#LoRa technology
Explore tagged Tumblr posts
Text
Bluetooth Beacon
The Bluetooth Beacon constantly advertises iBeacon messages according to settings. It has two built-in high-capacity button cells. The maximum battery life is approximately 5 years with a advertise interval @500ms.
The beacon is mainly used for indoor tracking and asset tracking. It can also be attached to assets and therefore used for asset tracking with the help of our Bluetooth gateways.
For More:
#Lansitec#LoRa#IoT#Bluetooth#BluetoothBeacon#AssetTracking#Technology#Beacon#IndoorTracking#Wireless
2 notes
·
View notes
Text
USB to LoRa Dongle: A Solution for Wireless Communications Device
In the remote sensor networks or any other IoT projects which are used to communicate over large distances with minimal power consumption, USB to LoRa Dongle can be a game changer device. The dongle offers an affordable, efficient and powerful solution for establishing seamless communication. This is a powerful and versatile device that acts as a bridge between your computer or microcontroller and LoRa based wireless.
The dongle comes with SX1268 next generation LoRa technology and is able to communicate up to 5km distance with license free frequency bands(433MHz/868MHz/915MHz). The dongle can connect Raspberry Pi, LoRa and any other microcontroller. The features and specifications of the dongle is given below:
Features and Specifications:
Communication: This module is enabled to communicate over long distances up to 5km range.
Frequency Support: Supports license free ISM frequency bands 433MHz, 868MHz and 915MHz with these features you can use it in different regions across the world.
Transmission Power: Comes with maximum transmission power of 160mW that ensures your data can be transmitted over long distances and it has a multi-level adjustable setting that helps you to optimize the device for your specific needs.
Next Generation LoRa technology: Comes with new generation LoRa technology based on SX1268 chip. Makes it highly reliable for applications. The air data rates for this module range from 0.3Kbps~ 62.5kbps. This range provides a good balance between communication range and data rate, allowing you to choose the best configuration for your specific needs.
Power Supply: can operate with a wide range of power inputs, supports 2.1V - 5.5V or 3.3V~5.5V power supply for the best performance.
Low Power Consumption: This device is energy efficient. It reduced 40% power consumption and 35% power consumption for hardware and software respectively.
Industrial Grade Durability: The industrial grade standard of the dongle ensures it operates in different temperatures from -40°C to 85°C for a long time.
Development Friendly Features: Dongle supports IPEX and stamp holes. This feature provides flexibility for developers and engineers looking to customize the dongle for specialized projects.
Easy Integration With Existing System: Can connect to any computer, Raspberry Pi, Rock, Beagle Bone or other microcontroller to integrate easily with your existing system.
Applications: USB to LoRa dongle can be used in various applications that require long range wireless connectivity, low power consumption and many IoT applications.
IoT Applications
Smart Home Automation
Asset Tracking
Emergency Services
Remote Monitoring
The USB to LoRa by SB Components is a combination of long-range communication, low power consumption, software adjustable transmission power and flexible power supply options. With these great features it provides a reliable and adjustable solution for various applications.
Whether you are building IoT networks, remote sensing systems, or smart agriculture solutions, the USB to LoRa device ensures you can communicate over long distances, reduce energy costs, and deploy solutions that are both reliable and efficient. With its industrial-grade durability, low power consumption, and development-friendly features, the USB to LoRa device is truly a game-changer in the world of wireless communication.
#usb dongle#lora#lora products#wireless#wireless connectivity#raspberry pi#rock#innovation#technology#electronics#iot#iot applications#projects#microcontrollers
0 notes
Text
What Is Fine-Tuning? And Its Methods For Best AI Performance
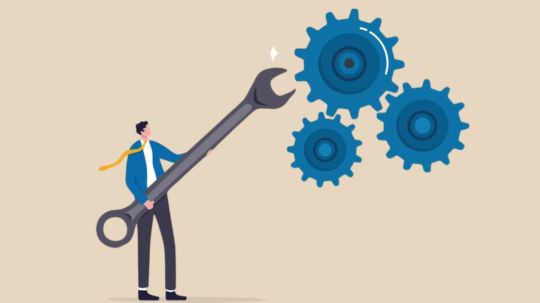
What Is Fine-Tuning?
In machine learning, fine-tuning is the act of modifying a learned model for particular tasks or use cases. It is now a standard deep learning method, especially for developing foundation models for generative artificial intelligence.
How does Fine-Tuning work?
When fine-tuning, a pre-trained model’s weights are used as a basis for further training on a smaller dataset of instances that more closely match the particular tasks and use cases the model will be applied to. Although supervised learning is usually included, it may also incorporate semi-supervised, self-supervised, or reinforcement learning.
The datasets that are utilized to fine-tune the pre-trained model communicate the particular domain knowledge, style, tasks, or use cases that are being adjusted. As an illustration:
An Large Language Model that has already been trained on general language might be refined for coding using a fresh dataset that has pertinent programming queries and sample code for each one.
With more labeled training samples, an image classification model that has been trained to recognize certain bird species may be trained to identify new species.
By using example texts that reflect a certain writing style, self-supervised learning may teach an LLM how to write in that manner.
When the situation necessitates supervised learning but there are few appropriate labeled instances, semi-supervised learning a type of machine learning that combines both labeled and unlabeled data is beneficial. For both computer vision and NLP tasks, semi-supervised fine-tuning has shown promising results and eases the difficulty of obtaining a sufficient quantity of labeled data.
Fine-Tuning Techniques
The weights of the whole network can be updated by fine-tuning, however this isn’t usually the case due to practical considerations. Other fine-tuning techniques that update just a subset of the model parameters are widely available and are often referred to as parameter-efficient fine-tuning (PEFT). Later in this part, it will discuss PEFT approaches, which help minimize catastrophic forgetting (the phenomena where fine-tuning results in the loss or instability of the model’s essential information) and computing demands, typically without causing significant performance sacrifices.
Achieving optimal model performance frequently necessitates multiple iterations of training strategies and setups, adjusting datasets and hyperparameters like batch size, learning rate, and regularization terms until a satisfactory outcome per whichever metrics are most relevant to your use case has been reached. This is because there are many different fine-tuning techniques and numerous variables that come with them.
Parameter Efficient Fine-Tuning (PEFT)
Full fine-tuning, like pre-training, is computationally intensive. It is typically too expensive and impracticable for contemporary deep learning models with hundreds of millions or billions of parameters.
Parameter efficient fine-tuning (PEFT) uses many ways to decrease the number of trainable parameters needed to adapt a large pre-trained model to downstream applications. PEFT greatly reduces computing and memory resources required to fine-tune a model. In NLP applications, PEFT approaches are more stable than complete fine-tuning methods.
Partial tweaks
Partial fine-tuning, also known as selective fine-tuning, updates just the pre-trained parameters most important to model performance on downstream tasks to decrease computing costs. The remaining settings are “frozen,” preventing changes.
The most intuitive partial fine-tuning method updates just the neural network’s outer layers. In most model architectures, the inner layers (closest to the input layer) capture only broad, generic features. For example, in a CNN used for image classification, early layers discern edges and textures, and each subsequent layer discerns finer features until final classification is predicted.
The more similar the new job (for which the model is being fine-tuned) is to the original task, the more valuable the inner layers’ pre-trained weights will be for it, requiring fewer layers to be updated.
Other partial fine-tuning strategies include changing just the layer-wide bias terms of the model, not the node weights. and ��sparse” fine-tuning that updates just a portion of model weights.
Additive tweaking
Additive approaches add layers or parameters to a pre-trained model, freeze the weights, and train just those additional components. This method maintains model stability by preserving pre-trained weights.
This may increase training time, but it decreases GPU memory needs since there are fewer gradients and optimization states to store: Lialin, et al. found that training all model parameters uses 12–20 times more GPU memory than model weights. Quantizing the frozen model weights reduces model parameter accuracy, akin to decreasing an audio file’s bitrate, conserving more memory.
Additive approaches include quick tweaking. It’s comparable to prompt engineering, which involves customizing “hard prompts” human-written prompts in plain language to direct the model toward the intended output, for as by selecting a tone or supplying examples for few-shot learning. AI-authored soft prompts are concatenated to the user’s hard prompt in prompt tweaking. Prompt tuning trains the soft prompt instead of the model by freezing model weights. Fast, efficient tuning lets models switch jobs more readily, but interpretability suffers.
Adapters
In another subclass of additive fine-tuning, adaptor modules new, task-specific layers added to the neural network are trained instead of the frozen model weights. The original article assessed outcomes on the BERT masked language model and found that adapters performed as well as complete fine-tuning with 3.6% less parameters.
Reparameterization
Low-rank transformation of high-dimensional matrices (such a transformer model’s large matrix of pre-trained model weights) is used in parameterization-based approaches like LoRA. To reflect the low-dimensional structure of model weights, these low-rank representations exclude unimportant higher-dimensional information, drastically lowering trainable parameters. This greatly accelerates fine-tuning and minimizes model update memory.
LoRA optimizes a delta weight matrix injected into the model instead of the matrix of model weights. The weight update matrix is represented as two smaller (lower rank) matrices, lowering the number of parameters to update, speeding up fine-tuning, and reducing model update memory. Pre-trained model weights freeze.
Since LoRA optimizes and stores the delta between pre-trained weights and fine-tuned weights, task-specific LoRAs can be “swapped in” to adapt the pre-trained model whose parameters remain unchanged to a given use case.
QLoRA quantizes the transformer model before LoRA to minimize computing complexity.
Common fine-tuning use cases
Fine-tuning may customize, augment, or extend the model to new activities and domains.
Customizing style: Models may be customized to represent a brand’s tone by using intricate behavioral patterns and unique graphic styles or by starting each discussion with a pleasant greeting.
Specialization: LLMs may use their broad language skills to specialized assignments. Llama 2 models from Meta include basic foundation models, chatbot-tuned variations (Llama-2-chat), and code-tuned variants.
Adding domain-specific knowledge: LLMs are pre-trained on vast data sets but not omniscient. In legal, financial, and medical environments, where specialized, esoteric terminology may not have been well represented in pre-training, using extra training samples may help the base model.
Few-shot learning: Models with high generalist knowledge may be fine-tuned for more specialized categorization texts using few samples.
Addressing edge cases: Your model may need to handle circumstances not addressed in pre-training. Using annotated samples to fine-tune a model helps guarantee such scenarios are handled properly.
Your organization may have a proprietary data pipeline relevant to your use case. No training is needed to add this information into the model via fine-tuning.
Fine-Tuning Large Language Models(LLM)
A crucial step in the LLM development cycle is fine-tuning, which enables the basic foundation models’ linguistic capabilities to be modified for a range of applications, including coding, chatbots, and other creative and technical fields.
Using a vast corpus of unlabeled data, self-supervised learning is used to pre-train LLMs. Autoregressive language models are trained to predict the next word or words in a sequence until it is finished. Examples of these models include OpenAI’s GPT, Google’s Gemini, and Meta’s Llama models. Pre-training involves giving models a sample sentence’s beginning from the training data and asking them to forecast each word in the sequence until the sample’s finish. The real word that follows in the original example phrase acts as the ground truth for each forecast.
Although this pre-training produces strong text production skills, it does not provide a true grasp of the intent of the user. Fundamentally, autoregressive LLMs only add text to a prompt rather than responding to it. A pre-trained LLM (that has not been refined) only predicts, in a grammatically coherent manner, what may be the following word(s) in a given sequence that is launched by the prompt, without particularly explicit direction in the form of prompt engineering.
In response to the question, “Teach me how to make a resume,” an LLM would say, “using Microsoft Word.” Although it is an acceptable approach to finish the phrase, it does not support the user’s objective. Due to pertinent information in its pre-training corpus, the model may already possess a significant amount of knowledge about creating resumes; however, this knowledge may not be accessible without further refinement.
Thus, the process of fine-tuning foundation models is essential to making them entirely appropriate for real-world application, as well as to customizing them to your or your company’s distinct tone and use cases.
Read more on Govindhtech.com
#finetuning#AI#artificalintelligence#llm#Additivetweaking#Partialtweaks#lora#govindhtech#news#technology#technologies#TechNews#technologynews#technologytrends
0 notes
Text
Perfect Breast SD1.5 Lora
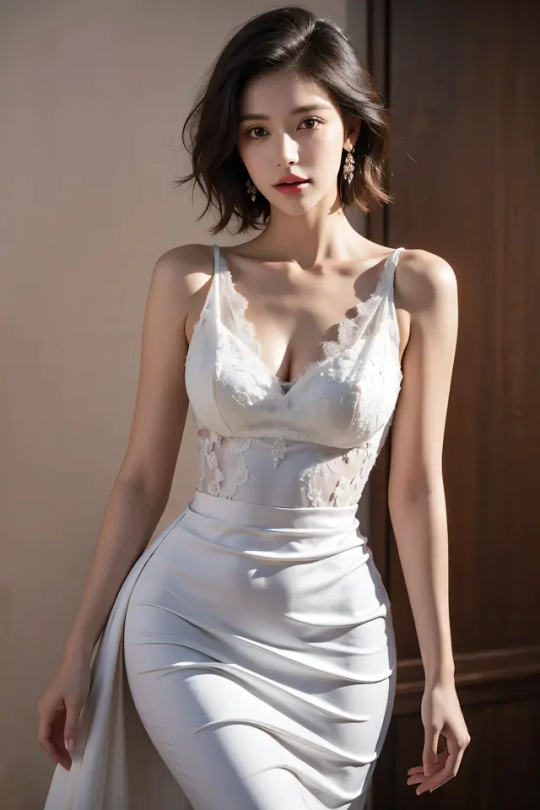
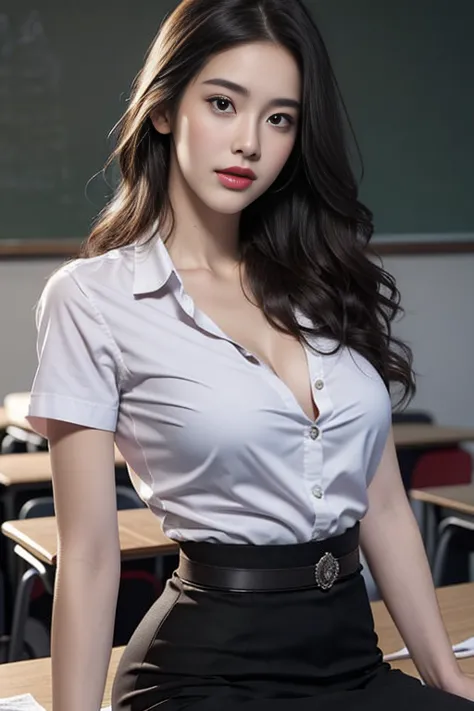
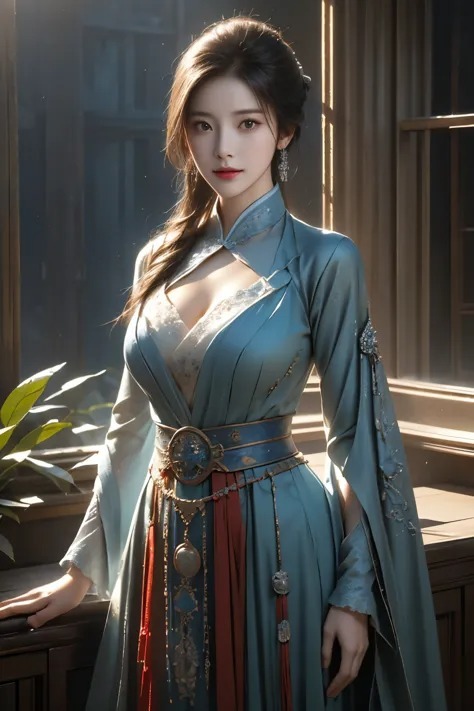
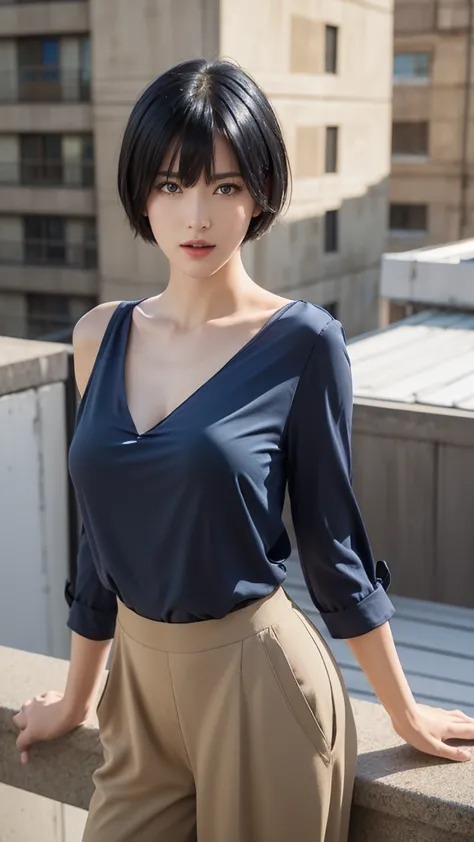
Perfect Breast SD1.5 Lora
My SeaArts AI Profiles
#artwork#digital art#digital illustration#digital painting#portrait#stable diffusion#ai#ai generated#ai art#ai artwork#artificial intelligence#illustration#art#lora#ai image#ai art generator#ai art gallery#ai artist#technology
1 note
·
View note
Text
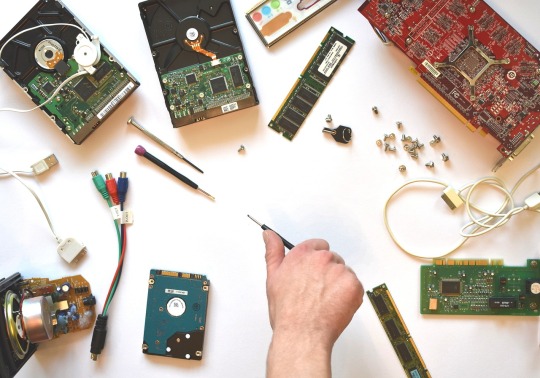
These days, some development boards come with an absolutely astounding variety of hardware. The EESP32, a tiny LoRa board with WiFi, Bluetooth, a transceiver that spans a large portion of the UHF band, and conveniences like OLED displays and an abundance of GPIO, are a perfect example. What about the documentation and firmware, though? Basically, do not say anything at all if you can not say something kind. Better yet, just take a roll. That is not true for every LoRa dev board available, of course, but [Rop] found that to be the case with the Heltec HTIT-WB32LA. This board needed some assistance to get over the line, but it has all the bells and whistles and would be ideal for LoraWAN and Meshtastic applications. Based on his fork of the RadioLib library, which includes a library that significantly lessens wear on the ESP32's flash memory, [Rop] has contributed a fair amount to this end. The library supports all of the hardware on the board, including the pushbutton, display, power management, battery charging, and blinkers, in addition to complete radio support. Many sample applications are included in [Jop], ranging from the minimal requirements to spin up the board to a fully functional spectrum analyzer. It is a wonderful piece of work and a wonderful way to support the LoRa community. And if you want to put one of these modules to work, you’re certainly in the right place. We’ve got everything from LoRaWAN networks to the magic of Meshtastic, so take your pick and get hacking.
1 note
·
View note
Text
youtube
#eteily#technologies#india#rf antenna#rf antenna manufacturers#rf antenna amplifier#4g antenna#rf antenna manufacturers in india#gps antenna#5g antenna#rf antenna price#iot lora antenna#Youtube
0 notes
Text
Exploring LoRa Modules: SX1262, ESP32, and More for Long-Range IoT Communication
In today’s rapidly evolving world of Internet of Things (IoT), the need for wireless communication devices is on the rise. One of the most popular wireless communication technologies used for IoT applications is the LoRa module. In this blog post, we will discuss the LoRa module and its various components such as the SX1262, ESP32, Wi-Fi, Bluetooth modules, and some popular LoRa modules such as the Lorawan-LPS8, Dragino LoRa Bee V1.1, and Hoperf RFM95–98(W).
What is a LoRa Module?
A LoRa module is a low-power, long-range wireless communication module that uses the LoRa modulation technique to enable long-range communication over the air. LoRa stands for “Long Range” and is a proprietary wireless technology that enables long-range communication between two devices without requiring a high-power transmitter or receiver. This technology is designed for low-power, long-range communication with a low data rate, making it ideal for IoT applications.
SX1262:
The SX1262 is a high-performance, sub-GHz transceiver from Semtech that is designed for long-range communication applications. It supports LoRa modulation, as well as FSK, GFSK, and OOK modulation schemes. It operates on the 150–960 MHz frequency range and provides up to 22 dBm of output power. The SX1262 also features a built-in packet handler, CRC, and AES encryption for secure communication. It is an essential component in LoRa modules as it enables long-range communication with low power consumption.
ESP32:
The ESP32 is a low-cost, low-power, Wi-Fi, and Bluetooth-enabled microcontroller from Espressif Systems. It is an ideal platform for IoT applications as it provides a powerful CPU, low power consumption, and built-in connectivity options. The ESP32 supports Wi-Fi 802.11b/g/n, Bluetooth v4.2 and BLE, making it easy to connect to a wide range of devices. It also features an integrated dual-core processor with a clock speed of up to 240 MHz and 520 KB SRAM, making it a powerful platform for IoT applications.
Wi-Fi Module:
The Wi-Fi module is an essential component of LoRa modules as it enables the device to connect to a Wi-Fi network. This connectivity option enables the device to connect to the internet and send and receive data from other devices on the network. The Wi-Fi module can be integrated into the ESP32 or provided as a separate module that can be connected to the device via an interface such as SPI or UART.
Bluetooth Module:
The Bluetooth module is another important component of LoRa modules that enables the device to communicate with other Bluetooth-enabled devices. This connectivity option is ideal for short-range communication between devices such as smartphones, tablets, and other IoT devices. The Bluetooth module can be integrated into the ESP32 or provided as a separate module that can be connected to the device via an interface such as SPI or UART.
Lorawan-LPS8:
The Lorawan-LPS8 is a compact, low-power LoRaWAN module that is designed for IoT applications. It is based on the SX1262 and features a built-in GPS, making it ideal for tracking applications. The module supports LoRaWAN 1.0.2 and provides up to 20 dBm of output power. It also features a built-in antenna, making it easy to integrate into devices. The Lorawan-LPS8 is an excellent choice for applications that require long-range communication with low power consumption and GPS tracking capabilities.
Dragino LoRa Bee V1.1:
The Dragino LoRa Bee V1.1 is another popular LoRa module that is designed for IoT applications. It is based on the Hoperf RFM95–98(W) transceiver and features a built-in ATmega328P microcontroller, making it easy to program and integrate into devices. The module supports LoRa modulation and provides up to 20 dBm of output power. It also features a built-in antenna, making it easy to integrate into devices. The Dragino LoRa Bee V1.1 is an excellent choice for applications that require low-cost, low-power, and long-range communication.
Hoperf RFM95–98(W):
The Hoperf RFM95–98(W) is a high-performance, sub-GHz transceiver that is designed for long-range communication applications. It supports LoRa modulation, as well as FSK, GFSK, and OOK modulation schemes. It operates on the 137–1020 MHz frequency range and provides up to 20 dBm of output power. The Hoperf RFM95–98(W) also features a built-in packet handler, CRC, and AES encryption for secure communication. It is an excellent choice for applications that require long-range communication with low power consumption.
LoRa modules are used in a wide range of IoT applications such as smart cities, smart agriculture, asset tracking, and industrial automation. The low-power, long-range communication capabilities of LoRa modules make them ideal for applications that require remote monitoring and control of devices and sensors.
Conclusion:
In conclusion, LoRa modules are an essential component of IoT applications that require low-power, long-range communication capabilities. The various components of LoRa modules such as the SX1262, ESP32, Wi-Fi, and Bluetooth modules work together to enable long-range communication with low power consumption. Some popular LoRa modules such as the Lorawan-LPS8, Dragino LoRa Bee V1.1, and Hoperf RFM95–98(W) are excellent choices for applications that require low-cost, low-power, and long-range communication. With the increasing demand for IoT applications, LoRa modules will continue to play a significant role in the development of IoT devices and solutions.
0 notes
Text
Amazon opens up the Sidewalk network to developers for free in the US to galvanise the IoT market
On 28th of March 2023, Amazon announced its Sidewalk network project launched in early 2020, now covers 90% of the US population and is open to developers to test, build and create new IoT applications. AWS also announced Sidewalk networks integration with AWS IoT core, enabling Sidewalk-enabled partner devices to easily provision, onboard, and monitor Sidewalk devices at scale. The service is currently available only in the US and developers can use the AWS IoT core for Amazon Sidewalk for free using the AWS Free Tier(details here)
A few companies working with Amazon have also announced the launch of sidewalk-enabled devices which includes:
New Cosmos: Natural gas alarm
Primax: Woody, a smart door lock
Netvox: environmental sensor
Meshify’s: Moisture sensor
Browan: Motion and carbon monoxide sensor
OnAsset Intelligence: Sentinel 200 Asset tracker
Is Amazon Sidewalk just a connectivity network platform?
Amazon Sidewalk communication network is one of the 3 core components of the Sidewalk Device-To-Cloud (DTC) platform which includes:
Sidewalk connectivity network: The Sidewalk network is built and operated by crowdsourcing participating customers who enable the Sidewalk network using consumer bridge devices such as the Amazon Echo and Ring devices.
Sidewalk end-devices: Includes Amazon Ring devices and third-party end-devices that are embedded with Sidewalk radio chipsets running the proprietary network protocol and security certificates required to communicate on the Sidewalk network.
Sidewalk Network Server: The Sidewalk network server verifies and routes the data packets from end devices to the application servers.
The #Sidewalk platform is a Device-to-Cloud platform that is built on a crowdsourced, shared #IoT network that connects Sidewalk end devices with enterprise applications using the #AWS IoT Core. The AWS IoT core also offers developers application-level services and security among other functionalities. The application servers hosting the end devices and applications can either use AWS or third-party cloud application services.
How does Amazon monetise the Sidewalk platform when the Sidewalk network Is free?
By integrating the Sidewalk connectivity network, with the AWS cloud services, Amazon monetizes the data sent by Sidewalk-enabled devices to the AWS cloud. As enterprises use the crowd-sourced network to connect their devices, enterprise customers are charged a small fee every time a Sidewalk device transmits data to the sidewalk network server located in the AWS IoT core. In the near term, the revenues from data alone will be negligible as most of the Sidewalk end devices at the most transmit data a dozen times per day adding to a few Kbs of data per month.
However, over time as sidewalk-enabled devices generate millions of data packets that are routed via the AWS IoT core rules engine, this will help grow its opportunity pipeline for its cloud application services. Amazon Sidewalk will help drive enterprise demand and adoption of its cloud applications especially to manage (sort and query) through large volumes of small data packets sent by end devices. AWS will gain the most value as enterprises start to build applications to leverage its consumer and industrial IoT analytics services, especially advanced analytics services (machine learning and AI services) generating new revenue streams.
Overview of the Amazon Sidewalk network technology
Amazon Sidewalk Network is a Multi-Radio Access Network(#MRAN) wireless connectivity platform used to build a crowd-sourced, community-owned, Neighbourhood Area Network (NAN). The network is powered by mostly consumer Sidewalk bridge devices that use a small fraction of the customer's internet bandwidth (~80Kbps) and share it with other sidewalk customers to create a low-bandwidth, low-power #NAN.
Amazon customers who had already set up a sidewalk gateway device (Echo Or Ring devices) at the time of the launch in early 2020, an over-the-air (#OTA) update automatically connected the edge gateway to the network. Echo or Ring customers have the option to turn off the Sidewalk network feature at any time. This OTA update route to create the Sidewalk network without customers’ prior consent is unlikely to be used in European countries with strict laws protecting customer privacy.
In return for the customer's participation, Amazon manages the network to create a city-wide network which end-users can use for free to connect their third-party sidewalk-enabled devices. The Sidewalk network is available for free to other enterprises to offer consumer and enterprise IoT solutions using Sidewalk-enabled 3rd party devices.
The Sidewalk network is built through the integration of 3 wireless connectivity technologies-
Long Range Communication: Proprietary Sub-Ghz (915 MHz ISM band) LPWAN developed on Chirp spread spectrum (CSS/LoRa) radio PHY. The LoRa Phy technology is also used in the LoRaWAN protocol, the #LPWANtechnology managed by the #LoRa Alliance. The LoRa technology is the bearer technology used for long-range communication.
Medium Range Communication: Sub-Ghz(902-928 MHz) Frequency Shift Keying (FSK) technology. This is a legacy wireless technology used in various smart home security applications such as motions sensors, contact sensors and garage door openers.
Short Range Communication: Frequency Hopping Spread Spectrum Phy(Bluetooth Low Energy or #BLE) operates in the 2.4 GHz ISM band.
Identifying potential growth opportunities for the Sidewalk platform
Anchored on the Sidewalk communication network, the key strength of the Sidewalk platform is the complete integrated device-to-cloud stack. The Sidewalk platform helps enterprises easily onboard Sidewalk devices at scale and the low-cost pricing of AWS cloud services helps solution vendors reduce the overall solution TCO. A glance at the AWS IoT core price calculator will provide an indication of the cost of running devices using the Sidewalk platform.
Growth Prospects for Sidewalk Network: The Sidewalk network is currently available in the US only and covers over 90% of the population coverage. Amazon has made public its plans to expand its network footprint to other regions worldwide in the coming years. However, the success of the Sidewalk network in the US will set the pace of its network footprint growth in other regions.
Since the Sidewalk network relies on Amazon #Echo and #Ring customers, One of the recurring challenges for Amazon will be to ensure Amazon Echo and Ring customers participating in the Sidewalk programme remain high and to reduce churn.
Amazon will also need to ensure customer participation in the Sidewalk network programme remains high to ensure sufficient network redundancies are in place, in case some customers decide to turn off their Sidewalk feature from their devices. The only way Amazon can ensure the Sidewalk network continues to thrive is by ensuring participating Echo and Ring customers see immediate and recurring value in the form of new digital devices and services.
In early 2022, to address potential opportunities in the enterprise IoT market, Amazon Ring announced its #Sidewalk Bridge Pro, a ruggedised outdoor gateway. The weatherproof, enterprise-grade gateway will help Amazon expand its network coverage to public spaces such as parks, city centres, universities, businesses, and other places which are not covered by consumer echo and ring bridge devices. The Sidewalk Bridge Pro is designed to be deployed by businesses and municipalities to complement the residential network coverage.
The Sidewalk Bridge Pro will help expand the Sidewalk network by enabling:
On-demand network availability for enterprises that have an immediate demand for campus networks and are willing to host the network infrastructure.
Will help expand network coverage from existing population coverage to wider regional coverage.
The outdoor gateway devices will also help add necessary network redundancies in residential places where Amazon Ring and Echo customer participation is low or the network requires densification.
The success of the Sidewalk network will depend on the growth in the device and solution ecosystem in the US before it launches the service in other regions. The future regional network roll-out will also depend on regional privacy laws and the residential penetration of Amazon Echo and Ring bridge devices used to build the network. The Sidewalks regional network which includes LoRa and FSK technologies will operate on different sub-GHz frequency bands
Based on Amazon’s Sidewalk bridge shipment data, regional network footprint growth will likely be as follows.
Rest of North America: Canada
Europe: Western Europe followed by Eastern Europe
APAC: India, East Asia and the rest of South East Asia. No presence in China.
Rest of the world: Latin America and MEA.
As the Amazon Sidewalk network operates as a single network, when regional networks outside of the US are rolled out, Amazon can offer a roaming-free global network as long as the device's radio hardware is capable of switching to regional Sub-GHz frequency bands.
Growth Prospects for Amazon Sidewalk Devices and Applications
Amazon's Sidewalk platform strategy is focused on driving device makers’ adoption of the platform to develop sidewalk-enabled devices for enterprises and consumer applications. Amazon has announced its partnership with hardware communication module vendors including #Nordic Semiconductor, #Silicon Labs, #Semtech, Texas Instruments and #Quectel.
As the Sidewalk radio hardware matures, one of the developments to watch out for will be a single SKU global radio hardware that enterprises can embed in their devices. This would help global device OEMs and solution providers drastically reduce their design costs of having to modify their devices to operate in each region. This would also have value for enabling application verticals such as asset tracking that requires seamless connectivity across different regions.
The Sidewalk platform operates on 3 business models to target consumer and enterprise markets:
Business to consumer: Consumer IoT(Smart Home)
Ring acquired by Amazon in 2018 provides consumer home security and smart home devices. The sidewalk network supports Amazon Echo and Ring customers to connect Ring devices including doorbells, outdoor cameras, outdoor lights, and Alarm systems that are beyond the range of the home Wi-Fi network and other Personal Area Networks (PANs). The Sidewalk network's extended range capabilities will also help Ring offer new consumer devices such as pet trackers, asset trackers, outdoor irrigation systems, motion sensors and other connected devices.
Business to Business to Consumer :Consumer IoT(Smart home, asset track and trace)
The sidewalk platform is open to other enterprises that want to develop IoT devices and services for end consumers. Some examples of enterprises using the Sidewalk platform to offer consumer IoT services are as follows.
Tile, a leading consumer track and trace solution provider is one of the early adopters of the Sidewalk platform.
Speciality insurer HSB has also announced its IoT subsidiary, Meshify will connect its proprietary water leak sensors for homeowners through the Amazon Sidewalk platform.
Careband, a wearable device for elderly care, has announced plans to test the Sidewalk platform to offer services to its CareBand customers.
Amazon announced the Fetch pet tracker in early 2020 and has since been developing its cloud-geolocation service to enable more consumer asset track and trace use cases.
Business to Business: Enterprise IoT: (Smart City, Industrial IoT, Asset visibility)
Amazon Sidewalk offers system integrators and solution providers a fully integrated DTC platform to cost-efficiently test, build and operate enterprise IoT applications and services. Utilities and city municipalities deploying smart city applications such as streetlights, water meters, parking sensors, air quality sensors, and other environmental monitoring sensors will benefit from an integrated DTC platform service in reducing its solution time to market and cost. Enterprises benefit from Amazon’s vast partner network which includes software partners, hardware partners, service and consulting partners, system integrators, and distributor partners. The AWS Partner Network has more than 100,000 partners from more than 150 countries, with almost 1/3rd or 30,000 partners based in the US.
In 2022, Amazon announced a collaboration with Arizona state university and Thingy to connect environmental sensors such as sunlight sensors, air quality indicators, and moisture sensors in places like commercial centres, parks, and wilderness areas.
Asset Visibility(Location tracking and condition monitoring): Asset track and trace applications have been a key vertical use case for the sidewalk network. Amazon has been partnering with solution vendors to develop its platform capabilities to offer more seamless and accurate geolocation services. In November 2022, Semtech announced a strategic agreement with AWS to license its LoRa cloud geolocation services to help AWS IoT core offer indoor and outdoor geolocation capability.
On March 28, 2023, Amazon announced its Sidewalk solution partner, #OnAssetintelligence, introduce its Sentinel 200, a Sidewalk-enabled asset tracker for logistics use cases. The Sentinel 200, is a multi-year battery life device with temperature, humidity, light, shock and motion sensors that provide timely and granular data on the location, status and chain-of-custody of shipments and assets moving through the global supply chain.
The sidewalk platform in its current form lacks several key network requirements to succeed in the B2B segment. One of the key network capabilities missing today is the Sidewalk Networks ability to guarantee network quality of service (QoS). However, The Sidewalk platform with its free communication network offers enterprises an opportunity to test proofs-of-concept at a minimal cost and examine the financial feasibility of digital transformation programmes.
Analyst view: “A rising tide lifts all boats…but those unable to change and adapt will fail”
With Sidewalk, Amazon integrates device-to-cloud data management capabilities with a communication network to offer simplicity to the enterprise's digital transformation programs. Device-to-cloud platforms that integrate multi-RAN technologies is a solution to reduce technology complexity. That said, the success of the Sidewalk platform will depend on how quickly the technology's adopted by device makers and enterprises to develop innovative new applications and services.
A cautionary tale: Sigfox, an early pioneer of the low-cost Device to Cloud platform using a proprietary LPWA network technology.
To give credit where it's due, #Sigfox was an early pioneer and strong advocate of a low-cost device-to-cloud (DTC) platform to address the market demand for massive IoT applications. Sigfox believed for enterprises to adopt massive IoT applications which may require deploying 100sK or millions of battery-operated sensor devices, it's crucial to drastically simplify the process and cost of transporting data from the device to the cloud. Sigfox was an advocate of the need for very low network TCO to encourage enterprise digital transformation.
To achieve this, Sigfox built its own DTC platform from the ground up based on its proprietary LPWA network also called Sigfox. Sigfox built and operated its cloud services located in Toulouse, France and only in 2021 had migrated its Sigfox network server to Google IoT core(which also shut down in 2022).
Sigfox launched its device-to-cloud platform services at around $1-$7 per annum per device. The cost varied depending on the volume of end-point connections but included regional sigfox connectivity service and sigfox cloud services. Sigfox as a disruptive technology faced severe criticism for its extremely low device-to-cloud connectivity pricing strategy and lack of financial viability to continue operating several of its large nationwide and citywide networks in Europe and the US respectively.
Sigfox witnessed some early success in smart home, smart metering and asset tracking use cases connecting almost 20 million end-points on its network within a span of 5 years. However, due several factors including financial difficulties due to slow revenue growth and the fallout from the Covid-19 pandemic led to Sigfox filing for bankruptcy in early 2022.
Sigfox assets were subsequently acquired by Unabiz, a Sigfox network operator and system integrator. Unabiz in collaboration with over 60 Sigfox network operators is managing the sigfox technology and developing integrated solutions for enterprise Digital transformation.
Amazon Sidewalk in many ways has a similar business model to Sigfox. Similar to Sigfox, the Amazon sidewalk platform offers very low-cost device-to-cloud connectivity, but with several strengths to ensure longer-term sustainability of the business.
By crowd-sourcing the network, majority of the network infrastructure costs required to build and operate the Sidewalk network (except for the Sidewalk Network server) are borne by its Amazon Echo and Ring customers.
The sidewalk network integrates 3 established network technologies ie. LoRa, FSK and BLE. These technologies are backed by a global ecosystem of hardware and solution vendors.
The sidewalk platform has a strong anchor business use case in its successful smart home business. Amazon Ring uses the Sidewalk network to expand its connectivity coverage for security sensors, lights, cameras and other sensor devices in the periphery of the customer's home and beyond.
The total cost of ownership (TCO) for solutions built on the Sidewalk platform will be a fraction of similar existing solutions using cellular or other LPWAN solutions in the market. The cost of BLE and LoRa connectivity hardware is sub $1 at scale. With free connectivity and the cost of the device to cloud platform service at a few 10s of US$ cents per device per annum, the Sidewalk platform drastically helps reduce the TCO for digital products and services.
AWS partner network consists of over 100,000 partners globally consisting of solution developers, system integrators, hardware partners, consultants and distributors.
The Sidewalk platform is integrated with the AWS IoT core which has over 200 services including machine learning and advanced analytics services.
Will Sidewalk complement or compete with other LoraWAN operators in the US?
There are several existing LoRaWAN service providers in the US including:
Public network operators: #Comcast #MachineQ, Senet and more recently Everynet.
Community networks: LoRiot, Things network and #Helium.
Private networks: #Senet, Comcast, Link Labs, and other enterprise customers.
Senet and Everynet two established LoRaWAN operators have deployed regional network infrastructure and offer it to enterprises in a Network as a Service (NaaS) model. Amazon Sidewalk network will compete with public network service providers but will potentially complement system integrators, solution vendors and other enterprise private networks. As the Sidewalk network footprint grows, it will become difficult for these public network operators to justify their connectivity costs compared to a free network. That said, Amazon Sidewalk which uses LoRa for long-range connectivity could benefit from partnering with LoRaWAN-managed network operators such as Senet and Everynet to densify its city-wide network and expand its geographical network footprint, especially in rural and industrial areas.
Nova Labs, Helium Network is one of the largest community-owned LoRaWAN networks in the US(coverage map-https://explorer.helium.com/iot/cities) and will directly compete with Amazon's Sidewalk Network. The Helium network has nearly a million hotspots running the LoRaWAN and is available in over 163 countries. Helium operates its LoRaWAN by rewarding its hotspot owners with blockchain-powered tokens that are generated for transferring device data to the cloud. Today Helium network has nearly 100 device makers and solution providers using the Helium network to offer IoT solutions.
System integrators such as Comcast MachineQ will benefit from leveraging the Sidewalk network by minimizing its network infrastructure costs and using its resources to develop vertical IoT applications and services for end customers.
Will Amazon Sidewalk complement or compete with Telcos Cellular LPWAN services in the US?
AT&T, #Verizon and #tmobile : Cellular LPWA network (#LTEM & #NBIoT) with nationwide coverage since early 2018. Teclos in the US has been monetizing its NB-IoT and LTE-M networks by offering connectivity services and several end-to-end solutions for enterprise customers. Today, Telcos cellular LPWAN connectivity services vary from $12-$36/device/annum and are calculated based on the end device’s data usage. Telcos have struggled to monetize their LPWA network infrastructure with connectivity service alone and have developed a three-pronged monetization strategy. Telcos in the US today offer enterprises a choice of IoT connectivity and/or software platform and/or end-to-end IoT solutions built through an ecosystem of hardware and solution partners.
Amazon Sidewalk will directly compete with telcos’ LTE-M and NB-IoT networks and their consumer smart home(B2C and B2B2C) offerings with the Sidewalk network. Furthermore, with Sidewalk Bridge Pro, Amazon will also be able to target enterprise IoT(B2B) applications such as smart buildings, smart parking, environmental monitoring etc where telcos offer connectivity services and/or end-to-end solutions.
That said, a heterogeneous network architecture that integrates cellular networks and the Sidewalk network will further augment the Sidewalk platform in addressing a broad range of enterprise IoT solution requirements. Cellular LPWA network's nationwide coverage will also help the sidewalk platform address applications such as outdoor asset-tracking requiring broader geographical coverage, lower latency and frequent data transmissions.
Will Amazon Sidewalk complement or compete with Mesh networking technologies used in smart city applications in the US?
Mesh networking technologies based on IEEE 802.15.4(#Zigbee, #Thread) and #Zwave are two dominant connectivity technologies used in smart home applications. Proprietary LPWAN technologies such as LoRa and Sigfox are more energy efficient and hence have gained market share in the smart home market replacing mesh technologies to connect battery-operated sensor devices. The extended range of capabilities of LPWAN technologies has an advantage over Wi-Fi and mesh network technologies that require additional gateway or bridge devices to extend the network range to basements or the periphery of the homes.
Mesh networking technologies also have a growing market share in several smart city applications such as smart metering, parking and smart street lighting. These applications’ network requirements are currently addressed through the deployment of private networks. The sidewalk network is likely to have little to no impact in these segments until it can guarantee network QoS which is a critical application and business requirement
#amazon#sidewalk#LPWA.#LoRa#IoT#technology#lorawan#devicetocloud#assetvisbility#geolocation#crowdsource#free
0 notes
Text
A big part of the drive for PierMesh is that I think based on Meshtastics ability to run on low power low cost boards that can be managed via a cellphone would make them helpful in disaster situations and particularly what Palestinians are going through right now. I've been trying to reach out to anyone in tech who is Palestinian/helping Palestinians who could spare some time to let me know if the efficacy of using LoRa (long range low power radio networking that can connect over kilometers) is worth trying to get some boards in that are preconfigured and encrypted. I have a lot more details I can provide and if you want to do some preliminary research yourself Meshtastics website is a good place to start:
What PierMesh would provide on top of this in its current state is multilingual support and currently I'm working on end to end encryption but even without that I think this technology could be very useful.
Thank your for your time
191 notes
·
View notes
Text
Container Tracker
The Container Tracker is a device that integrates GNSS (GPS, Beidou, Glonass), Bluetooth and LoRa technology for accurate outdoor and indoor positioning of assets. It has an IP68 enclosure that provides protection against water and dust ingress. It has a large battery capacity that can support up to 180,000 Bluetooth tracking messages over 30,000 GPS coordinates. It supports tracking up to 100 Bluetooth beacons, and also supports UUID change to avoid interference from other devices.
For More:
#lansitec#technology#iot#lora#tracking#lorawan#asset management#Container tracker#Industrial Tracker#GNSS Industrial Tracker#LoRaWAN GNSS Tracker
2 notes
·
View notes
Note
prev ask anon here. please know that i am quite familiar with AI generated art and know how the process works. i was around when the novelAI model leaked and i still generate a fair amount of it both locally on my system and with online services. i am familiar with the infrastructure and technology that goes into providing large scale AI and machine learning services as well as the small scale home PC hardware. please dont assume the worst intentions or least experience of me. as for specific instances the one that comes to mind is GwrrrUwU (now deactivated). they were copying Dross's style *exactly* and got tens of thousands of twitter followers and a lot of patreon subs. you can go look up their images still on a few boorus and they just aren't good quality. you can go look up their page on archive.org and (with a bit of finagling) see they had this in their paid tiers: "I will try to post daily, 1+ or 4+ posts minimum." 4+ images per day, every day. thats a lot. and most of it was slop. another example i just found is Modeus14 (twitter handle) who is blatantly copying __Asura_ (twitter handle). check out their patreon where they supposedly have 369 paid members and have posts with 12+ images coming out multiple times per day. do you really think they are putting in the time and care to make those images come out well? i dont. and in regards to the other reply - the fun part is that you can just insert LoRAs with a specific artists style into the process with *very* little effort. the thing is that these models trained on a specific artists' style all exist already. you can check this out yourself by going to civitai.com and just searching for a specific artist. or go to the "models" section and then click on the "style" tab. not to mention that on the various paid/online imagegen services you can just say the artist name you want to copy and it will use that style. this stuff is real and there truly is a lot of slop out there made by dishonest people. at least acknowledge that slop exists. acknowledge that people are copying styles *exactly* with little effort or care for the end product.
but like you said it’s crap — which, by the way, i have actually said *dozens* of times, most AI art looks shit. but what you’re describing is the same process that leads to mcdonald’s opening outside a local burger place to drive them out of business. like yeah it’s shitty but sorry “lower quality for cheaper faster” is literally just How Capitalism Works. you have a problem with capitalism. like i don’t see how you don’t realise you’re just complaining that a bad imitation of something is more popular than the original because it’s being pumped out cheaper and faster…. that’s how everything under capitalism works. there’s not one industry that doesn’t work this way?
29 notes
·
View notes
Text
RTX 4070 Ti SUPER for Stable Diffusion and AnimateDiff
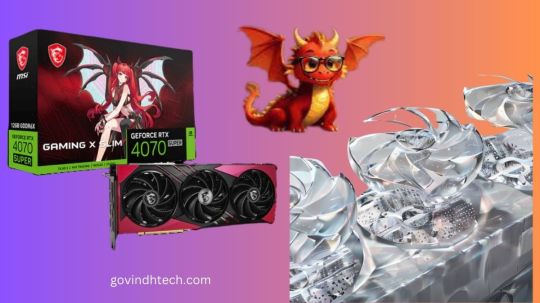
Nvidia GeForce RTX 4070 Ti SUPER 16G GAMING X SLIM
Unbound Gaming
The GAMING SLIM series is a more compact version of the GAMING series that nonetheless has an aggressive appearance and excellent performance capabilities. Those who are designing a gaming system with limited space might choose lighter designs.
Using DLSS 3
With NVIDIA GeForce RTX 4070 Ti SUPER, you can create and play games at a supercharged speed. The very effective NVIDIA Ada Lovelace architecture is used in its construction. Discover new creation possibilities, AI-accelerated speed with DLSS 3, lightning-fast ray tracing, and much more.
Accelerate Memory/Clock Speed
2670 MHz / 21 Gbps
16GB GDDR6X
DisplayPort x 3 (v1.4a) HDMI x 1 (Supports 4K@120Hz HDR, 8K@60Hz HDR, and Variable Refresh Rate as specified in HDMI 2.1a)
TRI-FROZR 3 Thermal Architecture
TORX Fan 5.0: To stabilize and sustain high-pressure airflow, fan blades connected by ring arcs and a fan cowl cooperate.
Copper Baseplate: A copper baseplate absorbs heat from the GPU and memory modules, which is then quickly transmitted to the core pipes.
Core Pipe: A segment of heat pipes shaped like squares distributes heat to the heatsink after making maximum contact with the GPU.
Airflow Control: Don’t worry, this feature directs airflow to the precise location required for optimal cooling.
The Afterburner
With the most well-known and extensively used graphics card overclocking software available, you can take complete control.
The new RTX 4070 Ti SUPER with 16GB VRAM buffer (up from 12GB) gives you a decent middle-ground in NVIDIA’s RTX 40-series portfolio. Following up on MSI’s first testing phase, they chose to assign the specialists a more challenging task: assessing NVIDIA GPUs using AnimateDiff, which generates animated graphics based on text and video inputs.
However, they will compare the new RTX 4070 Ti SUPER against the last-gen champions, the RTX 3080 Ti and 3080.
AnimateDiff image
With the help of the AnimateDiff pipeline, which combines Motion with the Stable Diffusion model, you may create animated GIFs or videos from text or video input.
AI Models with VRAM: How Much Is Needed?
RTX 4070 Ti SUPER
Anything above 12GB should work quite well, even if the RTX 4070 Ti SUPER’s larger VRAM capacity will be helpful for certain jobs. The RTX 4070 Ti SUPER should outperform its predecessors because to its massive 16GB VRAM buffer and unmatched power.
A “minimum VRAM” of 8GB is required for the Stability AI Stable Diffusion XL model. So let’s give it a little more juice and see whether they can significantly accelerate the processes involved in creating images!
RTX 4070 Ti SUPER 16G vs RTX 3080 Ti 12G versus RTX 3080 10G AnimateDiff Benchmarks
They will conduct some tests using Stable Diffusion 1.7 (via WebUI) in addition to jobs utilizing the AnimateDiff pipeline, so you can see how these GPUs respond to varying workloads.
Stable Diffusion 1.7 + ControlNet*2 + LoRA
The first test involves creating images using a LoRA and two ControlNets. For some reason, they find that the RTX 4080 struggles in this test compared to the RTX 4070 Ti SUPER, with just a little performance boost. Nevertheless, both cards easily defeated NVIDIA’s last-generation champions.
Compared to an RTX 3080 10G, the RTX 4070 Ti SUPER is a whooping 30% quicker, while the RTX 4080 SUPER is over 40% faster. Here, the RTX 4070 Ti SUPER delivers the best value because to its low cost and large VRAM buffer.
Stable Diffusion XL + ControlNet*2 + LoRA
In order to produce a few photos utilizing Stable Diffusion XL and the two ControlNets + LoRA from the earlier tests, they increase the resolution to 1024×1024 in the subsequent test.
Once again, the findings are a little surprising: The RTX 4080 16G outperformed the competition, boasting a strong ~53% advantage over the RTX 3080 10G. Furthermore, it surpasses the RTX 4070 Ti SUPER by around 21%.Image credit to MSI
With a lead of 6.5%, the RTX 4070 Ti SUPER doesn’t provide much of an advantage over the RTX 3080 Ti 12G, but it does produce pictures 26.6% quicker than the RTX 3080 10G.
AnimateDiff Text to Video + ControlNet
They don’t anticipate a significant impact in VRAM use while using AnimateDiff in ComfyUI to conduct a Text to Video workload. But because they are currently testing at comparatively lower resolutions (1024×1024), which won’t put too much strain on the VRAM, this is to be anticipated.
They will be creating an animated triple-fan graphics card for this test
RTX 4070 Super vs 3080 Ti
It looks a little off (very common for AI-generated graphics without much polish), but it should work well for us to monitor performance. As for the outcomes, the RTX 4070 Ti SUPER is doing well. Rendering animations 13% quicker than the RTX 3080 Ti 12G, it comfortably outperforms the previous generation champion. Furthermore, it outperforms the RTX 3080 by delivering a 35% quicker result!
AnimateDiff Video to Video
You may create an animation from a video by using the Video to Video pipeline for AnimateDiff. Since ComfyUI offers you a little bit more freedom than WebUI, they will employ it for this purpose.
This test’s findings are precisely what was anticipated, with the RTX 4070 Ti SUPER taking first place with ease once again. In testing, the RTX 4070 Ti SUPER outperformed the 3080 Ti 12G by 10.5% and the 3080 10G by 33%!Image credit to MSI
Playing Around with AnimateDiff LoRA
The group then made the enjoyable decision to create an animated version of Dr. Lucky! They needed to employ both a LoRA and a ControlNet to do this.
When creating AI pictures, you’ll come across all or at least one of these phrases since they’re crucial to producing outcomes that are significantly more reliable and useable. What then are they?
Overview of ControlNet and LoRA in Brief
ControlNets are neural network structures that let you use extra conditions to regulate diffusion models. Thus, by adding it to a model, you may manipulate the final picture without giving it too much information.
Conversely, a LoRA (Localized Representation Adjustment) modifies the outputs of Stable Diffusion by basing them on notions that are comparatively more limited, such as characters, topics, or styles of art.
Producing MSI Animated Fortune!
To get the ideal outcome, the personal “Lucky” LoRA had to be trained, as shown in the charming and bespectacled result below. It included choosing the appropriate dragon species, striking a suitable attitude, and other things. The video below provides more information on the procedure.
The Finest GPU for AnimateDiff-Generated Animated Videos
Even with reduced resolutions, the RTX 4070 Ti SUPER 16G surpasses even the best cards from NVIDIA’s previous generation in terms of producing animated films. At higher resolutions, the VRAM needs increase dramatically, and they anticipate seeing much more of a disparity between these GPUs.
If your job entails producing these kinds of movies, animations, or graphics on a regular basis, an RTX 4070 Ti SUPER 16G GPU with a 16GB VRAM buffer is a great choice. Because of its increased VRAM capacity, it not only outperforms all previous generation components at higher resolutions, but it also outperforms them in terms of raw performance!
Await the next tests, which will challenge these GPUs to produce even better quality and resolution animations.
Read more on Govindhtech.com
#stablediffusion#animateddiff#dlss3#govindhtech#LoRA#RTX4070TiSUPER#nvidia#StableDiffusionXL#news#technews#technologynews#technology#technologytrends
0 notes
Text
Musing out loud
This post may not be what you think. I just noticed a single negative comment in my feed for the first time in months. I can probably count on my hands the few times my 2,000+ posts have been criticized. Outside of our gay AI-generative bubble, there is a lot of noise around this still relatively new form of expression...the debate whether it's art or not or is the AI learning process actually learning or stealing from legit artists. The hetero creators seem to get more flack over their impossibly volumed busty creations. In my other life, I push pixels professionally with little use for generative AI (though machine learning tools in Adobe have been a lifesaver). My work hasn't been threatened yet. I chose to learn and embrace this technology so that when the time does come in my day job, I can adapt, rather than be left behind.
But here in our world of gay generative creations, we're having a lot of fun discovering what we can do. This is a new revolution that in many ways to can be compared to the tools Adobe and MetaCreations gave us years and decades ago with morph, warp, and liquify tools visualize our desires to share with others.
And there is room for this new form of expression without shoving out the works leading up to this point. HS Muscleboy's sketches are legendary and can still excite me! Silverjow will never be out-AI'ed (is that a thing) from their artwork that practically bulges off the screen without the need for 3d specs. And many, many others that I hope continue to create.
Back into the generative AI segment...I do hope that general consumers do understand that there are different levels of effort in this from casual or carefully worded prompts into MidJourney or Bing, to the works of those that train their own models and Loras, pushing and directing their creations via dozens of tweaks via ComfyUI, Automatic1111, and other emerging tools.
Myself? I fall somewhere in between. I'm not so good at training models which takes some patience and dozens if not hundreds of source images and coaxing. I do what I can and try to come in from the analog side of things and sketch the final touches of what the AI gives me.
Hey if you followed my musing this far...what do you think? I suppose I'm looking for some feedback. I wonder if this stuff is worth my time beyond just collecting likes. (I love the likes though and I like all of you back!)
34 notes
·
View notes
Text
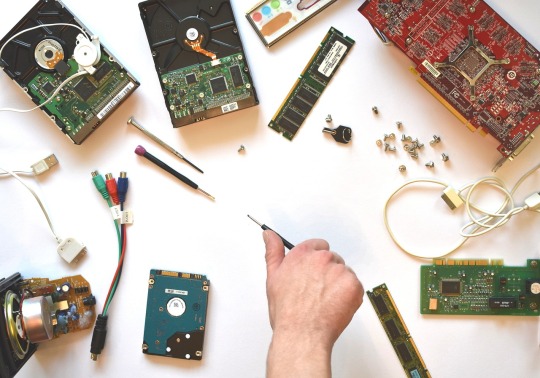
Custom Library Rescues Good LoRa Hardware From Bad Firmware These days, some development boards come with an absolutely astounding variety of hardware. The EESP32, a tiny LoRa board with WiFi, Bluetooth, a transceiver that spans a large portion of the UHF band, and conveniences like OLED display... https://stories.udo0.com/?p=5432&feed_id=299
1 note
·
View note
Text
tumblr is not currently selling your art to midjourney. the deal has not been made. even if it had, the data is currently unusable. i am begging you all to chill and stop sharing posts promoting nightshade and glaze as the last bastions of artistic integrity against evil tech companies.
i think what annoys me about a lot of the ways people online talk about AI art is that a lot of the proposed "solutions" i see championed are functionally just riding on the idea of un-opening pandora's box, which means they're incredibly ineffective because that's just not something we can do at this point. and worse, that sentiment is exploitable.
sure it makes you feel like you, personally, as a creator, have control over this new development threatening your livelihood. but that's not a good thing! glaze is a grift that uses the exact same technology as stable diffusion and straight up doesn't work as advertised, the creators bank on you feeling that way. it doesn't protect you against anything, it just makes you feel good, meanwhile the creators gets money and exposure out of your fear.
if you didn't know, the same developers who made glaze are also behind nightshade. and what do they do with nightshade's popularity? well it's simple, they've studied the effect it has on AI art algorithms. and then they sold the research.
and you must understand, even if everything i've said wasn't the case, making the pictures these algorithms produce compatible for training algorithms again is as easy as running them through a de-noising upscaler.
and i'm an artist myself. i do not want my art used in that way. i do not want to be in midjourney's training data, i don't want someone to make a LORA of my work without my consent, i don't want any of that. but still, ask yourself: who benefits from making us panicked and afraid every single time a new AI deal is mentioned? because it's not you or me. there is a problem, and no problem has ever been solved through fear.
which is also why i'm not here to say you're evil for using these tools, or that they are secretly worse than the companies you're trying to combat by using them. it's not wrong to want to feel safe, you are perfectly within your right to do what makes you feel in control. you can keep using them if that's what you want! but please, be aware of what's going on here.
there is no going back. the technology exists, we have to accept it. because the sooner we accept this is the reality we live in, the sooner we'll be able to fight it. but i am begging you all to stop pretending easy solutions exists to this problem, there are none.
demand transparency. demand control. demand that this things be opt-in. demand compensation.
you will not be saved from companies trying to profit from these algorithms by simply going to their competitors.
#shut up sender#ai#midjourney#tumblr#long post#this one has stayed in the drafts for a WHILE#kinda scared to post it but it's been bugging me especially the past few days#guess im getting controversial on main again
47 notes
·
View notes
Text
Long-Range IoT Communication with LoRa Modules: SX1278 and ESP32 with Display
SX1278 LoRa Module
The SX1278 LoRa module is a popular choice among developers due to its low power consumption, long-range capabilities, and support for multiple frequency bands. It is based on the Semtech SX1278 chip, which is a low-power, long-range transceiver designed for use in the Industrial, Scientific, and Medical (ISM) frequency bands. The module operates in the 433MHz frequency band and has a range of up to 5 km in open space.
The SX1278 LoRa module can be easily integrated into a variety of applications, including Internet of Things (IoT) devices, smart cities, and remote monitoring systems. It supports a wide range of data rates, from 300 bps to 37.5 kbps, and has a programmable output power up to +20 dBm.
One of the key advantages of the SX1278 LoRa module is its low power consumption. It has a sleep mode that consumes only 0.1 µA of current, making it an ideal choice for battery-powered applications. The module also has a built-in temperature sensor and a low battery detector, which can be used to optimize power consumption and extend battery life.
ESP32 LoRa with Display SX1278
The ESP32 LoRa with Display SX1278 is a popular implementation of the SX1278 LoRa module. It combines the low power consumption and long-range capabilities of the SX1278 with the processing power and connectivity features of the ESP32 microcontroller. The module has a 128x64 OLED display, which can be used to display sensor readings, status messages, and other information.
The ESP32 LoRa with Display SX1278 is compatible with the Arduino IDE and can be programmed using the Arduino programming language. It has a built-in WiFi and Bluetooth connectivity, which allows it to connect to other devices and the Internet. The module also has a built-in antenna, which simplifies the integration process and reduces the overall size of the device.
One of the key features of the ESP32 LoRa with Display SX1278 is its ease of use. It comes with a preloaded firmware that can be easily customized using the Arduino IDE. The firmware includes support for LoRaWAN, a popular protocol for building large-scale IoT networks. The module can also be used with other LoRa protocols, such as LoRa-MAC, LoRa-RAW, and LoRa-P2P.
ESP32 LoRa with Display SX1276
The ESP32 LoRa with Display SX1276 is another popular implementation of the ESP32 LoRa module, but this time with the SX1276 chip. The module operates in the 868 MHz frequency band and has a range of up to 10 km in open space. Like the SX1278 module, it has a low power consumption and can be used in a variety of applications, including smart agriculture, environmental monitoring, and asset tracking.
The ESP32 LoRa with Display SX1276 has a 128x64 OLED display, which can be used to display sensor readings and other information. It also has a built-in antenna and a WiFi/Bluetooth connectivity, which allows it to connect to other devices and the Internet.
One of the key advantages of the ESP32 LoRa with Display SX1276 is its compatibility with the Arduino IDE. It can be programmed using the Arduino programming language and comes with a preloaded firmware that can be easily customized. The firmware includes support for LoRaWAN and other LoRa protocols, making it easy to build large-scale IoT networks.
In addition to its low power consumption and long-range capabilities, the ESP32 LoRa with Display SX1276 has a variety of features that make it an ideal choice for IoT applications. It has a built-in accelerometer and gyroscope, which can be used for motion sensing and orientation detection. It also has a built-in GPS module, which can be used for location tracking and geofencing.
The module can be powered by a variety of sources, including batteries, solar panels, and external power sources. It also has a deep sleep mode that consumes only 10 µA of current, making it an ideal choice for battery-powered applications.
Conclusion
The SX1278 LoRa module and the ESP32 LoRa with Display modules are two popular choices for developers looking to build IoT applications that require long-range communication and low power consumption. The SX1278 module is a versatile solution that can be easily integrated into a variety of applications, while the ESP32 LoRa with Display modules provide additional features, such as processing power, connectivity, and display capabilities.
When selecting a LoRa module, developers should consider their specific requirements, such as range, power consumption, data rate, and frequency band. They should also consider the features and capabilities of the module, such as built-in sensors, connectivity options, and compatibility with different protocols and programming languages.
Overall, LoRa technology provides an attractive alternative to traditional wireless communication technologies, such as WiFi and Bluetooth, for IoT applications that require long-range communication and low power consumption. With the availability of versatile LoRa modules, such as the SX1278 LoRa module and the ESP32 LoRa with Display modules, developers have more options than ever before to build innovative IoT solutions.
0 notes