#automated process discovery
Explore tagged Tumblr posts
Text
#intelligent process discovery#process discovery#process discovery tools#what is process discovery#process discovery solutions#benefits of process discovery#automated process discovery
0 notes
Text
How Big Data Analytics is Changing Scientific Discoveries
Introduction
In the contemporary world of the prevailing sciences and technologies, big data analytics becomes a powerful agent in such a way that scientific discoveries are being orchestrated. At Techtovio, we explore this renewed approach to reshaping research methodologies for better data interpretation and new insights into its hastening process. Read to continue
#CategoriesScience Explained#Tagsastronomy data analytics#big data analytics#big data automation#big data challenges#big data in healthcare#big data in science#big data privacy#climate data analysis#computational data processing#data analysis in research#data-driven science#environmental research#genomics big data#personalized medicine#predictive modeling in research#real-time scientific insights#scientific data integration#scientific discoveries#Technology#Science#business tech#Adobe cloud#Trends#Nvidia Drive#Analysis#Tech news#Science updates#Digital advancements#Tech trends
1 note
·
View note
Text
Process Discovery
Every Automation development has a lifecycle that begins with the Process Discovery phase and ends with the release and support phase. In which the design, development, testing, deployment, and maintenance phase happens in between.What makes the end product shine? It’s not only the release phase,but the phases that come before releasing the product are equally important. As Gordon B Hinckley…
View On WordPress
0 notes
Text
A fully automated process, including a brand-new artificial intelligence (AI) tool, has successfully detected, identified and classified its first supernova. Developed by an international collaboration led by Northwestern University, the new system automates the entire search for new supernovae across the night sky—effectively removing humans from the process. Not only does this rapidly accelerate the process of analyzing and classifying new supernova candidates, it also bypasses human error. The team alerted the astronomical community to the launch and success of the new tool, called the Bright Transient Survey Bot (BTSbot), this week. In the past six years, humans have spent an estimated total of 2,200 hours visually inspecting and classifying supernova candidates. With the new tool now officially online, researchers can redirect this precious time toward other responsibilities in order to accelerate the pace of discovery.
Continue Reading.
61 notes
·
View notes
Text
Notes on technology in Campoestela:
Most spaceships are single-stage-to-orbit. They have rather standard jet engines to lift off from the ground like a standard plane.
To get into orbit, they use a rocket engine that uses a solid fuel made of a HIGHLY combustible (yet stable) carbon-nitrogen compound which allows a better fuel than anything previous. This was first discovered by Iranian scientists who named it "Nafta".
(sí, Beto tiene que estacionar su camión espacial para cargar nafta)
Nafta was a big discovery on its time, allowing cheap SSTO rockets. Nowadays it's produced in many worlds and widely available. It also has uses as weaponry, but it's not that efficient.
Nafta is used for lift-off and orbital burns. For manuevering in space, there are small jets on the nose and tail of spaceships, similar to the Space Shuttle.
Spaceship piloting is still not an easy task, but it's comparable to being a jet pilot, about 4 or 5 years to master. Hard, but something on the reach of many people. People from the generation ship clans are a bit more used to it and often represent an outsized part of space pilots, but there's always many wellers (from down the gravity well) who get their licenses too.
The hardest thing is always landing. Especially given all the different gravities, atmospheres, orbits and such you have to learn in each different case, even with all the automation in the world. Many spacers feel confident sticking to one or at most two or three planets they know.
Pilots that only do shuttle or cargo runs in the same star system or planet are called "Starters", because they go around the same star. It's rude, but many spacers do it.
FTL travel is another thing. FTL travel is done using a ring-like structure that projects a bubble around the ship and takes it to a (completely made-up for the setting) dimension called the Aether. The Aether is one of the meta-dimensions (there might be more) that uphold reality. Conveniently, you can use it as a shortcut to travel between stars, which project "shadows" on the Aether.
The Aether has its own navigation, with currents and whirpools and areas of thick dark matter (which, for cinematic purposes, actually look like bright nebulae) There are routes that are easier to travel and navigate, and these are where the most visited worlds are. Even stars that are close in real space might be very hard to get in Aetheric space, so there's routes that can take you all over the galaxy in a week, while many other places are out of reach.
Navigating the Aether is very similar to flying a plane through a cloudy sky. Some spacer says it's even easier than flying in real space.
Staying on the aether depends on how much you can keep the fields upholding your "bubble". This depends on the energy of your ship. Big ships can travel all over the galaxy but they have enormous energy consumption requirements.
Smaller ships (such as Beto's Mastropiero) dock with a ring-like structure that allows them to make short jumps. The average jump in an explored route is about 12-48 hours, so it's much like aircraft flights.
Exploring new aetheric routes is something that is very romanticized but in reality is a tedious process of jumping, cataloguing new systems (many of them empty and useful only as refuelling stations), seeing where the streams go and end, how they change, and more.
There is no FTL radio or live communication. There is a kind of aetheric radar that allows you to see incoming ships and do some morse-like communication, but it's not very efficient, there is no such thing as a galactic internet (though it's said ancient civilizations had one)
Aether travel engines require very sophisticated manufacturing and materials, which were hard for humans to develop. This was long only in the hands of governments and corporations, but after the Machine War, accessible aether starships hit the civilian market.
Smaller ships are still used by governments (more like loose "leagues") to do what big ships can't: supply satellites and equipment to remote bases, small-scale transport of engineers, researchers, aether "meteorology" and exploration, etc. This is very much like bush planes in remote regions or the role of Aeroflot in developing the USSR.
While humans in the setting, like most species, are composed of many different leagues, cultures and organizations, their technology is remarkably consistent. This is because cheap and reliable spaceflight depends on very reliable standarization. Some of the spaceship parts used six centuries after Gagarin are still the same used in the Soyuz. The ISO is perhaps one of the most enduring legacies of human civilization, along with FIFA.
#campoestela#science fiction#worldbuilding#cosas mias#I might go on later on but I'm tired#biotipo worldbuilding
15 notes
·
View notes
Text
Kopos Kos 🪿 for your travels
(wip content)
Kopos Kos, a proud and quickly developing nation located across what we know as Vietnam and Cambodia. The current emblem is definitely not my proudest work😓
The bird featured is a great cormorant, domesticated by our sophont wallabies (now called Walaba), used to aid in fishing to supply the Kepa-ka-o-o populations which base a lot of seafood in their diet as opposed to Walaba whom are completely herbivorous. The great cormorant represents initiative, resourcefulness and union between peoples, and is worn as a symbol of patriotism in Kopos Kos.
Kopos Kos was originally established as a farming colony, taking advantage of tropical soils and the abundance of wildlife but quickly established itself as a trade center, bringing in many Lau merchants and immigrants who began making their own settlements. The name Kopos Kos originally referred to a single town, though it is now a giant web of interconnected cities and villages across large stretches of land. By human terms it would be a country, however this concept doesn't yet exist on Beatha.
Because Walaba are herbivorous, livestock is generally uneeded in terms of meat, however domestic animals remain a common sight. The water pig, the equivalent of a Central Asian boar, is a new discovery that has been quickly integrated into agricultural life.
While many wild boars are aggressive when threatened or nervous, this domestic species tends to be docile and flees rather than fights if it comes down to it. Water pigs live in forests and jungles, preferring darker environments. Because of their excellent sense of smell, they are particularly good at locating food and water sources in areas that would be almost impossible to navigate through if you were unfamiliar with them, proving helpful to early settlers who often found the pigs near streams which lent them their name. Coincidentally, it was later discovered they have an excellent swimming ability. In Kopos Kos, water pigs are kept for their manure which is an incredibly efficient fertiliser for crops. The pigs also dig up root vegetables, and can be trained not to eat the roots and allow an owner to collect them instead. This process also aerates soil, which means automated tilling of small fields. Of course, even if they're not kept for meat, water pigs will eventually die. The skin can be harvested for leather, bones for jewellery and tools, hair for clothing and paintbrushes, etc, while the meat is sold off to Kepa-ka-o-o and Lau or used as food for meat eating domestic animals.
#Beatha#spec bio#spec evo#speculative biology#speculative evolution#digital art#art#speculative zoology#original species#this should be taken as a given but ignore what any words mean in other languages. olive oil or whatever
38 notes
·
View notes
Text
30 notes
·
View notes
Text
AI & IT'S IMPACT
Unleashing the Power: The Impact of AI Across Industries and Future Frontiers
Artificial Intelligence (AI), once confined to the realm of science fiction, has rapidly become a transformative force across diverse industries. Its influence is reshaping the landscape of how businesses operate, innovate, and interact with their stakeholders. As we navigate the current impact of AI and peer into the future, it's evident that the capabilities of this technology are poised to reach unprecedented heights.
1. Healthcare:
In the healthcare sector, AI is a game-changer, revolutionizing diagnostics, treatment plans, and patient care. Machine learning algorithms analyze vast datasets to identify patterns, aiding in early disease detection. AI-driven robotic surgery is enhancing precision, reducing recovery times, and minimizing risks. Personalized medicine, powered by AI, tailors treatments based on an individual's genetic makeup, optimizing therapeutic outcomes.
2. Finance:
AI is reshaping the financial industry by enhancing efficiency, risk management, and customer experiences. Algorithms analyze market trends, enabling quicker and more accurate investment decisions. Chatbots and virtual assistants powered by AI streamline customer interactions, providing real-time assistance. Fraud detection algorithms work tirelessly to identify suspicious activities, bolstering security measures in online transactions.
3. Manufacturing:
In manufacturing, AI is optimizing production processes through predictive maintenance and quality control. Smart factories leverage AI to monitor equipment health, reducing downtime by predicting potential failures. Robots and autonomous systems, guided by AI, enhance precision and efficiency in tasks ranging from assembly lines to logistics. This not only increases productivity but also contributes to safer working environments.
4. Education:
AI is reshaping the educational landscape by personalizing learning experiences. Adaptive learning platforms use AI algorithms to tailor educational content to individual student needs, fostering better comprehension and engagement. AI-driven tools also assist educators in grading, administrative tasks, and provide insights into student performance, allowing for more effective teaching strategies.
5. Retail:
In the retail sector, AI is transforming customer experiences through personalized recommendations and efficient supply chain management. Recommendation engines analyze customer preferences, providing targeted product suggestions. AI-powered chatbots handle customer queries, offering real-time assistance. Inventory management is optimized through predictive analytics, reducing waste and ensuring products are readily available.
6. Future Frontiers:
A. Autonomous Vehicles: The future of transportation lies in AI-driven autonomous vehicles. From self-driving cars to automated drones, AI algorithms navigate and respond to dynamic environments, ensuring safer and more efficient transportation. This technology holds the promise of reducing accidents, alleviating traffic congestion, and redefining mobility.
B. Quantum Computing: As AI algorithms become more complex, the need for advanced computing capabilities grows. Quantucm omputing, with its ability to process vast amounts of data at unprecedented speeds, holds the potential to revolutionize AI. This synergy could unlock new possibilities in solving complex problems, ranging from drug discovery to climate modeling.
C. AI in Creativity: AI is not limited to data-driven tasks; it's also making inroads into the realm of creativity. AI-generated art, music, and content are gaining recognition. Future developments may see AI collaborating with human creators, pushing the boundaries of what is possible in fields traditionally associated with human ingenuity.
In conclusion, the impact of AI across industries is profound and multifaceted. From enhancing efficiency and precision to revolutionizing how we approach complex challenges, AI is at the forefront of innovation. The future capabilities of AI hold the promise of even greater advancements, ushering in an era where the boundaries of what is achievable continue to expand. As businesses and industries continue to embrace and adapt to these transformative technologies, the synergy between human intelligence and artificial intelligence will undoubtedly shape a future defined by unprecedented possibilities.
19 notes
·
View notes
Text
Michael Esposito Staten Island: Innovative AI Solutions for Influencer Marketing in the Digital Age
In the ever-evolving landscape of digital marketing, influencer marketing has emerged as a powerful strategy for brands to connect with their target audience and drive engagement. With the rise of social media platforms, influencers have become key players in shaping consumer preferences and purchasing decisions. Michael Esposito Staten Island — Influence in the Digital Age exemplifies this trend, highlighting how digital influencers can significantly impact marketing strategies and outcomes. However, as the digital space becomes increasingly saturated with content, brands are turning to innovative AI solutions to enhance their influencer marketing efforts and stay ahead of the curve.
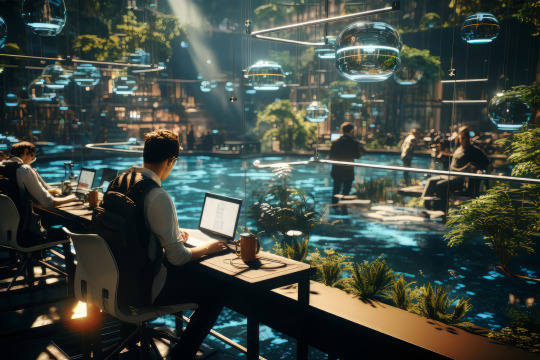
AI-Powered Influencer Discovery
One of the biggest challenges brands face in influencer marketing is finding the right influencers to collaborate with. Traditional methods of influencer discovery often involve manual research and outreach, which can be time-consuming and inefficient. However, AI-powered influencer discovery platforms leverage advanced algorithms to analyze vast amounts of data and identify influencers who are the best fit for a brand's target audience and campaign objectives. Michael Esposito Staten Island: An Influencer Marketer Extraordinaire, exemplifies how effective influencer collaboration can transform marketing strategies. By harnessing the power of AI, brands can streamline the influencer discovery process and identify high-potential collaborators with greater accuracy and efficiency.
Predictive Analytics for Campaign Optimization
Once influencers have been identified and partnerships established, brands can leverage AI-powered predictive analytics to optimize their influencer marketing campaigns. Predictive analytics algorithms analyze historical campaign data, audience demographics, and engagement metrics to forecast the performance of future campaigns. By leveraging these insights, brands can make data-driven decisions about content strategy, audience targeting, and campaign optimization, maximizing the impact of their influencer collaborations and driving measurable results.
AI-Driven Content Creation
Content creation is a critical component of influencer marketing campaigns, and AI is revolutionizing the way brands create and optimize content for maximum impact. AI-powered content creation tools can generate personalized, high-quality content at scale, helping brands maintain a consistent brand voice and aesthetic across their influencer collaborations. From automated image and video editing to natural language processing for caption generation, AI-driven content creation tools empower brands to create compelling, on-brand content that resonates with their target audience and drives engagement.
Sentiment Analysis for Brand Monitoring
Influencer marketing campaigns can have a significant impact on brand perception, and it's essential for brands to monitor and manage their online reputation effectively. AI-powered sentiment analysis tools analyze social media conversations and user-generated content to gauge public sentiment towards a brand or campaign. By tracking mentions, sentiment trends, and key themes, brands can quickly identify and address any potential issues or negative feedback, allowing them to proactively manage their brand reputation and maintain a positive online presence.
Automated Performance Reporting
Measuring the success of influencer marketing campaigns is crucial for determining ROI and informing future strategies. However, manual performance reporting can be time-consuming and prone to human error. AI-powered analytics platforms automate the process of performance reporting by aggregating data from multiple sources, analyzing key metrics, and generating comprehensive reports in real-time. By providing brands with actionable insights into campaign performance, audience engagement, and ROI, AI-driven analytics platforms enable brands to optimize their influencer marketing efforts and drive continuous improvement.
In conclusion, as influencer marketing continues to evolve in the digital age, brands must leverage innovative AI solutions to stay competitive and maximize the impact of their campaigns. From AI-powered influencer discovery and predictive analytics to automated content creation and sentiment analysis, AI is revolutionizing every aspect of influencer marketing, enabling brands to connect with their target audience more effectively and drive measurable results. By embracing these innovative AI solutions, brands can unlock the full potential of influencer marketing and achieve success in the digital era.
10 notes
·
View notes
Text

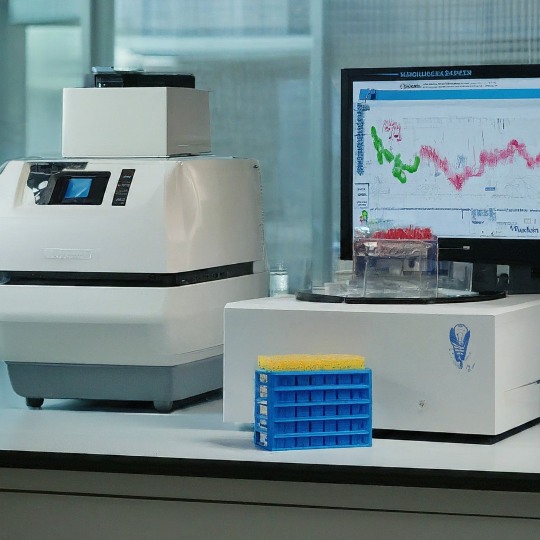
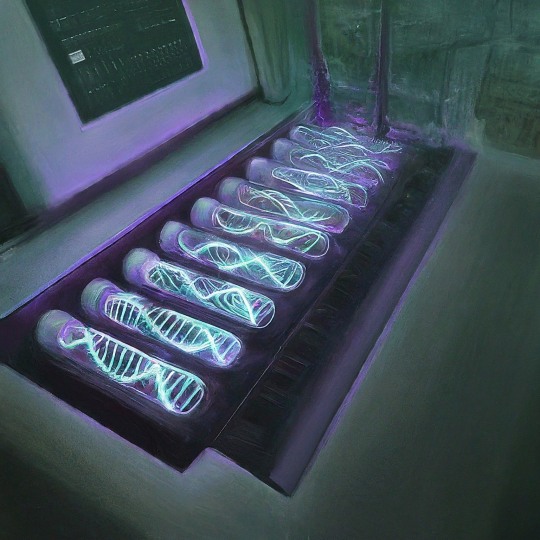
Amplifying Revolution: The Polymerase Chain Reaction (PCR)
Imagine a scenario where you have a crucial document, but there's only one fragile copy. You need numerous duplicates to analyze and share. This is exactly the challenge faced by scientists dealing with DNA. Thankfully, a revolutionary technique called Polymerase Chain Reaction (PCR) comes to the rescue. PCR, often referred to as molecular photocopying, is a fundamental tool in molecular biology. It allows scientists to exponentially amplify a specific DNA segment, creating millions of copies from a minuscule sample. This has revolutionized various fields, from diagnosing diseases to unraveling genetic mysteries.
The credit for inventing PCR is widely attributed to Kary Mullis, a biochemist working at Cetus Corporation in the early 1980s. Inspired by his nighttime drives through California, Mullis envisioned a method for exponentially copying DNA segments through repeated cycles of heating, annealing (primer attachment), and extension (polymerase-mediated DNA synthesis). This elegant concept became the foundation of PCR. Mullis's concept was brilliant, but a crucial hurdle remained. The process required a DNA polymerase enzyme that could withstand repeated heating and cooling cycles. The solution came from an unexpected source: hot springs. In 1976, researchers discovered Taq polymerase, a heat-stable enzyme isolated from the thermophilic bacterium Thermus aquaticus. This discovery was a game-changer, as Taq polymerase could function optimally during the high-temperature steps of PCR. In recognition of its transformative impact on science, Kary Mullis was awarded the Nobel Prize in Chemistry in 1993, alongside Michael Smith, who pioneered site-directed mutagenesis.
While the core concept of PCR was established, the technique required further refinement. Pioneering researchers like Henry Erlich at Cetus played a vital role in optimizing reaction conditions, automating the process, and developing the now-ubiquitous thermal cyclers that precisely control the temperature changes needed for PCR. The 1980s and 1990s witnessed a surge in PCR applications. In 1985, PCR was used for the first time to analyze sickle cell anemia, demonstrating its potential for clinical diagnostics. Forensic science embraced PCR in 1987, with the successful amplification of DNA from a single human hair. By 1989, highly sensitive DNA fingerprinting techniques based on PCR became a game-changer in criminal investigations.
At the heart of PCR lies a clever exploitation of the natural process of DNA replication. The key players in this drama are:
Template DNA: The DNA sequence that contains the target region to be amplified
Primers: Short sequences of nucleotides that flank the target DNA region and serve as starting points for DNA synthesis.
DNA Polymerase: Enzyme responsible for synthesizing new DNA strands by extending the primers using nucleotides.
Nucleotides: The building blocks of DNA, including adenine (A), thymine (T), cytosine (C), and guanine (G).
Buffer Solution: Provides optimal conditions for the enzymatic reactions to occur.
Thermal Cycler: Instrumentation used to automate the PCR process by cycling through different temperatures.
At its core, PCR mimics the natural process of DNA replication within an organism. However, PCR condenses this complex process into a series of controlled steps carried out within a test tube. Here's a breakdown of the PCR cycle:
Denaturation: The first step involves heating the reaction mixture to a high temperature (usually around 95°C), causing the double-stranded DNA to separate into two single strands. This process is known as denaturation.
Annealing: The temperature is then lowered to allow the primers to bind (anneal) to their complementary sequences on the single-stranded DNA. This typically occurs around 50-65°C, depending on the primer sequences.
Extension: With the primers bound, the temperature is raised again, and DNA polymerase synthesizes new DNA strands by extending from the primers using the nucleotides present in the reaction mixture. This step occurs at a temperature optimal for the DNA polymerase enzyme, typically around 72°C.
Cycle Repetition: These three steps—denaturation, annealing, and extension—are repeated multiple times (usually 20-40 cycles), resulting in an exponential increase in the number of DNA copies. Each cycle doubles the amount of DNA, leading to millions of copies of the target sequence after just a few cycles.
The beauty of PCR lies in its repetitive nature. With each cycle, the number of copies of the target DNA segment doubles. After 30 cycles, for example, you can have billions of copies of the specific DNA region, enough for further analysis.
This versatile technique has spawned numerous variations, each tailored for a specific purpose. Let's delve into some of the most common types of PCR:
Real-Time PCR (qPCR): Real-Time PCR, or quantitative PCR (qPCR), revolutionized nucleic acid quantification by enabling the real-time monitoring of DNA amplification. This technique utilizes fluorescent reporter molecules to measure the accumulation of PCR products during each cycle. qPCR is invaluable in gene expression analysis, microbial quantification, and diagnostic assays due to its high sensitivity and quantitative capabilities.
Reverse Transcription PCR (RT-PCR): Reverse Transcription PCR combines PCR with reverse transcription to amplify RNA sequences. This technique converts RNA into complementary DNA (cDNA) using reverse transcriptase enzyme before proceeding with PCR amplification. RT-PCR is pivotal in gene expression studies, viral load quantification, and the detection of RNA viruses such as HIV and SARS-CoV-2.
Nested PCR: Nested PCR involves two rounds of amplification, with the second round using a set of nested primers that bind within the product of the first round. This nested approach increases specificity and reduces nonspecific amplification, making it ideal for detecting low-abundance targets and minimizing contamination. Nested PCR is commonly used in forensic analysis, pathogen detection, and rare allele identification.
Multiplex PCR: Multiplex PCR allows simultaneous amplification of multiple target sequences within a single reaction. This technique employs multiple primer sets, each specific to a distinct target region, enabling the detection of multiple targets in a single assay. Multiplex PCR is valuable in microbial typing, genetic screening, and detection of pathogens with complex genetic profiles.
Digital PCR (dPCR): Digital PCR partitions the PCR reaction into thousands of individual micro-reactions, each containing a single DNA template molecule or none at all. By counting the number of positive and negative partitions, dPCR accurately quantifies target DNA molecules without the need for standard curves or reference samples. This technique is useful for absolute quantification of rare targets, allelic discrimination, and copy number variation analysis.
Allele-Specific PCR: Allele-Specific PCR selectively amplifies alleles containing specific nucleotide variations, enabling the detection of single nucleotide polymorphisms (SNPs) or mutations. This technique utilizes primers designed to match the target sequence with single-base mismatches at their 3' end, allowing discrimination between different alleles. Allele-Specific PCR finds applications in genetic testing, pharmacogenomics, and population studies.
PCR's ability to amplify DNA has made it an indispensable tool in various fields. Here are a few examples of its diverse applications:
Disease Diagnosis and Surveillance: PCR plays a pivotal role in the rapid and accurate diagnosis of infectious diseases. By amplifying specific nucleic acid sequences, PCR enables the detection of pathogens with high sensitivity and specificity. PCR-based tests have become indispensable in diagnosing viral infections such as HIV, hepatitis, influenza, and COVID-19. Additionally, PCR facilitates the surveillance of disease outbreaks and the monitoring of antimicrobial resistance.
Genetic Testing and Personalized Medicine: PCR empowers genetic testing by enabling the detection of genetic mutations, polymorphisms, and variations associated with inherited diseases, cancer, and pharmacogenomics. Through techniques like allele-specific PCR and real-time PCR, researchers can identify disease-causing mutations, assess drug efficacy, and tailor treatments to individual patients. PCR-based genetic tests have transformed healthcare by enabling early disease detection, risk assessment, and personalized therapeutic interventions.
Forensic Analysis and DNA Profiling: PCR has revolutionized forensic science by enabling the analysis of minute DNA samples collected from crime scenes. Techniques like short tandem repeat (STR) analysis and multiplex PCR allow forensic experts to generate DNA profiles with high resolution and accuracy. PCR-based DNA profiling is used in criminal investigations, paternity testing, disaster victim identification, and wildlife forensics, contributing to the administration of justice and conservation efforts worldwide.
Environmental Monitoring and Microbial Ecology: PCR facilitates the study of microbial communities in diverse environments, including soil, water, air, and the human microbiome. Environmental DNA (eDNA) analysis using PCR-based methods enables the detection and characterization of microbial species, including bacteria, fungi, and archaea. PCR-based assays are employed in environmental monitoring, food safety testing, and microbial source tracking, aiding in the preservation of ecosystems and public health.
Agricultural Biotechnology and Food Safety: PCR plays a vital role in agricultural biotechnology by enabling the detection of genetically modified organisms (GMOs), plant pathogens, and foodborne pathogens. PCR-based assays are used to verify the authenticity and safety of food products, detect allergens, and monitor the presence of contaminants such as pesticides and toxins. PCR-based technologies contribute to ensuring food security, quality control, and regulatory compliance in the food industry.
Evolutionary Biology and Phylogenetics: PCR-based methods are indispensable tools for studying evolutionary relationships and biodiversity. Techniques like DNA barcoding and metagenomics employ PCR to amplify and analyze DNA sequences from diverse organisms, elucidating their evolutionary history and ecological interactions. PCR facilitates the identification of new species, the study of population genetics, and the conservation of endangered species, enriching our understanding of the natural world.
PCR's versatility and precision make it indispensable in unlocking the secrets of genetics and unraveling complex biological mysteries. Its ability to amplify minute DNA samples with remarkable speed and accuracy has opened doors to countless possibilities in research and diagnostics. s we delve deeper into the intricacies of the genetic world, PCR will undoubtedly remain a powerful tool for unlocking the secrets of life itself.
#science sculpt#life science#science#molecular biology#biology#biotechnology#artists on tumblr#double helix#genetics#dna#polymerase chain reaction#medical science#the more you know#scientific research#scifiart#scientific advancements#scientific illustration#scientific instruments#scientific discovery
7 notes
·
View notes
Text
Revising a statement I wrote years ago. It both uses and addresses AI in the context of learning and thinking.
“If we value independence, if we are disturbed by the growing conformity of knowledge, of values, of attitudes, which our present system induces, then we may wish to set up conditions of learning which make for uniqueness, for self-direction, and for self-initiated learning.” — Carl Rogers “The conditions of a true critique and a true creation are the same: the destruction of an image of thought which presupposes itself and the genesis of the act of thinking in thought itself.” — Gilles Deleuze, Difference & Repetition
Thinking my own thoughts is often a struggle, but it is a struggle worth having. This struggle pushes against the forces of standardization, conformity, and passivity that pervade so much of modern life. I find myself asking: am I truly thinking, or merely going through the motions? I pose the same question to everyone I work with—collaborators, peers, students, and friends—encouraging them to resist the easy paths of imitation and regurgitation. The moments I value most are when people surprise me—and, even more, when they surprise themselves—with ideas or creations that challenge the boundaries of their thinking.
This struggle for independent thought faces new challenges and opportunities in the age of artificial intelligence and machine learning. Depending on how these tools are used and understood, they risk accelerating conformity and standardization, automating not only tasks but ways of thinking. If we reinforce an image of thought that presupposes itself, AI may close off opportunities for genuine rethinking. And yet, as Doug Engelbart envisioned with his concept of augmented intelligence, the true potential of these technologies lies not in replacing human thought but in amplifying it. Engelbart imagined tools that extend human capacities—helping us think more deeply, solve complex problems, and collaborate effectively. Learning, in this context, becomes a process where people partner with technology, harnessing its strengths while cultivating their own uniquely human qualities: curiosity, empathy, and imagination.
My project, Another Human in the Loop, reflects this philosophy. It explores how humans and AI can collaborate in ways that deepen creative processes, using AI not as a passive tool but as an active participant in generating new possibilities. Through this work, I’ve come to see that we must develop not only critical thinking and technical skills but also the ability to ask fundamental questions: How can AI challenge us to think differently? How do we avoid being constrained by the biases and patterns it reinforces? How do we maintain a spirit of play, wonder, and discovery in an age of algorithmic efficiency?
As Engelbart understood, the tools we use shape how we think, and the process of learning must prepare us to shape our tools in turn. This requires fostering a mindset of exploration and adaptability. I aim to create environments where distinctions between teacher, student, artist, and researcher blur—where we think with one another, not for one another, and where technology becomes a catalyst for deeper inquiry rather than a substitute for creativity.
At the heart of my practice is the belief that learning is a shared endeavor through which we become not only better thinkers and makers but more fully human: empathetic, adaptable, and imaginative. I encourage everyone to embrace the awkwardness of first steps and the uncertainty of experimentation, for these are the moments when true learning happens—when we surprise ourselves by creating something we never thought possible.
Learning, like augmented intelligence, thrives in collaboration. While AI and online tools provide powerful avenues for sharing information, the most meaningful discoveries often happen in shared presence. They occur in the quiet spaces where ideas take root, in the sparks of collective discovery, and in the ongoing dialogue of making and re-making. My role is to catalyze and coordinate a community of experimenters who see themselves as learners and creators, willing to challenge assumptions and embrace new possibilities.
Together, we can reimagine what it means to learn, think, and create in the 21st century. By integrating Engelbart’s vision, we move toward a model of learning that doesn’t merely adapt to technological change but actively shapes it—empowering us to harness technology as a force for growth, creativity, and connection.
2 notes
·
View notes
Text
#process discovery#what is process discovery#automated process discovery#benefits of process discovery
0 notes
Text
Sad that blogs as a method of music proliferation have largely went extinct. The main methods now as I see are social media marketing and gaming/working the algorithm of streaming and social sites. The loss of word of mouth as a prevalent discovery medium has led to the dissolution of being keen to obscure gems (that made up 2000s hipsterdom), now relying on algorithmic, technologically-aided taste-making.
There's no denying the algorithms have become excellent at "matching the mood". I use it frequently with Apple Music (or Spotify, I change between them every so often). With just the right amount of (perhaps also personally determined) eclecticism in automated track selection. Sadly, however, it seems that having your perspective or preconceptions jolted by a left-field or otherwise radically different sound is much less frequent. But I suppose that's what's wanted by the majority.
Or perhaps this is also accounted for in the algorithms. Those who would in previous years be blog-searching, obscured gem-finders are detected by the algos and are given sprinklings of oddities to satisfy their spirit of exploration and discovery.. In the same way on YouTube I'd be suggested seemingly random channels with no subscribers or hits at all.
Nonetheless, the art of blog-curation has gone the wayside in favor of an automated process. Despite however effective that may be in action, it is ultimately a technological outsourcing and to me represents a loss in the human excitement and beautiful imperfections of creating and sharing art.
3 notes
·
View notes
Text
What is Customer Analytics? – The Importance of Understanding It
Consumers have clear expectations when selecting products or services. Business leaders need to understand what influences customer decisions. By leveraging advanced analytics and engaging in data analytics consulting, they can pinpoint these factors and improve customer experiences to boost client retention. This article will explore the importance of customer analytics.
Understanding Customer Analytics
Customer analytics involves applying computer science, statistical modeling, and consumer psychology to uncover the logical and emotional drivers behind consumer behavior. Businesses and sales teams can work with a customer analytics company to refine customer journey maps, leading to better conversion rates and higher profit margins. Furthermore, they can identify disliked product features, allowing them to improve or remove underperforming products and services.
Advanced statistical methods and machine learning (ML) models provide deeper insights into customer behavior, reducing the need for extensive documentation and trend analysis.
Why Customer Analytics is Essential
Reason 1 — Boosting Sales
Insights into consumer behavior help marketing, sales, and CRM teams attract more customers through effective advertisements, customer journey maps, and post-purchase support. Additionally, these insights, provided through data analytics consulting, can refine pricing and product innovation strategies, leading to improved sales outcomes.
Reason 2 — Automation
Advances in advanced analytics services have enhanced the use of ML models for evaluating customer sentiment, making pattern discovery more efficient. Consequently, manual efforts are now more manageable, as ML and AI facilitate automated behavioral insight extraction.
Reason 3 — Enhancing Long-Term Customer Relationships
Analytical models help identify the best experiences to strengthen customers’ positive associations with your brand. This results in better reception, positive word-of-mouth, and increased likelihood of customers reaching out to your support team rather than switching to competitors.
Reason 4 — Accurate Sales and Revenue Forecasting
Analytics reveal seasonal variations in consumer demand, impacting product lines or service packages. Data-driven financial projections, supported by data analytics consulting, become more reliable, helping corporations adjust production capacity to optimize their average revenue per user (ARPU).
Reason 5 — Reducing Costs
Cost per acquisition (CPA) measures the expense of acquiring a customer. A decrease in CPA signifies that conversions are becoming more cost-effective. Customer analytics solutions can enhance brand awareness and improve CPA. Benchmarking against historical CPA trends and experimenting with different acquisition strategies can help address inefficiencies and optimize marketing spend.
Reason 6 — Product Improvements
Customer analytics provides insights into features that can enhance engagement and satisfaction. Understanding why customers switch due to missing features or performance issues allows production and design teams to identify opportunities for innovation.
Reason 7 — Optimizing the Customer Journey
A customer journey map outlines all interaction points across sales funnels, complaint resolutions, and loyalty programs. Customer analytics helps prioritize these touchpoints based on their impact on engaging, retaining, and satisfying customers. Address risks such as payment issues or helpdesk errors by refining processes or implementing better CRM systems.
Conclusion
Understanding the importance of customer analytics is crucial for modern businesses. It offers significant benefits, including enhancing customer experience (CX), driving sales growth, and preventing revenue loss. Implementing effective strategies for CPA reduction and product performance is essential, along with exploring automation-compatible solutions to boost productivity. Customer insights drive optimization and brand loyalty, making collaboration with experienced analysts and engaging in data analytics consulting a valuable asset in overcoming inefficiencies in marketing, sales, and CRM.
3 notes
·
View notes
Text
How is AI transforming every aspect of human life?
AI is transforming every aspect of human life by revolutionizing the way we work, communicate, learn, and live. Here are some key areas where AI is making a significant impact:
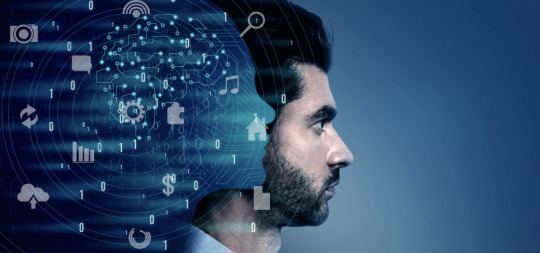
What is Artificial Intelligence?
Artificial Intelligence (AI) refers to the simulation of human intelligence in machines that can perform tasks requiring human-like cognitive abilities. It involves machine learning, natural language processing, computer vision, and other advanced techniques.
How does it impact every industry?
AI has the potential to revolutionize every industry by automating processes, analyzing vast amounts of data, and making intelligent predictions. It improves efficiency, enhances decision-making, and drives innovation across sectors such as healthcare, finance, manufacturing, and transportation.
How does it impact every individual?
AI impacts individuals by providing personalized experiences, virtual assistants, and smart devices. It enhances daily life through voice recognition, recommendation systems, and virtual customer support. AI-powered technologies make our lives easier, more convenient, and efficient.
AI is transforming every aspect of human life by revolutionizing the way we work, communicate, learn, and live. Here are some key areas where AI is making a significant impact:
1. Healthcare:
AI is enhancing medical diagnosis, drug discovery, and personalized treatment plans. It helps analyze vast amounts of patient data, identify patterns, and provide accurate predictions for disease prevention and early intervention.
According to Accenture, AI in healthcare could potentially save up to $150 billion annually for the U.S. healthcare economy by 2026.
The global AI in healthcare market is projected to reach $45.2 billion by 2026, growing at a compound annual growth rate (CAGR) of 44.9% from 2019 to 2026.
2. Education:
AI is revolutionizing education by enabling personalized learning experiences, adaptive tutoring, and intelligent assessment systems. It helps tailor educational content to individual student needs, track progress, and provide timely feedback for better learning outcomes.
The global AI in education market is expected to reach $3.68 billion by 2025, with a CAGR of 38.17% from 2018 to 2025.
A study by the American Institutes for Research found that AI-powered tutoring systems have a positive impact on student learning outcomes, resulting in an average percentile gain of 28 points.
3. Transportation:
AI is driving advancements in autonomous vehicles, optimizing traffic management systems, and improving transportation efficiency and safety. It enables self-driving cars, real-time navigation, and predictive maintenance, revolutionizing the way we commute and travel.
The global autonomous vehicle market is projected to reach $556.67 billion by 2026, with a CAGR of 39.47% from 2019 to 2026.
According to the National Highway Traffic Safety Administration, AI-powered advanced driver-assistance systems (ADAS) have the potential to reduce traffic fatalities by up to 94%.
4. Communication:
AI-powered language translation, natural language processing, and speech recognition technologies are transforming communication. Chatbots, virtual assistants, and language translation tools facilitate seamless cross-cultural communication and enhance accessibility.
The global AI in communication market is expected to reach $3.5 billion by 2026, growing at a CAGR of 34.7% from 2019 to 2026.
AI-powered language translation technologies have advanced significantly, with Google Translate handling more than 100 billion words daily in over 100 languages.
Virtual assistants like Siri, Alexa, and Google Assistant leverage AI to understand and respond to user commands, making voice-based communication more convenient and efficient.
5. Entertainment:
AI is reshaping the entertainment industry with personalized content recommendations, virtual reality experiences, and computer-generated imagery. It enhances user experiences, facilitates content curation, and enables immersive storytelling.
The global AI in the entertainment market is projected to reach $5.5 billion by 2026, with a CAGR of 25.4% from 2019 to 2026.
AI algorithms are used in content recommendation systems of streaming platforms like Netflix and Spotify, which account for a significant portion of their user engagement and revenue.
AI-powered computer-generated imagery (CGI) has transformed the visual effects industry, enabling the creation of realistic and immersive experiences in movies, video games, and virtual reality.
6. Finance:
AI is revolutionizing the financial industry with automated trading, fraud detection, risk assessment, and personalized financial advice. It enables efficient data analysis, real-time market insights, and improved decision-making processes.
A report by PwC estimates that AI could contribute up to $15.7 trillion to the global economy by 2030, with the financial sector being one of the largest beneficiaries.
AI-driven automated investment platforms, also known as robo-advisors, managed over $1 trillion in assets globally in 2020.
7. Smart Homes:
AI-powered smart home devices and virtual assistants, such as voice-activated speakers and smart thermostats, make our daily lives more convenient and efficient. They automate tasks, provide personalized recommendations, and create a connected and intelligent living environment.
The global smart home market is expected to reach $246.97 billion by 2027, with a CAGR of 11.6% from 2020 to 2027.
Voice-activated smart speakers, powered by AI assistants like Amazon Alexa and Google Assistant, have seen widespread adoption. As of 2021, there were over 200 million smart speakers in use worldwide.
8. Manufacturing:
AI-driven robotics and automation technologies optimize manufacturing processes, increase productivity, and improve product quality. AI-enabled machines and robots perform complex tasks, enhance precision, and enable predictive maintenance.
The global AI in manufacturing market is expected to reach $16.7 billion by 2026, growing at a CAGR of 49.5% from 2019 to 2026.
According to Deloitte, companies that invest in AI and advanced automation technologies in manufacturing can experience productivity gains of up to 30%.
AI-powered predictive maintenance can reduce equipment downtime by up to 50% and maintenance costs by up to 10-40%.
9. Agriculture:
AI is transforming agriculture by optimizing crop management, monitoring soil conditions, and predicting weather patterns. It enables precision farming techniques, reduces resource waste, and improves agricultural productivity.
The global AI in agriculture market is projected to reach $4 billion by 2026, with a CAGR of 22.5% from 2021 to 2026.
AI-powered agricultural robots and drones are expected to reach a market value of $1.3 billion by 2026.
The use of AI in agriculture can increase crop yields by up to 70%, according to a study by the International Data Corporation (IDC).
10. Cybersecurity:
AI is strengthening cybersecurity measures by detecting and preventing cyber threats, identifying anomalous behavior, and improving data protection. AI algorithms analyze large datasets to detect patterns and anomalies, enhancing security measures.
According to Gartner, by 2022, 90% of security budgets will be allocated to addressing AI-powered cyber threats.
The global AI in cybersecurity market is projected to reach $38.2 billion by 2026, growing at a CAGR of 23.3% from 2021 to 2026.
In summary:
AI is transforming every aspect of human life, from healthcare and education to transportation, communication, entertainment, finance, and beyond. Its applications are vast and diverse, revolutionizing industries, improving efficiency, and enhancing the overall human experience. As AI continues to advance, it holds immense potential to shape a future where intelligent technologies seamlessly integrate into our daily lives, making them more convenient, productive, and enriching.
#aiinnovation#artificialintelligence#airevolution#futuretechnology#transformativetech#aiadvancements#ai applications#aiprogress#aiinsociety#emergingtech#techtrendsin2023#aiimpact#aiintegration#aiforgood
14 notes
·
View notes
Text
Exploring the Latest Breakthroughs in Technology
Introduction
Technology is evolving at a rapid pace, bringing with it groundbreaking innovations that are reshaping our world. From artificial intelligence to renewable energy solutions, these advancements are enhancing our lives in ways we never imagined. In this article, we'll explore some of the most exciting recent breakthroughs in technology that are set to transform various industries and everyday life.
1. Artificial Intelligence and Machine Learning
Artificial Intelligence (AI) and Machine Learning (ML) are at the forefront of technological innovation. AI and ML are being integrated into a myriad of applications, from healthcare diagnostics to personalized marketing. These technologies analyze vast amounts of data to make predictions, automate processes, and provide valuable insights.
AI in Healthcare
AI is revolutionizing healthcare by improving diagnostic accuracy and patient care. Machine learning algorithms can analyze medical images to detect diseases like cancer at early stages, enabling timely treatment and better patient outcomes.
AI in Everyday Life
In our daily lives, AI powers virtual assistants like Siri and Alexa, enhances customer service through chat-bots, and personalizes our online shopping experiences. The continuous improvement of AI algorithms is making these applications smarter and more efficient.
2. Quantum Computing
Quantum Computing promises to solve problems that are currently insurmountable for classical computers. By leveraging the principles of quantum mechanics, quantum computers perform complex calculations at unprecedented speeds.
Advancements in Cryptography
Quantum computing has the potential to revolutionize cryptography by breaking encryption codes that secure our digital communications. This breakthrough necessitates the development of new cryptographic methods to protect sensitive information.
Applications in Drug Discovery
In the pharmaceutical industry, quantum computing can simulate molecular interactions at a granular level, accelerating the drug discovery process and leading to the development of new, effective medications.
3. Renewable Energy Technologies
The shift towards renewable energy technologies is crucial in combating climate change. Innovations in solar, wind, and battery technologies are making renewable energy more efficient and accessible.
Solar and Wind Energy
Recent advancements in solar panel efficiency and wind turbine design are increasing the amount of energy harvested from natural sources. These improvements are making renewable energy a viable alternative to fossil fuels.
Energy Storage Solutions
Enhanced battery technologies are crucial for storing renewable energy, ensuring a consistent power supply even when the sun isn't shining or the wind isn't blowing. Breakthroughs in battery capacity and lifespan are driving the adoption of renewable energy systems.
4. Internet of Things (IoT)
The Internet of Things (IoT) connects devices and systems, enabling them to communicate and share data. This connectivity is transforming homes, industries, and cities into smarter, more efficient environments.
Smart Homes
IoT technology is making homes smarter by automating lighting, heating, and security systems. Smart home devices can be controlled remotely, offering convenience and energy savings.
Industrial IoT
In industrial settings, IoT devices monitor equipment health and optimize manufacturing processes. Predictive maintenance enabled by IoT sensors can reduce downtime and improve efficiency.
5. Blockchain Technology
Blockchain is revolutionizing how we handle transactions and data security. This decentralized ledger technology ensures transparency and security in various applications.
Financial Transactions
Blockchain is streamlining financial transactions by eliminating the need for intermediaries. It provides a secure and transparent way to transfer funds and verify transactions.
Supply Chain Management
In supply chains, blockchain offers traceability and transparency, reducing fraud and ensuring the authenticity of products. This technology is particularly beneficial in industries like pharmaceuticals and food.
6. 5G Technology
The roll-out of 5G technology is set to enhance connectivity with faster speeds and lower latency. This advancement will support the growth of IoT, autonomous vehicles, and smart cities.
Enhanced Mobile Connectivity
5G technology promises to improve mobile experiences with seamless streaming and quick downloads. It will also enable new applications in virtual and augmented reality.
Smart Cities
5G will facilitate the development of smart cities, where real-time data exchange enhances urban management systems, traffic control, and emergency services.
7. Autonomous Vehicles
Autonomous vehicles are set to transform transportation. Advances in AI and sensor technology are bringing self-driving cars closer to reality, offering safer and more efficient travel options.
Safety and Efficiency
Autonomous vehicles can reduce accidents caused by human error and optimize traffic flow, reducing congestion and emissions. They hold the potential to revolutionize the logistics and delivery sectors.
Delivery Services
Self-driving delivery vehicles and drones are making logistics faster and more reliable. These innovations are particularly beneficial in urban areas, where they can reduce traffic and pollution.
8. Biotechnology
Biotechnology is advancing rapidly, offering solutions in healthcare, agriculture, and environmental management. Innovations in gene editing, synthetic biology, and bio-engineering are opening new possibilities.
Gene Editing
CRISPR technology is enabling precise gene editing, offering potential cures for genetic diseases and innovations in agriculture. This technology is paving the way for new treatments and sustainable farming practices.
Synthetic Biology
Synthetic biology is creating new biological systems and organisms, leading to advancements in medicine, bio-fuels, and sustainable materials. This field holds promise for addressing global challenges such as disease and climate change.
9. Augmented Reality (AR) and Virtual Reality (VR)
AR and VR technologies are providing immersive experiences in entertainment, education, and various professional fields. These technologies are creating new ways to interact with digital content.
Gaming and Entertainment
AR and VR are enhancing gaming experiences by creating immersive environments and interactive game-play. These technologies are also being used in movies and virtual concerts, offering new forms of entertainment.
Professional Training
In education and professional training, AR and VR offer realistic simulations for hands-on learning. Fields like medicine, engineering, and aviation benefit from these technologies by providing safe and effective training environments.
Conclusion
The latest breakthroughs in technology are driving significant changes across various sectors. From AI and quantum computing to renewable energy and autonomous vehicles, these innovations are shaping the future and improving our lives. Staying informed about these developments is crucial for individuals and businesses alike to leverage the benefits of these technological advancements. As we look to the future, these game-changing technologies will continue to evolve, offering new opportunities and solutions to the challenges we face.
#technology#artificial intelligence#virtual reality#immersive technology#renewableenergy#ai algorithm#valuable insights#internet of things#technological advancements
2 notes
·
View notes