#Min1
Explore tagged Tumblr posts
Text
@Liroh
2 notes
·
View notes
Text
1 note
·
View note
Text
drdt x dr lapse arei, charles, eden, hu, j and veronika
ace should've been there too but since his talent is the same i decided to not change anything in his design... sorry.
These six, Ace, Teruko, Whit, and Min1 are participants of the 50th Killing Game by XF-ture Tech (Preservation Project).
1Min is one of the two survivors of the 49th Killing Game. She killed the Ultimate Knight and got away with it. However, someone really wanted her to go through this nightmare again.
writing about all of them in one post is too long so i wont do it... but if you want to ask something about this au my askbox is always open, i'll try to answer as quickly as i can
yes, veronika's pose IS a matpat reference (i've never watched a single matpat video in my life)
#drdt#danganronpa: despair time#drdt au#arei nageishi#charles cuevas#eden tobisa#hu jing#j moreno#j rosales#veronika grebenshchikova
114 notes
·
View notes
Note
You horny level now?
Min1 max10

Honestly pretty comfy right now so the horny level is not that high 😇
23 notes
·
View notes
Note
Cuántos ships y oc tienes?

Aver mejor pongo los nombres para no olvidarme: brisa x Nat, tausi x Pao , Bianca x Paulo, Abby x Eddy, Sarai x mark, mei x julicia, jackson x alma, sara x nicolas, Valentino x akane, lsaac x lily, Freya x Aiden, Annie x Scarlett, Louis x Scarlett x Mila, Ximena x doku,Tábatha x asher , Amelia x Van (algo así se llama), akari x Paul, Yukitox Violet, Ana x jake, Daniela x Jonathan, emily x may, Diego x Andre, Lunaxy, Paula x Leila, lucia x keil, zoe x juny, Isabella x v3x, stefan x İzzy, Youssef x Olivia, Min x Harley, Robert x connor Fabricio x Berenice
Los de rosa aún no son canon Y aqui dejo a los creadores de los ocs :Nat,tausi, bianca @/winlik300 Eddy, Mark, Mei, Jackson, Nicolas, akane, Isaac @/totty-00 Aiden @/felixOwO Doku,Tábatha @/11n-min1 van @/star-dust824 Paul,Yukito @/ayase-yukina12345 Jake @/corderitonegrouwu Jonathan, emily, Diego @/fantasma027 y, Leila,keil, juny @/corrupted-and333 V3x,Stefan @/vampire-bun-bun Youssef @/loststuff min @/the-liminalwitch Connor @/lachingaderita-OwO fabricio @/puffystuff
Y sobre los nombres de mis ocs lo puedes encontrar fácil en mi descripción (aunque aún no lo e actualizado con mis otros ocs quq)
10 notes
·
View notes
Text


Аукцион 2 адопта. нач500р. мин60р. авто2000р. Каждый отдельно. Auction 2 adopt. start6$. min1$. auto24$ each separately.
4 notes
·
View notes
Text
Let's apply this idea to c major.
C Major/Ionian = C-D-E-F-G-A-B-C
D Dorian = D-E-F-G-A-B-C-E
E Phrygian = E-F-G-A-B-C-D-E
F Lydian= F-G-A-B-C-D-E-F
G Mixolydian= G-A-B-C-D-E-F-G
A Aeolian = A-B-C-D-E-F-G
B Locrian = B-C-D-E-F-G-A-B
Thankfully the chord pattern for each works the same way.
Ionian (Major)
I: C maj - II: D min - III: E min - IV: F Maj - V: G Maj - VI: A min - VII: B dim
Dorian
I: D min - II: E min - III: F maj-IV: G maj - V: A min - VI: B dim - VII: C maj1
Phrygian
I: E min - II: F maj - III: G maj - IV: A min - V: B dim - VI: C maj - VII: D min1
Lydian
I: F maj - II: G maj - III: A min - IV: B dim - V: C maj - VI: D min - VII: E min1
Mixolydian
I: G maj - II: A min - III: B dim - IV: C maj - V: D min - VI: E min - VII: F maj1
Aeolian (Natural minor)
I: A min - II: B dim - III: C maj - IV: D min - V: E min - VI: F maj - VII: G maj1
Locrian
I: B dim - II: C maj - III: D min - IV: E min - V: F maj - VI: G maj - VII: A min -
0 notes
Note
Your horny level now? Min1 max10
about a 5
my horniness comes in waves (pun not intended)
0 notes
Note
You how horny level now?
Min1 max10
Max 10 for sure always horny heh
0 notes
Text
week_4
here Basic scatter plot for Co2 emissions and breastcanserper100th.
this plot is over all data without subset.. we can see an outlier in CO2 emissions
outlier is with red colour marked below in picture.
the same Plot with regression line
I want to filterout this outlier to see the other measuring points more clearly.Because of that, I create a subset of data
scatter plot with data from sub2 dataframe
I create a new subset (sub4) to see the range until CO2 emission value 15.e9
scatterplot for data sub4 (co2 emissions <15e9) and breastcanserper100 is below.
we can see a positiv but weaker relationsheep.
distribution is very big
I create co2emissionsgroup in 10 Levels..
class 10 is the level for NaNs in rows.
bivariate bar graph and plot bar graph to see
I changed x Axis Labeling
the evaluation above is done with all datarows(without subsets)
if we are interested in specific areas, we need to filter and define data as a subset.
We can see a corolation between beastcanser and CO2 emissions.
other way1
we have to change labeling of x axis..
We can see in 9 groups of CO2 emissions , mean Value of breastcanser per 10000 women
other way2
We can see in 4 quartilies groups of CO2 emissions , mean Value of breastcanser per 10000 women
Corrolation shows, that with increased CO2 emissions, the number of women who get breast cancer also increases ...
print ('co2emissions') print ('---------------------') print ('mode') mode1 = data['co2emissions'].mode() print (mode1)
print ('mean') mean1 = data['co2emissions'].mean() print (mean1)
print ('std') std1 = data['co2emissions'].std() print (std1)
print ('min') min1 = data['co2emissions'].min() print (min1)
print ('max') max1 = data['co2emissions'].max() print (max1)
print ('median') median1 = data['co2emissions'].median() print (median1)
print ('breastcancerper100th') print ('---------------------')
print ('mode') mode2 = data['breastcancerper100th'].mode() print (mode2)
print ('mean') mean2 = data['breastcancerper100th'].mean() print (mean2)
print ('std') std2 = data['breastcancerper100th'].std() print (std2)
print ('min') min2 = data['breastcancerper100th'].min() print (min2)
print ('max') max2 = data['breastcancerper100th'].max() print (max2)
print ('median') median2 = data['breastcancerper100th'].median() print (median2)
0 notes
Link
0 notes
Link
0 notes
Text
Tametsi Effect
I see them in my sleep. Patterns, Mines, One-Two-Ones, Two out of Threes, Lines connecting squares indicating that YES, there is a EXACTLY one across all these fields. The patterns have texture, sometimes they are so familiar that even the logical connections of connections have become routine. Today I found a series of wild jumps in a corner and the whole mess of entanglements fell down into a singular, solid state of free and taken space.
Tametsi is a minesweeper game of 100 Puzzles and 60 bonus puzzles. It came out in 2017, and costs 2.35€ on Steam. Its also one of the greatest games I have ever played. I am not kidding.
The puzzles of Tametsi are basicaly just Minesweeper. Find the mines, mark them with a right click, find the safe spots and left click to reveal more numbers. The numbers say how many mines are next to them. There are a few different variations of shapes the board can be made out of, although mostly its just squares or hexagons. Oh and one more thing - these levels are all made by hand, crafted up to the smallest details
---by a mad architect who is forcing me to look at all possible futures all at once.---
There is never a point in Tametsi where you have to guess. Let me say that again - THERE IS ALWAYS EXACTLY ENOUGH INFORMATION YOU CAN SEE TO FIND OUT WHERE THE NEXT MINE IS AND IS NOT. no matter how obscure that information may be.
Tametsi teached me tricks. The most basic one I quickly had to put to constant use - marking tiles as connected by having an exact number of mines. If the mine is on the right, it can't be on the left, and vice verca.
---Each possibility nessecitates others to be false, in order to be true. For now, the cat is both dead and alive---
In order to compress information, Tametsi boasts an innovative feature: Painting Mode! Using a wide variety of colors and brush sizes you can make your screen look like you are trying to solve the great murder of 1978! My most simple tool is a simple connecting line ---one mine--- and if im fancy the double line ===two mines=== And soon after, I can start to combine the lines! A shorter line that exactly matches a longer one means all those extra tiles can't be mines! A single three long right next to a double three long means one mine here, and no mine there.
____ When one line meets a number in part, there can be only a maximum of one mine there, but also maybe none at all____ Max 1
But Max 1 on a 3 means Min2 on the rest of the spaces and oh look there is another 3 right next to it andthenmin1thereandthatgivesmemax1herebecauseofthe2ANDOHMYGODTHERENEEDSTOBEATLEAST1HERETHATMEANS
Min1 & Max1 => line Line & 2 => line Line & 3 => double Double & 3 => line
Uhh this line is shorter now!
What the fuck am I doing here?
Recently I have been obsessed with LOGIC. In first-order LOGIC there are TWO STATES a preposition can be in: MINE and NOT A MINE. These prepositions are interlinked/interlinked through logical connecters such as AND, OR, EXISTS, FOR ALL, TWO, THREE, LINE, DOUBLE LINE, MIN, MAX, FIFTEEN GREENS, FOUR IN COLUMN SIX
---Consider all possibilities to be in a possibility space---If either A or B or C have to be true and they all share that THERE IS A MINE ON THE OTHER FUCKING SIDE OF THE PUZZLE YOU ASSHOLE GENIUS TORTURE DEMON I HAVE SAT HERE FOR 20 MINUTES NOT ONE MINE TO MY NAME---Then all possibilities where there is no mine there can be discarded. The cat is dead.
Have fun!
0 notes
Photo
[transcript]
alex: look at you: all grown up, flying the nest.
hae-won: haha, thanks mom.
alex: i mean it, i’m actually really proud of you.
hae-won: this is crazy, isn’t it?
alex: oh god, absolutely.
hae-won: she could be an axe murderer.
alex: oh yeah. big time. but for someone who never used to leave the house, you did good. and if anyone had to live in the wilderness with a hot girl... well, i’m glad it’s you.
hae-won: hey, thanks. i’ll remember that in my final moments. then i’ll come back to haunt the shit out of you.
#ts4#sims 4#min1#min legacy#ts4 legacy#alex being back? instant serotonin#sry we arent gonna see her for a while sdghasg
19 notes
·
View notes
Audio
(_Min1_) MIN! TÁ NO MIC
2 notes
·
View notes
Photo
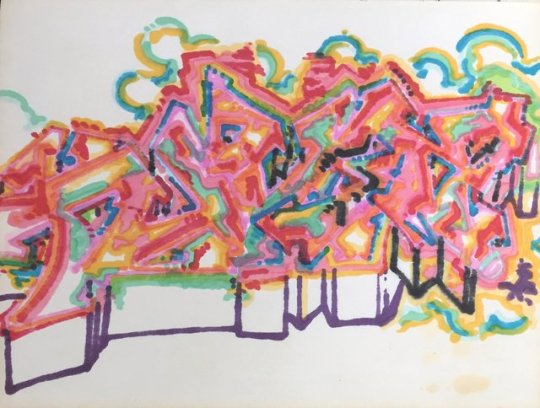
Min one
https://sketchordie.tumblr.com/post/185082065347/min-one
11 notes
·
View notes