#MACHINE LEARNING FOR PREDICTIVE MAINTENANCE
Explore tagged Tumblr posts
Text
Smart Meters: predictive maintenance with condition monitoring
Understanding Condition Monitoring:
Condition monitoring (CM) is a maintenance strategy that predicts machine functioning and safety by combining machine sensor data that measures vibration and other parameters (in real-time) with innovative machine monitoring software. This approach allows plant maintenance technicians to remotely monitor the functioning of each piece of machinery while also providing a comprehensive view of mechanical operations. When a change in machine health is detected, condition monitoring software sends an alert, allowing your maintenance technicians to assess the situation and determine whether corrective action is needed immediately.
Tеchnologiеs usеd in condition monitoring:
Condition monitoring is a key component of predictive maintenance, as it allows you to detect and diagnose faults in your equipment before they lead to failures or downtime. Using various methods and technologies, you can collect and analyze data on your assets' performance, health, and condition, and take proactive actions to optimize their efficiency and reliability.
IoT:
One of the primary driving forces behind condition monitoring is the advancement and proliferation of IoT devices, which allow you to collect and transmit data from your equipment in real time. IoT devices can measure various parameters, including vibration, temperature, pressure, humidity, sound, and more, to provide insights into your assets' operating conditions and potential issues. These IoT devices can be connected to wireless networks, cloud platforms, and edge computing, allowing you to store, process, and access data remotely and securely.
Data analytics and AI:
Data analytics and AI allow you to extract meaningful information and patterns from your data and make better decisions based on it. This helps you perform a variety of tasks, including data cleansing, feature extraction, anomaly detection, fault diagnosis, root cause analysis, and predictive modeling, while also providing you with actionable recommendations and alerts. Furthermore, data analytics and AI can take advantage of machine learning, deep learning, and neural networks, allowing you to learn from your data and continuously improve your models.
Cloud computing:
Cloud computing is fundamentally changing how we approach condition monitoring. Instead of being confined to on-site servers and limited processing power, cloud storage allows vast amounts of data to be securely stored and accessed from anywhere with an internet connection. This flexibility empowers real-time monitoring and analysis, regardless of location. These cloud-based solutions unlock the potential of advanced analytics and artificial intelligence. By integrating data with other sources and platforms in the cloud, businesses can leverage powerful algorithms to gain deeper insights into equipment health and predict potential issues before they become critical failures.
Machine learning:
Machine learning is transforming the way we monitor the condition of machinery. By collecting data from sensors and measuring vibration and temperature, we can analyze this information to forecast potential equipment issues before they lead to expensive breakdowns. This proactive approach allows us to shift from reactive maintenance, where we only fix things after they've broken to preventive maintenance, where we address problems before they escalate. This not only saves money but also ensures that operations run smoothly without unexpected interruptions.
Building a Condition Monitoring Solution with Smart Meters:
Data Acquisition and Preparation:
The first step involves gathering high-frequency energy usage data from smart meters installed throughout your facility. This data should capture information at both the equipment and facility levels. To create a comprehensive data pool for analysis, this smart meter data needs to be seamlessly integrated with existing data sources such as equipment records and historical maintenance logs. Once collected, data quality is ensured by addressing missing values, outliers, and inconsistencies. Preprocessing involves data transformation, normalization, and feature engineering to prepare it for use in predictive analytics models.
For more information click the below link : https://nu10.co/smart-meters-predictive-maintenance-with-condition-monitoring/
#CONDITION MONITORING#CONDITION MONITORING BENEFITS#DATA ANALYTICS#ENERGY AND UTILITY#EQUIPMENT HEALTH#IMPROVED ENERGY EFFICIENCY WITH SMART METERS#IOT.MACHINE HEALTH.MACHINE LEARNING#MACHINE LEARNING FOR PREDICTIVE MAINTENANCE#PREDICTIVE MAINTENANCE#PREVENTATIVE MAINTENANCE#REDUCING UNPLANNED DOWNTIME#SMART METER DATA ANALYTICS#SMART METRES
0 notes
Text
#Predictive Maintenance#Machine Learning#augmented reality#Manufacturing#AI#artificial intelligence#kompanions#industrial AR#Industrial metaverse#3D modeling
2 notes
·
View notes
Text
Will AI and Machine Learning Take Over Civil Engineering Degree?
If you’ve been following the latest trends in civil engineering degree, you might have noticed that Artificial Intelligence (AI) and Machine Learning (ML) are making quite a splash. But what does this mean for traditional civil engineering degrees? Will AI and ML render these programs obsolete, or will they enhance the educational landscape? The Changing Face of Civil Engineering Degree Civil…

View On WordPress
#AI applications in engineering#AI education#AI in civil engineering#automation in construction#civil engineering careers#civil engineering degrees#data analysis in civil engineering#degree#engineering job market#engineering technology#future of civil engineering#Generative Design#infrastructure development#Machine Learning in engineering#Predictive Maintenance#skills for engineers
0 notes
Text
Predictive Maintenance: Strategies for Enhancing Asset Performance and Reducing Costs
Meticulous Research®, a globally recognized market research firm, has recently released a comprehensive report titled “Predictive Maintenance Market by Offering (Software, Hardware), Deployment Mode, Organization Size, Technology (IoT, AI & ML), Application (Oil Analysis, Temperature Monitoring), End-use Industry, and Geography - Global Forecast to 2031.” This extensive study highlights the promising trajectory of the predictive maintenance market, which is expected to reach $79.1 billion by 2031, reflecting a remarkable compound annual growth rate (CAGR) of 30.9% from 2024 to 2031.
Download Sample Report Here @ https://www.meticulousresearch.com/download-sample-report/cp_id=5936?utm_source=article&utm_medium=social&utm_campaign=product&utm_content=29-10-24
Factors Driving Market Growth
The surge in the predictive maintenance market can be attributed to several pivotal factors, including the urgent need to reduce maintenance costs and enhance asset performance. The increasing complexity of infrastructure systems has necessitated the widespread adoption of predictive maintenance solutions. Despite the favorable outlook, the market grapples with significant challenges, particularly related to data privacy and security concerns. These issues pose potential barriers to growth, as organizations must navigate the complexities of compliance and consumer trust.
Another critical aspect of the market is the burgeoning demand for predictive maintenance solutions in healthcare devices and navigation systems. This trend presents valuable growth opportunities for companies operating within this sector. However, a notable challenge remains: the scarcity of skilled professionals capable of managing advanced predictive maintenance technologies. As organizations strive to adopt these sophisticated solutions, the lack of expertise may hinder their progress. Furthermore, emerging trends, such as the integration of digital twins and augmented reality (AR), are reshaping the market, introducing both innovative possibilities and operational challenges.
Market Segmentation Overview
The predictive maintenance market is meticulously segmented to provide a nuanced understanding of its various components. The key segments include:
Offering: Software, hardware, and services
Deployment Mode: Cloud-based and on-premise solutions
Organization Size: Large enterprises versus small and medium-sized enterprises (SMEs)
Technology: IoT, AI, machine learning, cloud connectivity, advanced analytics, and digital twins
Application: Vibration analysis, oil analysis, temperature monitoring, and other applications
End-use Industry: Manufacturing, healthcare, automotive, aerospace, and other sectors
Geography: North America, Europe, Asia-Pacific, Latin America, and the Middle East & Africa
This segmentation framework allows for a comprehensive analysis of market dynamics and growth drivers across various categories.
Discover More About Market Segmentation And Geographic Forecasts By Accessing The Full [PDF] Sample Research Report – https://www.meticulousresearch.com/request-sample-report/cp_id=5936?utm_source=article&utm_medium=social&utm_campaign=product&utm_content=29-10-24
Offering: Software, Hardware, and Services
The segmentation of the predictive maintenance market by offering includes software, hardware, and services. In 2024, the software segment is projected to capture a substantial market share, exceeding 81% of total revenue. This dominance is driven by the increasing necessity for organizations to reduce maintenance costs. Moreover, predictive maintenance software plays a vital role in ensuring compliance by providing essential documentation of maintenance activities and adherence to maintenance schedules.
Conversely, the services segment is expected to witness the highest CAGR during the forecast period. This growth can be attributed to the increasing adoption of predictive maintenance services that analyze equipment data to identify potential issues. As organizations seek to minimize overall maintenance costs, the integration of IoT, AI, and machine learning technologies enables real-time monitoring and proactive maintenance.
Deployment Mode: Cloud-Based vs. On-Premise
The predictive maintenance market is also categorized based on deployment modes, distinguishing between cloud-based and on-premise solutions. In 2024, cloud-based deployments are anticipated to hold a significant market share, exceeding 58%. This trend is largely attributed to the growing preference for cloud solutions that offer flexibility, scalability, and the capability to analyze large volumes of data in real-time. Furthermore, cloud platforms often incorporate advanced analytics features, including machine learning and predictive modeling, enhancing their appeal to organizations seeking comprehensive maintenance solutions.
The cloud-based segment is also expected to register the highest CAGR throughout the forecast period, as more businesses recognize the strategic advantages of cloud technology in predictive maintenance.
Organization Size: Large Enterprises vs. SMEs
Another important segmentation criterion is organization size, which differentiates between large enterprises and small and medium-sized enterprises (SMEs). In 2024, large enterprises are projected to account for the larger market share, exceeding 74%. This dominance is primarily driven by the growing adoption of predictive maintenance as a strategy to mitigate costly unplanned downtime and repairs. Large enterprises leverage predictive maintenance to monitor equipment health, identify performance degradation, and take proactive measures to maintain optimal operating conditions.
In contrast, the small and medium-sized enterprise segment is expected to exhibit the highest CAGR during the forecast period. This growth is fueled by the increasing adoption of predictive maintenance solutions that automate monitoring and analysis of equipment health, thereby alleviating the burden on maintenance personnel. Additionally, predictive maintenance enables SMEs to meet regulatory requirements and enhance operational efficiency, further contributing to the segment's expansion.
Technology: IoT, AI, and Machine Learning
The predictive maintenance market is also segmented by technology, encompassing IoT, AI, machine learning, cloud connectivity, and advanced analytics. In 2024, the IoT segment is anticipated to dominate the market, capturing the largest share. This trend is driven by the widespread adoption of IoT-based predictive maintenance solutions, which facilitate the prediction of equipment failures and improve technician efficiency by providing real-time information on equipment performance.
However, the AI and machine learning segment is expected to achieve the highest CAGR during the forecast period. The growing integration of AI and ML in predictive maintenance contributes to real-time analytics, energy savings, and reduced environmental impact for industrial operations. By leveraging AI and ML algorithms to analyze vast amounts of data from sensors and equipment logs, organizations can identify patterns and trends that drive proactive maintenance strategies.
Have specific research needs? Request a customized research report- https://www.meticulousresearch.com/request-sample-report/cp_id=5936?utm_source=article&utm_medium=social&utm_campaign=product&utm_content=29-10-24
Application: Vibration Analysis and Temperature Monitoring
The applications segment of the predictive maintenance market is categorized into various key areas, including vibration analysis, oil analysis, temperature monitoring, and more. In 2024, the temperature monitoring segment is projected to account for a substantial market share, exceeding 26%. This trend is driven by the increasing adoption of predictive maintenance practices aimed at anticipating equipment failures and facilitating timely notifications for maintenance personnel.
Conversely, the vibration analysis segment is expected to exhibit the highest CAGR during the forecast period. The growing emphasis on predictive maintenance for vibration analysis allows organizations to detect, measure, and analyze vibrations in rotating machinery parts, thus controlling downtime and enhancing product quality.
End-Use Industry: Manufacturing and Healthcare
The predictive maintenance market is further segmented by end-use industry, including manufacturing, healthcare, automotive, and other sectors. In 2024, the manufacturing segment is expected to dominate the market, accounting for over 30% of total revenue. This dominance is attributed to the increasing adoption of predictive maintenance strategies aimed at minimizing costs associated with unscheduled downtime, as well as the growing implementation of Industry 4.0 principles within the manufacturing landscape.
Conversely, the healthcare segment is anticipated to register the highest CAGR during the forecast period. This growth is primarily driven by the expanding utilization of IoT and telematics within healthcare facilities, as well as the increasing reliance on predictive maintenance to gather data on critical medical equipment parameters such as temperature and pressure. Predictive maintenance enables healthcare facility managers to schedule maintenance efficiently, ensuring the continuous operation of vital medical equipment.
Geographic Analysis: North America and Asia-Pacific
From a geographical perspective, the predictive maintenance market is segmented into North America, Europe, Asia-Pacific, Latin America, and the Middle East & Africa. In 2024, North America is projected to maintain its position as the market leader, accounting for over 33% of the overall share. This significant presence can be attributed to the rising demand for predictive maintenance solutions in the healthcare sector, coupled with the growing need to reduce equipment failure, maintenance costs, and downtime.
Conversely, the Asia-Pacific region is expected to exhibit the highest CAGR of over 32% during the forecast period. This growth is fueled by the rapid expansion of SMEs, increasing industrialization, and government initiatives aimed at enhancing operational efficiency. The emergence of Industry 4.0 in key countries, including China, India, and Japan, further propels the demand for predictive maintenance solutions.
Key Players in the Predictive Maintenance Market
The competitive landscape of the predictive maintenance market features several key players who are actively driving innovation and shaping the industry. These prominent organizations include:
International Business Machines Corporation (IBM) (U.S.)
ABB Ltd (Switzerland)
Hitachi, Ltd. (Japan)
Siemens AG (Germany)
Amazon Web Services, Inc. (a subsidiary of Amazon.com, Inc.) (U.S.)
Google LLC (a subsidiary of Alphabet Inc.) (U.S.)
Microsoft Corporation (U.S.)
Emerson Electric Co. (U.S.)
Oracle Corporation (U.S.)
Splunk Inc. (a subsidiary of Cisco Systems, Inc.) (U.S.)
Axiomtek Co., Ltd. (Taiwan)
Presage Insights Pvt Ltd (India)
XMPro Inc. (U.S.)
Faclon Labs Private Limited (India)
SenseGrow Inc. (U.S.)
These key players leverage their technological prowess, extensive industry experience, and innovative approaches to develop state-of-the-art predictive maintenance solutions tailored to meet the evolving needs of their clients.
Conclusion
The predictive maintenance market is on a promising growth trajectory, propelled by advancements in technology and the increasing demand for operational efficiency across various sectors. While challenges related to data privacy and workforce skills persist, the opportunities for expansion, particularly in emerging markets and industries, are substantial. With ongoing integration of advanced technologies such as IoT, AI, and machine learning, the predictive maintenance market is set to evolve, offering innovative solutions that address the diverse needs of businesses globally.
This landscape presents an opportunity for stakeholders to invest in predictive maintenance strategies that not only reduce operational costs but also drive sustainable growth and enhanced competitiveness in an increasingly dynamic market. As organizations continue to embrace the digital transformation journey, the role of predictive maintenance will become increasingly critical in ensuring asset reliability, performance, and longevity.
Read Full Report :- https://www.meticulousresearch.com/product/predictive-maintenance-market-5936
Contact Us: Meticulous Research® Email- [email protected] Contact Sales- +1-646-781-8004 Connect with us on LinkedIn- https://www.linkedin.com/company/meticulous-research
#Predictive Maintenance Market#Vibration Analysis#Oil Analysis#Acoustics Monitoring#Motor Circuit Analysis#Temperature Monitoring#Manufacturing#Oil & Gas#AI and Machine Learning#IoT#Data Acquisition Systems#Sensors
0 notes
Text
AI Revolution in Manufacturing: Key Benefits and Use Cases | CostItRight
Discover how AI is transforming the manufacturing industry with CostItRight’s detailed exploration. Our article delves into the pivotal role of machine learning algorithms and predictive maintenance in revolutionizing manufacturing processes. Learn about the specific benefits and real-world applications of AI technologies that are setting new standards in manufacturing efficiency and innovation.
Introduction to AI in Manufacturing: Understand the fundamental ways in which artificial intelligence is integrated into the manufacturing sector, enhancing capabilities and transforming traditional operations.
Machine Learning Algorithms: Dive into the specifics of how machine learning algorithms are applied within manufacturing. Explore their role in optimizing production lines, improving quality control, and reducing operational costs through enhanced data analysis.
Predictive Maintenance: Uncover the critical advantages of predictive maintenance enabled by AI. Discuss how this application predicts equipment failures before they occur, significantly reducing downtime and maintenance costs.
Enhanced Automation: Learn about the heightened levels of automation that AI brings to manufacturing. From robotic assembly lines to automated quality checks, AI technologies are making manufacturing processes faster, safer, and more cost-effective.
Supply Chain Optimization: Examine how AI contributes to streamlining supply chain management. AI systems analyze vast amounts of data to forecast supply needs, optimize logistics, and mitigate risks associated with supply chain volatility.
Customization and Personalization: Highlight how AI enables the mass customization of products by adapting manufacturing processes quickly to meet specific customer demands without sacrificing efficiency or increasing costs.
Energy Efficiency: Discuss the role of AI in enhancing energy efficiency within manufacturing plants. AI’s ability to monitor and adjust energy use can lead to significant reductions in energy costs and help companies move towards sustainability.
Real-World Use Cases: Provide real-world examples and case studies that illustrate the successful implementation of AI in manufacturing. These examples should show tangible benefits and the transformative impact of AI applications.
Challenges and Considerations: While AI presents numerous benefits, it's also essential to discuss the challenges, such as the initial cost of integration, the need for skilled personnel, and potential job displacement concerns.
Looking Ahead: Speculate on future trends and advancements in AI within the manufacturing sector. Consider how upcoming innovations could further enhance production efficiency, customization capabilities, and cost management.
Explore the comprehensive insights provided in our guide on CostItRight to understand how your manufacturing processes can benefit from the AI revolution, ensuring your operations remain at the cutting edge of technology and efficiency.
0 notes
Text
Why are cement plants the perfect candidates for Predictive Maintenance?

here are three facts about cement plants that are universally true:
The average machine age in a cement plant is at least 30-40 years.
Finding the right expertise to maintain them consistently is challenging.
Every machine – big or small – has the power to bring the whole process to a complete standstill.
These three facts establish that proactive machine maintenance in cement plants is critical to remain profitable and scale efficiently. As demand for cement grows hand-in-hand with blooming infrastructure, GDP growth & exports, the pressure on cement plants to produce continuous, high-quality output also increases proportionately.
This article discusses Predictive Maintenance, a new age approach for plant maintenance, and why an IoT-led Predictive Maintenance approach can solve most of your maintenance worries for your cement plants.
Introduction to Predictive Maintenance
Predictive Maintenance in process manufacturing plants such as the cement industry can identify deviations in machine health at the nascent stage before they escalate into full-blown problems that may result in unplanned downtime.
But that is putting it very mildly. If you look at the daunting results of a neglected cement plant, violent accidents and sky-high repair and replacement costs, while the downtime continues indefinitely, are two of many consequences of a system that is not armed with the intel that Predictive Maintenance can provide.
Here’s a simple example that explains the difference between the health of a machine that uses Predictive Maintenance and one that doesn’t – exam preparation.
An intelligent student looks at exam preparation as a daily occurrence, checking in regularly to maintain good grades and maximize performance at the end of the year. A weaker one only thinks about the exam preparation as a reaction to the possibility of failing and only begins to take action when things have started to go south.
Condition Monitoring & Predictive Maintenance operate how a good student goes about exam prep. While Condition Monitoring checks in with the machine’s health periodically, Predictive Maintenance sees that the machine is continuously monitored and will keep functioning like it is supposed to for as long as possible.
To Know more about cement plants the perfect candidates for Predictive Maintenance: https://www.infinite-uptime.com/why-are-cement-plants-the-perfect-candidates-for-predictive-maintenance/
#Predictive Maintenance#ai predictive analytics#industrialautomation#predictivemaintenance#machine learning
0 notes
Text
The Role Of Machine Learning In Predictive Maintenance
A machinery or equipment failure can lead to increased costs, production delays, and downtimes. This further can impact productivity and efficiency as well.
Therefore, before such failures occur, it is important to foresee equipment issues and perform maintenance exactly when needed. This helps maintain productivity and leads to cost savings. By adopting predictive maintenance based on machine learning, manufacturers can reduce downtime and repair time.
Predictive maintenance with machine learning can yield substantial benefits such as minimizing the time for maintenance schedules, cutting down maintenance costs, and increasing the runtime.
In this blog post, we’ll be exploring everything a manufacturer should know about predictive maintenance with the help of machine learning models, its applications, and the future of predictive maintenance. Read More!!!
#machine learning#Predictive Maintenance#Anomalies#Asset performance#Automotive#cost savings#Data analytics#Data collection#Downtime reduction#Equipment failures#Equipment reliability#Healthcare#Historical data#IoT devices#Maintenance schedules
0 notes
Text
Customized IoT Solutions Smartly Built For Your Every Need
IoT Works provides customised IoT solutions to optimise business operations across diverse industries. With over 30 years of experience, we offer cutting-edge IoT devices, including sensors, GPS asset tracking, and cold chain monitoring. Our services enhance asset management, predictive maintenance, and remote monitoring. We excel in creating personalised solutions that drive efficiency, connectivity, and sustainable growth. Our innovative IoT applications span building monitoring, fleet management, smart farming, and more. Committed to excellence, IoT Works ensures your business stays ahead with advanced technology and real-time data insights. Contact us for a free IoT consultation and transform your business operations.
1 note
·
View note
Text
Explore the power of AI in manufacturing with our easy-to-follow guide. Learn how to train Convolutional Neural Networks (CNNs) seamlessly using our step-by-step flowchart. From preparing data to refining models, grasp every stage of building effective image classifiers. Whether you're new to AI or an experienced user, our resource simplifies the process. Stay updated with Softlabs Group for more AI insights and developments.
0 notes
Text
Shoreline AI | Revolutionary APM & Methane Leak Detection
0 notes
Text
Unlocking the Power of Predictive Maintenance with AI and ML
Revolutionizing Reliability: How Predictive Maintenance Platforms Powered by AI and ML are Transforming Industries
In today's industrial landscape, downtime can be a costly nightmare. Unexpected equipment failures can grind operations to a halt, leading to lost production, delayed shipments, and frustrated customers. The traditional approach of reactive maintenance, where equipment is repaired only after it breaks down, is becoming increasingly unsustainable. This is where Predictive Maintenance Platforms (PMPs) powered by AI and ML development companies come in, offering a proactive and data-driven solution to ensure optimal equipment performance and minimize downtime.
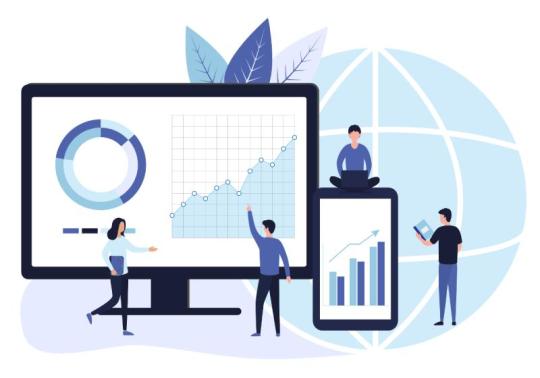
What is a Predictive Maintenance Platform (PMP)?
A PMP is a software application that leverages the power of AI and Machine Learning (ML) to analyze sensor data collected from industrial equipment. This data can include vibration levels, temperature readings, energy consumption, and other key metrics that provide insights into the health and performance of the equipment.
Here's how AI and ML development companies empower PMPs:
Machine Learning Algorithms: AI and ML development companies create sophisticated algorithms that can identify subtle patterns and anomalies in sensor data. These algorithms can learn from historical data and predict potential equipment failures before they occur, allowing for proactive maintenance interventions.
Data Analytics and Visualization: The platform provides advanced data analytics and visualization tools that help users understand equipment health, identify trends, and prioritize maintenance tasks. This allows for a data-driven approach to maintenance, ensuring resources are directed towards equipment most at risk of failure.
Benefits of Implementing a Predictive Maintenance Platform:
Reduced Downtime: By proactively identifying and addressing potential failures, PMPs significantly reduce unplanned downtime, leading to increased operational efficiency and production output.
Lower Maintenance Costs: Predictive maintenance allows for targeted maintenance interventions, minimizing the need for unnecessary repairs and replacements. This translates to significant cost savings over time.
Improved Equipment Lifespan: By identifying and addressing potential issues early on, PMPs help extend the lifespan of valuable equipment, reducing the need for expensive replacements.
Enhanced Safety: Predictive maintenance helps prevent catastrophic equipment failures that could lead to safety hazards for workers.
Industries Leading the Predictive Maintenance Revolution:
PMPs are finding application across various industries, including:
Manufacturing: Predictive maintenance is crucial for ensuring continuous operation in production lines, minimizing production delays and maximizing output.
Oil and Gas: PMPs play a vital role in monitoring the health of critical infrastructure such as pipelines and refineries, preventing environmental disasters and ensuring operational safety.
Power Generation: Predictive maintenance helps prevent unexpected outages in power plants, ensuring a reliable supply of electricity.
Transportation: PMPs are vital for airlines, railroads, and shipping companies to ensure the safety and reliability of their vehicles by predicting potential breakdowns and scheduling maintenance accordingly.
The Future of Predictive Maintenance:
As AI and ML development companies continue to refine their algorithms and data analysis capabilities, PMPs will become even more sophisticated and widely adopted. We can expect to see:
Integration with IoT: PMPs will seamlessly integrate with the Internet of Things (IoT) for real-time data collection and analysis, enabling even more accurate predictions.
Advanced Analytics: The incorporation of advanced analytics techniques, such as deep learning, will allow for a deeper understanding of equipment health and the identification of complex failure patterns.
Cloud-Based Solutions: Cloud-based PMPs will offer greater scalability, accessibility, and cost-effectiveness for companies of all sizes.
Conclusion:
Predictive maintenance platforms powered by AI and ML development companies represent a transformative shift in the way industries manage their assets. By proactively addressing equipment health, PMPs offer significant benefits in terms of reduced downtime, lower maintenance costs, and improved equipment lifespans. As technology continues to evolve, PMPs will play an even more critical role in ensuring the efficient, reliable, and safe operation of industrial processes across the globe.
0 notes
Text
Machine Learning Algorithms For Predictive Maintenance
Machine Learning Algorithms For Predictive Maintenance – Optimizing operational efficiency is critical for companies looking to stay competitive in today’s fast-paced industrial environment. With its proactive approach to equipment maintenance that minimizes downtime and lowers costs, predictive maintenance (PdM) has become a game-changer. This article clearly explains the benefits of using…

View On WordPress
#Machine Learning Algorithms#Machine Learning Algorithms For Predictive Maintenance#Predictive Maintenance#What Is Predictive Maintenance?
0 notes
Text
Predictive Maintenance Precision: Insights from AI
AI-Based Predictive Maintenance
AI-based predictive maintenance is revolutionizing industries by leveraging artificial intelligence to forecast equipment failures before they occur, thereby minimizing downtime, reducing costs, and enhancing operational efficiency. In this article, we delve into the significance, workings, benefits, challenges, and future prospects of AI-based predictive maintenance.
Discover how AI-based predictive maintenance is revolutionizing industries by leveraging artificial intelligence to forecast equipment failures and optimize maintenance strategies.
1. Introduction to AI-Based Predictive Maintenance
Predictive maintenance involves the use of data and analytics to predict when equipment failure is likely to occur, allowing for timely maintenance and preventing unexpected breakdowns. With the integration of artificial intelligence (AI), predictive maintenance has become more accurate, efficient, and cost-effective.
2. Importance of Predictive Maintenance in Industries
Industries across various sectors rely on machinery and equipment to maintain productivity and meet customer demands. Unexpected equipment failures can lead to costly downtime, reduced output, and compromised safety. Predictive maintenance helps mitigate these risks by enabling proactive maintenance actions based on data-driven insights.
3. Understanding AI in Predictive Maintenance
How AI Revolutionizes Predictive Maintenance
AI algorithms analyze historical data patterns and real-time sensor data to predict equipment failures with high accuracy. These algorithms continually learn and adapt, improving prediction accuracy over time.
Applications of AI in Predictive Maintenance
AI is applied in various predictive maintenance tasks, including anomaly detection, fault diagnosis, remaining useful life prediction, and scheduling maintenance activities based on equipment condition and workload.
4. Key Components of AI-Based Predictive Maintenance Systems
Data Collection and Monitoring
Data from sensors, IoT devices, and equipment logs are collected and monitored in real-time to identify abnormalities and patterns indicative of potential failures.
Machine Learning Algorithms
Machine learning algorithms process the collected data to identify correlations, trends, and anomalies, enabling predictive modeling and decision-making.
Predictive Analytics
Predictive analytics techniques, such as regression analysis and time-series forecasting, are used to predict equipment failures and prescribe optimal maintenance actions.
5. Benefits of AI-Based Predictive Maintenance
Cost Savings
By preventing unplanned downtime and minimizing maintenance costs, AI-based predictive maintenance helps organizations save money and optimize resource allocation.
Increased Equipment Reliability
Regular maintenance based on predictive insights enhances equipment reliability, prolongs asset lifespan, and improves overall operational efficiency.
Enhanced Safety
Proactive maintenance reduces the risk of equipment failures and associated safety hazards, creating a safer work environment for employees.
6. Challenges and Limitations of AI in Predictive Maintenance
Data Quality and Availability
The effectiveness of AI-based predictive maintenance relies on the quality and availability of data. Incomplete or inaccurate data can lead to unreliable predictions and false alarms.
Implementation Costs
Initial investments in AI infrastructure, sensors, and data management systems may pose financial challenges for organizations, especially small and medium-sized enterprises.
Integration with Existing Systems
Integrating AI-based predictive maintenance systems with existing equipment and enterprise software requires careful planning and coordination to ensure compatibility and seamless operation.
7. Case Studies Highlighting Successful AI-Based Predictive Maintenance Implementations
Several industries, including manufacturing, healthcare, transportation, and energy, have successfully implemented AI-based predictive maintenance solutions, resulting in improved asset performance, reduced maintenance costs, and increased operational efficiency.
8. Future Trends and Innovations in AI-Based Predictive Maintenance
The future of AI-based predictive maintenance holds exciting possibilities, including advancements in predictive algorithms, integration with emerging technologies like edge computing and 5G, and the development of predictive maintenance-as-a-service offerings.
9. Conclusion
In conclusion, AI-based predictive maintenance offers a proactive approach to equipment maintenance, enabling organizations to optimize asset performance, reduce downtime, and enhance operational efficiency. While challenges exist, the benefits of AI in predictive maintenance far outweigh the costs, paving the way for a more reliable and sustainable future.
FAQs
What industries benefit most from AI-based predictive maintenance?
How does AI improve the accuracy of predictive maintenance?
What are the primary challenges in implementing AI-based predictive maintenance?
Can small businesses afford AI-based predictive maintenance solutions?
What role does data quality play in the effectiveness of predictive maintenance systems?
#AI-based maintenance#Predictive analytics#Machine learning in maintenance#Equipment reliability#Predictive maintenance benefits#Industrial automation#Data-driven maintenance#Proactive maintenance strategies#Predictive maintenance implementation#IoT sensors in maintenance
0 notes
Text
#AI#Machine Learning#Industrial Applications#Predictive Maintenance#Quality Control#Robotics#Automation#Supply Chain Optimization#Energy Management#Autonomous Manufacturing#Hyper-Personalization#Cognitive Assistants#Collaborative Robotics#Sustainability Optimization
0 notes
Text
According to a recent report by Meticulous Research®, the predictive maintenance market is expected to reach $79.1 billion by 2031, growing at a compound annual growth rate (CAGR) of 30.9% from 2024 to 2031. This rapid expansion is driven by the increasing need to reduce maintenance costs, enhance asset performance, and the rising adoption of predictive maintenance in complex infrastructure systems. The integration of predictive maintenance into healthcare devices and navigation systems presents new growth opportunities, although challenges like data privacy concerns and the shortage of skilled workers hinder market growth. Key market trends include the use of digital twins and augmented reality (AR), with the market being segmented by offering (software, hardware, services), deployment mode (cloud-based and on-premise), organization size, technology (IoT, AI, cloud connectivity, and advanced analytics), application (vibration analysis, oil analysis, temperature monitoring), and end-use industry (manufacturing, energy, automotive, healthcare, and others). The report also evaluates regional and country-level market dynamics and competitors.
#Predictive Maintenance Market#Vibration Analysis#Oil Analysis#Acoustics Monitoring#Motor Circuit Analysis#Temperature Monitoring#Manufacturing#Oil & Gas#AI and Machine Learning#IoT#Data Acquisition Systems#Sensors
0 notes
Text
AI Revolution in Manufacturing: Benefits and Use Cases | Cost It Right
Discover how AI is transforming the manufacturing industry with Cost It Right. Dive into the benefits of integrating machine learning algorithms and predictive maintenance into your operations. Learn about practical AI applications that enhance efficiency, reduce downtime, and drive innovation.
0 notes