#LMStudio
Explore tagged Tumblr posts
Text
Obsidian And RTX AI PCs For Advanced Large Language Model
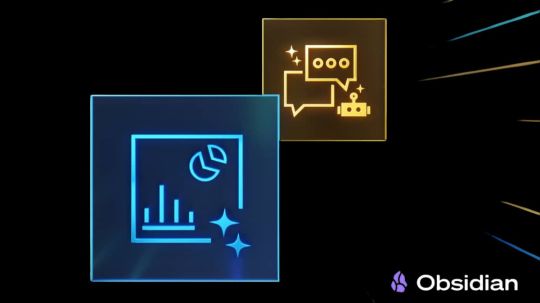
How to Utilize Obsidian‘s Generative AI Tools. Two plug-ins created by the community demonstrate how RTX AI PCs can support large language models for the next generation of app developers.
Obsidian Meaning
Obsidian is a note-taking and personal knowledge base program that works with Markdown files. Users may create internal linkages for notes using it, and they can see the relationships as a graph. It is intended to assist users in flexible, non-linearly structuring and organizing their ideas and information. Commercial licenses are available for purchase, however personal usage of the program is free.
Obsidian Features
Electron is the foundation of Obsidian. It is a cross-platform program that works on mobile operating systems like iOS and Android in addition to Windows, Linux, and macOS. The program does not have a web-based version. By installing plugins and themes, users may expand the functionality of Obsidian across all platforms by integrating it with other tools or adding new capabilities.
Obsidian distinguishes between community plugins, which are submitted by users and made available as open-source software via GitHub, and core plugins, which are made available and maintained by the Obsidian team. A calendar widget and a task board in the Kanban style are two examples of community plugins. The software comes with more than 200 community-made themes.
Every new note in Obsidian creates a new text document, and all of the documents are searchable inside the app. Obsidian works with a folder of text documents. Obsidian generates an interactive graph that illustrates the connections between notes and permits internal connectivity between notes. While Markdown is used to accomplish text formatting in Obsidian, Obsidian offers quick previewing of produced content.
Generative AI Tools In Obsidian
A group of AI aficionados is exploring with methods to incorporate the potent technology into standard productivity practices as generative AI develops and speeds up industry.
Community plug-in-supporting applications empower users to investigate the ways in which large language models (LLMs) might improve a range of activities. Users using RTX AI PCs may easily incorporate local LLMs by employing local inference servers that are powered by the NVIDIA RTX-accelerated llama.cpp software library.
It previously examined how consumers might maximize their online surfing experience by using Leo AI in the Brave web browser. Today, it examine Obsidian, a well-known writing and note-taking tool that uses the Markdown markup language and is helpful for managing intricate and connected records for many projects. Several of the community-developed plug-ins that add functionality to the app allow users to connect Obsidian to a local inferencing server, such as LM Studio or Ollama.
To connect Obsidian to LM Studio, just select the “Developer” button on the left panel, load any downloaded model, enable the CORS toggle, and click “Start.” This will enable LM Studio’s local server capabilities. Because the plug-ins will need this information to connect, make a note of the chat completion URL from the “Developer” log console (“http://localhost:1234/v1/chat/completions” by default).
Next, visit the “Settings” tab after launching Obsidian. After selecting “Community plug-ins,” choose “Browse.” Although there are a number of LLM-related community plug-ins, Text Generator and Smart Connections are two well-liked choices.
For creating notes and summaries on a study subject, for example, Text Generator is useful in an Obsidian vault.
Asking queries about the contents of an Obsidian vault, such the solution to a trivia question that was stored years ago, is made easier using Smart Connections.
Open the Text Generator settings, choose “Custom” under “Provider profile,” and then enter the whole URL in the “Endpoint” section. After turning on the plug-in, adjust the settings for Smart Connections. For the model platform, choose “Custom Local (OpenAI Format)” from the options panel on the right side of the screen. Next, as they appear in LM Studio, type the model name (for example, “gemma-2-27b-instruct”) and the URL into the corresponding fields.
The plug-ins will work when the fields are completed. If users are interested in what’s going on on the local server side, the LM Studio user interface will also display recorded activities.
Transforming Workflows With Obsidian AI Plug-Ins
Consider a scenario where a user want to organize a trip to the made-up city of Lunar City and come up with suggestions for things to do there. “What to Do in Lunar City” would be the title of the new note that the user would begin. A few more instructions must be included in the query submitted to the LLM in order to direct the results, since Lunar City is not an actual location. The model will create a list of things to do while traveling if you click the Text Generator plug-in button.
Obsidian will ask LM Studio to provide a response using the Text Generator plug-in, and LM Studio will then execute the Gemma 2 27B model. The model can rapidly provide a list of tasks if the user’s machine has RTX GPU acceleration.
Or let’s say that years later, the user’s buddy is visiting Lunar City and is looking for a place to dine. Although the user may not be able to recall the names of the restaurants they visited, they can review the notes in their vault Obsidian‘s word for a collection of notes to see whether they have any written notes.
A user may ask inquiries about their vault of notes and other material using the Smart Connections plug-in instead of going through all of the notes by hand. In order to help with the process, the plug-in retrieves pertinent information from the user’s notes and responds to the request using the same LM Studio server. The plug-in uses a method known as retrieval-augmented generation to do this.
Although these are entertaining examples, users may see the true advantages and enhancements in daily productivity after experimenting with these features for a while. Two examples of how community developers and AI fans are using AI to enhance their PC experiences are Obsidian plug-ins.
Thousands of open-source models are available for developers to include into their Windows programs using NVIDIA GeForce RTX technology.
Read more on Govindhtech.com
#Obsidian#RTXAIPCs#LLM#LargeLanguageModel#AI#GenerativeAI#NVIDIARTX#LMStudio#RTXGPU#News#Technews#Technology#Technologynews#Technologytrends#govindhtech
3 notes
·
View notes
Video
youtube
LM Studio - SUPER EASY Text AI - Windows, Mac & Linux / How To 👩💻📦📥 https://applevideos.co.uk/mac-studio/lm-studio-super-easy-text-ai-windows-mac-amp-linux-how-to
0 notes
Text
I have been experimenting with Deepseek AI's Deepseek 67b chat.
In my opinion, Meta AI's Llama-2 70b is too politically misaligned for my work. It has too much political bias in the training, which makes sense for an American corporation that's likely considering it for automatic moderation, but the problem is deeper - there isn't enough of the right political information in the training data.
Using an LLM for text is like using diffusion models for images - it's about calling up sources from the training data for the model to assemble. Even in versions of Llama-2 which have been "uncensored," it's difficult to get the model to "think strategically," so to speak.
Deepseek 67b chat is a lot like a Llama-2 which has been "toned down" in this respect, with a better ability to "think strategically" in a late modern way - which is starting to look like a difference between American and Chinese models more generally at this point.
Like Llama-2, Deepseek 67b has a rather short context window of 4096 tokens. (This was actually too short for today's 2,400 word longpost about writing, although I got a few casual comments by playing with extending the RoPE setting in LMStudio.)
Both are trounced in this respect by Nous Research's Nous Capybara 34b fine-tune of 01 AI's Yi-34b-200k, which has a 200,000 token context window.
4 notes
·
View notes
Text
"Belmont Abbey: Droned Out"
The first episode of our spinoff of Knights of Belmont, “Belmont Abbey,” is now out across all our social media! In “Droned Out,” technology is weird in the World of Belmont! Bart and Dave find that out the hard way! Featuring Emmy Edward as the voice of the drone pilot, with Chris Salinas reprising his role as Sir Dave and Taylor W. Wilson reprising his role as Bart the Bartender More LMStudios…
0 notes
Text
LM Studio with Phi 3.5 on Snapdragon X Elite No NPU support yet
LM Studio with Phi 3.5 on Snapdragon X Elite No NPU support yet #ai #npu #surfacepro11 #snapdragonxelite #arm64 #lmstudio #microsoftphi35 #llm #locallanguagemodel
I upgraded from my Surface Laptop 4 to a Surface Pro 11 with the Snapdragon X Elite processor and have been very satisfied so far with the performance, battery life, and the compatibility. I wanted to see if LM studio would run on the Surface Pro with the Snapdragon. To my surprise they have recently released LM Studio in a tech preview for Windows on ARM. So let’s look! Installing LM Studio…
0 notes
Text
GitHub - lmstudio-ai/lms: LM Studio CLI. Written in TypeScript/Node
https://github.com/lmstudio-ai/lms Enviado do meu telemóvel HONOR
View On WordPress
0 notes
Text
RAG Llama3 8B with RTX 3050
測試 Wsxqaza12 所分享的 RAG 方案,使用 lmstudio-community/Meta-Llama-3-8B-Instruct-GGUF 建構 RAG Llama3 8B 的系統。 (模型經Q4量化) 車輛裝載貨物需要注意些甚麼呢還有甚麼需要避免違法呢 Reference by: https://github.com/wsxqaza12/RAG_example
youtube
View On WordPress
0 notes
Video
youtube
Stop paying for ChatGPT with these two tools | LMStudio x AnythingLLM
0 notes
Text
#SemanticKernel - 📎Chat Service demo running Phi-2 LLM locally with #LMStudio
Hi! It’s time to go back to AI and NET, so today’s post is a small demo on how to run a LLM (large language model, this demo using Phi-2) in local mode, and how to interact with the model using Semantic Kernel. LM Studio I’ve tested several products and libraries to run LLMs locally, and LM Studio is on my Top 3. LM Studio is a desktop application that allows you to run open-source models…
View On WordPress
0 notes
Link
So good I had to share! Check out all the items I'm loving on @Poshmarkapp #poshmark #fashion #style #shopmycloset #truereligion #lmstudio #toms: https://posh.mk/uwoIjc3ztZ
0 notes
Text
AMD Ryzen AI 300 Series Improves LM Studio And llama.cpp
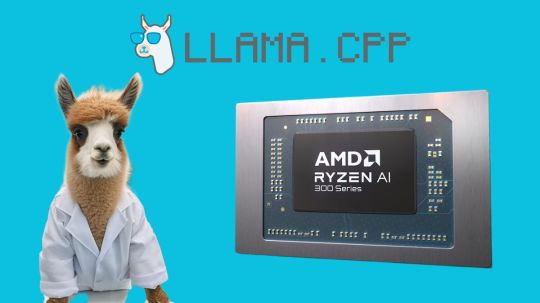
Using AMD Ryzen AI 300 Series to Speed Up Llama.cpp Performance in Consumer LLM Applications.
What is Llama.cpp?
Meta’s LLaMA language model should not be confused with Llama.cpp. It is a tool, nonetheless, that was created to improve Meta’s LLaMA so that it can operate on local hardware. Because of their very high computational expenses, LLaMA and ChatGPT currently have trouble operating on local computers and hardware. Despite being among of the best-performing models available, they are somewhat demanding and inefficient to run locally since they need a significant amount of processing power and resources.
Here’s where llama.cpp is useful. It offers a lightweight, resource-efficient, and lightning-fast solution for LLaMA models using C++. It even eliminates the need for a GPU.
Features of Llama.cpp
Let’s examine Llama.cpp’s features in further detail and see why it’s such a fantastic complement to Meta’s LLaMA language paradigm.
Cross-Platform Compatibility
One of those features that is highly valued in any business, whether it gaming, artificial intelligence, or other software types, is cross-platform compatibility. It’s always beneficial to provide developers the flexibility to execute applications on the environments and systems of their choice, and llama.cpp takes this very seriously. It is compatible with Windows, Linux, and macOS and works perfectly on any of these operating systems.
Efficient CPU Utilization
The majority of models need a lot of GPU power, including ChatGPT and even LLaMA itself. Because of this, running them most of the time is quite costly and power-intensive. This idea is turned on its head by Llama.cpp, which is CPU-optimized and ensures that you receive respectable performance even in the absence of a GPU. Even while a GPU will provide superior results, it’s still amazing that running these LLMs locally doesn’t cost hundreds of dollars. Additionally encouraging for the future is the fact that it was able to tweak LLaMA to operate so effectively on CPUs.
Memory Efficiency
Llama.cpp excels at more than just CPU economy. Even on devices without strong resources, LLaMA models can function successfully by controlling the llama token limit and minimizing memory utilization. Successful inference depends on striking a balance between memory allocation and the llama token limit, which is something that llama.cpp excels at.
Getting Started with Llama.cpp
The popularity of creating beginner-friendly tools, frameworks, and models is at an all-time high, and llama.cpp is no exception. Installing it and getting started are rather simple processes.
You must first clone the llama.cpp repository in order to begin.
It’s time to create the project when you’ve finished cloning the repository.
Once your project is built, you may use your LLaMA model to do llama inference. The following code must be entered in order to utilize the llama.cpp library to do inference:
./main -m ./models/7B/ -p “Your prompt here” To change the output’s determinism, you may play about with the llama inference parameters, such llama temperature. The llama prompt format and prompt may be specified using the -p option, and llama.cpp will take care of the rest.
An overview of LM Studio and llama.cpp
Since GPT-2, language models have advanced significantly, and users may now rapidly and simply implement very complex LLMs using user-friendly programs like LM Studio. These technologies, together with AMD, enable AI to be accessible to all people without the need for technical or coding skills.
The llama.cpp project, a well-liked framework for rapidly and simply deploying language models, is the foundation of LM Studio. Despite having GPU acceleration available, it is independent and may be accelerated only using the CPU. Modern LLMs for x86-based CPUs are accelerated by LM Studio using AVX2 instructions.
Performance comparisons: throughput and latency
AMD Ryzen AI provides leading performance in llama.cpp-based programs such as LM Studio for x86 laptops and speeds up these cutting-edge tasks. Note that memory speeds have a significant impact on LLMs in general. When the compared the two laptops, the AMD laptop had 7500 MT/s of RAM while the Intel laptop had 8533 MT/s.Image Credit To AMD
Despite this, the AMD Ryzen AI 9 HX 375 CPU outperforms its rivals by up to 27% when considering tokens per second. The parameter that indicates how fast an LLM can produce tokens is called tokens per second, or tk/s. This generally translates to the amount of words that are shown on the screen per second.
Up to 50.7 tokens per second may be produced by the AMD Ryzen AI 9 HX 375 CPU in Meta Llama 3.2 1b Instruct (4-bit quantization).
The “time to first token” statistic, which calculates the latency between the time you submit a prompt and the time it takes for the model to begin producing tokens, is another way to benchmark complex language models. Here, it can see that the AMD “Zen 5” based Ryzen AI HX 375 CPU is up to 3.5 times quicker than a similar rival processor in bigger versions.Image Credit To AMD
Using Variable Graphics Memory (VGM) to speed up model throughput in Windows
Every one of the AMD Ryzen AI CPU’s three accelerators has a certain workload specialty and set of situations in which they perform best. On-demand AI activities are often handled by the iGPU, while AMD XDNA 2 architecture-based NPUs provide remarkable power efficiency for permanent AI while executing Copilot+ workloads and CPUs offer wide coverage and compatibility for tools and frameworks.
With the vendor-neutral Vulkan API, LM Studio’s llama.cpp port may speed up the framework. Here, acceleration often depends on a combination of Vulkan API driver improvements and hardware capabilities. Meta Llama 3.2 1b Instruct performance increased by 31% on average when GPU offload was enabled in LM Studio as opposed to CPU-only mode. The average uplift for larger models, such as Mistral Nemo 2407 12b Instruct, which are bandwidth-bound during the token generation phase, was 5.1%.
In comparison to CPU-only mode, it found that the competition’s processor saw significantly worse average performance in all but one of the evaluated models while utilizing the Vulkan-based version of llama.cpp in LM Studio and turning on GPU-offload. In order to maintain fairness in the comparison, it have excluded the GPU-offload performance of the Intel Core Ultra 7 258v from LM Studio’s Vulkan back-end, which is based on Llama.cpp.
Another characteristic of AMD Ryzen AI 300 Series CPUs is Variable Graphics Memory (VGM). Programs usually use the second block of memory located in the “shared” section of system RAM in addition to the 512 MB block of memory allocated specifically for an iGPU. The 512 “dedicated” allotment may be increased by the user using VGM to up to 75% of the system RAM that is available. When this contiguous memory is present, memory-sensitive programs perform noticeably better.
Using iGPU acceleration in conjunction with VGM, it saw an additional 22% average performance boost in Meta Llama 3.2 1b Instruct after turning on VGM (16GB), for a net total of 60% average quicker speeds when compared to the CPU. Performance improvements of up to 17% were seen even for bigger models, such as the Mistral Nemo 2407 12b Instruct, when compared to CPU-only mode.
Side by side comparison: Mistral 7b Instruct 0.3
It compared iGPU performance using the first-party Intel AI Playground application (which is based on IPEX-LLM and LangChain) in order to fairly compare the best consumer-friendly LLM experience available, even though the competition’s laptop did not provide a speedup using the Vulkan-based version of Llama.cpp in LM Studio.
It made use of the Microsoft Phi 3.1 Mini Instruct and Mistral 7b Instruct v0.3 models that came with Intel AI Playground. To observed that the AMD Ryzen AI 9 HX 375 is 8.7% quicker in Phi 3.1 and 13% faster in Mistral 7b Instruct 0.3 using a same quantization in LM Studio.Image Credit To AMD
AMD is committed to pushing the boundaries of AI and ensuring that it is available to everybody. Applications like LM Studio are crucial because this cannot occur if the most recent developments in AI are restricted to a very high level of technical or coding expertise. In addition to providing a rapid and easy method for localizing LLM deployment, these apps let users to experience cutting-edge models almost immediately upon startup (if the architecture is supported by the llama.cpp project).
AMD Ryzen AI accelerators provide amazing performance, and for AI use cases, activating capabilities like variable graphics memory may result in even higher performance. An amazing user experience for language models on an x86 laptop is the result of all of this.
Read more on Govindhtech.com
#AMDRyzen#AMDRyzenAI300#ChatGPT#MetaLLaMA#Llama.cpp#languagemodels#MetaLlama#AMDXDNA#IntelCoreUltra7#MistralNemo#LMStudio#News#Technews#Technology#Technologynews#Technologytrends#govindhtech
0 notes
Text
Knights of Belmont: Episode 1 is LIVE!!!
The adventure begins! Click on the video’s title to view on YouTube! And don’t forget to subscribe! After a night of partying, King Kingsley has discovered a family member has gone missing, and his two worst-but-best knights are missing! Prepare for an exciting and comedic adventure with Sir Dave and Sir George in this first episode of Knights of Belmont! More LMStudios across socials!Website:…
0 notes
Photo

A cada homenagem e troféu que recebo, minha responsabidade como arte educador só aumenta!!! #juliomolinachoreographer #nitmodelsagency #danielsaboyadancestudio #LMStudio #shoppingjardimguadalupe #cubekidshiphop #CubeCrew #kids #teen #instagood https://www.instagram.com/p/Brh557uFpzs/?utm_source=ig_tumblr_share&igshid=2zfy1ugezicw
#juliomolinachoreographer#nitmodelsagency#danielsaboyadancestudio#lmstudio#shoppingjardimguadalupe#cubekidshiphop#cubecrew#kids#teen#instagood
0 notes
Text
Game Giveaway
https://www.kicktraq.com/projects/lmstudio/dungeonology-the-expedition/
0 notes
Text
Dungeonology: the expedition - Italian Kickstarter Campaign
Dungeonology: the expedition – Italian Kickstarter Campaign
This Kickstarter campaign, https://www.kickstarter.com/projects/lmstudio/dungeonology-the-expedition, was funded in 11 minutes. Considering its campaign goal was €53,000 (roughly $59,844 USD give or take), getting funded in 11 minutes is impressive. This is a slightly different game than one might expect. You will see why below. Here’s the Kicktraq for the campaign: http://www.kicktraq.com/projec…
View On WordPress
0 notes