#IBMwatsonx
Explore tagged Tumblr posts
Text
IBM Db2 AI Updates: Smarter, Faster, Better Database Tools
IBM Db2
Designed to handle mission-critical workloads worldwide.
What is IBM Db2?
IBM Db2 is a cloud-native database designed to support AI applications at scale, real-time analytics, and low-latency transactions. It offers database managers, corporate architects, and developers a single engine that is based on decades of innovation in data security, governance, scalability, and availability.
- Advertisement -
When moving to hybrid deployments, create the next generation of mission-critical apps that are available 24/7 and have no downtime across all clouds.
Support for all contemporary data formats, workloads, and programming languages will streamline development.
Support for open formats, including Apache Iceberg, allows teams to safely communicate data and information, facilitating quicker decision-making.
Utilize IBM Watsonx integration for generative artificial intelligence (AI) and integrated machine learning (ML) capabilities to implement AI at scale.
Use cases
Power next-gen AI assistants
Provide scalable, safe, and accessible data so that developers may create AI-powered assistants and apps.
Build new cloud-native apps for your business
Create cloud-native applications with low latency transactions, flexible scalability, high concurrency, and security that work on any cloud. Amazon Relational Database Service (RDS) now offers it.
Modernize mission-critical web and mobile apps
Utilize Db2 like-for-like compatibility in the cloud to modernize your vital apps for hybrid cloud deployments. Currently accessible via Amazon RDS.
Power real-time operational analytics and insights
Run in-memory processing, in-database analytics, business intelligence, and dashboards in real-time while continuously ingesting data.
Data sharing
With support for Apache Iceberg open table format, governance, and lineage, you can share and access all AI data from a single point of entry.
In-database machine learning
With SQL, Python, and R, you can create, train, assess, and implement machine learning models from inside the database engine without ever transferring your data.
Built for all your workloads
IBM Db2 Database
Db2 is the database designed to handle transactions of any size or complexity. Currently accessible via Amazon RDS.
IBM Db2 Warehouse
You can safely and economically conduct mission-critical analytical workloads on all kinds of data with IBM Db2 Warehouse. Watsonx.data integration allows you to grow AI workloads anywhere.
IBM Db2 Big SQL
IBM Db2 Big SQL is a high-performance, massively parallel SQL engine with sophisticated multimodal and multicloud features that lets you query data across Hadoop and cloud data lakes.
Deployment options
You require an on-premises, hybrid, or cloud database. Use Db2 to create a centralized business data platform that operates anywhere.
Cloud-managed service
Install Db2 on Amazon Web Services (AWS) and IBM Cloud as a fully managed service with SLA support, including RDS. Benefit from the cloud’s consumption-based charging, on-demand scalability, and ongoing improvements.
Cloud-managed container
Launch Db2 as a cloud container:integrated Db2 into your cloud solution and managed Red Hat OpenShift or Kubernetes services on AWS and Microsoft Azure.
Self-managed infrastructure or IaaS
Take control of your Db2 deployment by installing it as a conventional configuration on top of cloud-based infrastructure-as-a-service or on-premises infrastructure.
IBM Db2 Updates With AI-Powered Database Helper
Enterprise data is developing at an astonishing rate, and companies are having to deal with ever-more complicated data environments. Their database systems are under more strain than ever as a result of this. Version 12.1 of IBM’s renowned Db2 database, which is scheduled for general availability this week, attempts to address these demands. The latest version redefines database administration by embracing AI capabilities and building on Db2’s lengthy heritage.
The difficulties encountered by database administrators who must maintain performance, security, and uptime while managing massive (and quickly expanding) data quantities are covered in Db2 12.1. A crucial component of their strategy is IBM Watsonx’s generative AI-driven Database Assistant, which offers real-time monitoring, intelligent troubleshooting, and immediate replies.
Introducing The AI-Powered Database Assistant
By fixing problems instantly and averting interruptions, the new Database Assistant is intended to minimize downtime. Even for complicated queries, DBAs may communicate with the system in normal language to get prompt responses without consulting manuals.
The Database Assistant serves as a virtual coach in addition to its troubleshooting skills, speeding up DBA onboarding by offering solutions customized for each Db2 instance. This lowers training expenses and time. By enabling DBAs to address problems promptly and proactively, the database assistant should free them up to concentrate on strategic initiatives that improve the productivity and competitiveness of the company.
IBM Db2 Community Edition
Now available
Db2 12.1
No costs. No adware or credit card. Simply download a single, fully functional Db2 Community License, which you are free to use for as long as you wish.
What you can do when you download Db2
Install on a desktop or laptop and use almost anywhere. Join an active user community to discover events, code samples, and education, and test prototypes in a real-world setting by deploying them in a data center.
Limits of the Community License
Community license restrictions include an 8 GB memory limit and a 4 core constraint.
Read more on govindhtech.com
#IBMDb2AIUpdates#BetterDatabaseTools#IBMDb2#ApacheIceberg#AmazonRelationalDatabaseService#RDS#machinelearning#IBMDb2Database#IBMDb2BigSQL#AmazonWebServices#AWS#MicrosoftAzure#IBMWatsonx#Db2instance#technology#technews#news#govindhtech
0 notes
Text
How Is AI Transforming Coding?
How Is AI Transforming Coding? @IBM, thanks for sharing Discover More: https://bit.ly/40t7Fuy #IBMpartner #PaidPartnership #AI #IBMWatsonx from Ronald van Loon https://www.youtube.com/watch?v=Fc6POnyYTmQ
View On WordPress
#5G#ChatGPT#CXSummitEmea#Education#Five9Partner#Healthcare#HuaweiPartner#Innovation#Networking#Robotics#Technology#TFBPartner
0 notes
Text
IBM Watson X: The Future of AI for Business #IBMWatsonX #AI
youtube
1 note
·
View note
Text
IBM Guardium Data Security Center Boosts AI & Quantum Safety
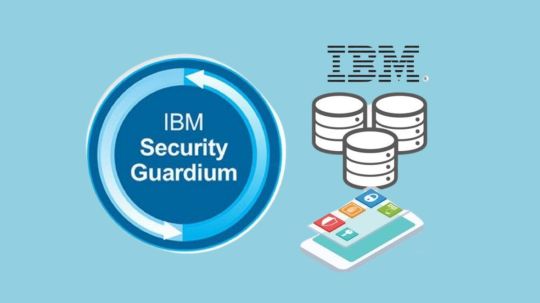
Introducing IBM Guardium Data Security Center
Using a unified experience, protect your data from both present and future threats, such as cryptography and artificial intelligence assaults.
IBM is unveiling IBM Guardium Data Security Center, which enables enterprises to protect data in any environment, during its full lifespan, and with unified controls, as concerns connected to hybrid clouds, artificial intelligence, and quantum technology upend the conventional data security paradigm.
To assist you in managing the data security lifecycle, from discovery to remediation, for all data types and across all data environments, IBM Guardium Data Security Center provides five modules. In the face of changing requirements, it enables security teams throughout the company to work together to manage data risks and vulnerabilities.
Why Guardium Data Security Center?
Dismantle organizational silos by giving security teams the tools they need to work together across the board using unified compliance regulations, connected procedures, and a shared perspective of data assets.
Safeguard both structured and unstructured data on-premises and in the cloud.
Oversee the whole data security lifecycle, from detection to repair.
Encourage security teams to work together by providing an open ecosystem and integrated workflows.
Protect your digital transformation
Continuously evaluate threats and weaknesses with automated real-time alerts. Automated discovery and classification, unified dashboards and reporting, vulnerability management, tracking, and workflow management are examples of shared platform experiences that help you safeguard your data while growing your company.
Security teams can integrate workflows and handle data monitoring and governance, data detection and response, data and AI security posture management, and cryptography management all from a single dashboard with IBM Guardium Data Security Center’s shared view of an organization’s data assets. Generative AI features in IBM Guardium Data Security Center can help create risk summaries and increase the efficiency of security professionals.
IBM Guardium AI Security
At a time when generative AI usage is on the rise and the possibility of “shadow AI,” or the existence of unapproved models, is increasing, the center offers IBM Guardium AI Security, software that helps shield enterprises’ AI deployments from security flaws and violations of data governance policies.
Control the danger to the security of private AI data and models.
Use IBM Guardium AI Security to continuously find and address vulnerabilities in AI data, models, and application usage.
Guardium AI Security assists businesses in:
Obtain ongoing, automated monitoring for AI implementations.
Find configuration errors and security flaws
Control how users, models, data, and apps interact with security.
This component of IBM Guardium Data Security Center enables cross-organization collaboration between security and AI teams through unified compliance policies, a shared view of data assets, and integrated workflows.
Advantages
Learn about shadow AI and gain complete insight into AI implementations
The Guardium the AI model linked to each deployment is made public by AI Security. It reveals the data, model, and application utilization of every AI deployment. All of the applications that access the model will also be visible to you.
Determine which high-risk vulnerabilities need to be fixed
You can see the weaknesses in your model, the data that underlies it, and the apps that use it. You can prioritize your next steps by assigning a criticality value to each vulnerability. The list of vulnerabilities is easily exportable for reporting.
Adapt to evaluation frameworks and adhere to legal requirements
You can handle compliance concerns with AI models and data and manage security risk with the aid of Guardium AI Security. Assessment frameworks, like OWASP Top 10 for LLM, are mapped to vulnerabilities so that you can quickly understand more about the risks that have been detected and the controls that need to be put in place to mitigate them.
Qualities
Continuous and automated monitoring for AI implementations
Assist companies in gaining complete insight into AI implementations so they can identify shadow AI.
Find configuration errors and security flaws
Determine which high-risk vulnerabilities need to be fixed and relate them to evaluation frameworks like the OWASP Top 10 for LLM.
Keep an eye on AI compliance
Learn about AI implementations and how users, models, data, and apps interact. IBM Watsonx.governance is included preinstalled.
IBM Guardium Quantum Safe
Become aware of your cryptographic posture. Evaluate and rank cryptographic flaws to protect your important data.
IBM Guardium Quantum Safe, a program that assists customers in safeguarding encrypted data from future cyberattacks by malevolent actors with access to quantum computers with cryptographic implications, is another element of IBM Guardium Data Security Center. IBM Research, which includes IBM’s post-quantum cryptography techniques, and IBM Consulting have contributed to the development of IBM Guardium Quantum Safe.
Sensitive information could soon be exposed if traditional encryption techniques are “broken.”
Every business transaction is built on the foundation of data security. For decades, businesses have depended on common cryptography and encryption techniques to protect their data, apps, and endpoints. With quantum computing, old encryption schemes that would take years to crack on a traditional computer may be cracked in hours. All sensitive data protected by current encryption standards and procedures may become exposed as quantum computing develops.
IBM is a leader in the quantum safe field, having worked with industry partners to produce two newly published NIST post-quantum cryptography standards. IBM Guardium Quantum Safe, which is available on IBM Guardium Data Security Center, keeps an eye on how your company uses cryptography, identifies cryptographic flaws, and ranks remediation in order to protect your data from both traditional and quantum-enabled threats.
Advantages
All-encompassing, combined visibility
Get better insight into the cryptographic posture, vulnerabilities, and remediation status of your company.
Quicker adherence
In addition to integrating with enterprise issue-tracking systems, users can create and implement policies based on external regulations and internal security policies.
Planning for cleanup more quickly
Prioritizing risks gives you the information you need to create a remediation map that works fast.
Characteristics
Visualization
Get insight into how cryptography is being used throughout the company, then delve deeper to assess the security posture of cryptography.
Keeping an eye on and tracking
Track and evaluate policy infractions and corrections over time with ease.
Prioritizing vulnerabilities
Rapidly learn the priority of vulnerabilities based on non-compliance and commercial effect.
Actions motivated by policy
Integrate with IT issue-tracking systems to manage policy breaches that have been defined by the user and expedite the remedy process.
Organizations must increase their crypto-agility and closely monitor their AI models, training data, and usage during this revolutionary period. With its AI Security, Quantum Safe, and other integrated features, IBM Guardium Data Security Center offers thorough risk visibility.
In order to identify vulnerabilities and direct remediation, IBM Guardium Quantum Safe assists enterprises in managing their enterprise cryptographic security posture and gaining visibility. By combining crypto algorithms used in code, vulnerabilities found in code, and network usages into a single dashboard, it enables organizations to enforce policies based on external, internal, and governmental regulations. This eliminates the need for security analysts to piece together data dispersed across multiple systems, tools, and departments in order to monitor policy violations and track progress. Guardium Quantum Safe provides flexible reporting and configurable metadata to prioritize fixing serious vulnerabilities.
For sensitive AI data and AI models, IBM Guardium AI Security handles data governance and security risk. Through a shared perspective of data assets, it assists in identifying AI deployments, addressing compliance, mitigating risks, and safeguarding sensitive data in AI models. IBM Watsonx and other generative AI software as a service providers are integrated with IBM Guardium AI Security. To ensure that “shadow AI” models no longer elude governance, IBM Guardium AI Security, for instance, assists in the discovery of these models and subsequently shares them with IBM Watsonx.governance.
An integrated strategy for a period of transformation
Risks associated with the hybrid cloud, artificial intelligence, and quantum era necessitate new methods of protecting sensitive data, including financial transactions, medical information, intellectual property, and vital infrastructure. Organizations desperately need a reliable partner and an integrated strategy to data protection during this revolutionary period, not a patchwork of discrete solutions. This integrated strategy is being pioneered by IBM.
IBM Consulting and Research’s more comprehensive Quantum Safe products complement IBM Guardium Quantum Safe. IBM Research has produced the technology and research that powers the software. The U.S. National Institute of Standards and Technology (NIST) recently standardized a number of IBM Research’s post-quantum cryptography algorithms, which is an important step in preventing future cyberattacks by malicious actors who might obtain access to cryptographically significant quantum computers.
These technologies are used by IBM Consulting’s Quantum Safe Transformation Services to assist organizations in identifying risks, prioritizing and inventorying them, addressing them, and then scaling the process. Numerous experts in cryptography and quantum safe technologies are part of IBM Consulting’s cybersecurity team. IBM Quantum Safe Transformation Services are used by dozens of clients in the government, financial, telecommunications, and other sectors to help protect their companies from existing and future vulnerabilities, such as harvest now, decrypt later.
Additionally, IBM is expanding its Verify offering today with decentralized identity features: Users can save and manage their own credentials with IBM Verify Digital Credentials. Physical credentials such as driver’s licenses, insurance cards, loyalty cards, and employee badges are digitized by the feature so they may be standardized, saved, and shared with complete control, privacy protection, and security. Identity protection throughout the hybrid cloud is provided by IBM Verify, an IAM (identity access management) service.
Statements on IBM’s future direction and intent are merely goals and objectives and are subject to change or withdrawal at any time.
Read more on govindhtech.com
#IBMGuardium#DataSecurityCenter#BoostsAI#QuantumSafety#AImodel#artificialintelligence#AIsecurity#IBMWatsonxgovernance#quantumcomputers#technology#technews#news#IBMWatsonx#NationalInstituteStandardsTechnology#NIST#govindhtech
0 notes
Text
IBM Watsonx.data Offers VSCode, DBT & Airflow Dataops Tools
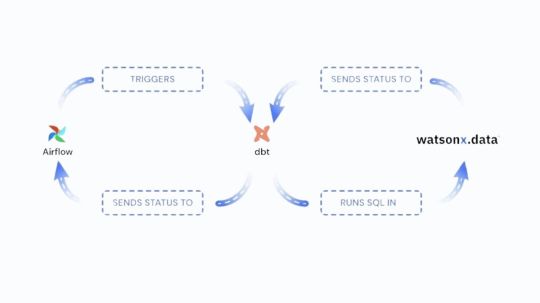
We are happy to inform that VSCode, Apache Airflow, and data-build-tool a potent set of tools for the contemporary dataops stack are now supported by IBM watsonx.data. IBM Watsonx.data delivers a new set of rich capabilities, including data build tool (dbt) compatibility for both Spark and Presto engines, automated orchestration with Apache Airflow, and an integrated development environment via VSCode. These functionalities enable teams to effectively construct, oversee, and coordinate data pipelines.
The difficulty with intricate data pipelines
Building and maintaining complicated data pipelines that depend on several engines and environments is a challenge that organizations must now overcome. Teams must continuously move between different languages and tools, which slows down development and adds complexity.
It can be challenging to coordinate workflows across many platforms, which can result in inefficiencies and bottlenecks. Data delivery slows down in the absence of a smooth orchestration tool, which postpones important decision-making.
A coordinated strategy
Organizations want a unified, efficient solution that manages process orchestration and data transformations in order to meet these issues. Through the implementation of an automated orchestration tool and a single, standardized language for transformations, teams can streamline their workflows, facilitating communication and lowering the difficulty of pipeline maintenance. Here’s where Apache Airflow and DBT come into play.
Teams no longer need to learn more complicated languages like PySpark or Scala because dbt makes it possible to develop modular structured query language (SQL) code for data transformations. The majority of data teams are already familiar with SQL, thus database technology makes it easier to create, manage, and update transformations over time.
Throughout the pipeline, Apache Airflow automates and schedules jobs to minimize manual labor and lower mistake rates. When combined, dbt and Airflow offer a strong framework for easier and more effective management of complicated data pipelines.
Utilizing IBM watsonx.data to tie everything together
Although strong solutions like Apache Airflow and DBT are available, managing a developing data ecosystem calls for more than just a single tool. IBM Watsonx.data adds the scalability, security, and dependability of an enterprise-grade platform to the advantages of these tools. Through the integration of VSCode, Airflow, and DBT within watsonx.data, it has developed a comprehensive solution that makes complex data pipeline management easier:
By making data transformations with SQL simpler, dbt assists teams in avoiding the intricacy of less used languages.
By automating orchestration, Airflow streamlines processes and gets rid of bottlenecks.
VSCode offers developers a comfortable environment that improves teamwork and efficiency.
This combination makes pipeline management easier, freeing your teams to concentrate on what matters most: achieving tangible business results. IBM Watsonx.data‘s integrated solutions enable teams to maintain agility while optimizing data procedures.
Data Build Tool’s Spark adaptor
The data build tool (dbt) adapter dbt-watsonx-spark is intended to link Apache Spark with dbt Core. This adaptor facilitates Spark data model development, testing, and documentation.
FAQs
What is data build tool?
A transformation workflow called dbt enables you to complete more tasks with greater quality. Dbt can help you centralize and modularize your analytics code while giving your data team the kind of checks and balances that are usually seen in software engineering workflows. Before securely delivering data models to production with monitoring and visibility, work together on them, version them, test them, and record your queries.
DBT allows you and your team to work together on a single source of truth for metrics, insights, and business definitions by compiling and running your analytics code against your data platform. Having a single source of truth and the ability to create tests for your data helps to minimize errors when logic shifts and notify you when problems occur.
Read more on govindhtech.com
#IBMWatsonx#dataOffer#VSCode#DBT#data#ApacheSpark#ApacheAirflow#Watsonxdata#DataopsTools#databuildtool#Sparkadaptor#UtilizingIBMwatsonxdata#technology#technews#news#govindhteh
0 notes
Text
IBM Watsonx Assistant For Z V2’s Document Ingestion Feature
IBM Watsonx Assistant for Z
For a more customized experience, clients can now choose to have IBM Watsonx Assistant for Z V2 ingest their enterprise documents.
A generative AI helper called IBM Watsonx Assistant for Z was introduced earlier this year at Think 2024. This AI assistant transforms how your Z users interact with and use the mainframe by combining conversational artificial intelligence (AI) and IT automation in a novel way. By allowing specialists to formalize their Z expertise, it helps businesses accelerate knowledge transfer, improve productivity, autonomy, and confidence for all Z users, and lessen the learning curve for early-tenure professionals.
Building on this momentum, IBM is announce today the addition of new features and improvements to IBM Watsonx Assistant for Z. These include:
Integrate your own company documents to facilitate the search for solutions related to internal software and procedures.
Time to value is accelerated by prebuilt skills (automations) offered for typical IBM z/OS jobs.
Simplified architecture to reduce costs and facilitate implementation.
Consume your own business records
Every organization has its own workflows, apps, technology, and processes that make it function differently. Over time, many of these procedures have been improved, yet certain specialists are still routinely interrupted to answer simple inquiries.
You can now easily personalize the Z RAG by ingesting your own best practices and documentation with IBM Watsonx Assistant for Z. Your Z users will have more autonomy when you personalize your Z RAG because they will be able to receive answers that are carefully chosen to fit the internal knowledge, procedures, and environment of your company.
Using a command-line interface (CLI), builders may import text, HTML, PDF, DOCX, and other proprietary and third-party documentation at scale into retrieval augmented generation (RAG). There’s no need to worry about your private content being compromised because the RAG is located on-premises and protected by a firewall
What is Watsonx Assistant for Z’s RAG and why is it relevant?
A Z domain-specific RAG and a chat-focused granite.13b.labrador model are utilized by IBM Watsonx Assistant for Z, which may be improved using your company data. The large language model (LLM) and RAG combine to provide accurate and contextually rich answers to complex queries. This reduces the likelihood of hallucinations for your internal applications, processes, and procedures as well as for IBM Z products. The answers also include references to sources.
Built-in abilities for a quicker time to value
For common z/OS tasks, organizations can use prebuilt skills that are accessible. This means that, without specialist knowledge, you may quickly combine automations like displaying all subsystems, determining when a program temporary fix (PTF) was installed, or confirming the version level of a product that is now operating on a system into an AI assistant to make it easier for your Z users to use them.
Additionally, your IBM Z professionals can accelerate time to value by using prebuilt skills to construct sophisticated automations and skill flows for specific use cases more quickly.
Streamlined architecture for easier deployment and more economical use
IBM Watsonx Discovery, which was required in order to provide elastic search, is no longer mandatory for organizations. Alternatively, they can utilize the integrated OpenSearch feature, which combines semantic and keyword searches to provide access to the Z RAG. This update streamlines the deployment process and greatly reduces the cost of owning IBM Watsonx Assistant for Z in addition to improving response quality.
Use IBM Watsonx Assistant for Z to get started
By encoding information into a reliable set of automations, IBM Watsonx Assistant for Z streamlines the execution of repetitive operations and provides your Z users with accurate and current answers to their Z questions.
Read more on govindhtech.com
#IBM#WatsonxAssistant#ZV2Document#IBMZ#IngestionFeature#artificialintelligence#AI#RAG#WatsonxAssistantZ#retrievalaugmentedgeneration#IBMWatsonx#automations#technology#technews#news#govindhtech
0 notes
Text
IBM Watsonx.governance Removes Gen AI Adoption Obstacles
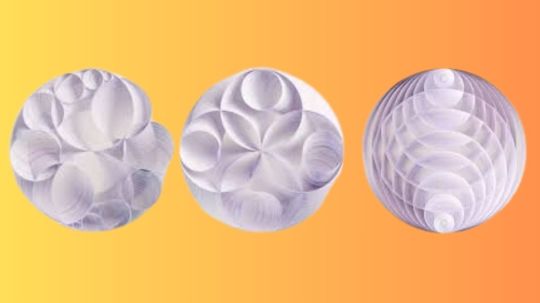
The IBM Watsonx platform, which consists of Watsonx.ai, Watsonx.data, and Watsonx.governance, removes obstacles to the implementation of generative AI.
Complex data environments, a shortage of AI-skilled workers, and AI governance frameworks that consider all compliance requirements put businesses at risk as they explore generative AI’s potential.
Generative AI requires even more specific abilities, such as managing massive, diverse data sets and navigating ethical concerns due to its unpredictable results.
IBM is well-positioned to assist companies in addressing these issues because of its vast expertise using AI at scale. The IBM Watsonx AI and data platform provides solutions that increase the accessibility and actionability of AI while facilitating data access and delivering built-in governance, thereby addressing skills, data, and compliance challenges. With the combination, businesses may fully utilize AI to accomplish their goals.
Forrester Research’s The Forrester Wave: AI/ML Platforms, Q3, 2024, by Mike Gualtieri and Rowan Curran, published on August 29, 2024, is happy to inform that IBM has been rated as a strong performer.
IBM is said to provide a “one-stop AI platform that can run in any cloud” by the Forrester Report. Three key competencies enable IBM Watsonx to fulfill its goal of becoming a one-stop shop for AI platforms: Using Watsonx.ai, models, including foundation models, may be trained and used. To store, process, and manage AI data, use watsonx.data. To oversee and keep an eye on all AI activity, use watsonx.governance.
Watsonx.ai
Watsonx.ai: a pragmatic method for bridging the AI skills gap
The lack of qualified personnel is a significant obstacle to AI adoption, as indicated by IBM’s 2024 “Global AI Adoption Index,” where 33% of businesses cite this as their top concern. Developing and implementing AI models calls both certain technical expertise as well as the appropriate resources, which many firms find difficult to come by. By combining generative AI with conventional machine learning, IBM Watsonx.ai aims to solve these problems. It consists of runtimes, models, tools, and APIs that make developing and implementing AI systems easier and more scalable.
Let’s say a mid-sized retailer wants to use demand forecasting powered by artificial intelligence. Creating, training, and deploying machine learning (ML) models would often require putting together a team of data scientists, which is an expensive and time-consuming procedure. The reference customers questioned for The Forrester Wave AI/ML Platforms, Q3 2024 report said that even enterprises with low AI knowledge can quickly construct and refine models with watsonx.ai’s “easy-to-use tools for generative AI development and model training .”
For creating, honing, and optimizing both generative and conventional AI/ML models and applications, IBM Watsonx.ai offers a wealth of resources. To train a model for a specific purpose, AI developers can enhance the performance of pre-trained foundation models (FM) by fine-tuning parameters efficiently through the Tuning Studio. Prompt Lab, a UI-based tools environment offered by Watsonx.ai, makes use of prompt engineering strategies and conversational engagements with FMs.
Because of this, it’s simple for AI developers to test many models and learn which one fits the data the best or what needs more fine tuning. The watsonx.ai AutoAI tool, which uses automated machine learning (ML) training to evaluate a data set and apply algorithms, transformations, and parameter settings to produce the best prediction models, is another tool available to model makers.
It is their belief that the acknowledgement from Forrester further confirms IBM’s unique strategy for providing enterprise-grade foundation models, assisting customers in expediting the integration of generative AI into their operational processes while reducing the risks associated with foundation models.
The watsonx.ai AI studio considerably accelerates AI deployment to suit business demands with its collection of pre-trained, open-source, and bespoke foundation models from third parties, in addition to its own flagship Granite series. Watsonx.ai makes AI more approachable and indispensable to business operations by offering these potent tools that help companies close the skills gap in AI and expedite their AI initiatives.
Watsonx.data
Real-world methods for addressing data complexity using Watsonx.data
As per 25% of enterprises, data complexity continues to be a significant hindrance for businesses attempting to utilize artificial intelligence. It can be extremely daunting to deal with the daily amount of data generated, particularly when it is dispersed throughout several systems and formats. These problems are addressed by IBM Watsonx.Data, an open, hybrid, and controlled data store that is suitable for its intended use.
Its open data lakehouse architecture centralizes data preparation and access, enabling tasks related to artificial intelligence and analytics. Consider, for one, a multinational manufacturing corporation whose data is dispersed among several regional offices. Teams would have to put in weeks of work only to prepare this data manually in order to consolidate it for AI purposes.
By providing a uniform platform that makes data from multiple sources more accessible and controllable, Watsonx.data can help to simplify this. To make the process of consuming data easier, the Watsonx platform also has more than 60 data connections. The software automatically displays summary statistics and frequency when viewed data assets. This makes it easier to quickly understand the content of the datasets and frees up a business to concentrate on developing its predictive maintenance models, for example, rather than becoming bogged down in data manipulation.
Additionally, IBM has observed via a number of client engagement projects that organizations can reduce the cost of data processing by utilizing Watsonx.data‘s workload optimization, which increases the affordability of AI initiatives.
In the end, AI solutions are only as good as the underlying data. A comprehensive data flow or pipeline can be created by combining the broad capabilities of the Watsonx platform for data intake, transformation, and annotation. For example, the platform’s pipeline editor makes it possible to orchestrate operations from data intake to model training and deployment in an easy-to-use manner.
As a result, the data scientists who create the data applications and the ModelOps engineers who implement them in real-world settings work together more frequently. Watsonx can assist enterprises in managing their complex data environments and reducing data silos, while also gaining useful insights from their data projects and AI initiatives. Watsonx does this by providing comprehensive data management and preparation capabilities.
Watsonx.Governance
Using Watsonx.Governance to address ethical issues: fostering openness to establish trust
With ethical concerns ranking as a top obstacle for 23% of firms, these issues have become a significant hurdle as AI becomes more integrated into company operations. In industries like finance and healthcare, where AI decisions can have far-reaching effects, fundamental concerns like bias, model drift, and regulatory compliance are particularly important. With its systematic approach to transparent and accountable management of AI models, IBM Watsonx.governance aims to address these issues.
The organization can automate tasks like identifying bias and drift, doing what-if scenario studies, automatically capturing metadata at every step, and using real-time HAP/PII filters by using watsonx.governance to monitor and document its AI model landscape. This supports organizations’ long-term ethical performance.
By incorporating these specifications into legally binding policies, Watsonx.governance also assists companies in staying ahead of regulatory developments, including the upcoming EU AI Act. By doing this, risks are reduced and enterprise trust among stakeholders, including consumers and regulators, is strengthened. Organizations can facilitate the responsible use of AI and explainability across various AI platforms and contexts by offering tools that improve accountability and transparency. These tools may include creating and automating workflows to operationalize best practices AI governance.
Watsonx.governance also assists enterprises in directly addressing ethical issues, guaranteeing that their AI models are trustworthy and compliant at every phase of the AI lifecycle.
IBM’s dedication to preparing businesses for the future through seamless AI integration
IBM’s AI strategy is based on the real-world requirements of business operations. IBM offers a “one-stop AI platform” that helps companies grow their AI activities across hybrid cloud environments, as noted by Forrester in their research. IBM offers the tools necessary to successfully integrate AI into key business processes. Watsonx.ai empowers developers and model builders to support the creation of AI applications, while Watsonx.data streamlines data management. Watsonx.governance manages, monitors, and governs AI applications and models.
As generative AI develops, businesses require partners that are fully versed in both the technology and the difficulties it poses. IBM has demonstrated its commitment to open-source principles through its design, as evidenced by the release of a family of essential Granite Code, Time Series, Language, and GeoSpatial models under a permissive Apache 2.0 license on Hugging Face. This move allowed for widespread and unrestricted commercial use.
Watsonx is helping IBM create a future where AI improves routine business operations and results, not just helping people accept AI.
Read more on govindhteh.com
#IBMWatsonx#governanceRemoves#GenAI#AdoptionObstacles#IBMWatsonxAI#fm#ml#machinelearningmodels#foundationmodels#AImodels#IBMWatsonxData#datalakehouse#Watsonxplatform#IBMoffers#AIgovernance#ibm#techniligy#technews#news#govindhtech
0 notes
Text
Meta Unveils Llama 3.1: A Challenger in the AI Arena
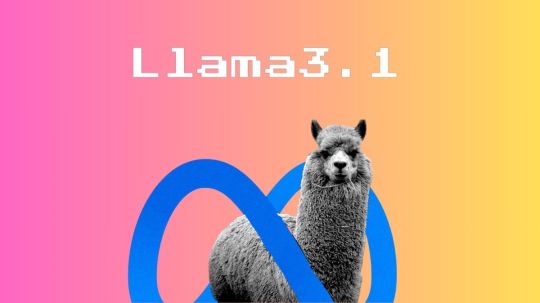
Meta launches new Llama 3.1 models, including anticipated 405B parameter version.
Meta released Llama 3.1, a multilingual LLM collection. Llama 3.1 includes pretrained and instruction-tuned text in/text out open source generative AI models with 8B, 70B, and 405B parameters.
Today, IBM watsonx.ai will offer the instruction-tuned Llama 3.1-405B, the largest and most powerful open source language model available and competitive with the best proprietary models.It can be set up on-site, in a hybrid cloud environment, or on the IBM cloud.
Llama 3.1 follows the April 18 debut of Llama 3 models. Meta stated in the launch release that “[their] goal in the near future is to make Llama 3 multilingual and multimodal, have longer context, and continue to improve overall performance across LLM capabilities such as reasoning and coding.”
Llama 3.1’s debut today shows tremendous progress towards that goal, from dramatically enhanced context length to tool use and multilingual features.
An significant step towards open, responsible, accessible AI innovation
Meta and IBM launched the AI Alliance in December 2023 with over 50 global initial members and collaborators. The AI Alliance unites leading business, startup, university, research, and government organisations to guide AI’s evolution to meet society’s requirements and complexities. Since its formation, the Alliance has over 100 members.
Additionally, the AI Alliance promotes an open community that helps developers and researchers accelerate responsible innovation while maintaining trust, safety, security, diversity, scientific rigour, and economic competitiveness. To that aim, the Alliance supports initiatives that develop and deploy benchmarks and evaluation standards, address society-wide issues, enhance global AI capabilities, and promote safe and useful AI development.
Llama 3.1 gives the global AI community an open, state-of-the-art model family and development ecosystem to explore, experiment, and responsibly scale new ideas and techniques. The release features strong new models, system-level safety safeguards, cyber security evaluation methods, and improved inference-time guardrails. These resources promote generative AI trust and safety tool standardisation.
How Llama 3.1-405B compares to top models
The April release of Llama 3 highlighted upcoming Llama models with “over 400B parameters” and some early model performance evaluation, but their exact size and details were not made public until today’s debut. Llama 3.1 improves all model sizes, but the 405B open source model matches leading proprietary, closed source LLMs for the first time.
Looking beyond numbers
Performance benchmarks are not the only factor when comparing the 405B to other cutting-edge models. Llama 3.1-405B may be built upon, modified, and run on-premises, unlike its closed source contemporaries, which can change their model without notice. That level of control and predictability benefits researchers, businesses, and other entities that seek consistency and repeatability.
Effective Llama-3.1-405B usage
IBM, like Meta, believes open models improve product safety, innovation, and the AI market. An advanced 405B-parameter open source model offers unique potential and use cases for organisations of all sizes.
Aside from inference and text creation, which may require quantisation or other optimisation approaches to execute locally on most hardware systems, the 405B can be used for:
Synthetic data can fill the gap in pre-training, fine-tuning, and instruction tuning when data is limited or expensive. The 405B generates high-quality task- and domain-specific synthetic data for LLM training. IBM’s Large-scale Alignment for chatBots (LAB) phased-training approach quickly updates LLMs with synthetic data while conserving model knowledge.
The 405B model’s knowledge and emergent abilities can be reduced into a smaller model, combining the capabilities of a big “teacher” model with the quick and cost-effective inference of a “student” model (such an 8B or 70B Llama 3.1). Effective Llama-based models like Alpaca and Vicuna need knowledge distillation, particularly instruction tailoring on synthetic data provided by bigger GPT models.
LLM-as-a-judge: The subjectivity of human preferences and the inability of standards to approximate them make LLM evaluation difficult. The Llama 2 research report showed that larger models can impartially measure response quality in other models. Learn more about LLM-as-a-judge’s efficacy in this 2023 article.
A powerful domain-specific fine-tune: Many leading closed models allow fine-tuning only on a case-by-case basis, for older or smaller model versions, or not at all. Meta has made Llama 3.1-405B accessible for pre-training (to update the model’s general knowledge) or domain-specific fine-tuning coming soon to the watsonx Tuning Studio.
Meta AI “strongly recommends” using a platform like IBM watsonx for model evaluation, safety guardrails, and retrieval augmented generation to deploy Llama 3.1 models.
Every llama 3.1 size gets upgrades
The long-awaited 405B model may be the most notable component of Llama 3.1, but it’s hardly the only one. Llama 3.1 models share the dense transformer design of Llama 3, but they are much improved at all model sizes.
Longer context windows
All pre-trained and instruction-tuned Llama 3.1 models have context lengths of 128,000 tokens, a 1600% increase over 8,192 tokens in Llama 3. Llama 3.1’s context length is identical to the enterprise version of GPT-4o, substantially longer than GPT-4 (or ChatGPT Free), and comparable to Claude 3’s 200,000 token window. Llama 3.1’s context length is not constrained in situations of high demand because it can be installed on the user’s hardware or through a cloud provider.. Llama 3.1 has few usage restrictions.
An LLM can consider or “remember” a certain amount of tokenised text (called its context window) at any given moment. To continue, a model must trim or summarise a conversation, document, or code base that exceeds its context length. Llama 3.1’s extended context window lets models have longer discussions without forgetting details and ingest larger texts or code samples during training and inference.
Text-to-token conversion doesn’t have a defined “exchange rate,” but 1.5 tokens per word is a good estimate. Thus, Llama 3.1’s 128,000 token context window contains 85,000 words. The Hugging Face Tokeniser Playground lets you test multiple tokenisation models on text inputs.
Llama 3.1 models benefit from Llama 3’s new tokeniser, which encodes language more effectively than Llama 2.
Protecting safety
Meta has cautiously and thoroughly expanded context length in line with its responsible innovation approach. Previous experimental open source attempts produced Llama derivatives with 128,000 or 1M token windows. These projects demonstrate Meta’s open model commitment, however they should be approached with caution: Without strong countermeasures, lengthy context windows “present a rich new attack surface for LLMs” according to recent study.
Fortunately, Llama 3.1 adds inference guardrails. The release includes direct and indirect prompt injection filtering from Prompt Guard and updated Llama Guard and CyberSec Eval. CodeShield, a powerful inference time filtering technology from Meta, prevents LLM-generated unsafe code from entering production systems.
As with any generative AI solution, models should be deployed on a secure, private, and safe platform.
Multilingual models
Pretrained and instruction tailored Llama 3.1 models of all sizes will be bilingual. In addition to English, Llama 3.1 models speak Spanish, Portuguese, Italian, German, and Thai. Meta said “a few other languages” are undergoing post-training validation and may be released.
Optimised for tools
Meta optimised the Llama 3.1 Instruct models for “tool use,” allowing them to interface with applications that enhance the LLM’s capabilities. Training comprises creating tool calls for specific search, picture production, code execution, and mathematical reasoning tools, as well as zero-shot tool use—the capacity to effortlessly integrate with tools not previously encountered in training.
Starting Llama 3.1
Meta’s latest version allows you to customise state-of-the-art generative AI models for your use case.
IBM supports Llama 3.1 to promote open source AI innovation and give clients access to best-in-class open models in watsonx, including third-party models and the IBM Granite model family.
IBM Watsonx allows clients to deploy open source models like Llama 3.1 on-premises or in their preferred cloud environment and use intuitive workflows for fine-tuning, prompt engineering, and integration with enterprise applications. Build business-specific AI apps, manage data sources, and expedite safe AI workflows on one platform.
Read more on govindhtech.com
#MetaUnveilsLlama31#AIArena#generativeAImodels#Llama3#LLMcapabilities#AIcapabilities#generativeAI#watsonx#LLMevaluation#IBMwatsonx#gpt40#Llama2#Optimisedtools#Multilingualmodels#AIworkflows#technology#technews#news#govindhtech
0 notes
Text
Mizuho & IBM Partner on Gen AI PoC to Boost Recovery Times
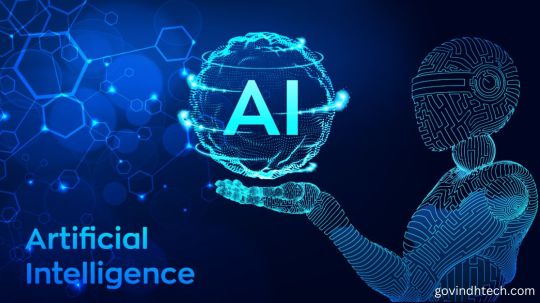
IBM and Mizuho Financial Group, worked together to create a proof of concept (PoC) that uses IBM’s enterprise generative AI and data platform, watsonx, to increase the effectiveness and precision of Mizuho’s event detection processes.
During a three-month trial, the new technology showed 98% accuracy in monitoring and reacting to problem alerts. Future system expansion and validation are goals shared by IBM and Mizuho.
The financial systems need to recover accurately and quickly after an interruption.
However, operators frequently receive a flood of messages and reports when an error is detected, which makes it challenging to identify the event’s source and ultimately lengthens the recovery period.
In order to solve the problem, IBM and Mizuho carried out a Proof of Concept using Watsonx to increase error detection efficiency.
In order to reduce the number of steps required for recovery and accelerate recovery, the Proof of Concept (PoC) integrated an application that supported a series of processes in event detection with Watsonx and incorporated patterns likely to result in errors in incident response.
By utilising Watsonx, Mizuho was also able to streamline internal operations and enable people on-site to configure monitoring and operating menus in a flexible manner when greater security and secrecy are needed.
In the upcoming year, Mizuho and IBM intend to extend the event detection and reaction proof of concept and integrate it into real-world settings. To increase operational efficiency and sophistication, Mizuho and IBM also intend to cooperate on incident management and advanced failure analysis using generative AI.
WatsonX for Proof of Concept (PoC)
IBM’s WatsonX is an enterprise-grade platform that integrates generative AI capabilities with data. It is therefore an effective tool for creating and evaluating AI solutions. This is a thorough examination of WatsonX use for a PoC:
Why Would a PoC Use WatsonX?
Streamlined Development: WatsonX can expedite the Proof of Concept development process by providing a pre-built component library and an intuitive UI.
Data Integration: It is simpler to include the data required for your AI model since the platform easily integrates with a variety of data sources.
AI Capabilities: WatsonX comes with a number of built-in AI features, including as computer vision, machine learning, and natural language processing, which let you experiment with alternative strategies within your proof of concept.
Scalability: As your solution develops, the platform can handle handling small-scale experimentation for your Proof of Concept.
How to Use a WatsonX PoC
Establish your objective: Clearly state the issue you’re attempting to resolve or the procedure you wish to use AI to enhance. What precise results are you hoping to achieve with your PoC?
Collect Information: Determine the kind of data that your AI model needs to be trained on. Make that the information is adequate, correct, and pertinent for the PoC’s scope.
Select Usability: Based on your objective, choose the WatsonX AI functionalities that are most relevant. Computer vision for image recognition, machine learning for predictive modelling, and natural language processing for sentiment analysis are examples.
Create a PoC: Create a rudimentary AI solution using WatsonX’s tools and frameworks. This could entail building a prototype with restricted functionality or training a basic model.
Test & Assess: Utilise real-world data to evaluate your proof of concept’s efficacy. Examine the outcomes to determine whether the intended goals were met. Point out any places that need work.
Refine and Present: Make iterations to the data, model, or functions to improve your Proof of Concept based on your assessment. Present your results to stakeholders at the end, highlighting the PoC’s shortcomings and areas for future improvement.
Extra Things to Think About
Keep your point of contact (PoC) concentrated on a single issue or task. At this point, don’t try to develop a complete solution.
Success Criteria: Prior to implementation, clearly define your PoC’s success metrics. This may entail cost-cutting, efficiency, or accuracy.
Ethical Considerations: Consider the ethical ramifications of your AI solution as well as any potential biases in your data.
Goal of Collaboration:
Boost Mizuho’s event detection and reaction activities’ precision and efficiency.
Technology Employed:
IBM’s WatsonX is a platform for corporate generative AI and data.
What they Found Out:
Created a proof of concept (PoC) that monitors error signals and reacts with WatsonX.
Over the course of a three-month study, the PoC attained a 98% accuracy rate.
Future Objectives:
Increase the breadth of the event detection and response situations covered by the PoC.
After a year, implement the solution in actual production settings.
To further streamline operations, investigate the use of generative AI for enhanced failure analysis and issue management.
Total Effect:
This project demonstrates how generative AI has the ability to completely transform the banking industry’s operational effectiveness. Mizuho hopes to increase overall business continuity and drastically cut down on recovery times by automating incident detection and reaction.
Emphasis on Event Detection and Response: The goal of the project is to enhance Mizuho’s monitoring and error-messaging processes by employing generative AI, notably IBM’s WatsonX platform.
Effective Proof of Concept (PoC): Mizuho and IBM worked together to create a PoC that showed a notable increase in accuracy. During a three-month trial period, the AI system identified and responded to error notifications with a 98% success rate.
Future Plans: Mizuho and IBM intend to grow the project in the following ways in light of the encouraging Proof of Concept outcomes:
Production Deployment: During the course of the upcoming year, they hope to incorporate the event detection and response system into Mizuho’s live operations environment.
Advanced Applications: Through this partnership, generative AI will be investigated for use in increasingly difficult activities such as advanced failure analysis and incident management. This might entail the AI automatically identifying problems and making recommendations for fixes, therefore expediting the healing procedures.
Overall Impact: This project demonstrates how generative AI has the ability to completely transform financial institutions’ operational efficiency. Mizuho may be able to lower interruption costs, boost overall service quality, and cut downtime by automating fault detection and response.
Consider these other points:
Generative AI can identify and predict flaws by analysing historical data and finding patterns. The calibre and applicability of the data utilised to train the AI models will determine this initiative’s success.
Read more on Govindhtech.com
0 notes
Text
IBM Think 2024 Conference: Scaling AI for Business Success
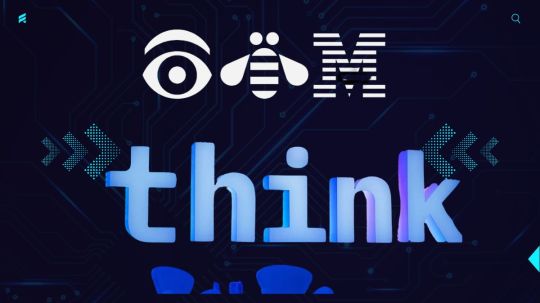
IBM Think Conference
Today at its annual THINK conference, IBM revealed many new enhancements to its watsonx platform one year after its launch, as well as planned data and automation features to make AI more open, cost-effective, and flexible for enterprises. IBM CEO Arvind Krishna will discuss the company’s goal to invest in, create, and contribute to the open-source AI community during his opening address.
“Open innovation in AI is IBM’s philosophy. Krishna said they wanted to use open source to do with AI what Linux and OpenShift did. “Open means choice. Open means more eyes on code, brains on issues, and hands on solutions. Competition, innovation, and safety must be balanced for any technology to gain pace and become universal. Open source helps achieve all three.”
IBM published Granite models as open source and created InstructLab, a first-of-its-kind capability, with Red Hat
IBM has open-sourced its most advanced and performant language and code Granite models, demonstrating its commitment to open-source AI. IBM is urging clients, developers, and global experts to build on these capabilities and push AI’s limits in enterprise environments by open sourcing these models.
Granite models, available under Apache 2.0 licences on Hugging Face and GitHub, are known for their quality, transparency, and efficiency. Granite code models have 3B to 34B parameters and base and instruction-following model versions for complicated application modernization, code generation, bug repair, code documentation, repository maintenance, and more. Code models trained on 116 programming languages regularly outperform open-source code LLMs in code-related tasks:
IBM tested Granite Code models across all model sizes and benchmarks and found that they outperformed open-source code models twice as large.
IBM found Granite code models perform well on HumanEvalPack, HumanEvalPlus, and reasoning benchmark GSM8K for code synthesis, fixing, explanation, editing, and translation in Python, JavaScript, Java, Go, C++, and Rust.
IBM Watsonx Code Assistant (WCA) was trained for specialised areas using the 20B parameter Granite base code model. Watsonx Code Assistant for Z helps organisations convert monolithic COBOL systems into IBM Z-optimized services.
The 20B parameter Granite base code model generates SQL from natural language questions to change structured data and gain insights. IBM led in natural language to SQL, a major industry use case, according to BIRD’s independent leaderboard, which rates models by Execution Accuracy (EX) and Valid Efficiency Score.
IBM and Red Hat announced InstructLab, a groundbreaking LLM open-source innovation platform.
Like open-source software development for decades, InstructLab allows incremental improvements to base models. Developers can use InstructLab to construct models with their own data for their business domains or sectors to understand the direct value of AI, not only model suppliers. Through watsonx.ai and the new Red Hat Enterprise Linux AI (RHEL AI) solution, IBM hopes to use these open-source contributions to deliver value to its clients.
RHEL AI simplifies AI implementation across hybrid infrastructure environments with an enterprise-ready InstructLab, IBM’s open-source Granite models, and the world’s best enterprise Linux platform.
IBM Consulting is also developing a practice to assist clients use InstructLab with their own private data to train purpose-specific AI models that can be scaled to meet an enterprise’s cost and performance goals.
IBM introduces new Watsonx assistants
This new wave of AI innovation might provide $4 trillion in annual economic benefits across industries. IBM’s annual Global AI Adoption Index indicated that 42% of enterprise-scale organisations (> 1,000 people) have adopted AI, but 40% of those investigating or experimenting with AI have yet to deploy their models. The skills gap, data complexity, and, most crucially, trust must be overcome in 2024 for sandbox companies.
IBM is unveiling various improvements and enhancements to its watsonx assistants, as well as a capability in watsonx Orchestrate to allow clients construct AI Assistants across domains, to solve these difficulties.
Watsonx Assistant for Z
The new AI Assistants include watsonx Code Assistant for Enterprise Java Applications (planned availability in October 2024), watsonx Assistant for Z to transform how users interact with the system to quickly transfer knowledge and expertise (planned availability in June 2024), and an expansion of watsonx Code Assistant for Z Service with code explanation to help clients understand and document applications through natural language.
To help organisations and developers meet AI and other mission-critical workloads, IBM is adding NVIDIA L40S and L4 Tensor Core GPUs and support for Red Hat Enterprise Linux AI (RHEL AI) and OpenShift AI. IBM is also leveraging deployable designs for watsonx to expedite AI adoption and empower organisations with security and compliance tools to protect their data and manage compliance rules.
IBM also introduced numerous new and future generative AI-powered data solutions and capabilities to help organisations observe, govern, and optimise their increasingly robust and complex data for AI workloads. Get more information on the IBM Data Product Hub, Data Gate for watsonx, and other updates on watsonx.data.
IBM unveils AI-powered automation vision and capabilities
Company operations are changing with hybrid cloud and AI. The average company manages public and private cloud environments and 1,000 apps with numerous dependencies. Both handle petabytes of data. Automating is no longer an option since generative AI is predicted to drive 1 billion apps by 2028. Businesses will save time, solve problems, and make choices faster.
IBM’s AI-powered automation capabilities will help CIOs evolve from proactive IT management to predictive automation. An enterprise’s infrastructure’s speed, performance, scalability, security, and cost efficiency will depend on AI-powered automation.
Today, IBM’s automation, networking, data, application, and infrastructure management tools enable enterprises manage complex IT infrastructures. Apptio helps technology business managers make data-driven investment decisions by clarifying technology spend and how it produces business value, allowing them to quickly adapt to changing market conditions. Apptio, Instana for automated observability, and Turbonomic for performance optimisation can help clients efficiently allocate resources and control IT spend through enhanced visibility and real-time insights, allowing them to focus more on deploying and scaling AI to drive new innovative initiatives.
IBM recently announced its intent to acquire HashiCorp, which automates multi-cloud and hybrid systems via Terraform, Vault, and other Infrastructure and Security Lifecycle Management tools. HashiCorp helps companies transition to multi-cloud and hybrid cloud systems.
IBM Concert
IBM is previewing IBM Concert, a generative AI-powered tool that will be released in June 2024, at THINK. IBM Concert will be an enterprise’s technology and operational “nerve centre.”
IBM Concert will use watsonx AI to detect, anticipate, and offer solutions across clients’ application portfolios. The new tool integrates into clients’ systems and uses generative AI to generate a complete image of their connected apps utilising data from their cloud infrastructure, source repositories, CI/CD pipelines, and other application management solutions.
Concert informs teams so they can quickly solve issues and prevent them by letting customers minimise superfluous work and expedite others. Concert will first enable application owners, SREs, and IT leaders understand, prevent, and resolve application risk and compliance management challenges.
IBM adds watsonx ecosystem access, third-party models
IBM continues to build a strong ecosystem of partners to offer clients choice and flexibility by bringing third-party models onto watsonx, allowing leading software companies to embed watsonx capabilities into their technologies, and providing IBM Consulting expertise for enterprise business transformation Global generative AI expertise at IBM Consulting has grown to over 50,000 certified practitioners in IBM and strategic partner technologies. Large and small partners help clients adopt and scale personalised AI across their businesses.
IBM and AWS are integrating Amazon SageMaker and watsonx.governance on AWS. This product gives Amazon SageMaker clients advanced AI governance for predictive and generative machine learning and AI models. AI risk management and compliance are simplified by clients’ ability to govern, monitor, and manage models across platforms.
Adobe: IBM and Adobe are working on hybrid cloud and AI, integrating Red Hat OpenShift and watsonx to Adobe Experience Platform and considering on-prem and private cloud versions of watsonx.ai and Adobe Acrobat AI Assistant. IBM is also offering Adobe Express assistance to help clients adopt it. These capabilities should arrive in 2H24.
Meta: IBM released Meta Llama 3, the latest iteration of Meta’s open big language model, on watsonx to let organisations innovate with AI. IBM’s cooperation with Meta to drive open AI innovation continues with Llama 3. Late last year, the two businesses created the AI Alliance, a coalition of prominent industry, startup, university, research, and government organisations with over 100 members and partners.
Microsoft: IBM is supporting the watsonx AI and data platform on Microsoft Azure and offering it as a customer-managed solution on Azure Red Hat OpenShift (ARO) through IBM and our business partner ecosystem.
IBM and Mistral AI are forming a strategic partnership to bring their latest commercial models to the watsonx platform, including the leading Mistral Large model, in 2Q24. IBM and Mistral AI are excited to collaborate on open innovation, building on their open-source work.
Palo Alto Networks: IBM and Palo Alto now offer AI-powered security solutions and many projects to increase client security. Read the news release for details.
Salesforce: IBM and Salesforce are considering adding the IBM Granite model series to Salesforce Einstein 1 later this year to add new models for AI CRM decision-making.
SAP: IBM Consulting and SAP are also working to expedite additional customers’ cloud journeys using RISE with SAP to realise the transformative benefits of generative AI for cloud business. This effort builds on IBM and SAP’s Watson AI integration into SAP applications. IBM Granite Model Series is intended to be available throughout SAP’s portfolio of cloud solutions and applications, which are powered by SAP AI Core’s generative AI centre.
IBM introduced the Saudi Data and Artificial Intelligence Authority (SDAIA) ‘ALLaM’ Arabic model on watsonx, bringing language capabilities like multi-Arabic dialect support.
Read more on Govindhtech.com
#IBMThink#IBM#Think#ai#ibmwatsonx#ibmcloud#llama3#metallama3#redhat#linux#technology#technews#news#govindhtech
0 notes
Text
Coding Power: How IBM Granite Models are Shaping Innovation
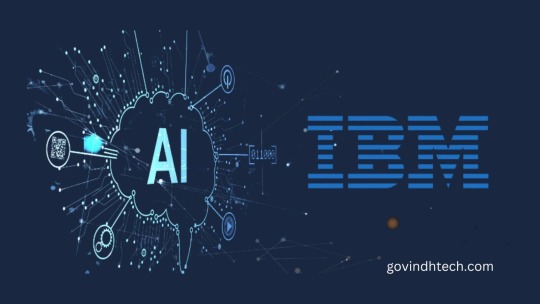
A family of generative AI models created specifically for corporate applications is IBM Granite model. Below is a summary of its salient attributes:
Emphasis on Generative AI: Granite models are helpful for jobs like content generation or code completion because they can generate new text, code, or other data types.
Business-focused: Granite models are designed to meet corporate needs and adhere to standards, having been trained on reliable enterprise data.
A Variety of Models Are Available: The Granite series has a number of versions with varying capacities and sizes to meet different needs.
Open Source Code Models: IBM has made its Granite code model family available as open-source, enabling programmers to use them for a range of coding jobs.
Put Trust and Customisation First: Granite models are designed with an emphasis on transparency and trust, and they can be customised to meet the unique requirements and values of an organisation.
All things considered, companies wishing to include generative AI into their processes can find a strong and flexible answer in IBM’s Granite models.
Optimising Generative AI Quality with Flexible Models
In dynamic gen AI, one-size-fits-all techniques fail. A variety of model options is needed for enterprises to exploit AI’s power:
Encourage creativity
Teams may respond to changing business requirements and consumer expectations by utilising a varied palette of models, which also encourages innovation by bringing unique strengths to bear on a variety of issues.
Customize for edge
A variety of methods enable businesses to do just that. Gen AI is adaptable to several jobs; it can be programmed to write code to generate short summaries or to answer questions in chat applications.
Cut down on time to market
Time is critical in the hectic corporate world of today. Companies may launch AI-powered products more quickly by streamlining the development process with a wide model portfolio. In the context of generation AI, this is particularly important since having access to the newest developments gives one a significant competitive edge.
Remain adaptable as things change
When things change, remember to remain adaptable. Both the market and company tactics are always changing. Companies may make swift and efficient changes by selecting from a variety of models. Having access to a variety of choices helps one to remain flexible and resilient by quickly adapting to emerging trends or strategic changes.
Reduce expenses for all use cases
The costs associated with various models differ. Enterprises can choose the most economical solution for every use case by utilising an assortment of models. More economical models can do certain activities without compromising quality, but others may not be able to handle the accuracy needed for expensive models. For example, in resource and development, accuracy is more important, but in customer care, throughput and latency may be more crucial.
Mitigate risks
Reduce risks by not depending too much on a single model or a small number of options. To ensure that firms are not overly affected by the limitations or failure of a particular strategy, a diversified portfolio of models is helpful in mitigating concentration risks. In addition to distributing risk, this approach offers backup plans in case problems develop.
Respect the law
Ethics are at the forefront of the ever-evolving regulatory landscape surrounding artificial intelligence. Fairness, privacy, and compliance can all be affected differently by different models. Businesses can choose models that adhere to ethical and legal requirements and handle this challenging environment with ease when there is a wide range of options.
Making the appropriate AI model choices
Now that IBM is aware of how important model selection is, how does the company handle the issue of too many options when choosing the best model for a given scenario? This difficult issue can be simplified by IBM into a few easy steps that you may use right now:
Choose an obvious use case
Find out what your company application’s particular needs and requirements are. For the model to closely match your goals, this entails creating intricate prompts that take into account nuances specific to your organisation and industry.
List every option for a model
Consider factors including latency, accuracy, size, and related dangers while evaluating different models. Accuracy, latency, and throughput trade-offs are just a few of the things you need to be aware of while evaluating any model.
Analyse the model’s characteristics
Consider how the model’s scale may impact its performance and the associated risks when you evaluate the model’s features. Determine whether the model’s size is acceptable for your purposes. Choosing the appropriate model size is crucial in this step, as larger models aren’t always better for the use case. In specific domains and application circumstances, smaller models can surpass larger ones in performance.
Test model options
Try out different model options to determine if the model behaves as predicted in situations that are similar to actual ones. For example, quick engineering or model tuning can be used to optimise the model’s performance. Output quality is assessed using academic benchmarks and domain-specific data sets.
Cost and deployment should guide your choice
Once you have tested, hone your selection by taking into account aspects like ROI, economy of scale, and the feasibility of implementing the model in your current infrastructure and processes. Consider other advantages like reduced latency or increased transparency while making your decision.
Select the most value-adding model
Decide on an AI model that best suits the requirements of your use case by weighing performance, cost, and associated hazards.
The IBM Watsonx Model Library
The IBM Watsonx library provides proprietary, open source, and third-party models by utilising a multimodel technique
Customers can choose from a variety of options, depending on what best suits their particular business needs, location, and risk tolerance.
Watsonx also gives customers the flexibility to install models on-premises, in hybrid cloud environments, and on-premises, allowing them to lower total cost of ownership and avoid vendor lock-in.
Enterprise-grade IBM Granite base models
The three primary traits that characterise foundation models can be combined. Businesses need to realise that if one quality is prioritised above the others, the others may suffer. The model must be adjusted to meet the unique needs of each organisation by striking a balance between these characteristics:
Reliable: Explicit, comprehensible, and non-toxic models.
Performant: Suitable performance level for the use cases and business domains being targeted.
Models with a lower total cost of ownership and lower risk are considered cost-effective.
IBM Research produced the flagship IBM Granite line of enterprise-grade models. Businesses can succeed in their gen AI ambitions with these models because they have an ideal combination of these traits, with a focus on trust and reliability. Do not forget that companies cannot grow artificial intelligence (AI) with unreliable foundation models.
Following a thorough refinement process, IBM Watsonx provides enterprise-grade AI models. This process starts with model innovation under the direction of IBM Research, which involves transparent data sharing through open collaborations and training on enterprise-relevant information in accordance with the IBM AI Ethics Code.
An instruction-tuning technique developed by IBM Research contributes features that are critical for enterprise use to both IBM-built and some open-source models. IBM’s “FM_EVAL” data set simulates enterprise AI systems that are used in real-world settings, going beyond academic benchmarks. Watsonx.ai offers clients dependable, enterprise-grade gen AI foundation models by showcasing the most robust models from this pipeline.
Most recent model announcements
Granite code models are a series of models that encompass both instruction-following model variants and a basic model, trained in 116 programming languages and with sizes ranging from 3 to 34 billion parameters.
Granite-7b-lab is a general-purpose work support system that is adjusted to integrate new abilities and information through the IBM large-scale alignment of chatbots (LAB) methodology.
Read more on govindhtech.com
#aimodels#ibmgranitemodels#IBMwatsonx#hybridcloud#artificialintelligence#News#technews#technology#technologynews#technologytrends#govindhtech#ibm
0 notes
Text
Artificial General Intelligence: Race to Create a Human Mind
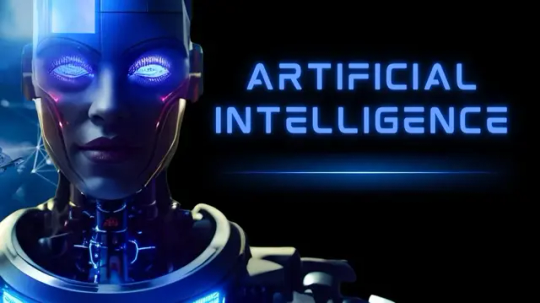
This is the potential of the hypothetical technology known as artificial general intelligence (AGI), which has the ability to completely transform almost every facet of human life and employment. Even if AGI is still theoretical, businesses may be proactive in getting ready for it by creating a strong data infrastructure and encouraging a collaborative atmosphere where AI and humans can coexist together.
Prepare for the future of artificial general intelligence with these examples AGI, also known as strong AI, is the science-fictional kind of artificial intelligence (AI) in which machine intelligence is able to learn, see, and think like a human. However, in contrast to humans, artificial general intelligence (AGI) is never tired, has no biological requirements, and is capable of processing information at unthinkable speeds. As machine intelligence continues to take on jobs that were previously regarded to be exclusively the domain of human intelligence and cognitive capacities, the possibility of creating artificial minds with the capacity to learn and solve complicated issues holds great potential for revolutionizing and upending numerous sectors.
Imagine an artificial general intelligence operating a self-driving automobile. Not only can it drive on unknown roads and pick up passengers from the airport, but it can also adjust the discussion in real time. It might respond to inquiries about the geography and culture of the area, tailoring its responses to the passenger’s preferences. On the basis of user preferences and current popularity, it might recommend a restaurant.
AI programmes such as LaMDA and GPT-3 are quite good at producing text of human caliber, completing particular jobs, translating across languages when necessary, and producing other forms of artistic material. It’s crucial to recognize that these LLM technologies are not the thinking robots that science fiction has promised, despite the fact that they may occasionally seem like it.
Sophisticated algorithms, computer science principles, and natural language processing (NLP) are combined to achieve these accomplishments. Because Chat GPT and other LLMs have been trained on vast volumes of textual data, they are able to identify statistical links and trends in language. They can better understand the subtleties of human language, such as grammar, syntax, and context, thanks to NLP approaches. These AI systems can then create text that appears human, translate languages with remarkable precision, and create creative content that imitates many styles by utilizing sophisticated AI algorithms and computer science techniques.
The artificial intelligence of today, which includes generative AI (gen AI), is sometimes referred to as narrow AI. It is excellent at sorting through enormous data sets to find patterns, automating processes, and producing writing of human caliber. These systems, however, are incapable of true understanding and cannot adjust to circumstances that are not familiar to them. This discrepancy demonstrates the enormous disparity between the capabilities of AGI and contemporary AI.
The transition from weak AI to full AGI is a huge problem, notwithstanding the exciting nature of the advancement. Researchers are currently investigating general problem-solving, commonsense reasoning, and artificial awareness in robots. Although the timeframe for creating a true artificial general intelligence (AGI) is still unknown, a company can ready its technology for future development by constructing a strong data-first infrastructure now.
Advancing AI To Achieve AGI Despite recent major advancements in AI, considerable obstacles still need to be cleared before real AGI machines with intelligence comparable to that of humans can be achieved.
Currently, AI struggles with the following seven crucial skills, which artificial general intelligence would need to master:
Visual perception: Computer vision is still far behind humans in terms of object detection and facial recognition, even though it has made great progress in these areas. AI systems now have difficulty recognizing colour, context, and how to respond to partially hidden objects. Audio perception: Despite advances in voice recognition, AI is still not able to correctly understand accents, sarcasm, or emotive speech tones. It also has trouble differentiating non-verbal signs like sighs, laughter, and volume changes from unimportant background noise. Fine motor skills: Artificial general intelligence software and robotics hardware could work together. In such case, the AGI would need to be able to swiftly adjust to new physical tasks, handle delicate things, and operate tools in real-world situations. Weak AI is good at tackling narrow, well-defined issues, but artificial general intelligence(AGI) would need to use reasoning and critical thinking to solve problems in a similar manner to humans. The AGI would have to deal with ambiguity and make judgements based on insufficient data. Navigating: Although self-driving cars have remarkable capabilities, human-like navigating necessitates quick environment adaption. People can move across congested cities, difficult terrain, and shifting circumstances with ease. Originality and freshness are essential components of actual creativity, even though AI is capable of producing new text structures to some extent. Human creativity is characterized by the generation of novel ideas, concepts, or solutions. Engagement on a social and emotional level: Human intelligence and social and emotional skills are closely related. Artificial general intelligence would need to be able to read facial expressions, tone of voice, and body language in order to recognize and understand emotions. AGI must modify its behaviour and communication to take into account the emotional states of others in order to react to emotions properly. Which AGI types exist? AGI would be a significant technological advancement that will permanently change the way businesses are conducted in sectors like manufacturing and healthcare. A lot of money is being invested in its creation by big tech companies and research institutes, and several schools of thought are attempting to solve the problem of creating computers with actual human-level intelligence.
The following are some main fields of investigation: Symbolic AI: This method focuses on creating systems that can represent knowledge and reasoning through the manipulation of symbols and logic. It seeks to develop a system that, like humans, is capable of understanding and resolving issues by applying rules.
Artificial Neural Networks: Artificial neural networks, or connectionist AI, take their cues from the structure and operations of the human brain. In order to learn and process information based on a large amount of input, artificial neural networks with interconnected nodes are constructed.
Artificial consciousness: Is the study of giving machines the ability to feel emotions and recognize themselves. Although it’s a very theoretical idea, it could be a crucial element of genuine intelligence.
Whole Brain Emulation: The goal of this ambitious strategy is to build an intricate computer model of the biological brain. The theory behind this simulation is that awareness and intelligence could emerge by imitating the composition and operations of the human brain.
Embodied AI And Embodied Cognition: This approach focuses on how an agent’s physical environment shapes its intellect. True intelligence, according to the theory, necessitates that an agent have a physical body through which to experience and absorb the universe.
The field of artificial general intelligence research is always changing. These are but a handful of the methods that have been investigated. It’s likely that AGI will eventually be realized through a combination of these methods or through completely new strategies.
Business operations with AI is the way of the future Even though AGI is still science fiction, businesses may prepare for it by developing an AI strategy for their industry on IBM Watsonx, a single collaborative AI and data platform. To help you scale and accelerate the effect of AI with reliable data throughout your organization, train, validate, adjust, and deploy AI models.
Read more on Govindhtech.com
0 notes
Text
IBM and TechD protect data and insights for gen AI
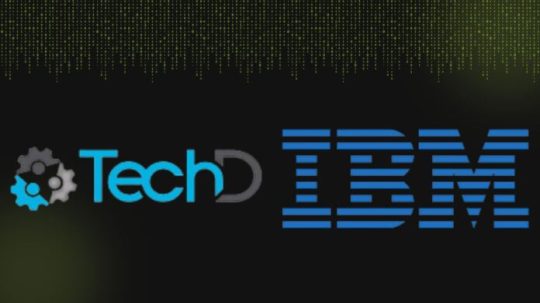
IBM and TechD unite to safely exchange data and use word AI to induce perceptivity. TechD is apprehensive that precise data source is critical to the quality of generative AI, or” word AI,” as technology advances. Information exchange across departments requires a reliable and secure data source.
By using generative AI, IBM may simply, fleetly, and efficiently come a resource for numerous others by participating TechD’S moxie. In the snappily changing digital world of moment, prompt responses are essential to furnishing exceptional stoner gests . TechD’s collaboration with IBM makes it easier to develop scalable results that businesses can snappily put into practice.
This effectively meets the demands for natural language processing, data operation, and conversational commerce. They’ve included IBM Watsonx Assistant, IBM Db2, and NeuralSeek in result.
TechD, A well- coordinated alliance How these instruments cooperate IBM give a complete result that simplifies data administration improves availability, and aids in icing security and integrity across your company . IBM Db2 A reliable, high- performing database with effective data storing, analysis, and reclamation developed for enterprise- position operations. boasting decades of experience in performance, security, scalability, and responsibility. The foundation is IBM Db2, which offers a strong database for handling and storing data. IBM Watsonx Assistant AI- powered system that comprehends and reacts to natural language inquiries, including database- related bones
In order to respond to client inquiries, Watsonx Assistant pulls data from Db2 databases and executes commands. also, Db2 fluently combines with Watsonx Assistant’s natural language processing features to assay and prize perceptivity from unshaped data.
NeuralSeek A slice- edge mileage that improves the natural language capabilities of being systems and makes large- scale database searching readily. Watsonx Assistant may more fluently find material information thanks to NeuralSeek’s enhancement of Db2’s hunt and reclamation features. also, NeuralSeek’s language appreciation chops enhance Watsonx Assistant’s perfection in responding by adding its capability to directly read stoner input. NeuralSeek puts the security and sequestration of its guestsfirst.
This is fulfilled by cracking data both during transmission and while it’s at rest, shielding it from unauthorised access and conserving its secretiveness. By taking stoner places and warrants into account, strong access control systems regulate data availability. This reduces the possibility of data breaches and unauthorised use by icing that only authorised individualities may see or alter the data. When used in tandem, these technologies enable businesses to produce strong, clever conversational interfaces that can comprehend, assay, and reply to stoner requests.
With the help of Techd intertwined result, you can fluently interact with consumers by combining quick access to data with sophisticated natural language processing capabilities. TechD result meets a variety of purposes, whether it’s answering customer questions or furnishing immediate backing. promoting stoner capability and relinquishment By easily integrating Techd’S result into current processes, IBM enable guests to enjoy the game- changing advantages of AI– driven perceptivity and effectiveness. guests may help secure the loftiest position of sequestration and security while completely realising the pledge of data by perfecting stoner relinquishment and capability.
It’s possible to conform training programmes so that they’re suitable for the specific places, liabilities, and skill situations of each individual member of yourteam.When you make an investment in the professional development of your workers by furnishing them with expansive original and continuing training, you give them the chops they need to apply their bents in a manner that’s both harmonious and confident about themselves. You’re also cordially invited to share in TechD yearly forums , during which IBM will bandy TechD’sproducts and offer guidance on their optimal utilisation. IBM eagerly anticipate your presence among us.
Product in use Using TechD product An American family- possessed retail company that’s well- known for its products using IBM Watsonx Assistant and NeuralSeek analytics to revolutionise online shopping. By bridging the gap between online and in- store gests , this integration bettered consumer commerce. Rapid deployment and the operation of the Db2 database made it easier to snappily acclimate to changing request requirements.
The accuracy and depth of your data source determines the quality of the replies generated by generative AI. Come celebrate the release of IBM Db2’s integration with Watsonx Assistant and NeuralSeek, a game-changing tool that will revolutionise the safe and natural language sharing of data across departments.
This system produced customised product descriptions with ease, making it easier to find material data and give specialised support. Retrieval enhanced generation technology reduced client annoyance, increased contact centre productivity, and offered priceless perceptivity into consumer geste.
In online shopping, having quick access to clear product descriptions helped businesses stay competitive. via the use of IBM Watsonx Assistant, IBM Db2, and NeuralSeek, the business was suitable to snappily and largely personalised respond to consumer demands, enabling informed decision- making via a thorough grasp of its online paperback demography. This each- encompassing strategy strengthened the company’s standing as an inventive assiduity leader devoted to excellence in addition to aiding in icing customer pleasure.
Read more on Govindhtech.com
0 notes
Text
Data Virtualization in Modern AI and Analytics Architectures
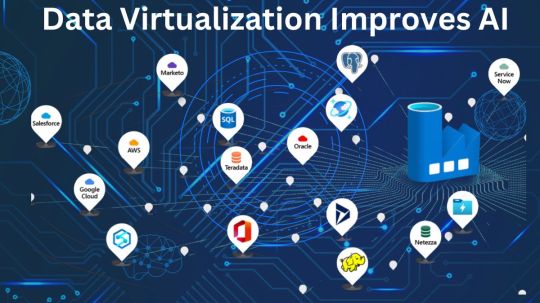
Data virtualization brings data together for seamless analytics and AI.
What is data virtualization? Before building any artificial intelligence (AI) application, data integration is a must. While there are several ways to begin this process, data virtualization helps organizations build and deploy applications more quickly.
With the help of data virtualization, organizations can unleash the full potential of their information, obtaining real-time artificial intelligence insights for innovative applications such as demand forecasting, fraud detection, and predictive maintenance.
Even with significant investments in technology and databases, many businesses find it difficult to derive more value from their data. This gap is filled by data virtualization, which enables businesses to leverage their current data sources for AI and analytics projects with efficiency and flexibility.
By acting as a bridge, virtualizing data allows the platform to access and display data from external source systems whenever needed. With this creative solution, data management is centralized and streamlined without necessitating actual storage on the platform. By creating a virtual layer between users and data sources, organizations can manage and access data without having to copy it or move it from its original place.
Why go with data virtualization? Data virtualization removes the need for physical duplication or migration, which speeds up the process of combining data from several sources. This minimizes the possibility of errors or data loss while also drastically cutting down on the time and cost of data integration.
Any organization, no matter where the data is stored, can obtain a centralized view of it.
Dismantling data silos: Using data virtualization to support machine learning breakthroughs AI and advanced analytical tools have transformed huge business operations and decision-making. Data virtualization acts as a central hub to integrate real-time data streams from equipment logs and sensor data and eliminate data silos and fragmentation.
Through data virtualization, historical data from extensive software suites utilized for a variety of tasks, including customer relationship management and business resource planning, is integrated with real-time data. Depending on the suite, this historical data offers insightful information about things like maintenance schedules, asset performance, or customer behavior.
Data virtualization integrates real-time and historical data from multiple sources to show an organization’s operational data environment. This holistic strategy helps firms improve procedures, make data-driven decisions, and gain a competitive edge.
This vast data set is being used by foundation models due to the rise of generative AI chatbots. By actively sorting through the data to find hidden trends, patterns, and correlations, these algorithms offer insightful information that helps advanced analytics forecast a variety of outcomes. These forecasts have the capacity to foresee future market trends and client demands, spot possible business opportunities, proactively identify and stop system problems and breakdowns, and optimize maintenance plans for optimal uptime and effectiveness.
Considering the design of virtualized data platforms
Real-time analysis and latency
Problem: Direct access to stored data usually results in lower latency than virtualized data retrieval. This might cause problems for real-time predictive maintenance analysis, as prompt insights are essential.
Design considerations: In order to provide real-time insights and reduce access times to virtualized data, IBM needs to use a two-pronged strategy. IBM will first examine the network architecture and enhance data transfer mechanisms. This may entail employing quicker protocols, like UDP, for specific data types or strategies like network segmentation to lessen congestion. They shorten the time it takes to get the information you require by streamlining data transport.
Secondly, in order to keep the dataset for analysis sufficiently current, they will put data refresh procedures into place. This could entail scheduling batch processes to update data incrementally on a regular basis while balancing the number of updates with the resources needed. Finding this balance is essential because too many updates can overload systems, while too few updates can result in out-of-date information and erroneous forecasts. These tactics when combined can result in minimal latency as well as a new data collection for the best possible analysis.
Maintaining a balance between update frequency and strain on source systems:
Virtualized data can be continuously queried for real-time insights, but this might overload source systems and negatively affect their performance. Given that AI and predictive analysis rely on regular data updates, this presents a serious risk.
Design considerations: You must carefully plan how it gets data in order to maximize query frequency for your predictive analysis and reporting. This entails concentrating on obtaining only the most important data points and maybe making use of data replication technologies to enable real-time access from several sources. Additionally, to improve overall model performance and lessen the burden on data systems, think about scheduling or batching data retrievals for particular critical periods rather than querying continuously.
Benefits to developers and abstraction of the virtualization layer Benefit: The data platform’s virtualization layer serves as an abstraction layer. This means that once the abstraction layer is complete, developers may focus on creating AI/ML or data mining applications for businesses rather than worrying about the precise location of the data’s physical storage. They won’t be sidetracked by the difficulties of data administration and can concentrate on developing the fundamental logic of their models. This results in quicker application deployment cycles and development cycles.
Benefits for developers: When working on data analytics, developers may concentrate on the fundamental reasoning behind their models by using an abstraction layer. This layer serves as a barrier, keeping the complexity of managing data storage hidden. As a result, developers won’t have to spend as much time grappling with the complexities of the data, which will eventually speed up the implementation of the predictive maintenance models.
Tips for optimizing storage While some data analysis applications may not directly benefit from storage optimisation techniques like normalisation or denormalization, they are nonetheless important when using a hybrid approach. This methodology entails the amalgamation of data that is ingested and data that is accessed via virtualization on the selected platform.
Evaluating the trade-offs between these methods contributes to the best possible storage utilisation for virtualized and ingested data sets. Building efficient ML solutions with virtualized data on the data platform requires careful consideration of these design factors.
Data virtualization Platform Data virtualization is now more than just a novel concept. It is used as a tactical instrument to improve the performance of different applications. A platform for data virtualization is a good example. Through the use of data virtualization, this platform makes it easier to construct a wide range of applications, greatly enhancing their effectiveness, flexibility, and ability to provide insights in almost real-time.
Let’s examine a few fascinating use examples that highlight data virtualization’s revolutionary potential.
Supply chain optimization in the context of globalization Supply chains are enormous networks with intricate dependencies in today’s interconnected global economy. Data virtualization is essential for streamlining these complex systems. Data from several sources, such as production metrics, logistics tracking information, and market trend data, are combined by a data virtualization platform. With a thorough overview of their whole supply chain operations, this comprehensive view enables enterprises.
Imagine being able to see everything clearly from every angle. You can anticipate possible bottlenecks, streamline logistics procedures, and instantly adjust to changing market conditions. An agile and optimised value chain that offers notable competitive advantages is the end outcome.
A thorough examination of consumer behavior: customer analytics Because of the digital revolution, knowing your clients is now essential to the success of your business. Data virtualization is a technique used by a data virtualization platform to eliminate data silos. Customer data from several touchpoints, including sales records, customer service exchanges, and marketing campaign performance measurements, is effortlessly integrated. This cohesive data ecosystem facilitates a thorough comprehension of consumer behavior patterns and preferences.
Equipped with these deep consumer insights, companies can design highly customized experiences, focus marketing, and develop cutting-edge items that more successfully appeal to their target market. This data-driven strategy fosters long-term loyalty and client happiness, both of which are essential for succeeding in the cutthroat business world of today.
In the era of digitalization, proactive fraud detection Financial fraud is a dynamic phenomenon that requires proactive detection using data virtualization platforms. By virtualizing and evaluating data from several sources, including transaction logs, user behavior patterns, and demographic information, the platform detects possible fraud attempts in real time. This strategy not only shields companies from monetary losses but also builds consumer trust, which is an invaluable resource in the current digital era.
These significant examples demonstrate the transformational potential of data virtualization.
Businesses can unleash the full potential of their data with the help of IBM Watsonx and IBM Cloud for Data platform, which spurs innovation and gives them a major competitive edge in a variety of industries. In addition, IBM provides IBM Knowledge Catalogue for data governance and IBM Data Virtualization as a shared query engine.
Read more on Govindhtech.com
0 notes
Text
IBM Watsonx Assistant improves responsible AI for your firm
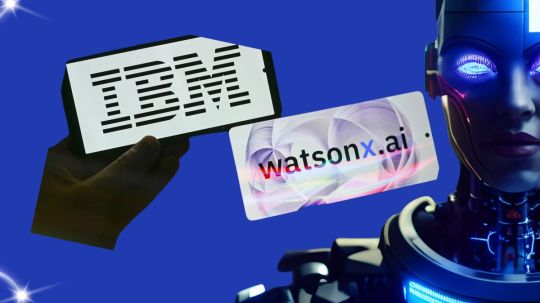
This explains why sports, where even a small competitive edge can mean the difference between first and second place, have seen such a high number of early adopters of AI.
Consider the US Open from last year, where IBM Watsonx predicted each player’s degree of advantage or disadvantage in the singles draw. Sevilla FC introduced a watsonx-based technology abroad to give scouts thorough data-driven identification and assessment of possible signings. Additionally, EDGE3 will integrate with IBM Watsonx to enhance the college programme and player decision-making process, assisting colleges and athletes in navigating the ever-complex world of recruiting.
The quick adoption of these examples in enterprises goes hand in hand. For example, in order to help important industries, they are collaborating with Adobe to integrate Watsonx into their platform. IBM team is collaborating with SAP to augment SAP products with more artificial intelligence, machine learning, and other intelligent technologies that can increase business outcomes for our mutual clients.AI efforts are being launched by companies of all sizes and sectors.
Giving Responsible AI Priority:
Governance: Watsonx.governance provides resources for risk management, transparency, and staying ahead of AI-related policies in the future. This contains features that guarantee exploitability and track machine learning models.
Data management: Watsonx.data supports ethical and safe data management. It guarantees that data is used in accordance with regulatory rules and offers a dedicated data repository that grows with your needs.
Openness: Because the platform is based on open-source technologies, AI models can be customized and expanded upon. This transparency encourages teamwork and helps prevent the prejudices that come with closed systems.
Features and Specifications:
Multi-model Variety: For particular jobs, you can integrate your own bespoke models or select from pre-built IBM models or open-source models.
AutoAI: This tool streamlines workflows for professionals and makes AI development more approachable for novices by automating tasks like model construction, data preparation, and hyperparameter tweaking.
AI Assistants: Tasks can be automated and AI implemented in a variety of business areas, such as customer service or HR, using pre-built applications powered by Watsonx. There is no need to create code in order to deploy these aids.
Flexibility: Watsonx can be deployed on a range of infrastructures, including on-premises servers, private clouds, and public clouds, to meet a variety of business requirements.
Watsonx hopes to enable companies to properly develop and implement AI by providing these characteristics, emphasising compliance, fairness, and transparency.
It’s crucial to remember that responsible AI implementation calls for more than simply a platform. To guarantee the ethical development and application of AI, businesses must have defined policies and procedures in place.
They ought to be posing the following two queries:
Is AI a good fit for my company?
Indeed. Every organization, whether large or little, is likely in a competitive industry where artificial intelligence can make a major difference. Before using AI, decide on the use case and goals.
In order to enhance their current technology, partners from a variety of industries are already incorporating IBM Watsonx into their processes, products, and solutions in novel ways. For instance, clients can leverage IBM AI with proprietary data stored in Box to speed up business operations by combining Watsonx with Box’s Content Cloud.
Quantum Street AI
Quantum Street AI is a financial investment and wealth management company that provides a comprehensive AI platform with Watsonx integration for professional investors. The platform covers 50,000 publicly traded worldwide firms and asset classes and represents USD 6 billion in client assets.
IBM’s strategy, which employs smaller models tailored to particular business use cases, also assists in lowering the barrier to entry for AI by assisting businesses in adopting particular models that operate on less expensive infrastructure and provide greater flexibility in terms of deployment on-premises, in private cloud, or on the public cloud.
How can I guarantee ethical AI innovation for my business?
Corporate executives of today should be considering how to use AI ethically rather than whether or not to investigate it for their company. There is no margin for mistake when implementing AI in the workplace; the proper safeguards must be put in place.
Governance is an essential component of responsible AI, and it need to be the primary focus of every developer, software supplier, or company planning to use AI. For this reason, Watsonx. Governance’s release was such a big deal for IBM. They give businesses a toolkit to help them embrace transparency, manage risk, and prepare for future regulations centered on artificial intelligence.
Watsonx.data
IBM Watsonx.data
Watsonx.data is a purpose-built data store that partners may use to use their data. It allows businesses to expand AI workloads for all of their data, regardless of where it is stored. IBM’s long-standing stance that such data belongs to the partner is a significant differentiator for IBM. As an alternative, the Granite model series (with publicly available data sources) is available on watsonx.ai, which is used by numerous partners.
IBM partners take the usage of data seriously, just like they do. Consider their partnership with Dun & Bradstreet, which combines Watsonx with the Dun & Bradstreet Data Cloud to assist businesses in expanding their usage of generative AI in an ethical manner.
IBM Watsonx Assistant is being used by Boxes, another IBM Partner, to assist retailers in obtaining buyer-ready data and customer insights so they may introduce new products and provide samples to customers. Additionally, Watsonx Foundation models are being used by partners like to address some of the other difficult aspects of AI, such hallucinations.
IBM Watsonx Assistant is being used by Boxes, another IBM Partner, to assist retailers in obtaining buyer-ready data and customer insights so they may introduce new products and provide samples to customers. Additionally, Watsonx Foundation models are being used by partners like to address some of the other difficult aspects of AI, such hallucinations.
IBM Watsonx Assistant is being used by Boxes, another IBM Partner, to assist retailers in obtaining buyer-ready data and customer insights so they may introduce new products and provide samples to customers. Additionally, Watsonx Foundation models are being used by partners like to address some of the other difficult aspects of AI, such hallucinations.
IBM Watsonx Assistant is being used by Boxes, another IBM Partner, to assist retailers in obtaining buyer-ready data and customer insights so they may introduce new products and provide samples to customers. Additionally, Watsonx Foundation models are being used by partners like to address some of the other difficult aspects of AI, such hallucinations.
IBM Watsonx Assistant is being used by Boxes, another IBM Partner, to assist retailers in obtaining buyer-ready data and customer insights so they may introduce new products and provide samples to customers. Additionally, Watsonx Foundation models are being used by partners like to address some of the other difficult aspects of AI, such hallucinations.
It’s wonderful to say that IBM uses its many years of experience leading the industry, making investments, and doing AI research to support partners at every stage of their AI journey. Regardless of your company’s age, size, form, or sector, IBM offers the appropriate partner programmed and technologies to support the adoption and growth of responsible AI in its enterprise.
Read more on Govindhtech.com
#govindhtech#watsonx#IBMWatsonX#WatsonXAssistant#ResponsibleAI#IBM#ibmwatsonxassistant#News#technews#technologynews#technologytrends#technology
0 notes
Text
How IBM Watsonx Code Assistant Boosts Productivity
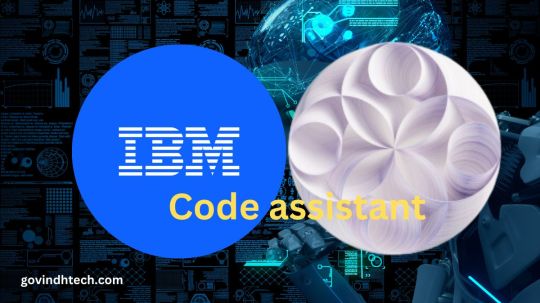
Accelerating Product Innovation with Gen AI Solutions
When used properly, generative artificial intelligence (GenAI solutions) may be a potent tool for stimulating product creation. IBM will talk about a few high-profile product use examples that show how artificial intelligence (AI) has the power to completely transform the way they create, sell, and deliver goods to consumers. Elevating your product organisation to a new level requires a solid foundation of data management, predictive analytics, and GenAI.
IBM Watsonx Code Assistant
Quickening the modernization of products
Manual product modernization operations may be automated with the help of GenAI. GenAI systems are able to gather competitor data by sifting through publicly accessible sources, such press releases, and comparing the present product mix with that of competitors. Additionally, it may identify competitive advantages and recommend smart modifications to current products. Realization of these novel discoveries is possible more quickly than in the past.
GenAI’s capacity to produce code is one of its main advantages. With the help of GenAI technologies, business users may now create prototype code for new product features without heavily depending on technical teams. To cut down on testing efforts, these same technologies may analyse code, find faults in it, and address them.
A cloud-based solution called IBM Watsonx Code Assistant uses generative AI to expedite code creation and increase developer productivity. It is especially designed for IT automation and corporate application modernization. Below is a summary of its salient attributes:
Generative AI for Writing Code
Generates function calls or code fragments from plain language instructions.
Pre-trained models guarantee reliable and accurate results for a certain programming language.
Pay Attention to Business Needs
Intended to speed up the modernization of programmes, particularly the conversion of old COBOL mainframe systems to Java.
Integrates with business environments’ current processes and tools.
Personalisation and Authority
Enables companies to adjust the underlying AI models so that they comply with best practices and coding standards.
Makes the created code’s source transparent for security and maintainability reasons.
IBM Watsonx Code Assistant Advantages:
Increased productivity for developers via the automation of tedious coding processes.
Quicker cycles for developing applications, particularly while modernization is being done.
Decreased possibility of mistakes in handwritten code thanks to AI-generated recommendations.
All things considered, IBM Watsonx Code Assistant is a potent tool for corporate development teams hoping to use AI to boost coding productivity and accelerate the production of applications, especially when working with legacy systems.
IBM Watsonx Code Assistant and other GenAI products satisfy the essential technological requirements of businesses. Businesses may earn 30% more productivity or reduce development effort by 30% using Watsonx Code Assistant. These instruments might bring about a revolution in technical procedures and accelerate the supply of technological products.
Using an AI-powered chatbot to respond to consumer enquiries
Being the first virtual chatbot driven by artificial intelligence and available to the public, ChatGPT stands apart. Businesses may now take up the fundamental ideas of this technology and incorporate them into their operations, enhancing them even further with contextualization and security. Businesses can create massive language models and train them with private data with IBM Watsonx Assistant, all while contributing to data security.
Numerous product applications for conversational AI systems may increase sales and enhance customer satisfaction. An intelligent chatbot, for example, may handle typical consumer inquiries about bill explanations. A chatbot driven by GenAI solutions can provide clients comprehensive explanations for their bills, including use and overage fee transaction records.
In addition, it may offer new product bundles or contract conditions that match a client’s historical consumption requirements, creating new income streams and raising client satisfaction. Companies using IBM Watsonx Assistant could anticipate a 20% rise in customer satisfaction and a 30% decrease in customer support expenses.
Examining consumer behaviour to provide customised product suggestions
Businesses may discover when certain consumers are most suitable for new items, get recommendations for suitable products, and get recommendations for the best ways to continue communicating with the customer by using the power of GenAI and predictive analytics. For example, predictive models based on past transactions might assess the possible demand for new goods if a client experiences a significant company shift, like an acquisition.
The salesperson may then study the email that GenAI sent to the client suggesting potential upsells. This enables sales teams to provide consumers with superior service while increasing speed to value. Enterprise data may be prepared for a variety of analytical and AI use cases using IBM Watsonx.data.
Examining client input to guide corporate strategy
By acting on consumer input more quickly, businesses may utilise GenAI to enhance the customer experience. For several forms of summarization, a range of industry-leading models are accessible via IBM Watsonx.ai. Large amounts of client input may be swiftly interpreted and summarised using this technology.
Then, with more detailed requirements and user stories, it may provide recommended product enhancements, quickening the pace of innovation and response. GenAI can identify patterns in lost client feedback, propose fresh approaches to closing deals, and provide sales teams with pre-planned follow-ups and business information.
Using client segmentation to inform strategic marketing
GenAI solutions has the power to completely transform digital marketing by making marketing procedures faster, more efficient, and more customised. Businesses may find patterns and clusters in data using common data analytics techniques, allowing for more precise consumer targeting.
GenAI can drive automated content development processes that target certain client segments across several platforms once the clusters are established. With IBM Watsonx Orchestrate, users may boost productivity by automating routine processes. Marketing teams may save time and money by using this technology, which can develop content, connect to many channels, and instantly disseminate changes across them.
The primary distinction of generative AI is its capacity for content generation and consumer engagement, which is one of the things that excites us about these emerging technologies. GenAI has the ability to convert costly, labor-intensive manual marketing procedures into quicker, automated ones.
Read more on govindhtech.com
#ibm#ai#ibmwatsonx#chatgpt#genai#generativeai#chatbot#ibmwatsonxcode#technology#technews#news#govindhtech
0 notes