#TensorRT
Explore tagged Tumblr posts
Text
Rekor Uses NVIDIA AI Technology For Traffic Management
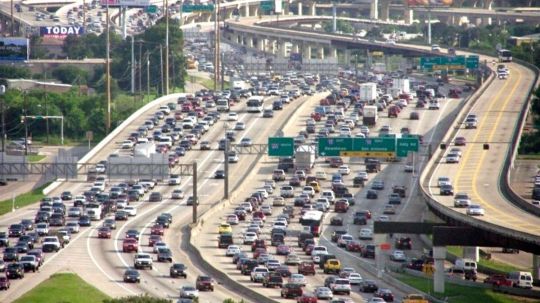
Rekor Uses NVIDIA Technology for Traffic Relief and Roadway Safety as Texas Takes in More Residents.
For Texas and Philadelphia highways, the company is using AI-driven analytics utilizing NVIDIA AI, Metropolis, and Jetson, which might lower fatalities and enhance quality of life.
Jobs, comedy clubs, music venues, barbecues, and more are all attracting visitors to Austin. Traffic congestion, however, are a major city blues that have come with this growth.
Due to the surge of new inhabitants moving to Austin, Rekor, which provides traffic management and public safety analytics, gets a direct view of the growing traffic. To assist alleviate the highway issues, Rekor collaborates with the Texas Department of Transportation, which is working on a $7 billion initiative to remedy this.
Based in Columbia, Maryland, Rekor has been using NVIDIA Jetson Xavier NX modules for edge AI and NVIDIA Metropolis for real-time video understanding in Texas, Florida, Philadelphia, Georgia, Nevada, Oklahoma, and many other U.S. locations, as well as Israel and other countries.
Metropolis is a vision AI application framework for creating smart infrastructure. Its development tools include the NVIDIA DeepStream SDK, TAO Toolkit, TensorRT, and NGC catalog pretrained models. The tiny, powerful, and energy-efficient NVIDIA Jetson accelerated computing platform is ideal for embedded and robotics applications.
Rekor’s initiatives in Texas and Philadelphia to use AI to improve road management are the most recent chapter in a long saga of traffic management and safety.
Reducing Rubbernecking, Pileups, Fatalities and Jams
Rekor Command and Rekor Discover are the two primary products that Rekor sells. Traffic control centers can quickly identify traffic incidents and areas of concern using Command, an AI-driven software. It provides real-time situational awareness and notifications to transportation authorities, enabling them to maintain safer and less congested municipal roads.
Utilizing Rekor’s edge technology, discover completely automates the collection of thorough vehicle and traffic data and offers strong traffic analytics that transform road data into quantifiable, trustworthy traffic information. Departments of transportation may better plan and carry out their next city-building projects by using Rekor Discover, which gives them a comprehensive picture of how cars travel on roads and the effect they have.
Command has been spread around Austin by the corporation to assist in problem detection, incident analysis, and real-time response to traffic activities.
Rekor Command receives a variety of data sources, including weather, linked vehicle information, traffic camera video, construction updates, and data from third parties. After that, it makes links and reveals abnormalities, such as a roadside incident, using AI. Traffic management centers receive the data in processes for evaluation, verification, and reaction.
As part of the NVIDIA AI Enterprise software platform, Rekor is embracing NVIDIA’s full-stack accelerated computing for roadway intelligence and investing heavily in NVIDIA AI and NVIDIA AI Blueprints, reference workflows for generative AI use cases constructed with NVIDIA NIM microservices. NVIDIA NIM is a collection of user-friendly inference microservices designed to speed up foundation model installations on any cloud or data center while maintaining data security.
Rekor is developing AI agents for municipal services, namely in areas like traffic control, public safety, and infrastructure optimization, leveraging the NVIDIA AI Blueprint for video search and summarization. In order to enable a variety of interactive visual AI agents that can extract complicated behaviors from vast amounts of live or recorded video, NVIDIA has revealed a new AI blueprint for video search and summarization.
Philadelphia Monitors Roads, EV Charger Needs, Pollution
The Philadelphia Industrial Development Corporation (PIDC), which oversees the Philadelphia Navy Yard, a famous tourist destination, has difficulties managing the roads and compiling information on new constructions. According to a $6 billion rehabilitation proposal, the Navy Yard property will bring thousands of inhabitants and 12,000 jobs with over 150 firms and 15,000 workers on 1,200 acres.
PIDC sought to raise awareness of how road closures and construction projects influence mobility and how to improve mobility during major events and projects. PIDC also sought to improve the Navy Yard’s capacity to measure the effects of speed-mitigating devices placed across dangerous sections of road and comprehend the number and flow of car carriers or other heavy vehicles.
In order to handle any fluctuations in traffic, Discover offered PIDC information about further infrastructure initiatives that must be implemented.
By knowing how many electric cars are coming into and going out of the Navy Yard, PIDC can make informed decisions about future locations for the installation of EV charging stations. Navy Yard can better plan possible locations for EV charge station deployment in the future by using Rekor Discover, which gathers data from Rekor’s edge systems which are constructed with NVIDIA Jetson Xavier NX modules for powerful edge processing and AI to understand the number of EVs and where they’re entering and departing.
By examining data supplied by the AI platform, Rekor Discover allowed PIDC planners to produce a hotspot map of EV traffic. The solution uses Jetson and NVIDIA’s DeepStream data pipeline for real-time traffic analysis. To further improve LLM capabilities, it makes advantage of NVIDIA Triton Inference Server.
The PIDC sought to reduce property damage and address public safety concerns about crashes and speeding. When average speeds are higher than what is recommended on certain road segments, traffic calming measures are being implemented using speed insights.
NVIDIA Jetson Xavier NX to Monitor Pollution in Real Time
Rekor’s vehicle identification models, which were powered by NVIDIA Jetson Xavier NX modules, were able to follow pollution to its origins, moving it one step closer to mitigation than the conventional method of using satellite data to attempt to comprehend its placements.
In the future, Rekor is investigating the potential applications of NVIDIA Omniverse for the creation of digital twins to model traffic reduction using various techniques. Omniverse is a platform for creating OpenUSD applications for generative physical AI and industrial digitization.
Creating digital twins for towns using Omniverse has significant ramifications for lowering traffic, pollution, and traffic fatalities all of which Rekor views as being very advantageous for its clients.
Read more on Govindhtech.com
#Rekor#NVIDIATechnology#TensorRT#AIapplication#NVIDIANIM#NVIDIANIMmicroservices#generativeAI#NVIDIAAIBlueprint#NVIDIAOmniverse#News#Technews#Technology#technologynews#Technologytrends#govindhtech
0 notes
Text
sentences that should be illegal to say to a girl:
This TensorFlow binary is optimized to use available CPU instructions in performance-critical operations
TF-TRT Warning: Could not find TensorRT
Cannot dlopen some GPU libraries
49 notes
·
View notes
Text
ok i want to learn - Loss Functions in LLMs (Cross-entropy loss, KL Divergence for distillation) Gradient Accumulation and Mixed Precision Training Masked Language Modeling (MLM) vs. Causal Language Modeling (CLM) Learning Rate Schedules (Warmup, cosine decay) Regularization Techniques (Dropout, weight decay) Batch Normalization vs. Layer Normalization Low-Rank Adaptation (LoRA) Prompt Engineering (Zero-shot, few-shot learning, chain-of-thought) Adapters and Prefix Tuning Parameter-Efficient Fine-Tuning (PEFT) Attention Head Interpretability Sparse Attention Mechanisms (BigBird, Longformer) Reinforcement Learning with Human Feedback (RLHF) Knowledge Distillation in LLMs Model Compression Techniques (Quantization, pruning) Model Distillation for Production Inference Optimization (ONNX, TensorRT)
4 notes
·
View notes
Text
MediaTek and NVIDIA Team up for Automotive AI
With more and more auto manufacturers pushing for smarter vehicles, there's been a considerably growing demand for more powerful smart automotive platforms, going beyond the simple act of pairing your smartphone with your car's Bluetooth console (think 'K.I.T.T.' from Knight Rider). It's no surprise then that we've seen an uptick of specially-designed hardware and software solutions that provide entertainment and navigation features for drivers and passengers alike. With that being said, MediaTek's push towards putting more AI tech into everyday consumer products has certainly yielded some very interesting results, and the company's newly-announced collaboration with PC gaming giant NVIDIA aims to do the same, at least in terms of automotive applications. More specifically, the mobile chip manufacturer formally announced that it has entered into a partnership with NVIDIA to develop new AI-powered software for vehicles, with the goal of creating a "smart cabin" for drivers and passengers. This collaboration will enable MediaTek to develop automotive SoCs, which will in turn integrate a new NVIDIA GPU "chiplet" with support for NVIDIA AI and graphics IP. Interestingly, these chiplets will be connected by specially-developed interconnect technology, at least according to MediaTek. Rick Tsai, Vice Chairman and CEO of MediaTek states: “NVIDIA is a world-renowned pioneer and industry leader in AI and computing. With this partnership,our collaborative vision is to provide a global one-stop shop for the automotive industry, designing thenext generation of intelligent, always-connected vehicles. Through this special collaboration with NVIDIA, we will together be able to offer a truly unique platform for the compute intensive, software-defined vehicle of the future.” NVIDIA CEO Jensen Huang says this combination of MediaTek and NVIDIA hardware will "enable new user experiences, enhanced safety and new connected services for all vehicle segments, from luxury to mainstream.” MediaTek adds that its smart cabin solutions will run NVIDIA DRIVE OS, DRIVE IX, CUDA and TensorRT software technologies. This then allows consumers to experience a full range of AI cabin and cockpit functionality with integrated AI, safety, and security features as well. While NVIDIA is more known to consumers as a PC and gaming-centric brand, the company does put a considerable amount of investment towards the development and production of AI and IoT (internet of things) technology, in addition to its powerful GPUs and processors. The Taiwanese company further states that by allowing MediaTek to tap into NVIDIA’s core expertise in AI, cloud, graphics technology, software and pairing with NVIDIA ADAS solutions, we can expect to see further improvement to the capabilities of the Dimensity Auto platform, MediaTek's flagship automotive software product. Dimensity Auto is designed for vehicles with support for compatible smart features. With all that being said, it should be interesting to see how both companies approach this new partnership, both on hardware and business fronts. Read the full article
2 notes
·
View notes
Link
0 notes
Photo
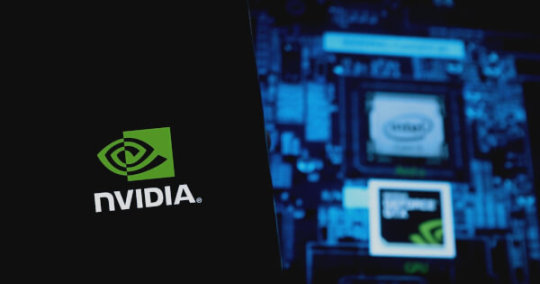
NVIDIA Enhances TensorRT-LLM with KV Cache Optimization Features
0 notes
Text
NVIDIA AI Software Party at a Hardware Show
New Post has been published on https://thedigitalinsider.com/nvidia-ai-software-party-at-a-hardware-show/
NVIDIA AI Software Party at a Hardware Show
A tremendous number of AI software releases at CES.
Created Using Midjourney
Next Week in The Sequence:
We start a new series about RAG! For the high performance hackers, our engineering series will dive into Llama.cpp. In research we will dive into Deliberative Alignment, one of the techniques powering GPT-03. The opinion edition will debate open endedness AI methods for long term reasoning and how far those can go.
You can subscribe to The Sequence below:
TheSequence is a reader-supported publication. To receive new posts and support my work, consider becoming a free or paid subscriber.
📝 Editorial: NVIDIA AI Software Party at a Hardware Show
The name NVIDIA is immediately associated with computing hardware and, in the world of AI, GPUs. But that is changing so rapidly. In several editions of this newsletter, we have highlighted NVIDIA’s rapidly growing AI software stack and aspirations. This was incredibly obvious last week at CES which is, well, mostly a hardware show!
NVIDIA unveiled not only a very clear vision for the future of AI but an overwhelming series of new products, many of which were AI software-related. Take a look for yourself.
NVIDIA NIM Microservices
NVIDIA’s NIM (NVIDIA Inference Microservices) is a significant leap forward in the integration of AI into modern software systems. Built for the new GeForce RTX 50 Series GPUs, NIM offers pre-built containers powered by NVIDIA’s inference software, including Triton Inference Server and TensorRT-LLM. These microservices enable developers to incorporate advanced AI capabilities into their applications with unprecedented ease, reducing deployment times from weeks to just minutes. With NIM, NVIDIA is effectively turning the once-daunting process of deploying AI into a seamless, efficient task—an essential advancement for industries looking to accelerate their AI adoption.
AI Blueprints
For developers seeking a head start, NVIDIA introduced AI Blueprints, open-source templates designed to streamline the creation of AI-powered solutions. These blueprints provide customizable foundations for applications like digital human generation, podcast creation, and video production. By offering pre-designed architectures, NVIDIA empowers developers to focus on innovation and customization rather than reinventing the wheel. The result? Faster iteration cycles and a smoother path from concept to deployment in AI-driven industries.
Cosmos Platform
NVIDIA’s Cosmos Platform takes AI into the realm of robotics, autonomous vehicles, and vision AI applications. By integrating advanced models with powerful video data processing pipelines, Cosmos enables AI systems to reason, plan, and act in dynamic physical environments. This platform isn’t just about data processing; it’s about equipping AI with the tools to operate intelligently in real-world scenarios. Whether it’s guiding a robot through a warehouse or enabling an autonomous vehicle to navigate complex traffic, Cosmos represents a new frontier in applied AI.
Isaac GR00T Blueprint
Robotic training just got a major upgrade with NVIDIA’s Isaac GR00T Blueprint. This innovative tool generates massive volumes of synthetic motion data using imitation learning, leveraging the capabilities of NVIDIA’s Omniverse platform. By producing millions of lifelike motions, Isaac GR00T accelerates the training process for humanoid robots, enabling them to learn complex tasks more effectively. It’s a groundbreaking approach to solving one of robotics’ biggest challenges—efficiently generating diverse, high-quality training data at scale.
DRIVE Hyperion AV Platform
NVIDIA’s DRIVE Hyperion AV Platform saw a significant evolution with the addition of the NVIDIA AGX Thor SoC. Designed to support generative AI models, this new iteration enhances functional safety and boosts the performance of autonomous driving systems. By combining cutting-edge hardware with advanced AI capabilities, Hyperion delivers a robust platform for developing the next generation of autonomous vehicles, capable of handling increasingly complex environments with confidence and precision.
AI Enterprise Software Platform
NVIDIA’s commitment to enterprise AI is reflected in its AI Enterprise Software Platform, now available on AWS Marketplace. With NIM integration, this platform equips businesses with the tools needed to deploy generative AI models and large language models (LLMs) for applications like chatbots, document summarization, and other NLP tasks. This offering streamlines the adoption of advanced AI technologies, providing organizations with a comprehensive, reliable foundation for scaling their AI initiatives.
RTX AI PC Features
At the consumer level, NVIDIA announced RTX AI PC Features, which bring AI foundation models to desktops powered by GeForce RTX 50 Series GPUs. These features are designed to support the next generation of digital content creation, delivering up to twice the inference performance of prior GPU models. By enabling FP4 computing and boosting AI workflows, RTX AI PCs are poised to redefine productivity for developers and creators, offering unparalleled performance for AI-driven tasks.
That is insane for the first week of the year! NVIDIA is really serious about its AI software aspirations. Maybe Microsoft, Google and Amazon need to get more aggressive about their GPU initiatives. Just in case…
🔎 AI Research
rStar-Math
In the paper “rStar-Math: Guiding LLM Reasoning through Self-Evolution with Process Preference Reward,” researchers from Tsinghua University, the Chinese Academy of Sciences, and Alibaba Group propose rStar-Math, a novel method for enhancing LLM reasoning abilities by employing self-evolution with a process preference reward (PPM). rStar-Math iteratively improves the reasoning capabilities of LLMs by generating high-quality step-by-step verified reasoning trajectories using a Monte Carlo Tree Search (MCTS) process.
BoxingGym
In the paper “BoxingGym: Benchmarking Progress in Automated Experimental Design and Model Discovery,” researchers from Stanford University introduce a new benchmark for evaluating the ability of large language models (LLMs) to perform scientific reasoning. The benchmark, called BoxingGym, consists of 10 environments drawn from various scientific domains, and the researchers found that current LLMs struggle with both experimental design and model discovery.
Cosmos World
In the paper “Cosmos World Foundation Model Platform for Physical AI,” researchers from NVIDIA introduce Cosmos World Foundation Models (WFMs). Cosmos WFMs are pre-trained models that can generate high-quality 3D-consistent videos with accurate physics, and can be fine-tuned for a wide range of Physical AI applications.
DOLPHIN
In the paper “DOLPHIN: Closed-loop Open-ended Auto-research through Thinking, Practice, and Feedback,” researchers from Fudan University and the Shanghai Artificial Intelligence Laboratory propose DOLPHIN, a closed-loop, open-ended automatic research framework2. DOLPHIN can generate research ideas, perform experiments, and use the experimental results to generate new research idea.
Meta Chain-of-Thoguht
In the paper“Towards System 2 Reasoning in LLMs: Learning How to Think With Meta Chain-of-Thought” researchers from SynthLabs.ai and Stanford University propose a novel framework called Meta Chain-of-Thought (Meta-CoT), which enhances traditional Chain-of-Thought by explicitly modeling the reasoning process. The researchers present empirical evidence of state-of-the-art models showing in-context search behavior, and discuss methods for training models to produce Meta-CoTs, paving the way for more powerful and human-like reasoning in AI.
LLM Test-Time Compute and Meta-RL
In a thoughtful blog post title“Optimizing LLM Test-Time Compute Involves Solving a Meta-RL Problem” from CMU explain that optimizing test-time compute in LLMs can be viewed as a meta-reinforcement learning (meta-RL) problem where the model learns to learn how to solve queries. The authors outline a meta-RL framework for training LLMs to optimize test-time compute, leveraging intermediate rewards to encourage information gain and improve final answer accuracy.
🤖 AI Tech Releases
NVIDIA Nemotron Models
NVIDIA released Llama Nemotron LLM and Cosmos Nemotron vision-language models.
Phi-4
Microsoft open sourced its Phi-4 small model.
ReRank 3.5
Cohere released its ReRank 3.5 model optimized for RAG and search scenarios.
Agentic Document Workfows
LlamaIndex released Agentic Document Workflow, an architecture for applying agentic tasks to documents.
🛠 AI Reference Implementations
Beyond RAG
Salesfoce discusses an enriched index technique that improved its RAG solutions.
📡AI Radar
NVIDIA released AI agentic blueprints for popular open source frameworks.
NVIDIA unveiled Project DIGITS, an AI supercomputer powered by the Blackwell chip.
NVIDIA announced a new family of world foundation models for its Cosmos platform.
Anthropic might be raising at a monster $60 billion valuation.
Hippocratic AI raised a massive $141 million round for its healthcare LLM.
Cohere announced North, its Microsoft CoPilot competitor.
OpenAI might be getting back to robotics.
Gumloop raised $17 million for its workflow automation platform.
#3d#adoption#ai#AI adoption#AI models#ai supercomputer#AI systems#AI-powered#Alibaba#Amazon#anthropic#applications#applied AI#approach#architecture#Art#artificial#Artificial Intelligence#automation#automation platform#autonomous#autonomous driving#autonomous vehicle#autonomous vehicles#AWS#Behavior#benchmark#benchmarking#billion#blackwell
0 notes
Quote
2024年12月27日 12時05分 AI検索エンジン「ChatGPT search」とGoogle検索を62件のクエリで比較してみた結果 OpenAIがChatGPTによる検索エンジンとして「ChatGPT search」をリリースしたことを受け、検索エンジン最適化(SEO)の専門家であるエリック・エンゲ氏が62件のクエリを使用してChatGPT searchとGoogle検索の違いを分析し、結果を公開しました。 ChatGPT search vs. Google: A deep dive analysis of 62 queries https://searchengineland.com/chatgpt-search-vs-google-analysis-449676 ChatGPT searchはAIがウェブ上の情報を検索して内容をまとめてくれるというもの。実際の使い方については下記の記事で確認できます。 ついにAI検索機能「ChatGPT search」が一般公開される、マップ機能も追加 - GIGAZINE 市場���査を行う企業「SparkToro」の調査によると、人々がGoogle検索を使用する時の「意図」は以下の通り。 ・ナビゲーション検索(32.15%) ナビゲーション検索は、例えばGIGAZINEにアクセスする際に「GIGAZINE」でGoogle検索して検索結果からアクセスする場合のように、訪問したいサイトが決まっているユーザーがウェブサイトのアドレスを入力する代わりにGoogle検索を使用する場合の分類です。 ・情報提供(52.65%) 興味のあるトピックに関する情報を探す場合の分類です。 ・商業目的(14.51%) 何かの製品の情報を検索したり、複数の製品を比較したりするためにGoogle検索を行うと「商業目的」として分類されます。 ・執行(0.69%) SparkToroは「マーケティングを行う上で価値のある検索」として、ユーザーがすでに何かの購入やサービスへの登録を決意した状態であることを示唆する検索を「執行」と別の分類に切り分けています。 エンゲ氏はSparkToroの調査結果を踏まえた上で、「情報提供」および「商業目的」に分類されるようなクエリに加え「ローカル検索」「コンテンツギャップ分析」「曖昧クエリ」という分類のクエリを合計62個用意。ローカル検索は「最寄りのピザ屋さんはどこ?」のようにユーザーの現在地が関係するクエリで、コンテンツギャップ分析は類似サイトの内容を比較するクエリ、曖昧クエリは「ジョーカーとは何ですか?」のように複数の意味が考えられるクエリです。 エンゲ氏はChatGPT searchとGoogle検索のそれぞれが返した結果に対し、下記の6つの基準で採点を行いました。 1:正確な情報を返したか? 2:重要な情報が欠落せずに含まれていたか? 3:回答に弱い部分は無かったか? 4:ユーザーのクエリの目的は解決されたか? 5:適切なフォローアップ情報を提供したか? 6:回答の全体的な品質はどうだったか? 分類ごとの結果は以下の通り。なお、クエリの中には複数の分類に重複して数えられているものがあるためクエリ数の合計が62個を超えています。 ・情報提供 クエリ数 42 勝者 Google ChatGPT searchの平均スコア 5.19 Googleの平均スコア 5.83 情報提供分野ではGoogleがわずかに優れているという結果になり、これまでGoogleが築き上げてきた情報検索における実績を改めて確かめることになりました。ただし、ChatGPT searchも多少の問題はありつつも良好なパフォーマンスを示したとのこと。 ・商業目的 クエリ数 16 勝者 Google ChatGPT searchの平均スコア 3.81 Googleの平均スコア 6.44 エンゲ氏は「Googleの方が製品やサービス関連の検索結果を表示する機能が優れている」と述べています。 ・ローカル検索 クエリ数 4 勝者 Google ChatGPT searchの平均スコア 2.00 Googleの平均スコア 6.25 Googleが多数のローカルビジネスのデータを確保していること��優位性へとつながっています。 ・コンテンツギャップ分析 クエリ数 4 勝者 ChatGPT search ChatGPT searchの平均スコア 3.25 Googleの平均スコア 1 類似サイトとのコンテンツの差を調べたり、検索結果ページの競合と比較したり、記事の内容を提案したりするクエリではChatGPT searchの方が優れているという結果になりました。ただし、全体的なスコアが低く、さらなる改善が必要とのこと。 ・曖昧クエリ クエリ数 7 勝者 ChatGPT search ChatGPT searchの平均スコア 6.00 Googleの平均スコア 5.29 ChatGPT searchは曖昧な言葉に対して複数の定義や解釈をより効果的に提示し、ユーザーに明確な情報を提供することができました。 今回の結果を踏まえ、エンゲ氏は「62個というクエリの数は極めて少ないサンプルであることに注意して欲しい」と述べた上で、「ChatGPT searchは情報提供クエリに関して良い回答をするものの、それでもGoogle検索の方が優れていた。結局、ほとんどの検索ではGoogleの方が優秀だと考えている」と結論付けています。 この記事のタイトルとURLをコピーする ・関連記事 Redditが掲示板上の話題を検索したり要約したりできるチャットAI「Reddit Answers」を発表 - GIGAZINE AIの台頭によって検索エンジンからサイトへのトラフィックが2026年までに25%減少すると調査会社が予測 - GIGAZINE GoogleのAIが検索結果をわかりやすく概説してくれる「AIによる概要」がついに日本語をサポート - GIGAZINE Metaが独自のAI搭載検索エンジンを開発中と報道される - GIGAZINE Mistral AIがチャットAI「Le Chat」を大幅アップデートしてウェブ検索機能や「FLUX1.1 [pro]」を利用した画像生成が可能に - GIGAZINE ・関連コンテンツ Google検索で「上手にググる」ための5つのポイントをソフトウェア工学の専門家が解説 Google検索チームに「Discoverに掲載されるには?」「長い記事は分割してもOK?」などを聞いてみた回答一覧まとめ Googleとフードデリバリーサービスが「広告」を使って地元のレストランから顧客を奪っているとの指摘 MozillaがYouTubeの動画推奨アルゴリズムを調査するために専用アドオンをリリース Microsoftの検索エンジンBingがTransformerからLLMとSLMの組み合わせに移行&TensorRT-LLMの統合を発表 Google検索結果の「AIによる概要」は全体の出現率が84%から15%に激減しているもののヘルスケア関連では63%と高水準 複数サイトのSEO関連情報比較を行う無料サービス「アクセス比較.jp」 Yahoo!とGoogleでの検索結果をRSSで出力できる「Search & RSS
AI検索エンジン「ChatGPT search」とGoogle検索を62件のクエリで比較してみた結果 - GIGAZINE
0 notes
Text
How I Passed the NVIDIA-Certified Associate: Generative AI LLMs Exam
Becoming a certified expert in generative AI is a significant milestone for any AI professional. The NVIDIA-Certified Associate: Generative AI LLMs (Large Language Models) Exam is designed to test an individual’s knowledge and proficiency in implementing and optimizing generative AI solutions using NVIDIA’s cutting-edge technologies. Anton R Gordon, a seasoned AI Architect with multiple certifications under his belt, shares his journey and strategies for acing this challenging certification.
Understanding the Exam
The NVIDIA-Certified Associate: Generative AI LLMs Exam focuses on foundational and practical aspects of generative AI using NVIDIA’s platforms. The key topics include:
Deep Learning Fundamentals: Understanding neural networks, training techniques, and optimization methods.
Generative Models: Proficiency in transformer models like GPT and BERT.
NVIDIA Frameworks: Familiarity with frameworks such as NVIDIA NeMo and TensorRT.
Deployment Strategies: Knowledge of deploying LLMs on NVIDIA GPUs for maximum efficiency.
Anton R Gordon emphasizes that understanding the real-world applications of these concepts is critical to performing well on the exam.
Preparation Tips
Anton’s success in earning this certification was the result of a well-structured preparation strategy. Here’s his step-by-step guide:
Leverage NVIDIA’s Resources
NVIDIA offers an array of learning materials, including online courses, technical blogs, and hands-on labs. Anton recommends starting with:
NVIDIA Deep Learning Institute (DLI): Take courses like Building Transformer-Based NLP Applications and Optimizing Deep Learning Models.
Documentation and Tutorials: Familiarize yourself with NeMo’s capabilities and use cases.
Master the Fundamentals
Before diving into advanced topics, ensure you have a strong grasp of:
Linear algebra and calculus for understanding model optimization.
Python programming, especially libraries like PyTorch and TensorFlow.
Neural network architectures and their training processes.
Anton advises dedicating at least two weeks to brushing up on these basics.
Practice with Real-World Scenarios
Hands-on experience is indispensable. Anton recommends:
Building transformer models using NeMo.
Fine-tuning pre-trained LLMs on domain-specific datasets.
Experimenting with deployment on NVIDIA GPUs using TensorRT.
Mock Exams and Community Engagement
Anton R Gordon stresses the importance of taking mock exams to identify weak areas. Additionally, participating in NVIDIA’s AI community forums can provide valuable insights and support.
Exam-Day Strategy
On the day of the exam, Anton suggests the following:
Time Management: Allocate time wisely for each section.
Focus on Practical Questions: Prioritize questions that test real-world application skills.
Stay Calm: Maintain composure to avoid mistakes under pressure.
Benefits of Certification
Achieving the NVIDIA-Certified Associate: Generative AI LLMs credential has numerous advantages:
Career Growth: Enhances your professional credibility and opens doors to advanced roles.
Technical Expertise: Demonstrates proficiency in deploying LLMs efficiently.
Networking Opportunities: Connects you with NVIDIA’s vibrant AI community.
Anton R Gordon attributes much of his career success to certifications like this, which validate and showcase his technical skills.
Conclusion
Passing the NVIDIA-Certified Associate: Generative AI LLMs Exam is a challenging but rewarding achievement. By following Anton R Gordon’s preparation strategies—leveraging resources, mastering fundamentals, and gaining hands-on experience—you can position yourself as an expert in generative AI. As the demand for AI professionals continues to grow, certifications like this are key to staying ahead in the competitive tech landscape.
0 notes
Text
First official release of Ceres, including several major enhancements:
support for Ceres neural networks
full support of Chess960 (also known as Fischer Random) and DFRC (Double Fischer Random Chess) with the "UCI_Chess960" option for mode selection (contribution by lepned)
support of ONNX neural networks via CUDA or TensorRT execution providers for Ceres and Lc0 networks
0 notes
Text
NVIDIA Nemotron-4 340B Open LLMs for Synthetic Data Training
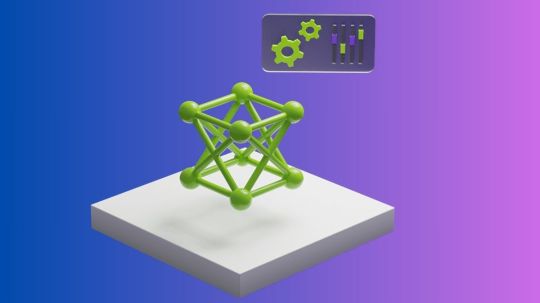
NVIDIA Nemotron-4 340B
NVIDIA unveiled Nemotron-4 340B, an open model family that allows developers to produce synthetic data for large language model (LLM) training in the industrial, retail, healthcare, and finance sectors, among other industries.
Robust training datasets might be prohibitively expensive and difficult to get, but they are essential to the performance, accuracy, and quality of responses from a bespoke LLM.
Nemotron-4 340B provides developers with a scalable, free method of creating synthetic data that may be used to construct robust LLMs, with a uniquely liberal open model licence.
Nemotron
The base, instruct, and reward models in the Nemotron-4 340B family work together to create synthetic data that is used to train and improve LLMs. The models are designed to function with NVIDIA NeMo, an open-source platform that enables data curation, customisation, and evaluation during the whole model training process. Additionally, they are designed using the open-source NVIDIA TensorRT-LLM library in mind for inference.
You may now get Nemotron-4 340B from Hugging Face. The models will be packaged as an NVIDIA NIM microservice with a standard application programming interface that can be deployed anywhere.
Getting Around the Nemotron to Produce Synthetic Data
LLMs can be useful in situations where access to big, diverse labelled datasets is limited for developers creating synthetic training data.
The Nemotron-4 340B Instruct model generates a variety of synthetic data that closely resembles real-world data, enhancing data quality to boost the robustness and performance of custom LLMs in a range of domains.
A large language model (LLM) called Nemotron-4-340B-Instruct can be utilised in a pipeline for synthetic data creation to produce training data that will aid in the development of LLMs by researchers and developers. This is a refined Nemotron-4-340B-Base model designed for English-speaking single- and multi-turn chat scenarios. A context length of 4,096 tokens is supported.
A dataset of 9 trillion tokens, comprising a wide range of English-based literature, more than 50 natural languages, and more than 40 coding languages, was used to pre-train the base model. The Nemotron-4-340B-Instruct model then underwent more alignment procedures, such as:
Monitoring and Adjustment (SFT)
Optimisation of Direct Preference (DPO)
Preference Optimisation with Reward Awareness (RPO)
While over 98% of the data utilised for supervised fine-tuning and preference fine-tuning (DPO & RPO) was synthesised by NVIDIA’s data creation pipeline, the company only relied on about 20,000 human-annotated data throughout the alignment process.
As a result, a model that can produce high-quality synthetic data for a range of use scenarios is created that is matched for human chat preferences and enhances mathematical thinking, coding, and instruction following.
NVIDIA affirms under the terms of the NVIDIA Open Model Licence:
The models can be used commercially.
It is not prohibited for you to develop and share derivative models.
Any outputs produced utilising the Models or Derivative Models are not attributed to NVIDIA.
Developers can then utilise the Nemotron-4 340B Reward model to filter for high-quality responses, which will improve the quality of the AI-generated data. Five criteria are used by Nemotron-4 340B Reward to score responses: verbosity, coherence, accuracy, helpfulness, and complexity. As of right now, it holds the top spot on the AI2-created Hugging Face RewardBench scoreboard, which assesses the strengths, vulnerabilities, and safety of reward models.
By combining their private data with the included HelpSteer2 dataset, researchers can further customise the Nemotron-4 340B Base model to construct their own teach or reward models.
Large language models (LLMs) such as Nemotron-4-340B-Base can be utilised in a synthetic data production pipeline to produce training data that aids in the development of LLMs by researchers and developers. With 4,096 tokens in the context, this model supports 340 billion parameters. It has been pre-trained on a total of 9 trillion tokens, which include more than 40 coding languages, more than 50 natural languages, and a wide range of English-based writings.
To enhance the quality of the pre-trained model, a continuous pre-training of 1 trillion tokens was carried out on top of the pre-trained model following an initial pre-training phase of 8 trillion tokens. NVIDIA changed the distribution of the data used during continuous pre-training from the one that was present at the start of training.
TensorRT-LLM Inference Optimisation, NeMo Fine-Tuning
Developers can maximise the effectiveness of their instruct and reward models to provide synthetic data and score responses by utilising the open-source NVIDIA NeMo and NVIDIA TensorRT-LLM.
Tensor parallelism a kind of model parallelism in which individual weight matrices are divided among several GPUs and servers is a sort of parallelism that is optimised into all Nemotron-4 340B models using TensorRT-LLM. This allows for effective inference at scale.
Nemotron-4 340B the NeMo architecture allows Base, which was trained on 9 trillion tokens, to be tailored to certain use cases or domains. Extensive pretraining data aids in this fine-tuning process, which produces outputs that are more accurate for particular downstream tasks.
The NeMo framework offers a range of customisation options, such as parameter-efficient fine-tuning techniques like low-rank adaptation, or LoRA, and supervised fine-tuning techniques.
Developers can use NeMo Aligner and datasets annotated by Nemotron-4 340B Reward to align their models and improve model quality. Using methods like reinforcement learning from human feedback (RLHF), a model’s behaviour is refined during alignment, a crucial phase in LLM training, to make sure its outputs are accurate, safe, acceptable for the context, and compatible with the model’s stated goals.
NeMo and TensorRT-LLM are also available to businesses via the cloud-native NVIDIA AI Enterprise software platform, which offers rapid and effective runtimes for generative AI foundation models. This platform is ideal for those looking for enterprise-grade support and security for production environments.
Assessing Model Security and Beginning
After undergoing a thorough safety examination that included adversarial tests, the Nemotron-4 340B Instruct model demonstrated good performance over a broad spectrum of risk indicators. It is still important for users to carefully assess the model’s outputs to make sure the artificially created data is appropriate, secure, and accurate for their use case.
Read more on Govindhtech.com
#nvidia#nvidianemotron#nemotron#nemotron4#govindhtech#news#technology#technews#technologytrends#tensorrt#tensorrtllm#Nemotron4340B
0 notes
Text
Chat with RTX: Create Your Own AI Chatbot
We hope you enjoyed this article about Chat with RTX, NVIDIA and generative AI. Please share your feedback, questions, or comments below. We would love to hear from you and learn from your experience.
Image Source – Newspatron Creative Team AI-Generated Image for representative purpose [Read About Us to know more] Do you want to have your own personal assistant, tutor, or friend that can answer any question you have, help you with any task you need, or entertain you with any topic you like? If yes, then you should check out Chat with RTX, a free tech demo from NVIDIA that lets you create…
View On WordPress
0 notes
Text
Nvidia Open-Source LLM - GPT-4 Rival
Join the newsletter: https://avocode.digital/newsletter/
Introduction to Nvidia's Open-Source LLM
The tech world is abuzz with excitement as Nvidia, a leader in computing power and graphics processing, has officially released its open-source Large Language Model (LLM), which many are calling a rival to OpenAI's famed GPT-4. This strategic move marks Nvidia's deeper foray into the realm of artificial intelligence, positioning itself as a formidable competitor in the AI landscape. With advancements that suggest it might be on par with, or even surpass, current industry standards, this innovation has captivated both developers and tech enthusiasts alike.
Why Nvidia's Move Matters
Nvidia's decision to introduce an open-source LLM is significant for several reasons: 1. Democratization of AI technology: By releasing this model as open-source, Nvidia is enabling developers, researchers, and organizations across the globe to access cutting-edge AI technology. This accessibility fosters innovation and collaboration across various sectors such as healthcare, finance, and entertainment. 2. Competition Drives Innovation: With GPT-4 setting a high standard, Nvidia's entry into the space shows healthy competition. This rivalry pushes both companies to continuously improve and innovate, benefiting the entire tech ecosystem. 3. Leverage of Computational Power: Nvidia is renowned for its high-performance GPUs. By integrating its LLM with its hardware, it promises unparalleled performance and efficiency, setting a new benchmark in AI processing power.
Nvidia's LLM Features and Capabilities
Nvidia's open-source LLM brings several innovative features to the table:
Advanced Natural Language Processing
The model boasts highly sophisticated NLP abilities, capable of understanding and generating human-like text. Its prowess in language comprehension and generation makes it ideal for applications ranging from chatbots to complex data analysis.
Enhanced Scalability
Built to be scalable, Nvidia's model can be deployed across various platforms, from personal computers to large data centers. This flexibility ensures that businesses of all sizes can leverage its capabilities without sacrificing performance or incurring excessive costs.
Integration with Nvidia's Ecosystem
The open-source LLM seamlessly integrates with Nvidia's existing ecosystem. Developers can take advantage of Nvidia's CUDA and TensorRT for efficient deployment, while the model benefits from the acceleration provided by Nvidia GPUs. This symbiosis results in faster training times and real-time AI applications.
Comparing Nvidia's LLM with GPT-4
While Nvidia's open-source endeavor invites comparisons to OpenAI's GPT-4, there are distinct differences that merit attention: -
Open-Source Approach: Unlike GPT-4, which is proprietary, Nvidia's LLM is open-source, encouraging innovation and adaptation across diverse user groups.
-
Hardware Optimization: Nvidia's model is optimized for its GPU architecture, providing potentially superior performance metrics in some scenarios compared to GPT-4.
-
Community Involvement: By allowing a broader range of contributions and experiments from the tech community, Nvidia’s model could evolve rapidly in ways that GPT-4 may not.
Potential Applications
The possibilities with Nvidia's LLM are endless, spanning multiple industries and applications:
Healthcare
In healthcare, the LLM can be utilized for accurate diagnostic predictions by analyzing patient data and medical literature to provide insights and potential treatment plans.
Automated Customer Service
Businesses can customize the LLM to develop intelligent chatbots and virtual assistants that offer personalized customer interactions, enhancing user satisfaction and operational efficiency.
Content Creation
The model's sophisticated language generation capabilities can aid media companies by streamlining content creation processes, aiding in the production of articles, scripts, or even creative writing projects.
Challenges and Considerations
While the potential benefits of Nvidia's open-source LLM are substantial, there are challenges and considerations to address:
Data Privacy and Security
With AI models handling sensitive data, ensuring strict adherence to data privacy laws and using secure data handling practices is crucial.
Ethical Concerns
Like other AI models, Nvidia's LLM must contend with ethical concerns such as bias and misinformation. Developers need to actively work towards minimizing biases in training data and ensuring the responsible use of AI technology.
The Future of AI with Nvidia's Open-Source LLM
As Nvidia steps forward with its LLM, the future of AI appears increasingly dynamic and collaborative. The open-source model not only levels the playing field by providing access to advanced AI technology but also motivates other tech giants to innovate at a similar pace. In conclusion, Nvidia's introduction of its open-source LLM signifies a pivotal moment in the AI industry. By making sophisticated AI accessible and encouraging a collaborative spirit, Nvidia is not only aiming for parity with GPT-4 but also charting a new course for AI development, one marked by openness and innovation. This development represents a quantum leap forward in how LLMs can be built, shared, and utilized across industries, setting the stage for an exciting future in artificial intelligence. Want more? Join the newsletter: https://avocode.digital/newsletter/
0 notes
Photo
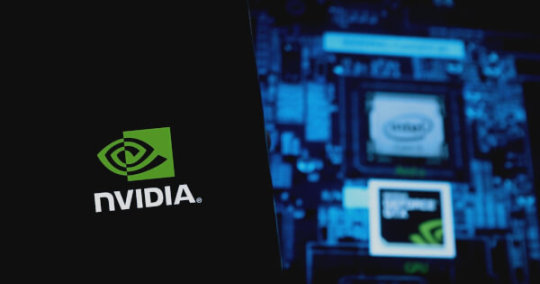
NVIDIA Enhances TensorRT-LLM with KV Cache Optimization Features
0 notes
Text
Some Non-Obvious Points About OpenAI 01
New Post has been published on https://thedigitalinsider.com/some-non-obvious-points-about-openai-01/
Some Non-Obvious Points About OpenAI 01
Plus some major funding rounds by World Labs and Glean , Mistral’s new release and more.
Image Credit: OpenAI
Next Week in The Sequence:
Edge 431: Our series about space state models(SSMs) continues with an overview of multimodal SSMs. We discuss the Cobra SSM multimodal model and NVIDIA’s TensorRT-LLM framework.
Edge 432: Dives into NVIDIA’s Minitron models distilled from Llama 3.1.
You can subscribe to The Sequence below:
TheSequence is a reader-supported publication. To receive new posts and support my work, consider becoming a free or paid subscriber.
📝 Editorial: Some Non-Obvious Points About OpenAI 01
The release of OpenAI’s new model dominated headlines this week. The o1 models are specialized in reasoning and planning, areas that have long been of interest to OpenAI. Much of the debate in online circles has focused on the model’s specific capabilities, such as whether the terms “reasoning” and “thinking” are appropriate, so there is plenty of content discussing that. Instead of contributing to the debate, I wanted to highlight a few key points that I found particularly interesting while reading the o1 technical report.
It seems that the o1 models were trained and fine-tuned using different methodologies compared to their predecessors. Specifically, OpenAI used reinforcement learning optimized for chain of thought (CoT) scenarios, which is somewhat unique.
Initial results indicate that this reinforcement learning for CoT technique can scale significantly, potentially leading to new breakthroughs in reasoning and planning.
Only CoT summaries, rather than complete CoT traces, are available via the API, making it difficult to determine how the model arrives at specific outputs.
Somewhat paradoxically, CoT-focused models might lower the entry point for interpretability since we are starting with a baseline of reasoning traces.
One of the most interesting aspects of o1 is the shift from training to inference compute time. Inference, rather than training, is increasingly becoming a key requirement for complex reasoning tasks. The reasoning core doesn’t necessarily need to be a large model, which could translate into decreases in training time. We will need to see how this strategy evolves over time.
This point makes me think we might be witnessing the start of a new set of scaling laws focused on inference.
The red-teaming efforts for o1, with companies such as Apollo Research and Haize Labs, are quite impressive and worth diving into in the technical report.
Unsurprisingly, o1 is much harder to jailbreak than previous models, and it spends much more time on inference. That said, there have already been several successful jailbreak attempts.
OpenAI o1 clearly shows that reasoning is one of the next frontiers of foundation model research and, more importantly, that improvements in foundation model architectures are not stalling—they may just take some time to materialize.
🔎 ML Research
LLMs for Novel Research Ideas
AI researchers from Stanford University published a study about the research ideation capabilities of LLMs. The experiment draws a comparison between human- and LLM generated ideas across different nove fields. The results might surprise you —> Read more.
Agent Workflow Memory
Researchers from MIT and Carnegie Mellon University published a paper introducing Agent Workflow Memory(AWM), a method for reusable tasks workflows in agents. AWM, introduces reusable tasks to agents so that they can be used to guide future actions —> Read more.
Modular LLMs
Researchers from Princeton University, Carnegie Mellon University , Tsinghua University, UCLA and several other AI labs published a paper proposing a modular design for LLMs. Specifically, the paper introduces the term of “brick�� to define a functional block within an LLM and highlights the efficiencies of following this composable approch for LLM construction —> Read more.
Better Math Agents
Google DeepMind published a paper introducing a preference learning framework to optimize the performance of math AI models. The framework uses techniques such as multi-turn and tool-integrated reasoning to improve the efficiency of single-turn math models —> Read more.
WINDOWSAGENTARENA
Researchers from Microsoft, Columbia University and Carnegie Mellon University published a paper detailing WINDOWSAGENTARENA, an environment for evaluating agents in tasks in the Windows OS. The environment includes over 150 diverse tasks that requires capabilites such as screen understanding, tool usage and planning —> Read more.
LLaMA-Omni
Researchers from several elite chinese AI labs published a paper proposing LLaMA-Omni, an architecture for integrating speech interactions with open source LLMs. LLaMA-Omni integrates a pretrained speech encoder, a speech adapter and a streaming speech decoder with an LLM such as LLaMA in order to process text and speech data simulataneously —> Read more.
🤖 AI Tech Releases
OpenAI o1
OpenAI released a new family of models specialized in reasoning —> Read more.
AgentForce
Salesforce unveiled AgentForce, its platform for autonomous AI agents —> Read more.
DataGemma
Google open sourced DataGemma, a series of small models grounded in factual data —> Read more.
Pixtral 12B
Mistral released Pixtral 12B, its first multimodal model for images and text —> Read more.
🛠 Real World AI
AI for Coding at Salesforce
Salesforce discusses CodeGenie, an internal tool used to boost developer productivity using generative AI —> Read more.
Data Center Cooling at Meta
Meta discusses the reinforcement learning techniques used for cooling optimization in their data centers —> Read more.
📡AI Radar
AI pioneer Fei-Fei Li’s company World Labs raised another $230 million.
AI-search platform Glean raised $260 million in a Series E.
OpenAI is rumoured to be raising a new round at a $150 billion valuation.
Google co-founder Sergey Brin gave a rare interview about his recent work on AI.
Arcee AI released its SuperNova 70B model.
AI agent platform Landbase came out of stealth with $12.5 million in funding.
InMobi secured $100 million for AI acquisition ahead of its IPO.
AI bookkeeping startup Finally raised $200 million.
Stability AI and Lenovo partnered for text-to-image capabilities.
AI translation platform Smartcat raised $43 million.
ServiceNow unveiled a series of AI agents for customer service, procurement, HR and others.
OffDeal announced a $4.7 million round to improve M&A for small businesses.
AI-powered compliance platform Datricks raised $15 million in a new round.
TheSequence is a reader-supported publication. To receive new posts and support my work, consider becoming a free or paid subscriber.
#acquisition#agent#agents#ai#ai agent#AI AGENTS#AI models#AI-powered#amp#API#architecture#autonomous#billion#Carnegie Mellon University#circles#coding#Companies#comparison#compliance#construction#content#cooling#customer service#data#Data Center#Data Centers#decoder#DeepMind#Design#Developer
0 notes