#Robotica
Explore tagged Tumblr posts
Text










Duckverse ladies - Duck comics, Ducktales, Duck Avenger, Double Duck, Darkwing Duck, Quack Pack - Happy Women’s Day - Classic Disney
Although it may be too late, I wish everyone a happy Women’s Day, which is celebrated on March 8!
I already posted it three years ago, where you can see it here: https://ducklooney.tumblr.com/post/678203544865816576/happy-women-day-but-with-classic-disney
First part: https://ducklooney.tumblr.com/post/777495112860188672/mouseverse-goofyverse-dogverse-and-chip-and
I decided to post it again, this time divided into two parts from classic Disney. The first is the Mouseverse and Goofyverse with the Chip and Dale Rescue Rangers team with ladies and women, the second is the Duckverse female ladies. Since I didn't care about everything in the previous post, I decided to post it with Duckverse female characters in two parts, so this is the second part. There's more, but it would be really too much to post them all. Sorry! Of course, to everyone who celebrates, I wish you a happy women’s day and if you are a fan of these female characters, feel free to like and reblog this.
#happy international women's day#women's day#happy women's day#duckverse#ducktales#disney duck comics#comics#disney#cartoons#duck comics#disney comics#darkwing duck#the legend of the three caballeros#duck avenger#fantomius#double duck#quack pack#disney ducks#disney birds#disney duckverse#xandra#clara cluck#m'ma crackshell#robotica#xadhoom#birdie mcquack#sara bellum#camillie chameleon#millionaira vanderbucks#zafire
54 notes
·
View notes
Text

Robotica from DuckTales.
Gyro was very lonely when he made her.
#domono08#cartooning#blogs#artists on tumblr#hand drawn#animation#black artist#fan artist#furry fandom#anthro#ducktales#ducktales 1987#disney afternoon#disney#disney fanart#robotica#maid#android#robot#duck#anthro art#art#my art#furry#furry art#furryart#furrydrawing#furry character#fanart
49 notes
·
View notes
Text
The robot girl of the day is Robotica from DuckTales (1987)!
63 notes
·
View notes
Text

🚨No es ciencia ficción: han revivido arañas muertas como robots.
Lo llaman “necrobótica” y permite aumentar la fuerza de agarre de las arañas hasta mil veces. 🕷️
Te dejamos el video de cómo lo hicieron en el enlace. 👇
11 notes
·
View notes
Text
Random screencaps about my fav character from ducktales 1987


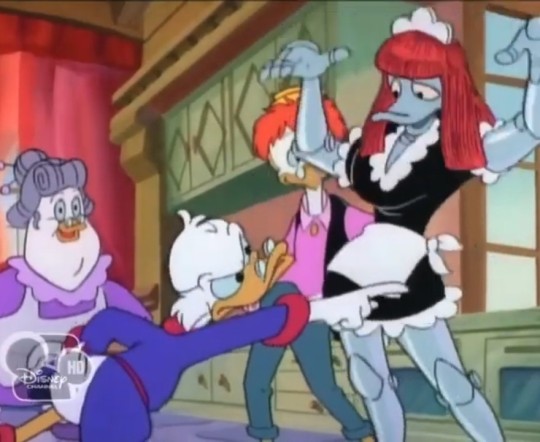
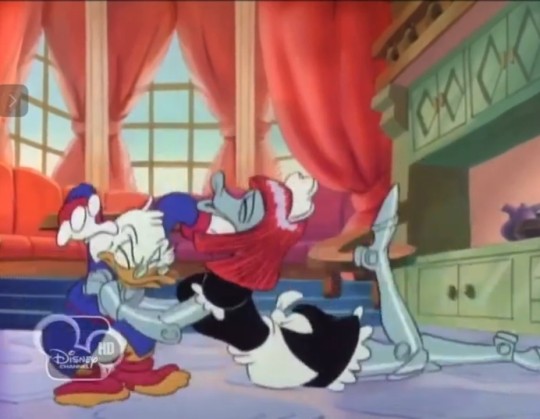
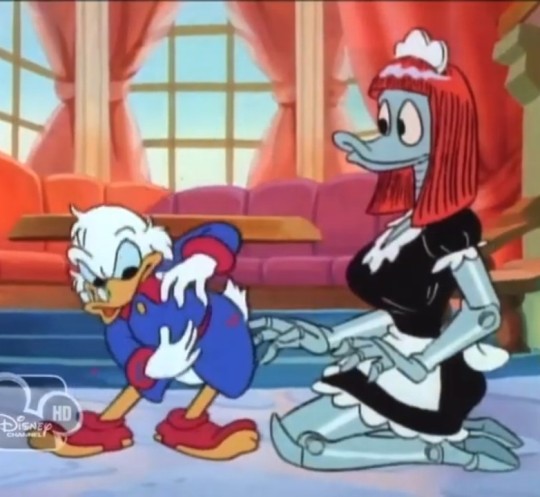


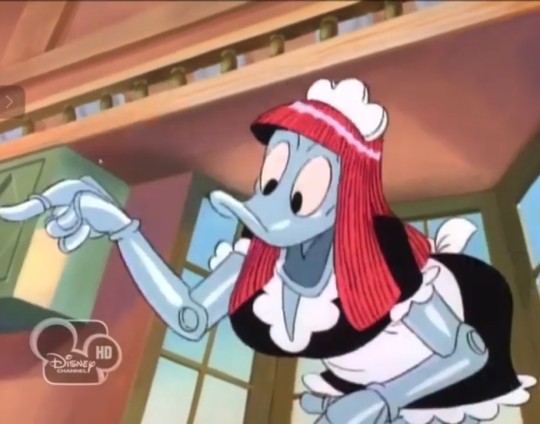

This screencap is my fav XD
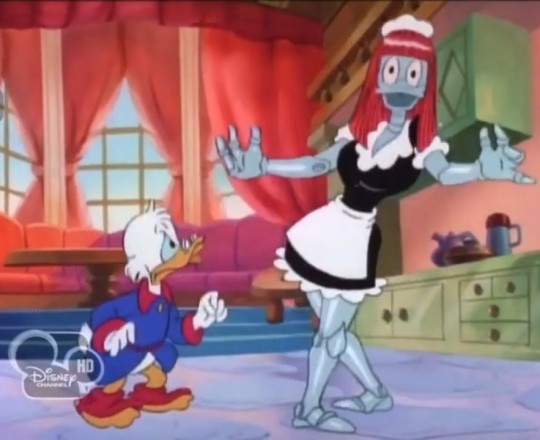
29 notes
·
View notes
Text
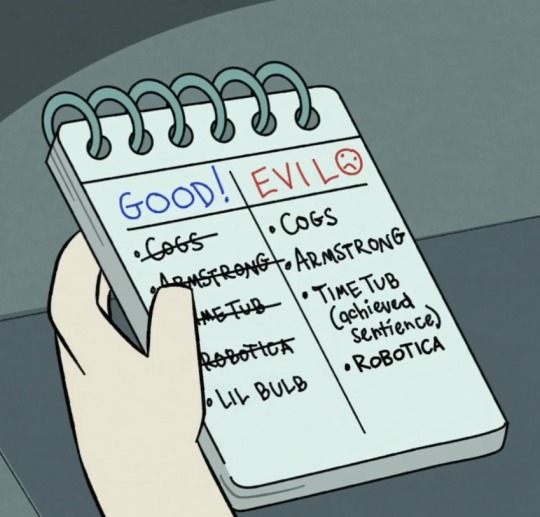


#ducktales polls#ducktales fandom#duckblr#ducktales 2017#ducktales 1987#gyro gearloose#Armstrong#Robotica
19 notes
·
View notes
Photo

kaminosekkei : No. 442: Robotica. (📷 by @renellaice and model: @zenduh)
#kaminosekkei#kami no sekkei#ely lara#robotica#renellaice#zenduh#cyberpunk#art#photomanipulation#cyborg#mech#tech#cybernetics#body mods
66 notes
·
View notes
Text
#robot#robotics#robots#technology#art#engineering#arduino#d#electronics#transformers#mecha#tech#toys#anime#robotic#scifi#gundam#ai#drawing#artificialintelligence#digitalart#innovation#illustration#electrical#automation#robotica#diy#design#arduinoproject#iot
33 notes
·
View notes
Text
Robotica / Deadalus on SEGA Saturn 50/60Hz comparison and region changes.
A little while back, I got an email from a kind reader telling me the PAL version of Robotica Cybernation Revolt looked optimised on Saturn. It was an early list entry and on re-review having picked up a cheap Japanese copy of the game, I can confirm it was, but only a little.
What's interesting is as I got a Japanese version, I noticed a number of region changes, between the two versions not really discussed online. Robotica is one game I wrote off a bit back in the day as an average FPS.
Sitting down and playing it this weekend, it's an interesting early Saturn titles with some impressive ideas and elements. It does get very repetitive and the environments are rather dull, but it's got some gameplay elements rarely seen on the system.
The game also does do a great job of building the atmosphere and tension. The game is a Roguelike or Mystery Dungeon games that has you battling your way through 30 floors of the labyrinth that make up the Deadalus space computer. The Japanese name is a nod to Greek mythology as Deadalus was the builder of the labyrinth that trapped the Minotaur.
Most of the 30 floors are randomly generated each time to play, apart from a few mission based ones. The game is clearly designed for people to speed run over and over again, as there is no save feature or option menu and the game can be cleared in under two hours. The aim is simple on each floor find the key and escape. Where the key is, the map layout and the exit are changes each time you play, as do enemy placements and rooms. So you can land in a starting room with the exit and key in it or have to explore every corner of the map for both to find them in another floor.
It's a decent FPS, with a soundtrack that gets the tension right, the music also has a subtle touch of becoming more upbeat on each stage after the key is found. The map slowly fills itself in as you explore, it's all real time and tracks the path you have taken as well. Each floor also has a computer terminal placed on it that when found and accessed, shows the whole floor and also turns on the lights in some. Few games did this at the time and the map is a full on 2D transparency, along with other elements of the HUD.
You quickly learn you have to balance your ammo and robots abilities in order to survival.
The lighting effects for an early 3D Saturn game are also well done. The enemy sprites also scale really well. Where it doesn't hold up as well is the frame rate which ranges from about 8-30fps a second, pending on what your are doing. The game seems to run at 60fps like almost all Saturn games, but doesn't use it and most elements are locked at 30fps, only the damage effect on your crafts seems to use it and only for a few frames.
I do wonder if this is a game that isn't tied to the frame rate as special skills like the hover jet allow you to zoom around the map in seconds, if at uncontrollable speeds.
The main reason for this blog post is the region changes are rather interesting. On the PAL optimisation front the images has been stretched a little. It's hard to tell if the speed has been changed due to the yo-yo like nature of the frame rate. At 50Hz it is slower that the NTSC-J version at 60Hz and having compared both versions at 50Hz they look to run at roughly the same speed.
Left is the NTSC Japanese version and right is the PAL version, both running at 50Hz.
As we can see the PAL version is stretch a little at 50Hz to take up a little more of the screen. However this ratio changes mean that elements of the map are a little distorted. At 60Hz in the Japanese version the game's map is a perfect square, which is not the case with the PAL version running at 50 or 60Hz. The PAL FMV intros are also misaligned a little on screen at 60Hz and are a little bit lower than they should be, but nothing major.
Where things get interesting is the HUD in the PAL and NTSC U versions. The main cursor for the HUD seen in the NTSC J version has been removed.
While it looks like a big circle in the middle of the screen, it acts as the crosshair for your weapons. As you move the yellow marker in the center moves up or down with the Laocorn, to show the direction any shot will travel in. The arrows light red to show your direction of travel and also the speed etc.. It's rather useful and to the left of the HUD it has a timer telling the player how long they are taking, perfect for the speed nature of the game. Below the timer is the radar which is the only part of the center HUD that was kept in the western versions, but pushed to the far left of the screen.
Other changes are that the English text is slightly improved in the western versions and the collected key image looks different. Its position has also changed, can't help, but think the key in the western version doesn't look right and I suspect the visual change might be a bug. Also the energy bar's position on screen has been lowered a little in the PAL version.
Left NTSC J version and Right PAL version both at 60Hz. Which show the text and key design changes.
You can also see the PAL version takes up the whole screen at 60Hz in the above images.
Finally one last little change Japanese text was included in the opening intro, but was removed in the English version.
There aren't a huge amount of changes, but the HUD's removal does impact the gameplay. I would take a guess that SEGA of Europe or Acclaim who published it in the USA felt the HUD took up to much screen space. Pictures of the HUD are on the European box, but not in-game. The lost of the HUD does lose some of the immersion of the game as you are meant to be controlling the Laocorn robot I will admit. It also makes shooting a little harder as you lose the crosshair.
Its removal does nothing to improve the game and I think a much needed high score and save system, should have been added. To track your completed runs would have been a better addition. It's not the greatest game ever made, but can still be picked up fairly cheaply and is a fun game in short bursts if you like this type of gameplay. I will admit that I will be playing the Japanese version from here on out. As the original HUD to me improves the game.
Follow Randomised Gaming on Tumblr, YouTube, Twitch & Twitter for video gaming & video content! Buy us a tea on Ko-fi
6 notes
·
View notes
Text

The greatest lesson in life is to know that even fools are right sometimes. (Winston Churchill)
#nftartwork#nftmarketplace#digitalillustrator#robotica#nftartcollector#marchofrobots#gianluca#nft4art#illustratordesign#robotspirits#iloverobots#robotshow#vectorrobot#vectorandroid
23 notes
·
View notes
Text
Robô humanoide visto passeando com cachorro robô chamou atenção em Niterói, no Rio de Janeiro.
#tecnologia#tech#technology#robotica#robotics#robot#robots#robos#robo#cao#dog#humanoid#humanoide#niteroi#rio de janeiro#brasil#brazil
3 notes
·
View notes
Text
New poll, second part. The best Duck Female in the Disney Duckverse. Who do you think is the best female duck character from the Duckverse?
Since unfortunately not everyone could fit in the previous poll who is the best female Duckverse character from the Donald Duck franchise (comics and cartoons), I will add another poll with the best female characters from the Duckverse, so the best from this poll and the previous ones will go head to head in the final. And you should decide who you think is the best female character from the Duckverse. This is the second part and this is just for fun. The best passes at the end. Although it has passed, I certainly wish all women a happy women’s day! And this month has been declared the month of women! Yes, it is celebrated on March 8! There are certainly a lot of female characters from the Duckverse, and I will leave only the most important ones from various franchises (cartoons, comics) and you have the choice to choose who you think is the best female Duck character from the Duckverse in general. Happy Women’s Day once again and this is just a poll for fun and a little play!
The previous poll about female duck characters see here: https://ducklooney.tumblr.com/post/745243822575042560/new-poll-the-best-duck-female-in-the-disney

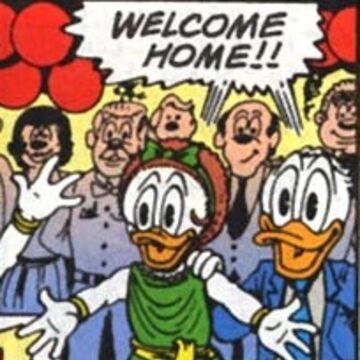

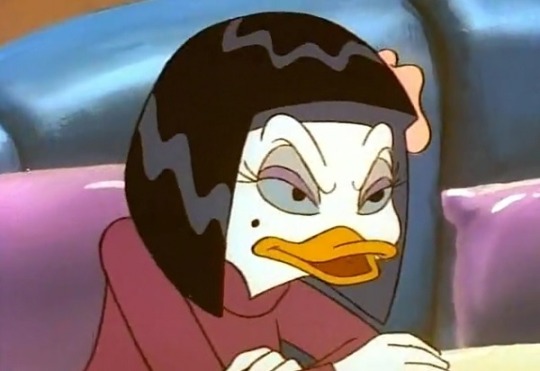

#poll#happy women’s day#women's day#women's month#duckverse#ducktales#comics#darkwing duck#duck comics#disney duck comics#ducktales 1987#pkna#three caballeros#ze carioca comics#grandma duck#splatter phoenix#elvira coot#bentina beakley#matilda mcduck#rosinha maria vaz#rosinha vaz#hortense mcduck#robotica#cinnamon teal#binkie muddlefoot#princess oona#downy o'drake#mrs beakley#downy mcduck#disney ducks
31 notes
·
View notes
Text

25 notes
·
View notes
Text
youtube
#estoesloquesomos#tumblrvideo#explore#robotica#tecnologia#inteligenciaartificial#automatizacion#innovacion#ingenieria#futuro#robot#Youtube
5 notes
·
View notes
Text
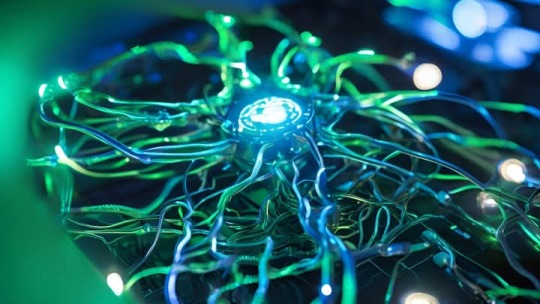
🚨ATENCIÓN🚨
Una investigación reciente ha revelado la posibilidad de crear neuronas robóticas capaces de procesar información a velocidades muy superiores a las humanas, con importantes implicaciones en la inteligencia artificial y otras áreas.
Te explicamos como lo consiguieron:
4 notes
·
View notes
Text
Il cibo del futuro sarà più sostenibile, personalizzato e tecnologico. Questo articolo ti porta alla scoperta delle innovazioni che stanno rivoluzionando l'industria alimentare: fattorie verticali che producono cibo a km zero, stampanti 3D che creano piatti su misura e robot chef che automatizzano la cucina. Preparati a un'esperienza culinaria completamente nuova!
#foodtech#agritech#agricolturaurbana#stampanti3dfood#robotica#cucinadelfuturo#sostenibilità#innovazione#foodinnovation
2 notes
·
View notes