing campaigns during several days.
Data Security and Compliance: Cloud providers still use high measures to steer clear of the international laws of the handling of information, it’s safer for companies to handle sensitive details. They also afford methods for the anonymisation and encryption of data, which can also be useful on their own.
Innovation Opportunities: Cloud AI enables companies to test out new business models without high costs of initial investments associated with these ideas. The applied AI allows firms to iron out any problems that it might possess and develop its potential before investing a great deal of money in it.
Enhanced Customer Experiences: Advancement in artificial intelligence works towards making communications personalised and automated hence enhancing the satisfaction of the customer. For example, such features as proper searching and filtering of the required information and the ability to create intelligent and friendly chatbots can help clients to feel unique, and thus are favorable for the brand.
Challenges and Future of Cloud AI
In the same breath, Cloud AI comes with its own challenges as we will discuss before introducing more information about this AI. Security concerns have not disappeared, and data protection is still a hot issue as more and more businesses collaborate with third parties to store and process their data. Thanks to regulations such as the GDPR coming into play, cloud providers are constantly thinking about compliance, yet, businesses cannot simply rely on such providers to do all the work for them. Another problem is the “lock-in” situation when changing a provider or moving the data becomes painful. To this end, to avoid sticking to one provider, many businesses are using multiple clouds services in different situations. In the next paradigm, the Cloud AI will also have promising growth in the days to come then concepts such as quantum computing, edge IA, and federated learning. These advances will further strengthen Cloud AI and increase its adaptability and security – and open up new opportunities for every industry.
Conclusion
Cloud AI is revolutionizing the capabilities of enterprises and is leading to the mainstream availability of the most sophisticated tools. Cloud AI has a myriad of applications including the training of deep learning models, enhancing customer experience through NLP, among others, that are helping companies deliver technological advancements at higher rates than ever before. This means that as the new generations of AI technologies emerge, the concept of Cloud AI will be instrumental in designing the new world where intellect will be an open resource. To stay relevant and on top of competition, Cloud AI must function as not just a strategy, but an imperative for enterprises. With Cloud AI, companies – no matter if they are a startup or an enterprise – can leverage their data to its full extent and provide their clients with meaningful insights about the world around them. Now is the right time to engage with Cloud AI.
For more information visit = https://cevious.com/
0 notes
Text
Top Tools and Technologies for Fintech App Developers
In the rapidly evolving world of financial technology, staying ahead of the curve is crucial for success. For Fintech app developers, leveraging the right tools and technologies can make the difference between a mediocre application and a revolutionary one. As Fintech continues to transform the Banking, Financial Services, and Insurance (BFSI) sectors, understanding and utilizing the best available resources is essential. This discussion will explore the top tools and technologies that Fintech App Developers should consider creating cutting-edge applications.

Tools and Technologies Fintech App Developers Should Consider:
1. Blockchain Technology
Blockchain technology is revolutionizing the Fintech industry by providing a secure and transparent way to handle transactions. For Fintech app developers, integrating blockchain can enhance security, reduce fraud, and ensure immutable records. Platforms like Ethereum and Hyperledger offer robust frameworks for building decentralized applications (dApps), which are becoming increasingly popular in the Fintech space. By leveraging blockchain, developers can create applications that facilitate peer-to-peer transactions without the need for intermediaries, significantly reducing costs and increasing efficiency.
2. Artificial Intelligence and Machine Learning
Artificial Intelligence (AI) and Machine Learning (ML) are indispensable for Fintech app developers. These technologies enable the creation of intelligent systems capable of analyzing vast amounts of data to provide insights and predictions. AI and ML can be used in various Fintech applications, such as fraud detection, credit scoring, and personalized financial advice. Tools like TensorFlow, Keras, and Scikit-learn are popular among developers for building AI and ML models. By integrating these technologies, Fintech apps can offer enhanced security, improved customer experience, and better financial management solutions.
3. Cloud Computing
Cloud computing has become a cornerstone for Fintech App Development Services. It provides scalable infrastructure, enabling developers to build and deploy applications quickly and efficiently. Services like Amazon Web Services (AWS), Microsoft Azure, and Google Cloud Platform (GCP) offer a range of tools and services that cater specifically to Fintech needs, such as data storage, analytics, and machine learning. By utilizing cloud computing, developers can ensure their applications are scalable, reliable, and secure, meeting the high demands of the Fintech industry.
4. API Integration
Application Programming Interfaces (APIs) are crucial for Fintech app developers as they allow seamless integration with various financial services and systems. APIs enable applications to communicate with each other, facilitating functionalities such as payment processing, data sharing, and customer authentication. Popular APIs in the Fintech space include Plaid, Stripe, and PayPal, which provide robust solutions for connecting banking systems, handling transactions, and managing user data. Effective API integration ensures that Fintech apps can offer a comprehensive range of services, enhancing their functionality and user experience.
5. Cybersecurity Tools
Security is paramount in Fintech app development, given the sensitive nature of financial data. Cybersecurity tools help protect applications from threats such as data breaches, malware, and phishing attacks. Tools like OWASP ZAP, Burp Suite, and Snyk are widely used by Fintech app developers to identify and mitigate vulnerabilities. Additionally, implementing strong encryption protocols, multi-factor authentication, and regular security audits are essential practices to safeguard Fintech applications. By prioritizing cybersecurity, developers can build trust with users and comply with regulatory requirements.
6. Big Data Analytics
Big Data analytics plays a significant role in Fintech app development by providing valuable insights into user behavior, market trends, and financial risks. Tools like Apache Hadoop, Apache Spark, and Tableau help developers process and analyze large datasets efficiently. By leveraging big data, Fintech app developers can create applications that offer personalized financial products, predictive analytics, and improved risk management. These capabilities are critical for enhancing customer engagement and making informed business decisions.
7. Mobile Development Frameworks
With the increasing use of smartphones for financial transactions, mobile development frameworks are essential for Fintech app developers. Frameworks like React Native, Flutter, and Xamarin allow developers to create high-performance mobile applications that run seamlessly on both iOS and Android platforms. These frameworks offer a wide range of pre-built components and libraries, reducing development time and costs. By focusing on mobile-first development, Fintech apps can reach a broader audience and provide a user-friendly experience.
Conclusion
For Fintech app developers, staying updated with the latest tools and technologies is crucial for creating innovative and secure applications. By leveraging blockchain, AI and ML, cloud computing, API integration, cybersecurity tools, big data analytics, and mobile development frameworks, developers can build robust and efficient Fintech solutions. As the Fintech industry continues to grow and evolve, these technologies will play a vital role in shaping the future of financial services. Embracing these tools not only enhances the capabilities of Fintech applications but also ensures they meet the dynamic needs of the BFSI sector.
0 notes
Text
From Legacy to Leader: Modernize Your Apps and Drive Business Growth

At DTC Infotech, we understand the challenges businesses face with legacy applications. These systems, while once reliable, can struggle to keep pace with the ever-evolving digital landscape. Here’s where application modernization comes in — a strategic approach to revitalizing your existing applications to unlock their full potential and empower your business for future success.
Why Modernize Your Applications?
The benefits of application modernization are numerous:
Enhanced Agility and Scalability
Modern cloud-based solutions provide the flexibility to easily scale your applications up or down to meet fluctuating demands. This agility allows you to respond quickly to market changes and seize new opportunities.
Improved Performance and Security
Leverage the latest technologies to optimize application performance and strengthen security measures. This translates to a more reliable and secure user experience.
Reduced Costs
Modernization can lead to significant cost savings by eliminating the need to maintain outdated infrastructure and reducing ongoing support expenses.
Increased Innovation
Modern applications provide a robust foundation for further innovation. By integrating cutting-edge technologies like AI and ML, you can unlock new functionalities and create a more competitive edge.
Our 5-Step Application Modernization Strategy
DTC Infotech offers a comprehensive, yet streamlined, approach to application modernization:
Discovery and Assessment
We begin by collaborating with you to understand your business goals and the specific challenges your legacy applications present. Our team will then conduct a thorough assessment of your applications, identifying areas for improvement and compatibility with modern technologies.
2. Modernization Planning
Based on the assessment findings, we’ll develop a customized modernization plan tailored to your unique needs. This plan will outline the specific modernization approach (rehosting, replat forming, or refactoring) that will deliver the most significant benefits.
3. Remediation and Optimization
Our skilled developers will address any compatibility issues or code inefficiencies identified during the assessment. This remediation ensures a smooth transition to the cloud and optimizes your application for peak performance.
4. Cloud Migration
We leverage the power of Microsoft Azure, a leading cloud platform, to seamlessly migrate your applications. Azure offers a robust set of tools and services that ensure a secure and efficient migration process.
5. Continuous Management and Support
Our commitment extends beyond migration. We provide ongoing support and maintenance to ensure your modernized applications remain secure, optimized, and aligned with your evolving business needs.
Why Choose DTC Infotech for Your Application Modernization?
At DTC Infotech, we combine our deep technical expertise with a passion for understanding your business objectives. We believe in a collaborative approach, working closely with you every step of the way to ensure a successful and value-driven modernization journey.
Ready to Modernize?
Don’t let legacy applications hold your business back. Contact DTC Infotech today for a free consultation and unlock the full potential of your applications. Let’s work together to transform your business for the future!
#cloud computing#Digital Transformation#custom software development#data analytics#it consulting#artificial intelligence#automation#ai#data science
0 notes