#BollywoodMovies
Explore tagged Tumblr posts
Text

They don't make soldiers like this anymore.
#telugu#bollywood#tollywood#indiancinema#rrr#india#chiranjeevi#desi#cherrycharam#megapowerstar#ramcharanfans#indians#tollywoodactor#southindian#southindiancinema#telugucinema#bollywoodmovies#magadheera#ramcharan
50 notes
·
View notes
Text









i get so sad when i see my fellow brown girlies gushing over anthony bridgerton when we have THIS
#love#poetry#angst#heartbreak#poem#urdu#lyrics#romance#pakistan#heart#srk#shahrukhkhan#dilse#bollywood#bollywoodquote#Bollywood movie#bollywoodsong#bollywooddialogue#romantic#quote#movie#famous#movies#bollywoodmovies#bollywoodmusic#Spotify
19 notes
·
View notes
Text
aashiqui 2 and jhol. aashiqui 2 and paro. i mean.
'mera koi hor nahi aa sadd ditta duniya nu', 'jadon ve door jaave jaan saddi kadd jaave'. 'tere te main vaari jaavan, tere gun gaavan main'
'ke ab kuch hosh nahi hai, tu mujhko pila degi kya, main pee kar jo bhi kahunga, tu subah bhula degi kya, tu baahon me rakh le do pal, phir chaahe dur haa de, main god me rakh lu agar sar, tu mujhko sula degi kya'
deadly.
#aashiqui 2#shraddha kapoor#aditya roy kapoor#desiblr#desi shit posting#random rants#desi aesthetic#desi stuff#desi tumblr#love#sings#bollywoodsongs#paro#jhol#bollywoodmovies
3 notes
·
View notes
Text
Download Pushpa_2_The_Rule Reloaded version full movie in ORG PRINT.🔥❤
Download Pushpa_2_The_Rule Reloaded version full movie in ORG PRINT.🔥❤
2 notes
·
View notes
Text
'Kanguva' release controversy: Five films of Suriya that were postponed after announcing the release date
Kanguva is one of the highly exciting films in the South Cinema. The upcoming film, featuring Suriya in the lead role and directed by Siva is creating a unique buzz in the industry.
The promotional material have grabbed the interests of the audiences so far and taking the hype to next level, the makers launched the trailer today.
Sharing the trailer, the makers wrote, “The Rise of a King,” on social media.
The trailer introduces the world of Kanguva where many mysteries are hidden. And then, the antagonist Bobby Deol is introduced with his army.
The trailer presents him as a ruthless ruler who will go any extent in the power struggle.
And to put an end to his atrocities, Suriya emerges as the leader and how he battles Bobby Deol forms the story.
The same plot has been envisioned in this amazing trailer cut. The element of revenge is the key for the plot and it has been cleverly induced but kept as an underlying element to hold the suspense.
The film will deal with two different timelines but the present trailer hides the fascinating intrigues of the present timeline.
The clever trailer will satisfy the fans who want Suriya to take up the action mode.
The visuals look grand and Devi Sri Prasad’s score is impressive. Technically, the film looks grand.
Made on a big budget, the film also features Disha Patani,Jagapathi Babu, Natty Natarajan, KS Ravikumar, and Kovai Sarala in pivotal roles. The film is scheduled for a grand release on the 10th of October 2024.
Hindi:- 'कंगुवा' रिलीज विवाद: सूर्या की पांच फिल्में जो रिलीज डेट घोषित होने के बाद स्थगित हो गईं कंगुवा साउथ सिनेमा की बेहद रोमांचक फिल्मों में से एक है।
सूर्या की मुख्य भूमिका वाली और शिवा द्वारा निर्देशित आगामी फिल्म इंडस्ट्री में एक अनोखी चर्चा का विषय बनी हुई है।
प्रचार सामग्री ने अब तक दर्शकों का ध्यान खींचा है और प्रचार को अगले स्तर पर ले जाते हुए निर्माताओं ने आज ट्रेलर लॉन्च किया।
ट्रेलर को शेयर करते हुए निर्माताओं ने सोशल मीडिया पर लिखा, "द राइज ऑफ ए किंग।" ट्रेलर कंगुवा की दुनिया से परिचय कराता है जहां कई रहस्य छिपे हैं।
और फिर, प्रतिपक्षी बॉबी देओल को उसकी सेना के साथ पेश किया जाता है। ट्रेलर में उसे एक क्रूर शासक के रूप में पेश किया गया है जो सत्ता संघर्ष में किसी भी हद तक जा सकता है।
और उसके अत्याचारों को खत्म करने के लिए, सूर्या नेता के रूप में उभरता है और वह बॉबी देओल से कैसे लड़ता है, यही कहानी है। इस अद्भुत ट्रेलर कट में उसी कथानक की कल्पना की गई है।
बदला लेने का तत्व कथानक की कुंजी है और इसे चतुराई से प्रेरित किया गया है, लेकिन रहस्य को बनाए रखने के लिए इसे एक अंतर्निहित तत्व के रूप में रखा गया है।
फिल्म दो अलग-अलग समयसीमाओं से निपटेगी, लेकिन वर्तमान ट्रेलर वर्तमान समयरेखा की आकर्षक साज़िशों को छुपाता है।
चतुर ट्रेलर उन प्रशंसकों को संतुष्ट करेगा जो चाहते हैं कि सूर्या एक्शन मोड में आए। दृश्य भव्य दिखते हैं और देवी श्री प्रसाद का स्कोर प्रभावशाली है। तकनीकी रूप से, फिल्म भव्य दिखती है।
बड़े बजट पर बनी इस फिल्म में दिशा पटानी, जगपति बाबू, नट्टी नटराजन, केएस रविकुमार और कोवई सरला भी प्रमुख भूमिकाओं में हैं। फिल्म 10 अक्टूबर 2024 को भव्य रिलीज के लिए निर्धारित है।
youtube
#aimisentertainment#worldwidemovies#hollywoodmovies#youtube#bollywoodmovies#90sbollywoodmovies#tollywwod#90shollywoodhindidubbed#Youtube
3 notes
·
View notes
Text
Say goodbye to those annoying dents in seconds! 🚗✨ Our Dent Remover from Glowzymart is your go-to solution for a flawless car finish. No more costly repairs—just simple, effective results. 💪 #CarCareMadeEasy #DentRemover #CarCareTips #Glowzymart #AutoDetailing #CarMaintenance #CarLovers #DIYCarRepair #CarCleaning #ViralReel #AutoRepair #CarHacks #CarDetailingIndia #TrendingNow #CarAccessories #CarDetailingProducts #Stree2 #HorrorComedy #BollywoodMovies #IndianCinema #HorrorFilm #ComedyHorror #MovieNight #DesiVibes #Bollywood2024
#CarCareMadeEasy#DentRemover#CarCareTips#Glowzymart#AutoDetailing#CarMaintenance#CarLovers#DIYCarRepair#CarCleaning#ViralReel#AutoRepair#CarHacks#CarDetailingIndia#TrendingNow#CarAccessories#CarDetailingProducts#Stree2#HorrorComedy#BollywoodMovies#IndianCinema#HorrorFilm#ComedyHorror#MovieNight#DesiVibes#Bollywood2024
2 notes
·
View notes
Text
Full notok: Link-1: https://o-trim.co/2wwoGi Link-2: https://o-trim.co/rpanWP
#travis kelce#russia#deadpool#fdom#head in hands#dungeon meshi#fdp#fdstlgd#gentle fdom#fda#urban#musicalmelodies#midnight#loveinthecity#viral#timetraveladventure#Hollywood#southern#shorts#bollywoodmovies#tamil#netflixmovies#hollywoodmovies#disneymovies#kannadamovies#classicmovies#marvelmovies#80smovies#oldmovies#moviestarplanet
6 notes
·
View notes
Text
youtube
#aimisentertainment#southmovies#worldwidemovies#bollywoodmovies#90sbollywoodmovies#80sbollywood#youtube#90shollywoodhindidubbed#hollywoodmovies#chinesemovies#Youtube
2 notes
·
View notes
Video
Tirangaa 1993 HdRip Hindi 720p Worldwide Movies
2 notes
·
View notes
Text
youtube
2 notes
·
View notes
Video
youtube
What's The REAL Difference Between Thai Ghosts And Western Hauntings
1 note
·
View note
Text
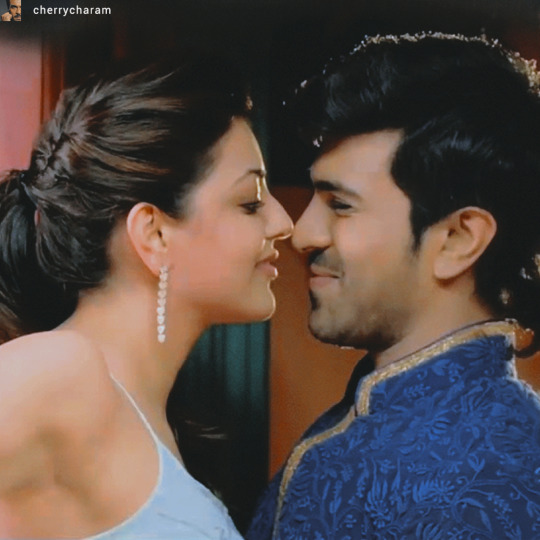
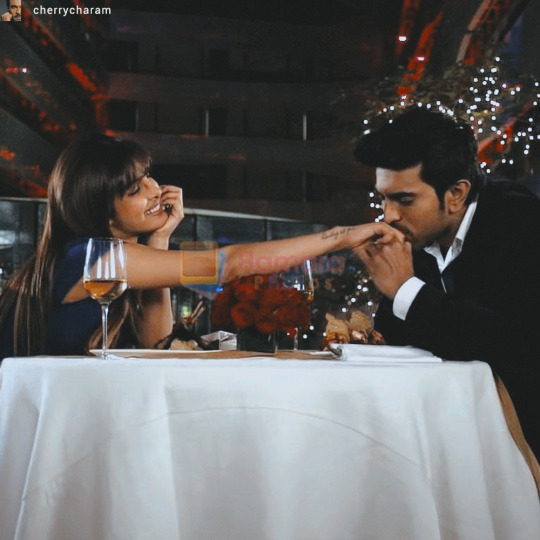
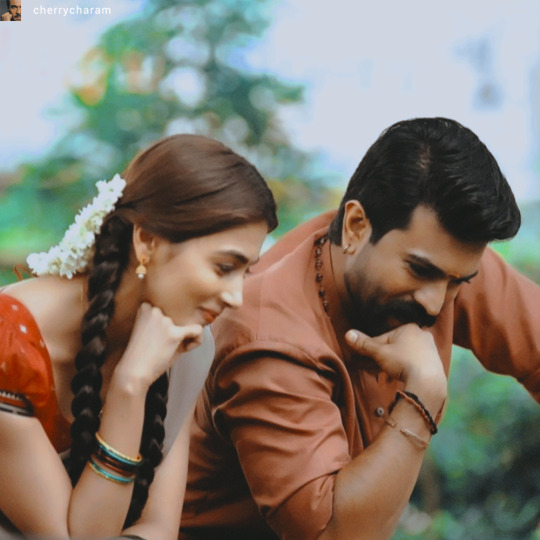
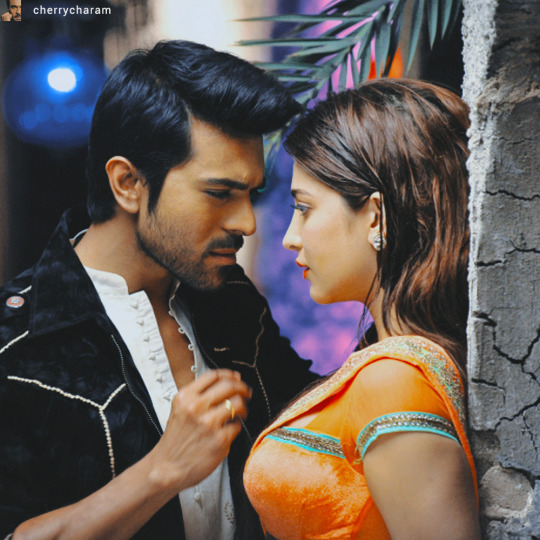
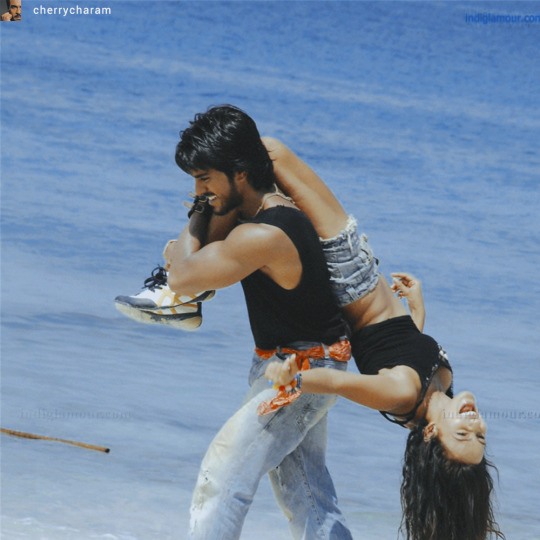
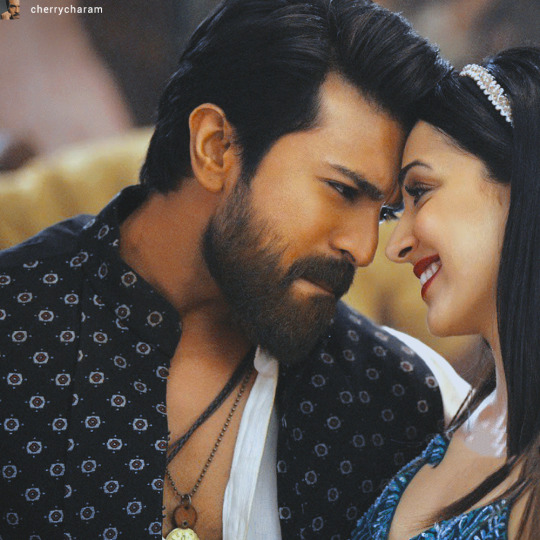

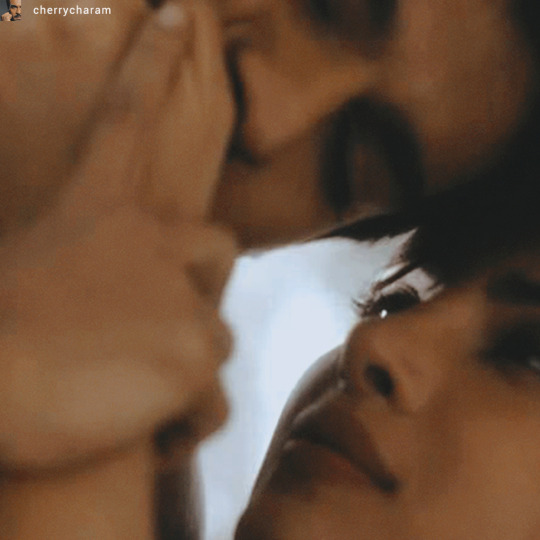
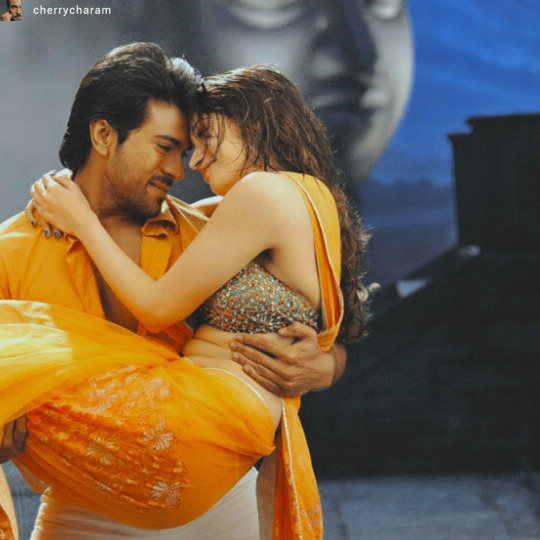
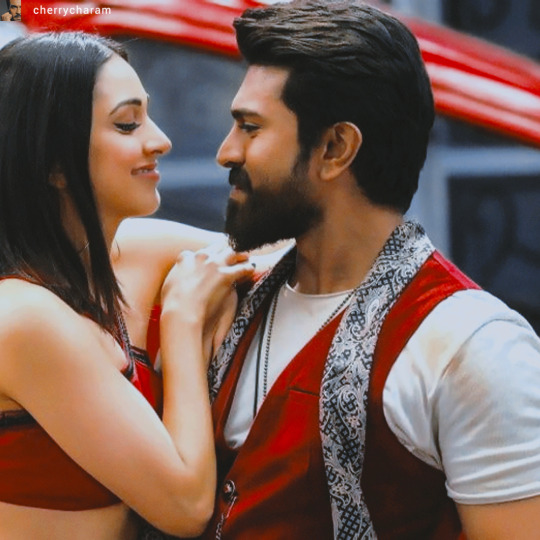
Must be nice.
#ramcharan#telugu#bollywood#tollywood#indiancinema#fitness#rrr#india#chiranjeevi#desi#cherrycharam#megapowerstar#ramcharanfans#indians#tollywoodactor#southindian#southindiancinema#telugucinema#bollywoodmovies#priyankachopra#kiaraadvani#shrutihassan#tamannaah#tamannaahbhatia#poojahegde#kajalagarwal#kajalaggarwal
27 notes
·
View notes
Text
Bollywood Movie Recommendations: Math vs. Machine Learning (Cosine Similarity in Action!)
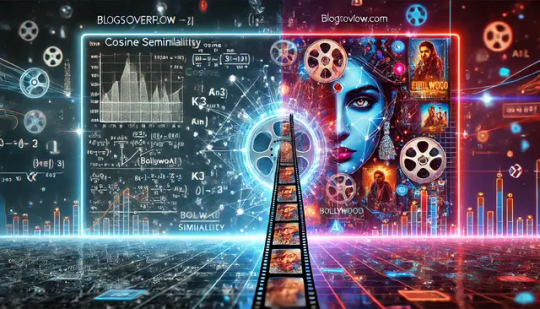
Bollywood Movie Recommendations: Math vs. AI (Cosine Similarity!) Problem Statement: With the ever-growing number of Bollywood movies, users often struggle to find films that match their preferences. Traditional recommendation methods, such as manual curation or simple genre-based filtering, fail to capture nuanced similarities between movies. This blog explores how mathematical approaches like Cosine Similarity and machine learning models can enhance Bollywood movie recommendations by analyzing factors such as genres, actors, directors, and user ratings. We aim to compare the effectiveness of both approaches in delivering accurate and personalized movie suggestions. Solving Bollywood Movie Recommendations Using Math (Cosine Similarity) We will calculate the similarity between two users' movie preferences step by step using pure math, without programming. Step 1: Define User Ratings as Vectors We represent user movie preferences as vectors in n-dimensional space (where each dimension represents a movie). Let's assume two viewers Dinesh & Jyoti watched movies (Fighter, Dunki ,Animal , Sam Bahadur and 12th Fail) and gave rating as below table. And based on this we want to recommend movies to another users Manish. MovieFighter (2024)Dunki (2023)Animal (2023)Sam Bahadur (2023)12th Fail (2023)Dinesh (A)54532Jyoti (B)43421 Dinesh → A = (5, 4, 5, 3, 2) - Called vector A Jyoti → B = (4, 3, 4, 2, 1) - Called vector B Step 2: Apply the Cosine Similarity Formula The formula for cosine similarity between two vectors A and B is: cos(θ) = (A ⋅ B) / (‖A‖ ⋅ ‖B‖) where: - A⋅B = Dot product of A and B - ∣∣A|| = Magnitude (length) of A - |B∣∣ = Magnitude (length) of B Step 3: Compute the Dot Product The dot product of two vectors is: Dinesh → A = (5, 4, 5, 3, 2) - vector A Jyoti → B = (4, 3, 4, 2, 1) - vector B A⋅B = (5×4)+(4×3)+(5×4)+(3×2)+(2×1) = 20+12+20+6+2=60 Step 4: Compute the Magnitudes The magnitude of a vector is: # Magnitude of vector A: ||A|| = sqrt(5² + 4² + 5² + 3² + 2²) = sqrt(25 + 16 + 25 + 9 + 4) = sqrt(79) ≈ 8.89 # Magnitude of vector B: ||B|| = sqrt(4² + 3² + 4² + 2² + 1²) = sqrt(16 + 9 + 16 + 4 + 1) = sqrt(46) ≈ 6.78 Step 5: Compute Cosine Similarity # Step 5: Compute Cosine Similarity cos(θ) = 60 / (8.89 �� 6.78) = 60 / 60.3 ≈ 0.995 Step 6: Interpret the Result Since 0.995 is very close to 1, the two users have very similar movie tastes. 👉 If User 1 liked "Animal", it is very likely that User 2 will also like "Animal", so we recommend it to them. Conclusion: How We Solved This Using Math? - We represented user ratings as vectors. - Used cosine similarity formula. - Computed dot product and magnitudes. - Found a similarity of 0.995, meaning their movie tastes are very close. This is the core math behind Netflix & Prime Video recommendations! 🚀 Would you like me to extend this with real IMDb ratings or explore other machine learning metrics? 🎬 Solving Bollywood Movie Recommendations Using Programming Language Python (Cosine Similarity) from numpy import dot from numpy.linalg import norm # Movie ratings for Dinesh and Jyoti Dinesh = # Fighter, Dunki, Animal, Sam Bahadur, 12th Fail Jyoti = # Compute cosine similarity cosine_similarity = dot(Dinesh, Jyoti) / (norm(Dinesh) * norm(Jyoti)) print("Cosine Similarity:", cosine_similarity) Output : Cosine Similarity: 0.9953109657524404
Why It’s Useful? If the similarity is close to 1, users have similar preferences, and we can recommend movies watched by one user to the other. Now you can see cosine similarity calculation is very easy programmatically. Now we can recommend similar movies to Manish. Conclusion: Bollywood Movie Recommendations Using Math & Machine Learning 🎬📊 In this example, we used mathematical metrics (cosine similarity) to analyze movie preferences and recommend Bollywood films based on user ratings. Key Takeaways: - Mathematics in Recommendations – By treating user movie ratings as vectors and applying cosine similarity, we measured how closely two users' preferences aligned. - Machine Learning in Action – In real-world applications, this concept extends to collaborative filtering in machine learning, where algorithms recommend movies based on patterns in user behavior. - Bollywood Movie Example – If two users rated movies like Animal (2023) and Dunki (2023) similarly, they are likely to enjoy similar future releases like Azaad (2025) or Deva (2025). - Scaling Up – In practical applications (Netflix, Prime Video), machine learning models process large datasets using deep learning (e.g., neural networks) for more accurate, personalized recommendations. By combining mathematical techniques with ML models, recommendation engines enhance user experience by suggesting the best movies based on real data. 🚀🍿 Justification of the Statement: "If two users rated movies like Animal (2023) and Dunki (2023) similarly, they are likely to enjoy similar future releases like Azaad (2025) or Deva (2025)." This statement is justified based on the principles of collaborative filtering and cosine similarity in machine learning and recommendation systems. Here’s why: 1️⃣ Concept of User Similarity in Recommendations - If User A and User B have rated movies like Animal (2023) and Dunki (2023) similarly, their preferences are likely aligned. - When a new movie (Azaad (2025) or Deva (2025)) is released, if one of them likes it, the other user is statistically likely to enjoy it too. This forms the basis of collaborative filtering, where recommendations are made based on patterns in user behavior. 2️⃣ Mathematical Justification: Cosine Similarity We can calculate cosine similarity between users' rating vectors: MovieAnimal (2023)Dunki (2023)Azaad (2025)Deva (2025)User A54??User B5454 If the similarity score (cosine similarity) between User A and User B is close to 1, then their future ratings for Azaad (2025) and Deva (2025) are likely to be very similar. 3️⃣ Real-World Examples: How Netflix and Amazon Prime Use This - Platforms like Netflix, Prime Video, and Hotstar use collaborative filtering + deep learning models to recommend content. - If you like action-thrillers (Animal), the system suggests similar highly-rated action movies (e.g., Deva). - If you like emotional or patriotic movies (Dunki), you may get recommendations for period dramas (Azaad). 4️⃣ Machine Learning Implementation In real-world ML models, we would use: ✅ Collaborative Filtering (User-User or Item-Item Similarity) ✅ Content-Based Filtering (Movie Genre, Cast, Director, etc.) ✅ Hybrid Models (Combining Both Approaches with Deep Learning) For example, a Neural Collaborative Filtering (NCF) model would predict ratings for Azaad (2025) or Deva (2025) based on historical data of similar users. Conclusion ✔️ If two users have similar past preferences, they are more likely to enjoy similar movies in the future. ✔️ This is the core principle behind movie recommendation engines used by Netflix, Prime Video, and YouTube. ✔️ Mathematical metrics (cosine similarity) + Machine Learning (collaborative filtering) provide accurate movie suggestions. Read the full article
#AIinBollywood#Bollywoodmovies#cosinesimilarity#MachineLearning#moviealgorithms#movierecommendations
0 notes
Video
youtube
Chef Cat is now in Dubai to watch belly dancing!! #facebook #facebookpag...
#youtube#dubai#bellydancing#arabiannights#dubai2025#catlover#bollywoodmovies#bollywood2025#bollywoodaction#hollywood2025#hollywoodmovies#hollywoodblockbuster#facebook#facebookpages
0 notes
Text
How "Chhava" Became a Box Office Hit: A Breakdown of Its Winning Strategy
The historical epic Chhava, based on Chhatrapati Sambhaji Maharaj, has taken the box office by storm, proving that a well-executed marketing and promotional strategy can drive massive success. Despite initial controversies around casting Rashmika Mandanna as Yesu Bai, music by A.R. Rahman, and the absence of Marathi actors, the film has managed to captivate audiences nationwide. So, what made…
#ARRahman#ARRMusic#Bollywood#BollywoodMovies#BoxOfficeHit#ChhavaBlockbuster#ChhavaMovie#ChhavaReview#Cinephile#EpicBackgroundScore#FilmDiscussion#HistoricalDrama#IndianCinema#IndianHistory#MarathaWarrior#MarathiHistory#MovieLovers#MovieReview#MovieSoundtrack#MustWatchMovie#PanIndiaFilm#RashmikaMandanna#SambhajiMaharaj#SambhajiMaharajLegacy#VickyKaushal#YesuBai#Digital Marketing
1 note
·
View note