#WatsonX
Explore tagged Tumblr posts
Text
#IA #AI #Tecnologia #data #Orquestacion #Flujo #BI #InteligenciaNegocios
0 notes
Text
watsonx.data: Scale AI Workloads, for all your data, anywhere
Data is fundamental to every business, fueling applications, predictive insights, and improved experiences. However, fully harnessing data’s potential is often hindered by limitations in storage and access for analytics and AI.
AI begins with how your data is stored, managed, and governed to ensure it is reliable and scalable. watsonx.data, leveraging a data lakehouse architecture, can help you cut your data warehouse costs by up to 50% and streamline access to governed data for AI.
IBM watsonx.data is an open, hybrid, and governed data store designed to optimize all data, analytics, and AI workloads.
watsonx.data enables enterprises to seamlessly expand their analytics and AI capabilities by leveraging a purpose-built data store. This data store is built on an open lakehouse architecture, incorporating robust querying, governance, and open data formats to facilitate efficient data access and sharing. By utilizing watsonx.data, you can establish connections to data sources in a matter of minutes, swiftly obtain reliable insights, and significantly reduce costs associated with traditional data warehousing.
With watsonx.data, users can access their increasing amount of data through a single point of entry while applying the multiple fit for purpose query engines to unlock the valuable insights.
Know more about watsonx.data: https://pragmaedge.com/watsonx-data/
Find answers to your questions about Watsonx.data: https://pragmaedge.com/watsonx-data-faqs/
0 notes
Text
Meta Unveils Llama 3.1: A Challenger in the AI Arena
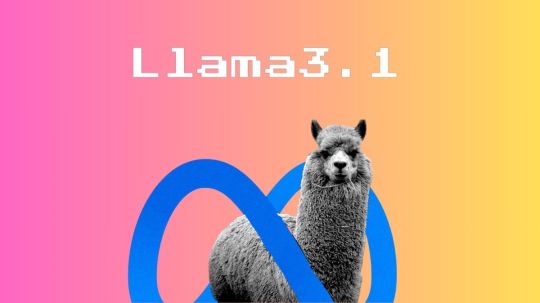
Meta launches new Llama 3.1 models, including anticipated 405B parameter version.
Meta released Llama 3.1, a multilingual LLM collection. Llama 3.1 includes pretrained and instruction-tuned text in/text out open source generative AI models with 8B, 70B, and 405B parameters.
Today, IBM watsonx.ai will offer the instruction-tuned Llama 3.1-405B, the largest and most powerful open source language model available and competitive with the best proprietary models.It can be set up on-site, in a hybrid cloud environment, or on the IBM cloud.
Llama 3.1 follows the April 18 debut of Llama 3 models. Meta stated in the launch release that “[their] goal in the near future is to make Llama 3 multilingual and multimodal, have longer context, and continue to improve overall performance across LLM capabilities such as reasoning and coding.”
Llama 3.1’s debut today shows tremendous progress towards that goal, from dramatically enhanced context length to tool use and multilingual features.
An significant step towards open, responsible, accessible AI innovation
Meta and IBM launched the AI Alliance in December 2023 with over 50 global initial members and collaborators. The AI Alliance unites leading business, startup, university, research, and government organisations to guide AI’s evolution to meet society’s requirements and complexities. Since its formation, the Alliance has over 100 members.
Additionally, the AI Alliance promotes an open community that helps developers and researchers accelerate responsible innovation while maintaining trust, safety, security, diversity, scientific rigour, and economic competitiveness. To that aim, the Alliance supports initiatives that develop and deploy benchmarks and evaluation standards, address society-wide issues, enhance global AI capabilities, and promote safe and useful AI development.
Llama 3.1 gives the global AI community an open, state-of-the-art model family and development ecosystem to explore, experiment, and responsibly scale new ideas and techniques. The release features strong new models, system-level safety safeguards, cyber security evaluation methods, and improved inference-time guardrails. These resources promote generative AI trust and safety tool standardisation.
How Llama 3.1-405B compares to top models
The April release of Llama 3 highlighted upcoming Llama models with “over 400B parameters” and some early model performance evaluation, but their exact size and details were not made public until today’s debut. Llama 3.1 improves all model sizes, but the 405B open source model matches leading proprietary, closed source LLMs for the first time.
Looking beyond numbers
Performance benchmarks are not the only factor when comparing the 405B to other cutting-edge models. Llama 3.1-405B may be built upon, modified, and run on-premises, unlike its closed source contemporaries, which can change their model without notice. That level of control and predictability benefits researchers, businesses, and other entities that seek consistency and repeatability.
Effective Llama-3.1-405B usage
IBM, like Meta, believes open models improve product safety, innovation, and the AI market. An advanced 405B-parameter open source model offers unique potential and use cases for organisations of all sizes.
Aside from inference and text creation, which may require quantisation or other optimisation approaches to execute locally on most hardware systems, the 405B can be used for:
Synthetic data can fill the gap in pre-training, fine-tuning, and instruction tuning when data is limited or expensive. The 405B generates high-quality task- and domain-specific synthetic data for LLM training. IBM’s Large-scale Alignment for chatBots (LAB) phased-training approach quickly updates LLMs with synthetic data while conserving model knowledge.
The 405B model’s knowledge and emergent abilities can be reduced into a smaller model, combining the capabilities of a big “teacher” model with the quick and cost-effective inference of a “student” model (such an 8B or 70B Llama 3.1). Effective Llama-based models like Alpaca and Vicuna need knowledge distillation, particularly instruction tailoring on synthetic data provided by bigger GPT models.
LLM-as-a-judge: The subjectivity of human preferences and the inability of standards to approximate them make LLM evaluation difficult. The Llama 2 research report showed that larger models can impartially measure response quality in other models. Learn more about LLM-as-a-judge’s efficacy in this 2023 article.
A powerful domain-specific fine-tune: Many leading closed models allow fine-tuning only on a case-by-case basis, for older or smaller model versions, or not at all. Meta has made Llama 3.1-405B accessible for pre-training (to update the model’s general knowledge) or domain-specific fine-tuning coming soon to the watsonx Tuning Studio.
Meta AI “strongly recommends” using a platform like IBM watsonx for model evaluation, safety guardrails, and retrieval augmented generation to deploy Llama 3.1 models.
Every llama 3.1 size gets upgrades
The long-awaited 405B model may be the most notable component of Llama 3.1, but it’s hardly the only one. Llama 3.1 models share the dense transformer design of Llama 3, but they are much improved at all model sizes.
Longer context windows
All pre-trained and instruction-tuned Llama 3.1 models have context lengths of 128,000 tokens, a 1600% increase over 8,192 tokens in Llama 3. Llama 3.1’s context length is identical to the enterprise version of GPT-4o, substantially longer than GPT-4 (or ChatGPT Free), and comparable to Claude 3’s 200,000 token window. Llama 3.1’s context length is not constrained in situations of high demand because it can be installed on the user’s hardware or through a cloud provider.. Llama 3.1 has few usage restrictions.
An LLM can consider or “remember” a certain amount of tokenised text (called its context window) at any given moment. To continue, a model must trim or summarise a conversation, document, or code base that exceeds its context length. Llama 3.1’s extended context window lets models have longer discussions without forgetting details and ingest larger texts or code samples during training and inference.
Text-to-token conversion doesn’t have a defined “exchange rate,” but 1.5 tokens per word is a good estimate. Thus, Llama 3.1’s 128,000 token context window contains 85,000 words. The Hugging Face Tokeniser Playground lets you test multiple tokenisation models on text inputs.
Llama 3.1 models benefit from Llama 3’s new tokeniser, which encodes language more effectively than Llama 2.
Protecting safety
Meta has cautiously and thoroughly expanded context length in line with its responsible innovation approach. Previous experimental open source attempts produced Llama derivatives with 128,000 or 1M token windows. These projects demonstrate Meta’s open model commitment, however they should be approached with caution: Without strong countermeasures, lengthy context windows “present a rich new attack surface for LLMs” according to recent study.
Fortunately, Llama 3.1 adds inference guardrails. The release includes direct and indirect prompt injection filtering from Prompt Guard and updated Llama Guard and CyberSec Eval. CodeShield, a powerful inference time filtering technology from Meta, prevents LLM-generated unsafe code from entering production systems.
As with any generative AI solution, models should be deployed on a secure, private, and safe platform.
Multilingual models
Pretrained and instruction tailored Llama 3.1 models of all sizes will be bilingual. In addition to English, Llama 3.1 models speak Spanish, Portuguese, Italian, German, and Thai. Meta said “a few other languages” are undergoing post-training validation and may be released.
Optimised for tools
Meta optimised the Llama 3.1 Instruct models for “tool use,” allowing them to interface with applications that enhance the LLM’s capabilities. Training comprises creating tool calls for specific search, picture production, code execution, and mathematical reasoning tools, as well as zero-shot tool use—the capacity to effortlessly integrate with tools not previously encountered in training.
Starting Llama 3.1
Meta’s latest version allows you to customise state-of-the-art generative AI models for your use case.
IBM supports Llama 3.1 to promote open source AI innovation and give clients access to best-in-class open models in watsonx, including third-party models and the IBM Granite model family.
IBM Watsonx allows clients to deploy open source models like Llama 3.1 on-premises or in their preferred cloud environment and use intuitive workflows for fine-tuning, prompt engineering, and integration with enterprise applications. Build business-specific AI apps, manage data sources, and expedite safe AI workflows on one platform.
Read more on govindhtech.com
#MetaUnveilsLlama31#AIArena#generativeAImodels#Llama3#LLMcapabilities#AIcapabilities#generativeAI#watsonx#LLMevaluation#IBMwatsonx#gpt40#Llama2#Optimisedtools#Multilingualmodels#AIworkflows#technology#technews#news#govindhtech
0 notes
Text
IBM Unveils Next Chapter of watsonx with Open Source, Product & Ecosystem Innovations to Drive Enterprise AI at Scale
Releases a family of IBM Granite models into open source, including its most capable and efficient Code LLMs that can out-perform larger code models on many industry benchmarks Jointly with Red Hat, launches InstructLab, a first-of-its-kind model alignment technique, to bring open-source community contributions directly into LLMs Unveils new vision and momentum for new data and automation…
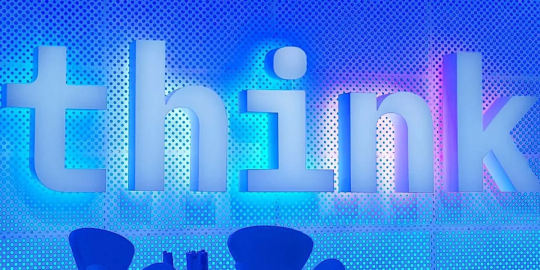
View On WordPress
0 notes
Text
生成式 AI 變球探!西班牙西維爾足球俱樂部率先使用
由 IBM 和西班牙西維爾足球俱樂部(Sevilla FC)日前宣布共同推出一款創新的生成式AI工具Scout Advisor,顧名思義「球探顧問」,西維爾足球俱樂部將使用該工具為其球探團隊提供全面的、數據驅動的潛在新球員識別和評估服務。 西維爾足球俱樂部的 Scout Advisor 以 IBM 專為企業設計的AI與數據平台watsonx構建,將與其現有自主開發的數據密集型應用套件進行整合。 Continue reading 生成式 AI 變球探!西班牙西維爾足球俱樂部率先使用
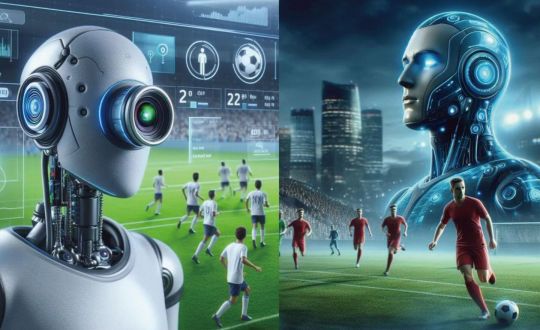
View On WordPress
0 notes
Link
0 notes
Text
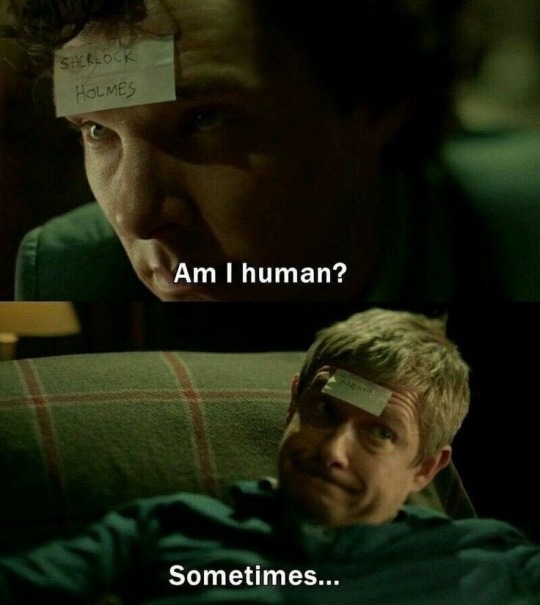
Sherlock: “I don’t want to go outside. There are people there.”
(Font: Pinterest)
#bbc sherlock#aesthetic#quotes#daily life#life quotes#after the storm#books and reading#series tv#tv shows#bbc shows#sherlock holmes#i am sherlocked#john watson#watsonx#shirley
1 note
·
View note
Text
#IA - IBM Planea incluir Llama 2 dentro de Watsonx AI y Data Platform
Como parte del despliegue continuo de su plataforma de IA y Data Platform para empresas, watsonx, IBM planea alojar el modelo de 70 mil millones de parámetros de Llama 2-chat de Meta en watsonx.ai studio, con acceso anticipado y disponible para clientes y socios selectos. Esto se basará en la colaboración de IBM con Meta en innovación abierta para la IA, incluyendo el trabajo con proyectos de…
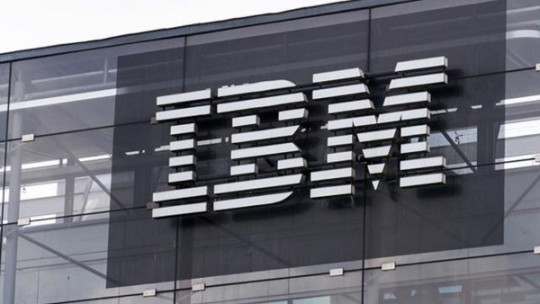
View On WordPress
0 notes
Text
IBM presenta Granite 3.0: modelos de IA de alto rendimiento diseñados para las empresas
Los nuevos modelos Granite 3.0 8B y 2B, publicados bajo la licencia permisiva Apache 2.0, ofrecen un sólido rendimiento en múltiples pruebas académicas y empresariales, siendo capaces de superar o igualar a modelos de tamaño similar Los nuevos modelos Granite Guardian 3.0 ofrecen las funciones de protección más completas de IBM para fomentar una IA segura y fiable IBM presenta la próxima…
#AI#Apache 2.0#Granite 3.0#Granite 3.0 1B A400M Base#Granite 3.0 1B A400M Instruct#Granite 3.0 2B Base#Granite 3.0 2B-Instruct#Granite 3.0 3B A800M Base#Granite 3.0 3B A800M Instruct#Granite 3.0 8B Base#Granite 3.0 8B-Instruct#Granite Guardian 3.0 2B#Granite Guardian 3.0 8B#IA#IA empresarial#IA responsable#IBM#IBM Consulting Advantage#IBM Granite.Code#Modelos de lenguaje#RAG#TechXchange#watsonx Code Assistant#watsonx.ai
0 notes
Text
IBM y la NASA crean un modelo fundacional de IA geoespacial de código abierto en Hugging Face
Continue reading Untitled
View On WordPress
#Acuerdo de Ley Espacial#AI#Código abierto#Clark University#Clima#Datos geoespaciales#Datos Satelitales#Deforestación#Gases de efecto invernadero#Harmonized Landsat Sentinel-2 satellite data#HLS#Hugging Face#IA#IBM#IBM Environmental Intelligence Suite#IBM Watsonx#Im��genes Satelitales#Iniciativa Científica de Código Abierto#Inteligencia Artificial#Nasa#satélite#watsonx#watsonx.ai
0 notes
Video
IBM: Let’s create the right AI for your business with watsonx
This commercial came on while I was getting ready for work this morning. It made me stop short and listen. Is that???? Yes. Yes, it is.
#youtube#oscar isaac#when you become so familiar with a person's voice#watson x#commercial voice over#good morning to me
4 notes
·
View notes
Text
Unlocking the Future of AI and Data with Pragma Edge's Watson X Platform
In a rapidly evolving digital landscape, enterprises are constantly seeking innovative solutions to harness the power of artificial intelligence (AI) and data to gain a competitive edge. Enter Pragma Edge's Watson X, a groundbreaking AI and Data Platform designed to empower enterprises with scalability and accelerate the impact of AI capabilities using trusted data. This comprehensive platform offers a holistic solution, encompassing data storage, hardware, and foundational models for AI and Machine Learning (ML).
The All-in-One Solution for AI Advancement
At the heart of Watson X is its commitment to providing an open ecosystem, allowing enterprises to design and fine-tune large language models (LLMs) to meet their operational and business requirements. This platform is not just about AI; it's about transforming your business through automation, streamlining workflows, enhancing security, and driving sustainability goals.
Key Components of Watson X
Watsonx.ai: The AI Builder's Playground
Watsonx.ai is an enterprise development studio where AI builders can train, test, tune, and deploy both traditional machine learning and cutting-edge generative AI capabilities.
It offers a diverse array of foundation models, training and tuning tools, and cost-effective infrastructure to facilitate the entire data and AI lifecycle.
Watsonx.data: Fueling AI Initiatives
Watsonx.data is a specialized data store built on the open lakehouse architecture, tailored for analytics and AI workloads.
This agile and open data repository empowers enterprises to efficiently manage and access vast amounts of data, driving quick decision-making processes.
Watsonx.governance: Building Responsible AI
Watsonx.governance lays the foundation for an AI workforce that operates responsibly and transparently.
It establishes guidelines for explainable AI, ensuring businesses can understand AI model decisions, fostering trust with clients and partners.
Benefits of WatsonX
Unified Data Access: Gain access to information data across both on-premises and cloud environments, streamlining data management.
Enhanced Governance: Apply robust governance measures, reduce costs, and accelerate model deployment, ensuring high-quality outcomes.
End-to-End AI Lifecycle: Accelerate the entire AI model lifecycle with comprehensive tools and runtimes for training, validation, tuning, and deployment—all in one location.
In a world driven by data and AI, Pragma Edge's Watson X Platform empowers enterprises to harness the full potential of these technologies. Whether you're looking to streamline processes, enhance security, or unlock new business opportunities, Watson X is your partner in navigating the future of AI and data. Don't miss out on the transformative possibilities—explore Watson X today at watsonx.ai and embark on your journey towards AI excellence.
Learn more: https://pragmaedge.com/watsonx/
#Watsonx#WatsonxPlatform#WatsonxAI#WatsonxData#WatsonxGovernance#WatsonxStudio#AIBuilders#FoundationModels#AIWorkflows#Pragma Edge
0 notes
Text
Mizuho & IBM Partner on Gen AI PoC to Boost Recovery Times
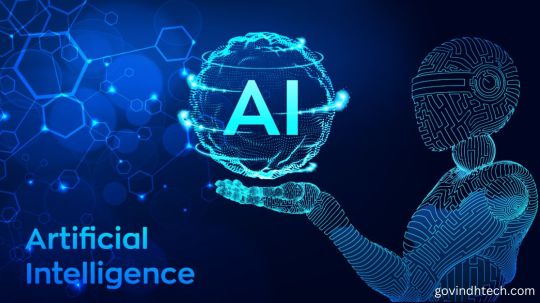
IBM and Mizuho Financial Group, worked together to create a proof of concept (PoC) that uses IBM’s enterprise generative AI and data platform, watsonx, to increase the effectiveness and precision of Mizuho’s event detection processes.
During a three-month trial, the new technology showed 98% accuracy in monitoring and reacting to problem alerts. Future system expansion and validation are goals shared by IBM and Mizuho.
The financial systems need to recover accurately and quickly after an interruption.
However, operators frequently receive a flood of messages and reports when an error is detected, which makes it challenging to identify the event’s source and ultimately lengthens the recovery period.
In order to solve the problem, IBM and Mizuho carried out a Proof of Concept using Watsonx to increase error detection efficiency.
In order to reduce the number of steps required for recovery and accelerate recovery, the Proof of Concept (PoC) integrated an application that supported a series of processes in event detection with Watsonx and incorporated patterns likely to result in errors in incident response.
By utilising Watsonx, Mizuho was also able to streamline internal operations and enable people on-site to configure monitoring and operating menus in a flexible manner when greater security and secrecy are needed.
In the upcoming year, Mizuho and IBM intend to extend the event detection and reaction proof of concept and integrate it into real-world settings. To increase operational efficiency and sophistication, Mizuho and IBM also intend to cooperate on incident management and advanced failure analysis using generative AI.
WatsonX for Proof of Concept (PoC)
IBM’s WatsonX is an enterprise-grade platform that integrates generative AI capabilities with data. It is therefore an effective tool for creating and evaluating AI solutions. This is a thorough examination of WatsonX use for a PoC:
Why Would a PoC Use WatsonX?
Streamlined Development: WatsonX can expedite the Proof of Concept development process by providing a pre-built component library and an intuitive UI.
Data Integration: It is simpler to include the data required for your AI model since the platform easily integrates with a variety of data sources.
AI Capabilities: WatsonX comes with a number of built-in AI features, including as computer vision, machine learning, and natural language processing, which let you experiment with alternative strategies within your proof of concept.
Scalability: As your solution develops, the platform can handle handling small-scale experimentation for your Proof of Concept.
How to Use a WatsonX PoC
Establish your objective: Clearly state the issue you’re attempting to resolve or the procedure you wish to use AI to enhance. What precise results are you hoping to achieve with your PoC?
Collect Information: Determine the kind of data that your AI model needs to be trained on. Make that the information is adequate, correct, and pertinent for the PoC’s scope.
Select Usability: Based on your objective, choose the WatsonX AI functionalities that are most relevant. Computer vision for image recognition, machine learning for predictive modelling, and natural language processing for sentiment analysis are examples.
Create a PoC: Create a rudimentary AI solution using WatsonX’s tools and frameworks. This could entail building a prototype with restricted functionality or training a basic model.
Test & Assess: Utilise real-world data to evaluate your proof of concept’s efficacy. Examine the outcomes to determine whether the intended goals were met. Point out any places that need work.
Refine and Present: Make iterations to the data, model, or functions to improve your Proof of Concept based on your assessment. Present your results to stakeholders at the end, highlighting the PoC’s shortcomings and areas for future improvement.
Extra Things to Think About
Keep your point of contact (PoC) concentrated on a single issue or task. At this point, don’t try to develop a complete solution.
Success Criteria: Prior to implementation, clearly define your PoC’s success metrics. This may entail cost-cutting, efficiency, or accuracy.
Ethical Considerations: Consider the ethical ramifications of your AI solution as well as any potential biases in your data.
Goal of Collaboration:
Boost Mizuho’s event detection and reaction activities’ precision and efficiency.
Technology Employed:
IBM’s WatsonX is a platform for corporate generative AI and data.
What they Found Out:
Created a proof of concept (PoC) that monitors error signals and reacts with WatsonX.
Over the course of a three-month study, the PoC attained a 98% accuracy rate.
Future Objectives:
Increase the breadth of the event detection and response situations covered by the PoC.
After a year, implement the solution in actual production settings.
To further streamline operations, investigate the use of generative AI for enhanced failure analysis and issue management.
Total Effect:
This project demonstrates how generative AI has the ability to completely transform the banking industry’s operational effectiveness. Mizuho hopes to increase overall business continuity and drastically cut down on recovery times by automating incident detection and reaction.
Emphasis on Event Detection and Response: The goal of the project is to enhance Mizuho’s monitoring and error-messaging processes by employing generative AI, notably IBM’s WatsonX platform.
Effective Proof of Concept (PoC): Mizuho and IBM worked together to create a PoC that showed a notable increase in accuracy. During a three-month trial period, the AI system identified and responded to error notifications with a 98% success rate.
Future Plans: Mizuho and IBM intend to grow the project in the following ways in light of the encouraging Proof of Concept outcomes:
Production Deployment: During the course of the upcoming year, they hope to incorporate the event detection and response system into Mizuho’s live operations environment.
Advanced Applications: Through this partnership, generative AI will be investigated for use in increasingly difficult activities such as advanced failure analysis and incident management. This might entail the AI automatically identifying problems and making recommendations for fixes, therefore expediting the healing procedures.
Overall Impact: This project demonstrates how generative AI has the ability to completely transform financial institutions’ operational efficiency. Mizuho may be able to lower interruption costs, boost overall service quality, and cut downtime by automating fault detection and response.
Consider these other points:
Generative AI can identify and predict flaws by analysing historical data and finding patterns. The calibre and applicability of the data utilised to train the AI models will determine this initiative’s success.
Read more on Govindhtech.com
0 notes
Text
AI in 2025: Purpose-driven models, human integration, and more
New Post has been published on https://thedigitalinsider.com/ai-in-2025-purpose-driven-models-human-integration-and-more/
AI in 2025: Purpose-driven models, human integration, and more
As AI becomes increasingly embedded in our daily lives, industry leaders and experts are forecasting a transformative 2025.
From groundbreaking developments to existential challenges, AI’s evolution will continue to shape industries, change workflows, and spark deeper conversations about its implications.
For this article, AI News caught up with some of the world’s leading minds to see what they envision for the year ahead.
Smaller, purpose-driven models
Grant Shipley, Senior Director of AI at Red Hat, predicts a shift away from valuing AI models by their sizeable parameter counts.
“2025 will be the year when we stop using the number of parameters that models have as a metric to indicate the value of a model,” he said.
Instead, AI will focus on specific applications. Developers will move towards chaining together smaller models in a manner akin to microservices in software development. This modular, task-based approach is likely to facilitate more efficient and bespoke applications suited to particular needs.
Open-source leading the way
Bill Higgins, VP of watsonx Platform Engineering and Open Innovation at IBM, expects open-source AI models will grow in popularity in 2025.
“Despite mounting pressure, many enterprises are still struggling to show measurable returns on their AI investments—and the high licensing fees of proprietary models is a major factor. In 2025, open-source AI solutions will emerge as a dominant force in closing this gap,” he explains.
Alongside the affordability of open-source AI models comes transparency and increased customisation potential, making them ideal for multi-cloud environments. With open-source models matching proprietary systems in power, they could offer a way for enterprises to move beyond experimentation and into scalability.
This plays into a prediction from Nick Burling, SVP at Nasuni, who believes that 2025 will usher in a more measured approach to AI investments.
“Enterprises will focus on using AI strategically, ensuring that every AI initiative is justified by clear, measurable returns,” said Burling.
Cost efficiency and edge data management will become crucial, helping organisations optimise operations while keeping budgets in check.
Augmenting human expertise
For Jonathan Siddharth, CEO of Turing, the standout feature of 2025 AI systems will be their ability to learn from human expertise at scale.
“The key advancement will come from teaching AI not just what to do, but how to approach problems with the logical reasoning that coding naturally cultivates,” he says.
Competitiveness, particularly in industries like finance and healthcare, will hinge on mastering this integration of human expertise with AI.
Behavioural psychology will catch up
Understanding the interplay between human behaviour and AI systems is at the forefront of predictions for Niklas Mortensen, Chief Design Officer at Designit.
“With so many examples of algorithmic bias leading to unwanted outputs – and humans being, well, humans – behavioural psychology will catch up to the AI train,” explained Mortensen.
The solutions? Experimentation with ‘pause moments’ for human oversight and intentional balance between automation and human control in critical operations such as healthcare and transport.
Mortensen also believes personal AI assistants will finally prove their worth by meeting their long-touted potential in organising our lives efficiently and intuitively.
Bridge between physical and digital worlds
Andy Wilson, Senior Director at Dropbox, envisions AI becoming an indispensable part of our daily lives.
“AI will evolve from being a helpful tool to becoming an integral part of daily life and work – offering innovative ways to connect, create, and collaborate,” Wilson says.
Mobile devices and wearables will be at the forefront of this transformation, delivering seamless AI-driven experiences.
However, Wilson warns of new questions on boundaries between personal and workplace data, spurred by such integrations.
Driving sustainability goals
With 2030 sustainability targets looming over companies, Kendra DeKeyrel, VP ESG & Asset Management at IBM, highlights how AI can help fill the gap.
DeKeyrel calls on organisations to adopt AI-powered technologies for managing energy consumption, lifecycle performance, and data centre strain.
“These capabilities can ultimately help progress sustainability goals overall,” she explains.
Unlocking computational power and inference
James Ingram, VP Technology at Streetbees, foresees a shift in computational requirements as AI scales to handle increasingly complex problems.
“The focus will move from pre-training to inference compute,” he said, highlighting the importance of real-time reasoning capabilities.
Expanding context windows will also significantly enhance how AI retains and processes information, likely surpassing human efficiency in certain domains.
Rise of agentic AI and unified data foundations
According to Dominic Wellington, Enterprise Architect at SnapLogic, “Agentic AI marks a more flexible and creative era for AI in 2025.”
However, such systems require robust data integration because siloed information risks undermining their reliability.
Wellington anticipates that 2025 will witness advanced solutions for improving data hygiene, integrity, and lineage—all vital for enabling agentic AI to thrive.
From hype to reality
Jason Schern, Field CTO of Cognite, predicts that 2025 will be remembered as the year when truly transformative, validated generative AI solutions emerge.
“Through the fog of AI for AI’s sake noise, singular examples of truly transformative embedding of Gen AI into actual workflows will stand out,” predicts Schern.
These domain-specific AI agents will revolutionise industrial workflows by offering tailored decision-making. Schern cited an example in which AI slashed time-consuming root cause analyses from months to mere minutes.
Deepfakes and crisis of trust
Sophisticated generative AI threatens the authenticity of images, videos, and information, warns Siggi Stefnisson, Cyber Safety CTO at Gen.
“Even experts may not be able to tell what’s authentic,” warns Stefnisson.
Combating this crisis requires robust digital credentials for verifying authenticity and promoting trust in increasingly blurred digital realities.
2025: Foundational shifts in the AI landscape
As multiple predictions converge, it’s clear that foundational shifts are on the horizon.
The experts that contributed to this year’s industry predictions highlight smarter applications, stronger integration with human expertise, closer alignment with sustainability goals, and heightened security. However, many also foresee significant ethical challenges.
2025 represents a crucial year: a transition from the initial excitement of AI proliferation to mature and measured adoption that promises value and a more nuanced understanding of its impact.
See also: AI Action Summit: Leaders call for unity and equitable development
Want to learn more about AI and big data from industry leaders? Check out AI & Big Data Expo taking place in Amsterdam, California, and London. The comprehensive event is co-located with other leading events including Intelligent Automation Conference, BlockX, Digital Transformation Week, and Cyber Security & Cloud Expo.
Explore other upcoming enterprise technology events and webinars powered by TechForge here.
#2025#adoption#Agentic AI#agents#ai#ai & big data expo#ai action summit#AI AGENTS#AI models#ai news#AI systems#AI-powered#algorithmic bias#amp#analyses#applications#approach#Article#Artificial Intelligence#automation#Bias#Big Data#bridge#budgets#california#CEO#change#Cloud#coding#collaborate
5 notes
·
View notes
Link
0 notes