#Customer behavior analysis
Explore tagged Tumblr posts
Text

AI Customer Behavior Analysis
Gain deep insights into customer behavior with our advanced analytics tools and solutions. Our platform helps you track, analyze, and predict customer actions, enabling data-driven decisions to enhance engagement, improve marketing strategies, and drive business growth.
#Customer Behavior Analysis#AI Analytics Solutions#customer insights analysis#customer analytics#Primathon
1 note
·
View note
Text
Mastering Efficiency: Retail Inventory Management Solutions
Business intelligence (BI) in the retail industry delivers comprehensive data on contact between customers and eCommerce stores. This data can allow merchants to make better business decisions. It can also assist brick-and-mortar stores in understanding client behavior, making merchandise adjustments, and altering prices accordingly. Business intelligence helps merchants be prepared for pricing, stock availability, trends, shipping, and more.
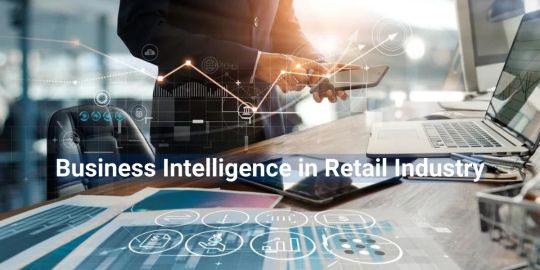
Optimize your retail operations with precision. Explore our advanced inventory management solutions for seamless efficiency and improved profitability.
#Benefits of BI in Retail#Best BI software for Retail Industry#BI for retail industry#BI in Retail Industry#BI solutions for retail#Business analytics in retail industry#Business Intelligence in Retail Industry#Business Intelligence Tools for Retail#Customer Behavior Analysis#Retail Inventory Management#Omnichannel Retail Analytics#Predictive Analytics for Retail#Retail Analytics Solutions#Retail Business Intelligence#Retail Competitive Intelligence#Retail Data Analysis#Retail Dashboard Solutions#Retail Intelligence Software#Retail Performance Metrics#Retail Sales Forecasting#Retail Marketing Analytics#Supply Chain Analytics for Retail
1 note
·
View note
Text
AI-Powered Marketing: ChatGPT4 Creates Perfect Campaign with Zero Human Intervention
In today’s digital age, the use of artificial intelligence (AI) is becoming increasingly prevalent in various industries. From healthcare to finance to marketing, AI-powered solutions are transforming the way we work and operate. And the latest breakthrough comes from ChatGPT4, a language model trained by OpenAI, which has successfully created a marketing campaign with zero human intervention,…
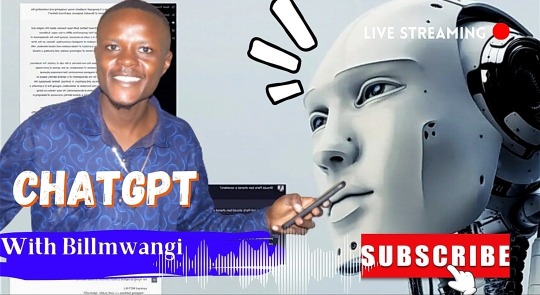
View On WordPress
#A/B testing#AI-generated content#AI-powered marketing#Automation in advertising#Brand awareness#Brand building#Business growth#Campaign performance tracking#ChatGPT4#Competitive advantage#Consumer insights#Content marketing#Conversion rate optimization#Cost-effective marketing#Creative automation#Customer behavior analysis#Customer engagement#Customer relationship management#Customer service automation#Data-driven marketing#Digital advertising#Digital Marketing#E-commerce#Email marketing#Influencer Marketing#Innovative marketing#Lead generation#Machine learning#Market research#Marketing analytics
0 notes
Text
What Are the Qualifications for a Data Scientist?
In today's data-driven world, the role of a data scientist has become one of the most coveted career paths. With businesses relying on data for decision-making, understanding customer behavior, and improving products, the demand for skilled professionals who can analyze, interpret, and extract value from data is at an all-time high. If you're wondering what qualifications are needed to become a successful data scientist, how DataCouncil can help you get there, and why a data science course in Pune is a great option, this blog has the answers.
The Key Qualifications for a Data Scientist
To succeed as a data scientist, a mix of technical skills, education, and hands-on experience is essential. Here are the core qualifications required:
1. Educational Background
A strong foundation in mathematics, statistics, or computer science is typically expected. Most data scientists hold at least a bachelor’s degree in one of these fields, with many pursuing higher education such as a master's or a Ph.D. A data science course in Pune with DataCouncil can bridge this gap, offering the academic and practical knowledge required for a strong start in the industry.
2. Proficiency in Programming Languages
Programming is at the heart of data science. You need to be comfortable with languages like Python, R, and SQL, which are widely used for data analysis, machine learning, and database management. A comprehensive data science course in Pune will teach these programming skills from scratch, ensuring you become proficient in coding for data science tasks.
3. Understanding of Machine Learning
Data scientists must have a solid grasp of machine learning techniques and algorithms such as regression, clustering, and decision trees. By enrolling in a DataCouncil course, you'll learn how to implement machine learning models to analyze data and make predictions, an essential qualification for landing a data science job.
4. Data Wrangling Skills
Raw data is often messy and unstructured, and a good data scientist needs to be adept at cleaning and processing data before it can be analyzed. DataCouncil's data science course in Pune includes practical training in tools like Pandas and Numpy for effective data wrangling, helping you develop a strong skill set in this critical area.
5. Statistical Knowledge
Statistical analysis forms the backbone of data science. Knowledge of probability, hypothesis testing, and statistical modeling allows data scientists to draw meaningful insights from data. A structured data science course in Pune offers the theoretical and practical aspects of statistics required to excel.
6. Communication and Data Visualization Skills
Being able to explain your findings in a clear and concise manner is crucial. Data scientists often need to communicate with non-technical stakeholders, making tools like Tableau, Power BI, and Matplotlib essential for creating insightful visualizations. DataCouncil’s data science course in Pune includes modules on data visualization, which can help you present data in a way that’s easy to understand.
7. Domain Knowledge
Apart from technical skills, understanding the industry you work in is a major asset. Whether it’s healthcare, finance, or e-commerce, knowing how data applies within your industry will set you apart from the competition. DataCouncil's data science course in Pune is designed to offer case studies from multiple industries, helping students gain domain-specific insights.
Why Choose DataCouncil for a Data Science Course in Pune?
If you're looking to build a successful career as a data scientist, enrolling in a data science course in Pune with DataCouncil can be your first step toward reaching your goals. Here’s why DataCouncil is the ideal choice:
Comprehensive Curriculum: The course covers everything from the basics of data science to advanced machine learning techniques.
Hands-On Projects: You'll work on real-world projects that mimic the challenges faced by data scientists in various industries.
Experienced Faculty: Learn from industry professionals who have years of experience in data science and analytics.
100% Placement Support: DataCouncil provides job assistance to help you land a data science job in Pune or anywhere else, making it a great investment in your future.
Flexible Learning Options: With both weekday and weekend batches, DataCouncil ensures that you can learn at your own pace without compromising your current commitments.
Conclusion
Becoming a data scientist requires a combination of technical expertise, analytical skills, and industry knowledge. By enrolling in a data science course in Pune with DataCouncil, you can gain all the qualifications you need to thrive in this exciting field. Whether you're a fresher looking to start your career or a professional wanting to upskill, this course will equip you with the knowledge, skills, and practical experience to succeed as a data scientist.
Explore DataCouncil’s offerings today and take the first step toward unlocking a rewarding career in data science! Looking for the best data science course in Pune? DataCouncil offers comprehensive data science classes in Pune, designed to equip you with the skills to excel in this booming field. Our data science course in Pune covers everything from data analysis to machine learning, with competitive data science course fees in Pune. We provide job-oriented programs, making us the best institute for data science in Pune with placement support. Explore online data science training in Pune and take your career to new heights!
#In today's data-driven world#the role of a data scientist has become one of the most coveted career paths. With businesses relying on data for decision-making#understanding customer behavior#and improving products#the demand for skilled professionals who can analyze#interpret#and extract value from data is at an all-time high. If you're wondering what qualifications are needed to become a successful data scientis#how DataCouncil can help you get there#and why a data science course in Pune is a great option#this blog has the answers.#The Key Qualifications for a Data Scientist#To succeed as a data scientist#a mix of technical skills#education#and hands-on experience is essential. Here are the core qualifications required:#1. Educational Background#A strong foundation in mathematics#statistics#or computer science is typically expected. Most data scientists hold at least a bachelor’s degree in one of these fields#with many pursuing higher education such as a master's or a Ph.D. A data science course in Pune with DataCouncil can bridge this gap#offering the academic and practical knowledge required for a strong start in the industry.#2. Proficiency in Programming Languages#Programming is at the heart of data science. You need to be comfortable with languages like Python#R#and SQL#which are widely used for data analysis#machine learning#and database management. A comprehensive data science course in Pune will teach these programming skills from scratch#ensuring you become proficient in coding for data science tasks.#3. Understanding of Machine Learning
3 notes
·
View notes
Text
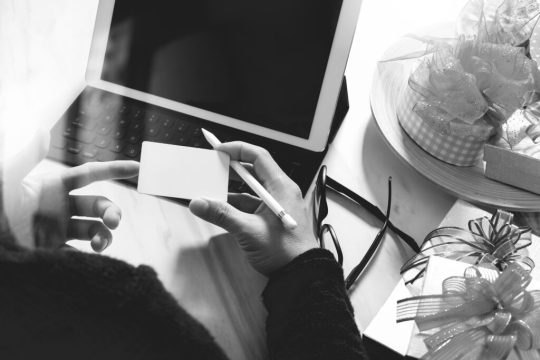
Customer Satisfaction Survey | Consumer Insights Research in UAE
Want to conduct Customer Satisfaction Surveys or consumer insights research? Boost your customer experience, happiness & loyalty with Researchers in Dubai, Abu Dhabi.
#Customer loyalty#Service delivery#Product optimization#Employee training#Feedback analysis#Customer retention#Brand reputation#Consumer behavior#Customer needs#Business growth
2 notes
·
View notes
Text
Customer Research Unplugged: Innovative Engagement Tips
Discover innovative strategies to deeply understand your audience, engage effectively, and drive customer experiences with customer research. For more detail visit here: https://www.philomathresearch.com/blog/2025/02/17/customer-research-unplugged-innovative-strategies-to-understand-and-engage-your-audience/
0 notes
Text
AI’s Honeymoon Phase Is Over, So What Comes Next?
New Post has been published on https://thedigitalinsider.com/ais-honeymoon-phase-is-over-so-what-comes-next/
AI’s Honeymoon Phase Is Over, So What Comes Next?


Countless discussions about AI’s transformative potential have taken place over the past two years since ChatGPT’s initial release generated so much excitement. Corporate leaders have been eager to use the technology to reduce operational expenses. Perhaps surprising, though, is that for many leaders, the key metric used to evaluate the success of an AI tool is not the lifetime return on investment (ROI). It’s the speed to ROI.
Amid shrinking risk tolerance and increased revenue pressure, leaders expect investments to drive changes and pay off quickly. At the same time, the hype around AI is dying down, making way for more pragmatic conversations around the return on AI investments.
The Next Phase: Getting Real About Where AI Works
Success in today’s market—where subscriptions are king—relies on how well you keep customers, not how well you acquire them. In most sectors, the market is oversaturated, and many organizations offer similar services of near-identical quality. Add in a decline in customer loyalty, rising expectations and an increased willingness to switch brands, and organizations find themselves with no room for error to keep up with fierce competition. Customer experience (CX) is the factor that determines whether subscription-based organizations thrive or fall short.
In this environment, organizations can compete best by leaning into incremental improvements rather than away from spending. Each and every choice the organization makes must be oriented toward specific, customer-centric goals — even if it costs a bit more at the start. That extends to AI implementation. Organizations have been asking how AI can recoup its cost by using it as a replacement for existing resources. Now, they need to ask how AI can create value for the organization by improving how they work with customers.
The answer is straightforward enough. AI has numerous potential applications that improve CX both directly and indirectly. AI-powered tools can enhance personalization by using customer behavior data to ensure the users see the right message or promotion at the right time. The same data can help guide product development, highlighting gaps in the market that the organization might capitalize on to better serve customers’ needs. They can also make organizations more proactive, helping them anticipate disruptions, activate contingency plans and communicate necessary information to users.
However, this work happens primarily behind the scenes, and it cannot happen overnight.
Want AI at Its Best? Start With ‘Invisible’ Applications
The only way to know for certain whether a back- or front-end use case will yield the results you’re after is to leverage AI’s more discreet, behind-the-scenes capabilities first.
Behind the headlines about instant transformation is AI’s core capability: analysis. Large language models (LLMs) like ChatGPT turned heads for their apparent flexibility, but they perform only one task no matter where they operate. They summarize information. It’s on organizations to make the right information available, and that takes time. Those are two facts that have often been lost in the conversation, and they represent an end to the “quick fix” reputation AI has come to enjoy.
The next era will be defined by the invisible improvements facilitated by AI as organizations build up their technical foundations. Organizations can start with LLMs that help:
Integrate existing databases and break down silos to provide end-to-end visibility – and the context that comes with it.
Implement real-time data collection tools to ensure insights are up to date and reflect the most recent trends, patterns and disruptions.
Expedite reconciliation and management to ensure accuracy and free up workers to focus on higher-level tasks that require a human touch.
Organizational change is the first step to effective implementation and extends to both systems and staff. At this point, leaders should also consider the ways AI deployments might affect staff and work to get ahead of potential obstacles. Developing upskilling and reskilling programs will help ensure staff is ready to work effectively alongside the new technologies. AI itself can help in these efforts—another of its invisible applications. For example, it can highlight individual knowledge gaps based on utilization data. This kind of information can guide training programs to make sure workers have everything they need to thrive.
Once organizations have integrated, accurate and up-to-date records and a staff that understands how and when to use AI, they can add another layer of “invisible” tools. The next wave of solutions should focus on analytics that help cultivate a deep understanding of how the business runs, what customers want and obstacles getting in the way. These solutions build on one another, with each step revealing a new level of insight.
More specifically, descriptive analytics use historical data to identify historical patterns; they tell organizations what happened. Diagnostic analytics use additional data to contextualize what happened, identify causes and highlight the effects of incidents and changes; they tell organizations why things happened the way they did. Predictive analytics use insights from past events to model the impacts of proposed changes and keep tabs on trends; they show organizations what might happen. Prescriptive analytics use all of these outputs to make informed decisions; they tell organizations what to do next.
Though analytics solutions like these may tap into AI’s more advanced capabilities, it’s worth noting that—at first—nearly all these processes happen behind the scenes. Eventually, predictive and prescriptive algorithms may make their way into consumer-facing solutions, but that can only happen once this critical, internal foundation is laid.
As AI’s honeymoon ends, so too will its reputation as a magic fix—but shedding this perception is critical to realizing the technology’s full potential. Leaders who want to make headlines tomorrow with innovative AI applications must first complete this foundational work, which may be a hard pill to swallow amid pressure for faster and faster returns. However, moving toward more holistic, incremental and long-term assessments of AI’s value will enable organizations to expedite returns. This approach gives leaders the tools and time to develop a clear picture of what needs to be fixed, insight into the small changes that will have the biggest impacts and the ability to develop sound strategies that yield returns today without damaging profitability tomorrow.
Pragmatism from End-to-End
Though flashy use cases may entice customers at first glance, and cost-cutting opportunities might catch the eye of corporate leaders, neither is likely to define AI’s impact in the long run. Instead, the technology will become synonymous with behind-the-scenes work that drives tangible improvement at scale.
The end of the honeymoon phase marks the beginning of a more mature relationship with AI, one that requires careful consideration of how it can genuinely enhance customer experiences and drive profitability. Ultimately, the key is to view AI not as a quick fix but as a strategic partner in the pursuit of customer loyalty, satisfying experiences and simple solutions in today’s increasingly complex operations.
In the coming months and years, the organizations that excel will be those that dig deeper, commit to change and recognize AI’s potential as both a short- and long-term investment.
#ADD#ai#AI-powered#Algorithms#Analysis#Analytics#applications#approach#Behavior#behavior data#brands#Business#change#chatGPT#competition#CSG#customer experience#customer experiences#customer loyalty#cutting#data#data collection#databases#development#effects#Environment#Events#excel#eye#factor
0 notes
Text
The Role of Syndicated Reports in Consumer Behavior Analysis

Understanding consumer behavior is crucial for businesses aiming to meet customer needs, improve product offerings, and develop effective marketing strategies. Consumer preferences, motivations, and purchasing habits are constantly evolving due to factors like technological advancements, economic shifts, cultural changes, and market trends. In this dynamic landscape, syndicated reports offer valuable insights into consumer behavior, helping businesses make informed decisions and stay competitive. This article explores how syndicated reports play a critical role in analyzing consumer behavior and how companies can leverage these insights to improve their products, services, and marketing strategies.
1. Identifying Emerging Consumer Trends
Syndicated reports are a vital resource for identifying new and emerging consumer trends. By analyzing data from various markets, industries, and demographic groups, these reports provide a broad view of how consumer preferences are shifting. Businesses can use this information to stay ahead of trends and adapt their offerings to meet changing demands.
Trend Spotting: Syndicated reports often track shifts in consumer preferences across different industries, such as the growing demand for sustainable products, an increasing focus on health and wellness, or the rising influence of digital technologies in purchasing decisions.
Early Indicators: By identifying emerging trends early, businesses can capitalize on new opportunities, such as launching innovative products or services that align with evolving consumer preferences.
Market Segmentation: Syndicated reports break down trends by market segments, such as age, gender, income level, and geographic region. This helps businesses understand which consumer groups are driving specific trends and adjust their strategies accordingly.
For example, a report on the fashion industry might reveal that younger consumers are increasingly interested in eco-friendly clothing, prompting businesses to introduce more sustainable options.
2. Understanding Purchase Drivers and Motivations
Consumer behavior is influenced by a wide range of factors, from emotional and psychological drivers to external influences such as social media, peer recommendations, and economic conditions. Syndicated reports provide in-depth analysis of these purchase drivers and motivations, giving businesses a better understanding of why consumers make certain purchasing decisions.
Psychological Factors: Reports analyze how emotions, attitudes, and perceptions affect consumer choices, helping businesses understand what drives loyalty, brand affinity, and repeat purchases.
Cultural Influences: Syndicated reports explore how cultural factors, such as societal norms or values, influence consumer preferences in different regions or demographic groups.
Economic Drivers: Insights into how economic conditions, such as income levels, inflation, and employment rates, impact purchasing power and consumer spending habits are often included in these reports.
By understanding the underlying motivations behind consumer behavior, businesses can tailor their marketing messages, product positioning, and pricing strategies to better resonate with their target audience.
3. Segmenting Consumer Behavior
Effective consumer behavior analysis requires a deep understanding of different market segments. Consumers within various demographic, psychographic, or geographic categories often exhibit distinct purchasing habits and preferences. Syndicated reports provide detailed segmentation analysis, allowing businesses to focus their efforts on the most relevant consumer groups.
Demographic Segmentation: Syndicated reports categorize consumers based on factors such as age, gender, income, and education level, helping businesses identify which segments are most likely to purchase their products or services.
Geographic Segmentation: By analyzing consumer behavior across different regions, businesses can adapt their strategies to meet local needs and preferences, whether they are expanding into new markets or optimizing their presence in existing ones.
Psychographic Segmentation: Syndicated reports often include insights into the lifestyles, interests, and values of consumers, offering a deeper understanding of what drives their purchasing decisions.
With this segmented data, businesses can create personalized marketing campaigns, design products that cater to specific consumer needs, and enhance customer engagement through targeted messaging.
4. Analyzing Buying Patterns and Habits
Syndicated reports provide businesses with valuable insights into consumer buying patterns, including the frequency, timing, and method of purchases. Understanding these patterns allows businesses to optimize their sales strategies and product offerings to align with consumer habits.
Purchase Frequency: Reports often provide data on how frequently consumers buy certain products or services, helping businesses identify opportunities for cross-selling or upselling.
Preferred Channels: Syndicated reports analyze where consumers make their purchases, whether online, in physical stores, or through a combination of both (omnichannel shopping). This helps businesses optimize their distribution and marketing strategies across different channels.
Seasonal Trends: Reports track seasonal variations in consumer behavior, such as increased spending during holidays or specific times of the year. By identifying these trends, businesses can adjust their inventory, marketing, and promotions to capitalize on peak purchasing periods.
For example, a retailer might use syndicated reports to discover that consumers in a particular region prefer shopping online for specific categories of products, prompting them to enhance their digital presence and offer targeted online promotions.
5. Gauging Brand Perception and Loyalty
A strong brand is essential for long-term business success, and understanding how consumers perceive a brand can provide invaluable insights for improving customer loyalty and engagement. Syndicated reports often include data on consumer opinions, brand sentiment, and loyalty metrics, allowing businesses to gauge how well they are resonating with their target audience.
Brand Awareness: Syndicated reports measure how well-known a brand is within a specific market or demographic, helping businesses understand their visibility and reach.
Brand Affinity: Reports also explore how consumers feel about a brand, whether they associate it with positive attributes such as quality, innovation, or sustainability.
Customer Loyalty: By analyzing data on repeat purchases, customer satisfaction, and Net Promoter Scores (NPS), syndicated reports help businesses identify areas where they can improve customer loyalty and retention.
Armed with this data, companies can refine their brand messaging, improve customer experiences, and invest in strategies that boost long-term loyalty.
6. Measuring the Impact of Digital and Social Media
The digital age has transformed how consumers interact with brands and make purchasing decisions. Social media, e-commerce platforms, and online reviews have become major influencers of consumer behavior. Syndicated reports provide critical insights into how digital channels impact purchasing decisions and how businesses can leverage these platforms to connect with consumers.
Social Media Influence: Syndicated reports often examine how social media platforms, such as Instagram, Facebook, and TikTok, influence consumer preferences and drive purchases. These reports highlight the types of content, influencers, and campaigns that resonate with different audiences.
E-commerce Trends: With the rise of online shopping, syndicated reports provide valuable data on e-commerce trends, such as the most popular platforms, payment methods, and delivery preferences.
Omnichannel Experiences: Reports also analyze how consumers engage with brands across multiple touchpoints, whether online, in-store, or through mobile apps, allowing businesses to optimize their omnichannel strategies for a seamless customer experience.
By understanding how consumers behave in the digital realm, businesses can develop more effective online marketing strategies, enhance their social media presence, and improve their e-commerce offerings.
7. Leveraging Predictive Analytics for Future Trends
One of the key benefits of syndicated reports is their ability to provide predictive analytics. By analyzing historical data and current market trends, syndicated reports can forecast future consumer behavior, helping businesses stay ahead of the curve and anticipate market shifts.
Demand Forecasting: Syndicated reports use data models to predict future demand for products or services, allowing businesses to adjust their production and inventory levels accordingly.
Trend Projections: Reports often project how current consumer trends will evolve over time, enabling businesses to prepare for changes in customer preferences and plan their product development strategies.
Market Opportunities: By identifying untapped market segments or emerging product categories, syndicated reports provide businesses with actionable insights for future growth and expansion.
With these forward-looking insights, businesses can proactively adapt to changing consumer behavior, capitalize on emerging opportunities, and ensure long-term success.
Conclusion
Syndicated reports are an invaluable resource for analyzing consumer behavior and providing businesses with the insights they need to make data-driven decisions. From identifying emerging trends and understanding purchase drivers to segmenting audiences and forecasting future demand, these reports offer a comprehensive view of how consumers interact with products, brands, and markets. By leveraging the insights provided in syndicated reports, businesses can refine their marketing strategies, optimize their product offerings, and better meet the needs of their target customers, ultimately driving growth and enhancing their competitive edge.
Stay ahead of consumer trends and make informed decisions with our comprehensive syndicated reports. Explore our consumer behavior reports to gain valuable insights that drive your business forward.
0 notes
Text
Consumer Behavior Analysis: Insights from Sikkim Manipal University
Hello, curious brains and fans of marketing! Have you ever wondered why you consistently eat the same sort of cereal or why you can’t resist purchasing the newest technology? It is primarily the subject of consumer behavior analysis, and Sikkim Manipal University (SMU) is researching this fascinating subject. Let’s examine some intriguing results from studies and research on consumer behavior…
#Behavioral Analysis#Business Growth#Business Intelligence#Consumer Behavior#Consumer Insights#Consumer Psychology#Customer Experience#Data-Driven#Digital Marketing#Market Research#Market Trends#Marketing Analytics#Marketing Strategy#Marketing Trends#Sikkim Manipal Distance MBA#Strategic Marketing
0 notes
Text
Google Search Results Data Scraping
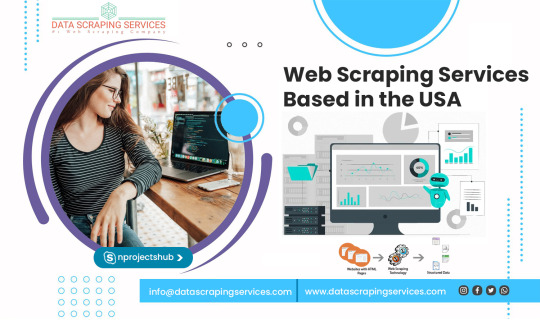
Google Search Results Data Scraping
Harness the Power of Information with Google Search Results Data Scraping Services by DataScrapingServices.com. In the digital age, information is king. For businesses, researchers, and marketing professionals, the ability to access and analyze data from Google search results can be a game-changer. However, manually sifting through search results to gather relevant data is not only time-consuming but also inefficient. DataScrapingServices.com offers cutting-edge Google Search Results Data Scraping services, enabling you to efficiently extract valuable information and transform it into actionable insights.
The vast amount of information available through Google search results can provide invaluable insights into market trends, competitor activities, customer behavior, and more. Whether you need data for SEO analysis, market research, or competitive intelligence, DataScrapingServices.com offers comprehensive data scraping services tailored to meet your specific needs. Our advanced scraping technology ensures you get accurate and up-to-date data, helping you stay ahead in your industry.
List of Data Fields
Our Google Search Results Data Scraping services can extract a wide range of data fields, ensuring you have all the information you need:
-Business Name: The name of the business or entity featured in the search result.
- URL: The web address of the search result.
- Website: The primary website of the business or entity.
- Phone Number: Contact phone number of the business.
- Email Address: Contact email address of the business.
- Physical Address: The street address, city, state, and ZIP code of the business.
- Business Hours: Business operating hours
- Ratings and Reviews: Customer ratings and reviews for the business.
- Google Maps Link: Link to the business’s location on Google Maps.
- Social Media Profiles: LinkedIn, Twitter, Facebook
These data fields provide a comprehensive overview of the information available from Google search results, enabling businesses to gain valuable insights and make informed decisions.
Benefits of Google Search Results Data Scraping
1. Enhanced SEO Strategy
Understanding how your website ranks for specific keywords and phrases is crucial for effective SEO. Our data scraping services provide detailed insights into your current rankings, allowing you to identify opportunities for optimization and stay ahead of your competitors.
2. Competitive Analysis
Track your competitors’ online presence and strategies by analyzing their rankings, backlinks, and domain authority. This information helps you understand their strengths and weaknesses, enabling you to adjust your strategies accordingly.
3. Market Research
Access to comprehensive search result data allows you to identify trends, preferences, and behavior patterns in your target market. This information is invaluable for product development, marketing campaigns, and business strategy planning.
4. Content Development
By analyzing top-performing content in search results, you can gain insights into what types of content resonate with your audience. This helps you create more effective and engaging content that drives traffic and conversions.
5. Efficiency and Accuracy
Our automated scraping services ensure you get accurate and up-to-date data quickly, saving you time and resources.
Best Google Data Scraping Services
Scraping Google Business Reviews
Extract Restaurant Data From Google Maps
Google My Business Data Scraping
Google Shopping Products Scraping
Google News Extraction Services
Scrape Data From Google Maps
Google News Headline Extraction
Google Maps Data Scraping Services
Google Map Businesses Data Scraping
Google Business Reviews Extraction
Best Google Search Results Data Scraping Services in USA
Dallas, Portland, Los Angeles, Virginia Beach, Fort Wichita, Nashville, Long Beach, Raleigh, Boston, Austin, San Antonio, Philadelphia, Indianapolis, Orlando, San Diego, Houston, Worth, Jacksonville, New Orleans, Columbus, Kansas City, Sacramento, San Francisco, Omaha, Honolulu, Washington, Colorado, Chicago, Arlington, Denver, El Paso, Miami, Louisville, Albuquerque, Tulsa, Springs, Bakersfield, Milwaukee, Memphis, Oklahoma City, Atlanta, Seattle, Las Vegas, San Jose, Tucson and New York.
Conclusion
In today’s data-driven world, having access to detailed and accurate information from Google search results can give your business a significant edge. DataScrapingServices.com offers professional Google Search Results Data Scraping services designed to meet your unique needs. Whether you’re looking to enhance your SEO strategy, conduct market research, or gain competitive intelligence, our services provide the comprehensive data you need to succeed. Contact us at [email protected] today to learn how our data scraping solutions can transform your business strategy and drive growth.
Website: Datascrapingservices.com
Email: [email protected]
#Google Search Results Data Scraping#Harness the Power of Information with Google Search Results Data Scraping Services by DataScrapingServices.com. In the digital age#information is king. For businesses#researchers#and marketing professionals#the ability to access and analyze data from Google search results can be a game-changer. However#manually sifting through search results to gather relevant data is not only time-consuming but also inefficient. DataScrapingServices.com o#enabling you to efficiently extract valuable information and transform it into actionable insights.#The vast amount of information available through Google search results can provide invaluable insights into market trends#competitor activities#customer behavior#and more. Whether you need data for SEO analysis#market research#or competitive intelligence#DataScrapingServices.com offers comprehensive data scraping services tailored to meet your specific needs. Our advanced scraping technology#helping you stay ahead in your industry.#List of Data Fields#Our Google Search Results Data Scraping services can extract a wide range of data fields#ensuring you have all the information you need:#-Business Name: The name of the business or entity featured in the search result.#- URL: The web address of the search result.#- Website: The primary website of the business or entity.#- Phone Number: Contact phone number of the business.#- Email Address: Contact email address of the business.#- Physical Address: The street address#city#state#and ZIP code of the business.#- Business Hours: Business operating hours#- Ratings and Reviews: Customer ratings and reviews for the business.
0 notes
Text
Customer Insights: Unravelling Data
Ever wondered how to turn customer data into true understanding? What insights lie hidden in the numbers? Join me on a journey to unravel the mysteries, gaining actionable wisdom for customer-centric success. Your pathway to profound insights begins here! The Pitfall of Relying Solely on DataCracking the Code of Consumer BehaviorThe Human Element in Marketing StrategyStories Over Statistics:…
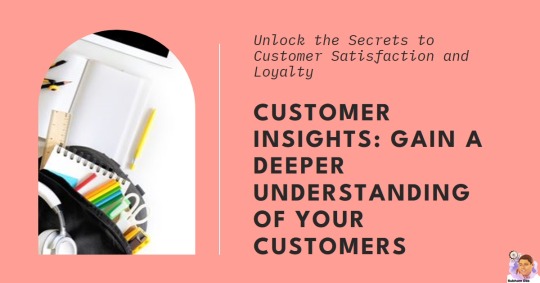
View On WordPress
#artificial intelligence#audience engagement#business growth#business strategy#content creation#customer experience#customer feedback#customer journey#customer relationship#customer satisfaction#data analytics#customer insights#marketing data#customer behavior#data-driven marketing#marketing insights#customer data#business intelligence#digital marketing#data analysis#marketing trends#customer segmentation#data-driven strategies#marketing optimization#big data#data science#marketing performance#customer analytics#customer retention#data visualization
0 notes
Text
How To Target Your Most Profitable Customers With Retail Business Intelligence
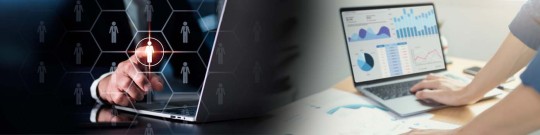
The retail industry is highly competitive, with businesses constantly vying for customer attention and loyalty. To stay ahead of the competition, retailers need to make informed and data-driven decisions. This is where Retail Business Intelligence (BI) comes into play. By harnessing the power of data analysis and advanced analytics, Retail BI enables retailers to identify and target their most profitable customers, optimize marketing strategies, and drive business growth.
#Best BI software for Retail Industry#Retail Business Intelligence#Retail Analytics Solutions#Retail Data Analysis#Retail Performance Metrics#Retail Intelligence Software#Inventory Management Analytics#Customer Behavior Analysis#Predictive Analytics for Retail#Omnichannel Retail Analytics#Business Intelligence Tools for Retail#Retail Dashboard Solutions#Supply Chain Analytics for Retail#Retail Sales Forecasting#Retail Marketing Analytics#Customer Segmentation in Retail#Retail Competitive Intelligence
0 notes
Text
Brand Tracking Guide: Methods, Benefits, and a Case Study
Discover the essential methods and numerous benefits of brand tracking in our comprehensive guide. Learn how to effectively monitor brand performance and make informed decisions to enhance your brand's success.
#Brand tracking#Brand awareness#Brand perception#Brand equity#Market research#Consumer insights#Competitive analysis#Marketing strategy#Consumer behavior#Brand loyalty#Customer satisfaction#Market trends#Data analytics#Performance measurement#Brand positioning#Market intelligence#Tracking metrics#Brand health#Brand image#Brand recall#Purchase intent#Customer engagement#Social media monitoring#Online presence#Customer sentiment#Brand consistency#Trend analysis#Benchmarking#Perception gaps#Target audience
0 notes
Text
Discover how consumer insights in Abu Dhabi are shaping market trends, enhancing customer experiences, and driving data-driven marketing strategies for business success.
#Consumer insights in Abu Dhabi#Consumer Behavior Trends#Market Research Insights#Customer Experience Analysis#Data-Driven Marketing#Consumer Preferences
0 notes
Text
Customer Research Unplugged: Innovative Engagement Tips
Are you truly in tune with your audience? Do you know what keeps them up at night, what drives their decisions, and why they choose your brand over others? Customer research isn’t just a “nice-to-have” anymore—it’s the lifeblood of any business striving to stay ahead. And in the fast-paced, ever-changing world of today’s market, understanding your customer isn’t just about surveying them once a year. It’s about ongoing, real-time engagement and truly listening to their voices.
Imagine having the ability to read your customer’s mind. What if you could tap into their preferences, behaviors, and needs without guessing or assuming? Well, that’s what customer research can offer, and in this blog, we’ll dive deep into the latest, most innovative strategies to make sure you’re not just hearing your customers but understanding them in ways that drive real impact.
So, let’s start with a few questions to get the gears turning:
When was the last time you truly engaged with your customer on a personal level?
Have you ever analyzed customer behavior using data, or do you rely on intuition?
Do you know what motivates your customers to purchase or engage with your brand?
If these questions got you thinking, then it’s time to take a closer look at customer research—and how you can leverage innovative strategies to truly understand and engage your audience.
What is Customer Research and Why Does It Matter?
Customer research, at its core, involves gathering data and insights to understand customer behaviors, needs, and preferences. It’s about answering key questions like:
What do customers want?
What drives their buying decisions?
How can we improve their experience with our brand?
Businesses use customer research to develop better products, improve services, enhance customer satisfaction, and ultimately, boost sales. And here’s the kicker: companies that use customer insights effectively grow faster and build stronger customer loyalty. According to a report by McKinsey, businesses that integrate customer feedback into their strategy are 60% more likely to outperform competitors in terms of profitability and customer satisfaction.
But let’s face it: traditional methods of customer research—like surveys and focus groups—are evolving. The world is moving towards faster, more digital solutions, and businesses need to adapt.
Innovative Customer Research Strategies
So, what innovative strategies can you adopt to dive deeper into customer behavior and ensure you’re engaging your audience effectively? Let’s break it down.
1. Real-Time Feedback with Online Communities
Gone are the days when market research was conducted in isolation. Today, online communities have become a powerful tool for continuous, real-time customer engagement. These communities are groups of engaged customers who actively participate in sharing feedback, answering surveys, and discussing their experiences with your brand.
For instance, brands like Coca-Cola have turned to brand communities to shape their marketing strategies. Coca-Cola created a “Coca-Cola Creations” community where users can submit ideas, vote on new flavors, and provide real-time feedback on products. The result? A more loyal customer base, richer insights, and a greater sense of community.
Real-time feedback through online communities gives you the ability to capture immediate reactions, which allows you to make adjustments in real-time, whether it’s for a product, an ad campaign, or a service offering.
2. Social Listening and Sentiment Analysis
Another innovative approach to understanding your audience is through social listening. Social listening involves monitoring online conversations about your brand, products, or industry to understand customer sentiments and trends. Tools like Hootsuite or Brandwatch help businesses track customer feedback in real-time across platforms like Twitter, Facebook, and Instagram.
Here’s an example: Nike uses social listening to track conversations around athletic wear, sneakers, and even cultural trends. By tapping into customer sentiments, they can align their marketing efforts, respond to potential issues, and even identify emerging trends before their competitors.
With social listening, companies can make their marketing more personalized, reactive, and agile. It’s a strategy that enables brands to truly meet their customers where they are and provide immediate responses.
3. Behavioral Analytics: The Power of Data
What if you could track your customers’ every click, scroll, and purchase decision? Well, with behavioral analytics, you can. Unlike traditional surveys that ask customers about their opinions, behavioral analytics monitors real-time actions, giving you the full picture of how customers interact with your brand.
Amazon is a prime example. The company uses behavioral analytics to recommend products based on past purchases, browsing history, and even the time of day. These tailored recommendations are the result of continuous customer data analysis, which allows Amazon to predict future behavior with remarkable accuracy.
With tools like Google Analytics, Hotjar, and Mixpanel, businesses can track user journeys across websites and apps, identifying pain points, drop-off zones, and areas where customers are most engaged. This level of insight helps businesses refine their user experiences, boost conversions, and drive more targeted marketing campaigns.
4. Predictive Analytics and AI for Trend Spotting
Predictive analytics is one of the most futuristic customer research methods currently available. By using historical data and machine learning algorithms, businesses can predict future behavior with impressive accuracy. AI-powered tools can identify patterns and trends, which can help you stay ahead of the curve.
For example, Spotify uses predictive analytics to recommend playlists and songs based on user listening habits, even before the user knows they’ll like the track. Similarly, Netflix applies predictive algorithms to forecast what shows you’re most likely to watch, keeping you engaged and loyal to the platform.
Predictive analytics isn’t just about forecasting the future—it’s about making data-driven decisions today to improve the customer experience, optimize marketing strategies, and even develop new products that customers didn’t know they needed.
5. Customer Journey Mapping
Understanding your customer’s journey is key to improving their experience. Customer journey mapping involves visualizing the entire customer experience from the first point of contact with your brand all the way to post-purchase interactions. It helps businesses understand pain points, moments of delight, and areas for improvement.
For example, Zappos, the online shoe retailer, has mastered customer journey mapping. They track every step of the customer’s experience, from browsing shoes online to their delivery experience and even returns. This meticulous mapping has helped Zappos create an outstanding customer experience that leads to high levels of satisfaction and loyalty.
Mapping your customer’s journey isn’t just a one-time exercise. It’s an ongoing process that should evolve with customer expectations and market trends.
6. Interactive Surveys and Gamification
Traditional surveys can often feel like a chore, but what if they were fun, engaging, and interactive? That’s where gamification comes in. By turning surveys into a game-like experience, you can capture your customers’ attention and get more detailed responses.
A great example of this comes from Starbucks. The coffee giant regularly uses gamified loyalty programs to engage with customers and encourage repeat purchases. Customers earn rewards and can even unlock achievements by participating in surveys or taking actions that align with Starbucks’ business goals.
Gamifying surveys makes them feel less like a task and more like a reward, leading to better participation rates and more meaningful data.
7. Personalized Email and Mobile Surveys
Not all customers are the same, and today’s businesses must treat each customer as an individual. Personalized email and mobile surveys leverage customer data to tailor surveys to individual preferences and behaviors, making the survey feel more relevant and timely.
For instance, Airbnb sends personalized surveys to customers based on their recent bookings and experiences. These surveys ask questions related to the specific listing or service they used, which increases the likelihood of detailed and actionable feedback.
By sending personalized surveys, businesses not only gather more useful data, but they also show customers that their opinions truly matter.
8. Ethnographic Research: The Deep Dive
Ethnographic research is about immersing yourself in your customers’ world. It’s a qualitative approach where researchers spend time observing and interacting with customers in their natural environment to gain deeper insights into behaviors, attitudes, and motivations.
Conclusion: The Future of Customer Research
In a world where customer preferences are constantly shifting, relying on traditional customer research methods is no longer enough. Brands that leverage innovative strategies—whether it’s real-time feedback, predictive analytics, or gamified surveys—will have a competitive edge in understanding and engaging their audiences.
The key takeaway here is that customer research is a continuous, evolving process. You must adapt to changing technologies, customer expectations, and market conditions. By embracing these innovative strategies, you’ll not only gain a deeper understanding of your audience but also create more personalized, impactful experiences that drive business success.
As you embark on your next customer research project, think of it not as an isolated task but as a strategic, ongoing initiative that empowers you to understand, engage, and ultimately delight your customers in new and exciting ways.
Ready to unlock the full potential of your customer research? Let’s dive deeper together with Philomath Research—we’re here to help you make data-driven decisions that transform your business.
FAQs
What is customer research, and why is it important?
Customer research involves gathering data to understand customer behaviors, needs, and preferences. It’s crucial because it allows businesses to make informed decisions, improve customer satisfaction, develop better products, and drive growth. Effective customer research helps businesses stay competitive by aligning their offerings with what customers truly want.
2. How can online communities help in customer research?
Online communities provide a space for customers to share feedback, ideas, and experiences in real time. This continuous engagement offers valuable insights that allow businesses to adjust their strategies, improve products, and increase customer loyalty. Coca-Cola’s “Coca-Cola Creations” community is a great example of how businesses can tap into real-time feedback for better brand engagement.
3. What is social listening, and how can it benefit my business?
Social listening involves monitoring social media conversations about your brand or industry to understand customer sentiments and trends. By analyzing online conversations, businesses can gain insights into customer opinions, identify potential issues, and spot emerging trends. Brands like Nike use social listening to stay ahead of the curve and refine their marketing strategies based on customer feedback.
4. How does behavioral analytics help in customer research?
Behavioral analytics tracks customer actions, such as clicks, scrolls, and purchases, to understand how customers interact with your website, apps, or services. Unlike traditional surveys, this data gives you a clearer picture of customer behavior in real-time. Amazon uses behavioral analytics to personalize product recommendations based on user history, which enhances customer experience and increases sales.
5. What is predictive analytics, and how can it improve customer research?
Predictive analytics uses historical data and machine learning to forecast future customer behavior. It helps businesses anticipate trends, optimize marketing strategies, and develop products that meet future demand. Companies like Netflix and Spotify use predictive analytics to offer personalized recommendations, keeping customers engaged and loyal.
6. What is customer journey mapping, and why is it important?
Customer journey mapping visualizes the entire experience a customer has with your brand, from initial contact to post-purchase. By mapping each touchpoint, businesses can identify pain points, opportunities for improvement, and areas where they can enhance the customer experience. Zappos, for example, uses journey mapping to ensure a seamless experience from browsing to delivery.
7. How can gamification improve survey participation and data quality?
Gamification turns surveys into engaging, game-like experiences, making them more fun and interactive for customers. This approach encourages higher participation rates and generates more detailed responses. Starbucks uses gamified loyalty programs to boost customer engagement, rewarding them for completing surveys and participating in brand activities.
8. What is the benefit of personalized surveys?
Personalized surveys tailor the questions and experience based on customer data, making them more relevant and timely. By targeting specific customer preferences and behaviors, businesses can gather more actionable insights. For example, Airbnb sends personalized surveys based on customers’ recent bookings to gather more detailed feedback on their experiences.
9. What is ethnographic research, and how can it improve my understanding of customers?
Ethnographic research involves observing and interacting with customers in their natural environment to gain deeper insights into their behaviors, attitudes, and motivations. This qualitative approach helps businesses design products or services that closely match customers’ real-world needs. IKEA is known for conducting ethnographic studies to better understand how people use their furniture in their homes.
10. How can Philomath Research help my business with customer research?
Philomath Research specializes in innovative customer research strategies that help businesses understand and engage their audiences. We use advanced tools like behavioral analytics, social listening, and real-time feedback mechanisms to provide actionable insights that drive business success. Contact us to learn how we can help your business gather meaningful data and make data-driven decisions.
0 notes
Text
How to Leverage RevOps to Overcome Data Inefficiencies and Drive Revenue Within Your Business
New Post has been published on https://thedigitalinsider.com/how-to-leverage-revops-to-overcome-data-inefficiencies-and-drive-revenue-within-your-business/
How to Leverage RevOps to Overcome Data Inefficiencies and Drive Revenue Within Your Business
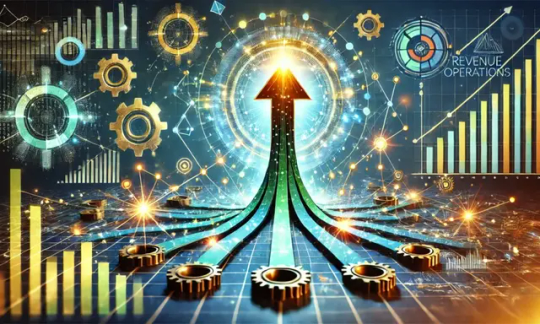
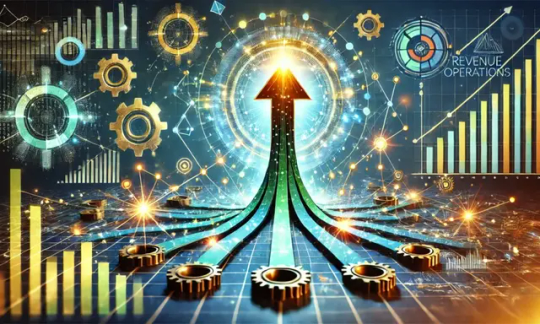
In today’s fast-paced and increasingly complex business landscape, the pressure is on for organizations to drive sustainable growth while maintaining profitability. Traditional siloed approaches to sales, marketing, and customer success often fall short in an era that demands agility, efficiency, and alignment across all revenue-driven functions. Enter Revenue Operations (RevOps), a strategic framework that integrates these critical departments into a unified force aimed at optimizing the entire revenue lifecycle.
Coupled with the rise of artificial intelligence (AI), RevOps is gaining momentum, with 75% of the fastest-growing companies projected to implement a RevOps model by 2026.
But what exactly is RevOps, and how can business leaders leverage it to maximize revenue? In this article, we will explore how AI-driven RevOps technologies can be used to drive revenue growth, streamline operations, and enhance customer experiences.
What is Revenue Operations (RevOps)?
At its core, RevOps is a method that aligns organizational operations such as sales, marketing, and customer success into a seamless system that enhances revenue generation.
Leading businesses, such as Salesforce, Microsoft, Google, IBM, Johnson & Johnson, are dedicating resources towards creating and maintaining RevOps functions in order to optimize their go-to-market approach.
By breaking down departmental silos, RevOps enables more efficient workflows, better decision-making, and improved customer experiences, ultimately driving consistent, sustainable revenue growth. However, what really sets RevOps apart in today’s business world is its ability to leverage cutting-edge AI technologies to drive revenue to new heights, revolutionizing the way RevOps teams operate.
The Power of AI in RevOps
By merging RevOps into AI tools and technologies, businesses can enhance their operational efficiency, make data-driven decisions faster, and drive better outcomes across the revenue generation process. There are many ways that AI does this, here a few examples:
1. Accurate Forecasting
One of the biggest challenges for any business is forecasting revenue accurately. With 80% of leaders reporting that they’ve missed a quarterly forecast and over half missing it multiple times, accurate forecasting is crucial in order to optimize sales teams and take them to the next level. AI-powered predictive analytics analyze historical data and identify patterns to help RevOps teams generate more precise forecasts. This not only provides insight into future revenue streams but also helps identify areas of potential risk or opportunity. Leaders can then take proactive steps to mitigate risks, such as pursuing unqualified leads and setting unrealistic goals, or capitalize on high-growth areas, like customer relationship management (CRM).
2. Automating Repetitive Tasks
Revenue operations often involves a significant amount of manual data entry, reporting, and administrative work. Powered by AI, teams can automate these repetitive tasks, freeing up time to focus on high-value activities like strategy development and customer engagement. By automating routine tasks like data hygiene and pipeline analysis, businesses can improve efficiency and ensure that resources are allocated to areas that drive revenue–like personalized customer outreach, targeted marketing campaigns, and optimizing sales strategies.
3. Sales Pipeline Optimization
AI tools can monitor sales pipelines in real-time to detect any obstructions or inefficiencies that limit success. Whether it’s working to identify stages where deals are stalling or alerting sales teams when customers are showing signs of disengagement, AI provides actionable insights that allow RevOps teams to act quickly. By optimizing the sales pipeline and removing friction, businesses can accelerate the sales cycle and increase revenue conversion rates.
4. Personalizing Customer Interactions
Customer personalization is a critical aspect of RevOps, and AI is transforming the way businesses approach this key function. By analyzing customer data, AI delivers actionable insights into customer preferences, behaviors, and buying patterns. This allows businesses to personalize their outreach,which increases engagement and builds stronger customer relationships. AI-driven personalization plays an important role in optimizing the entire revenue lifecycle, from top of funnel to bottom of the funnel, ultimately driving sustainable revenue growth.
5. AI-Powered Lead Scoring and Opportunity Prioritization
Efficient and effective lead assessment is essential for driving revenue growth. AI can transform the way businesses assess and prioritize these leads by analyzing vast amounts of data points, such as past buying behavior, customer interactions, and demographic information. This enables RevOps teams to identify high-quality prospects with the highest likelihood to buy and optimize resource allocation by focusing efforts on the most lucrative lead sources. This targeted approach allows sales and marketing teams to concentrate efforts on high-value opportunities, ultimately driving better results and maximizing return on interest (ROI).
6. Real-Time Insights for Agile Decision-Making
In today’s dynamic and volatile market landscape, the ability to adapt is vital to growth. AI enables RevOps teams to access real-time data and insights, empowering them to make fast, informed decisions. Whether it’s tweaking marketing campaigns or adjusting sales strategies based on changing customer behaviors, AI helps businesses remain agile and responsive.
Striking the Right Balance: The Future of RevOps
The future of RevOps lies in finding the right balance between the capabilities of AI and the expertise of human teams. As AI continues to evolve, its impact on revenue generation will grow, but the most successful RevOps teams will be those that use AI to optimize routine tasks, gain valuable insights, and personalize customer interactions, while leaving the nuanced, strategic decision-making to human experts. By aligning teams, improving processes, and leveraging the best of both AI and human expertise, RevOps can become the engine that powers long-term growth and profitability.
Business leaders looking to drive maximum revenue in the coming years must invest in building a robust RevOps strategy, enhanced by AI. As AI-driven tools become more accessible and powerful, businesses will become more efficient and uncover new revenue opportunities.
By breaking down silos, improving cross-functional alignment, and automating repetitive tasks, RevOps teams can optimize processes and make smarter, data-driven decisions. However, as AI continues to evolve, its role must be balanced with the critical input of human expertise to ensure that businesses stay agile, customer-focused, and capable of navigating the complexities of an ever-changing marketplace. RevOps—with the support of AI—will be a key driver of consistent and scalable revenue growth in the years to come.
#agile#ai#ai tools#AI-powered#amp#Analysis#Analytics#approach#Article#artificial#Artificial Intelligence#assessment#Behavior#Best Of#Building#Business#Companies#crm#customer data#customer engagement#customer experiences#customer relationship management#customer relationships#cutting#data#data-driven#data-driven decisions#deals#development#driving
0 notes