#Automated machine learning
Explore tagged Tumblr posts
Text
Unlocking the Power of Machine Learning in 2024

Discover how machine learning is transforming businesses in 2024. From automated machine learning to explainable AI and edge computing, explore the latest trends and how they can benefit your organization. AquSag Technologies specializes in leveraging machine learning to drive innovation and achieve your goals.
For more information visit : https://aqusag.com/blog/aqusag-technologies-blog-5/machine-learning-in-2024-how-businesses-can-benefit-from-the-latest-trends-69
#machine learning#automated machine learning#explainable AI#edge computing#personalization#ethical AI#data privacy#business innovation#technology trends.
0 notes
Text

The automated machine learning market is experiencing significant growth and is expected to reach USD 15,499.3 million by 2030. This expansion is fueled by the increasing demand for advanced fraud detection solutions, the growing need for personalized product recommendations, and the rising significance of predictive lead scoring.
Cloud computing is widely embraced for its ability to enhance competitiveness through cost efficiency, agility, scalability, and optimized resource usage. Unlike other technologies that can be encapsulated easily, cloud computing consists of multiple components that, when integrated, deliver substantial benefits. It provides access to cloud-native technology, improves operational efficiency, and fosters the development of machine learning (ML) and artificial intelligence (AI). As demand for cloud-based platforms grows, it has become a key driver of market expansion.
Cloud-based solutions, such as Software-as-a-Service (SaaS), allow users to remotely access automated machine learning tools via internet services. This model offers flexibility, scalability, cost-effectiveness, and reduces IT infrastructure expenses.
Fraud detection remains a critical challenge for companies across industries. This need has led to an increased focus on AutoML solutions, as fraud detection systems become more prevalent. For instance, U.S. Federal Agencies were found to have made improper payments totaling USD 247 billion in 2022, with improper payments since 2003 reaching approximately USD 2.4 trillion, further driving the need for AutoML solutions.
0 notes
Text
0 notes
Text
Automated machine learning: The revolution of artificial intelligence
Automated machine learning #AI #Technology #AITech #ML #MachineLearning
What is Automated Machine Learning (AutoML)? What is Automated Machine Learning (AutoML)?How does AI plays a revolutionary role in AutoML?Pros and Cons of AutoMLProsConsAutoML tool featuresFuture PredictionLargest manufacturers of the AutoML marketReal-life case study of AutoMLConclusion TechTarget states, “Automated machine learning (AutoML) is the process of applying machine learning (ML)…
View On WordPress
0 notes
Text
Applying machine learning to process automation
Implementing machine learning in process automation can provide various advantages to businesses. Here, we look at its use cases and benefits for enterprises.
The practice of applying Machine Learning (ML) models to real-world situations through automation is known as Automated Machine Learning. It automates the selection, construction, and parameterization of machine learning models in particular. Since then, Artificial Intelligence (AI) and its subsets have become clever additions to the field of automation, attempting to tackle problems that go beyond simply taking over repetitive tasks.
Machine learning is one form of applied AI that is now being used in Business Process Automation (BPA) and Robotic Process Automation (RPA). Machine learning is the use of artificial intelligence to enable systems to learn and make decisions without being specifically programmed to do so. In RPA, ML can move robots beyond repetitive process execution and enable them to perform jobs that previously needed human decision-making. Artificial Intelligence skills may also be used to improve data integrity, give structure to unstructured and semi-structured data sources, increase business insights, and improve automated execution.
In this blog, we’re taking a closer look at the role of machine learning in automation.
Machine Learning in Process Automation
The applications of machine learning are expanding rapidly to help organizations and society solve real-world issues. ML works on the basis of encapsulating a vast quantity of data (or knowledge) into some form of a mathematical model. The model may be used to solve issues by using the knowledge it contains.
Machine learning can be applied to a wide range of problems where large amounts of historical data can be used to predict or make decisions in specific areas. It should be highlighted that, unlike constructing an algorithm, it is based on the creation of a knowledge base. However, the ML technique is particularly efficient in dealing with such problems.
Process automation has evolved to provide solutions to assist, simplify, and expedite processes. It all started with robotic process automation based on automating step-by-step workflows in a single platform or application. RPA has transformed the way people work today, with millions of bots working alongside humans.
The majority of RPA implementations make use of software bots that automate operations based on pre-defined or established criteria. As corporate processes became more sophisticated, artificial intelligence technology such as machine learning began to impact how bots might accomplish more. With the inclusion of ML, bots continually learn from human activities, emulating human cognition to a great extent. The more training and learning supplied, the closer machines can get to reading, visualizing, learning, and thinking like humans.
How to apply machine learning to process automation
The simplicity with which a workflow or process can be automated is RPA’s biggest strength. However, it has a restriction in that it cannot be automated if it requires decision-making supported by knowledge application. This is where machine learning comes in; it assists in the creation of a knowledge base based on past data, which is then used for decision-making and prediction.
Integrating Machine Learning with robotic process automation can result in strong automation solutions. Many RPA software suppliers are striving to include this functionality inside the tool itself in order to broaden its capabilities. However, because ML is a new subject, it needs the expertise of engineers with extensive knowledge and abilities in order to construct correct ML models.
Improving the Execution of Automation
Algorithms for machine learning can also be used to improve the delivery of automated services. Algorithms can be used in computer vision, for example, to teach robots how to detect and interact with onscreen fields and components. Machine learning models are also employed for error management, and recursion is frequently used to minimize code complexity and optimize robot runtime.
Another growing use of machine learning in automation is task mining. In this case, robots are taught to assess daily task information obtained from employees in order to create process maps and recommend procedures for automation based on the maximum return on investment (ROI.) This application can be a mixed bag since it needs extensive training to reach the proper balance between ROI, degree of effort, and overall suitability for automation.
Attended Automation
Attended automation, also known as Remote Desktop Automation (RDA), is when robots work alongside people to augment their job or help them make better decisions. Machine learning may be used to absorb data from several sources in real-time, allowing robots to assist humans in determining the optimal next step in their workflow.
Machine learning may also be integrated with other cognitive skills, such as Natural Language Processing (NLP), to allow robots to emulate the easier decision-making inside a human’s workflow, bringing us even closer to end-to-end automation.
Conclusion
From the above breakdown of process automation technologies, we can surmise that investing in machine learning to augment BPA and RPA can provide various advantages to businesses. It is a powerful and continuously evolving technology, and its ramifications will only grow in the future years. As a result, it is a worthwhile improvement over manual processes and a crucial step towards helping your teams become more productive. Machine learning algorithms can help to reduce risk and eliminate the cost of operating clunky, outdated software.
Reimagine business efficiency with automation and data solutions built by VBeyond Digital.
Get in touch to speak with our experts.
0 notes
Text
The surprising truth about data-driven dictatorships
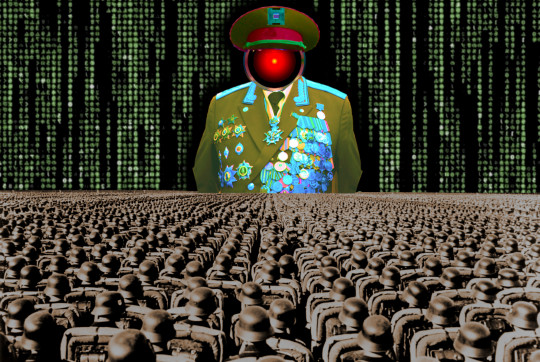
Here’s the “dictator’s dilemma”: they want to block their country’s frustrated elites from mobilizing against them, so they censor public communications; but they also want to know what their people truly believe, so they can head off simmering resentments before they boil over into regime-toppling revolutions.
These two strategies are in tension: the more you censor, the less you know about the true feelings of your citizens and the easier it will be to miss serious problems until they spill over into the streets (think: the fall of the Berlin Wall or Tunisia before the Arab Spring). Dictators try to square this circle with things like private opinion polling or petition systems, but these capture a small slice of the potentially destabiziling moods circulating in the body politic.
Enter AI: back in 2018, Yuval Harari proposed that AI would supercharge dictatorships by mining and summarizing the public mood — as captured on social media — allowing dictators to tack into serious discontent and diffuse it before it erupted into unequenchable wildfire:
https://www.theatlantic.com/magazine/archive/2018/10/yuval-noah-harari-technology-tyranny/568330/
Harari wrote that “the desire to concentrate all information and power in one place may become [dictators] decisive advantage in the 21st century.” But other political scientists sharply disagreed. Last year, Henry Farrell, Jeremy Wallace and Abraham Newman published a thoroughgoing rebuttal to Harari in Foreign Affairs:
https://www.foreignaffairs.com/world/spirals-delusion-artificial-intelligence-decision-making
They argued that — like everyone who gets excited about AI, only to have their hopes dashed — dictators seeking to use AI to understand the public mood would run into serious training data bias problems. After all, people living under dictatorships know that spouting off about their discontent and desire for change is a risky business, so they will self-censor on social media. That’s true even if a person isn’t afraid of retaliation: if you know that using certain words or phrases in a post will get it autoblocked by a censorbot, what’s the point of trying to use those words?
The phrase “Garbage In, Garbage Out” dates back to 1957. That’s how long we’ve known that a computer that operates on bad data will barf up bad conclusions. But this is a very inconvenient truth for AI weirdos: having given up on manually assembling training data based on careful human judgment with multiple review steps, the AI industry “pivoted” to mass ingestion of scraped data from the whole internet.
But adding more unreliable data to an unreliable dataset doesn’t improve its reliability. GIGO is the iron law of computing, and you can’t repeal it by shoveling more garbage into the top of the training funnel:
https://memex.craphound.com/2018/05/29/garbage-in-garbage-out-machine-learning-has-not-repealed-the-iron-law-of-computer-science/
When it comes to “AI” that’s used for decision support — that is, when an algorithm tells humans what to do and they do it — then you get something worse than Garbage In, Garbage Out — you get Garbage In, Garbage Out, Garbage Back In Again. That’s when the AI spits out something wrong, and then another AI sucks up that wrong conclusion and uses it to generate more conclusions.
To see this in action, consider the deeply flawed predictive policing systems that cities around the world rely on. These systems suck up crime data from the cops, then predict where crime is going to be, and send cops to those “hotspots” to do things like throw Black kids up against a wall and make them turn out their pockets, or pull over drivers and search their cars after pretending to have smelled cannabis.
The problem here is that “crime the police detected” isn’t the same as “crime.” You only find crime where you look for it. For example, there are far more incidents of domestic abuse reported in apartment buildings than in fully detached homes. That’s not because apartment dwellers are more likely to be wife-beaters: it’s because domestic abuse is most often reported by a neighbor who hears it through the walls.
So if your cops practice racially biased policing (I know, this is hard to imagine, but stay with me /s), then the crime they detect will already be a function of bias. If you only ever throw Black kids up against a wall and turn out their pockets, then every knife and dime-bag you find in someone’s pockets will come from some Black kid the cops decided to harass.
That’s life without AI. But now let’s throw in predictive policing: feed your “knives found in pockets” data to an algorithm and ask it to predict where there are more knives in pockets, and it will send you back to that Black neighborhood and tell you do throw even more Black kids up against a wall and search their pockets. The more you do this, the more knives you’ll find, and the more you’ll go back and do it again.
This is what Patrick Ball from the Human Rights Data Analysis Group calls “empiricism washing”: take a biased procedure and feed it to an algorithm, and then you get to go and do more biased procedures, and whenever anyone accuses you of bias, you can insist that you’re just following an empirical conclusion of a neutral algorithm, because “math can’t be racist.”
HRDAG has done excellent work on this, finding a natural experiment that makes the problem of GIGOGBI crystal clear. The National Survey On Drug Use and Health produces the gold standard snapshot of drug use in America. Kristian Lum and William Isaac took Oakland’s drug arrest data from 2010 and asked Predpol, a leading predictive policing product, to predict where Oakland’s 2011 drug use would take place.
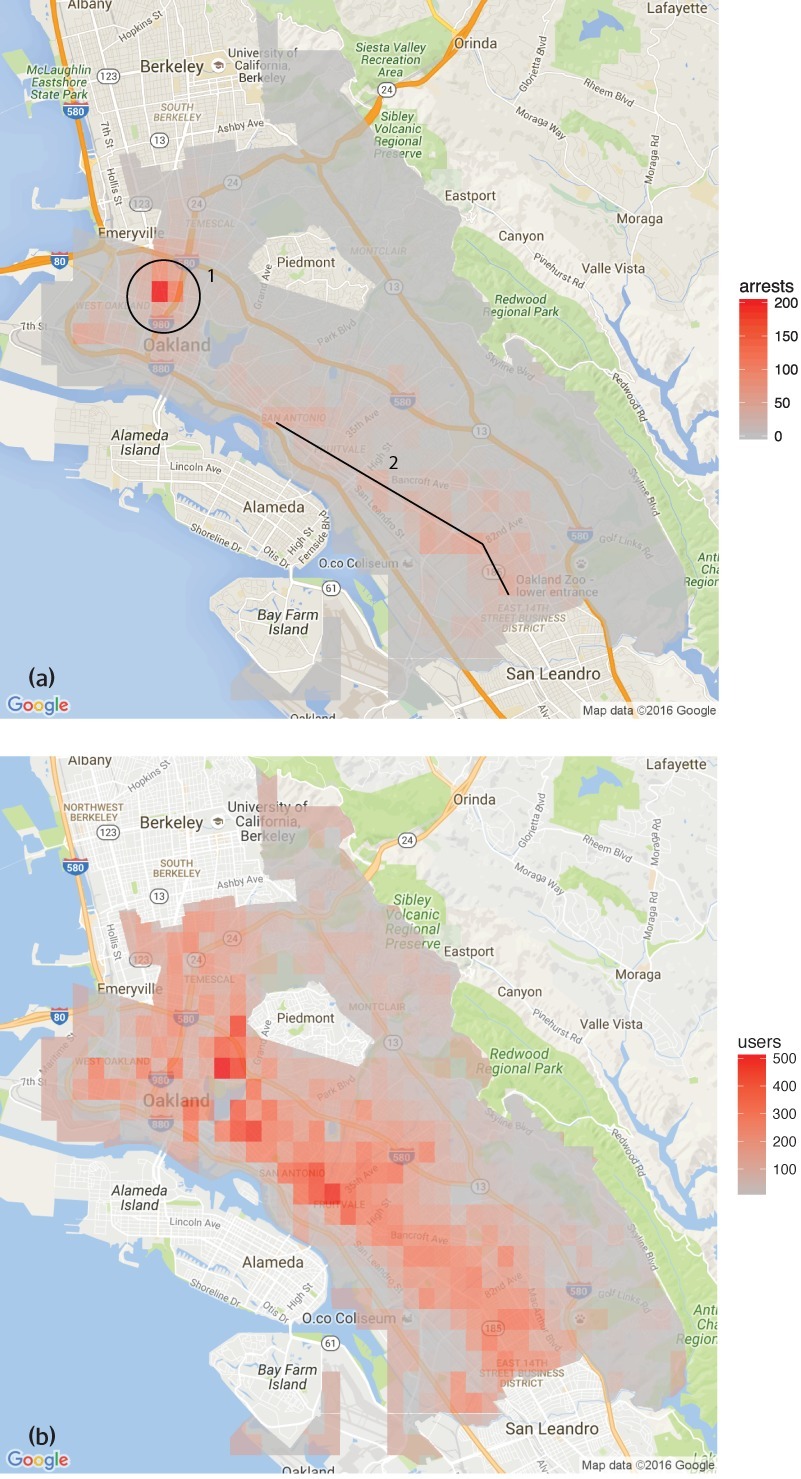
[Image ID: (a) Number of drug arrests made by Oakland police department, 2010. (1) West Oakland, (2) International Boulevard. (b) Estimated number of drug users, based on 2011 National Survey on Drug Use and Health]
Then, they compared those predictions to the outcomes of the 2011 survey, which shows where actual drug use took place. The two maps couldn’t be more different:
https://rss.onlinelibrary.wiley.com/doi/full/10.1111/j.1740-9713.2016.00960.x
Predpol told cops to go and look for drug use in a predominantly Black, working class neighborhood. Meanwhile the NSDUH survey showed the actual drug use took place all over Oakland, with a higher concentration in the Berkeley-neighboring student neighborhood.
What’s even more vivid is what happens when you simulate running Predpol on the new arrest data that would be generated by cops following its recommendations. If the cops went to that Black neighborhood and found more drugs there and told Predpol about it, the recommendation gets stronger and more confident.
In other words, GIGOGBI is a system for concentrating bias. Even trace amounts of bias in the original training data get refined and magnified when they are output though a decision support system that directs humans to go an act on that output. Algorithms are to bias what centrifuges are to radioactive ore: a way to turn minute amounts of bias into pluripotent, indestructible toxic waste.
There’s a great name for an AI that’s trained on an AI’s output, courtesy of Jathan Sadowski: “Habsburg AI.”
And that brings me back to the Dictator’s Dilemma. If your citizens are self-censoring in order to avoid retaliation or algorithmic shadowbanning, then the AI you train on their posts in order to find out what they’re really thinking will steer you in the opposite direction, so you make bad policies that make people angrier and destabilize things more.
Or at least, that was Farrell(et al)’s theory. And for many years, that’s where the debate over AI and dictatorship has stalled: theory vs theory. But now, there’s some empirical data on this, thanks to the “The Digital Dictator’s Dilemma,” a new paper from UCSD PhD candidate Eddie Yang:
https://www.eddieyang.net/research/DDD.pdf
Yang figured out a way to test these dueling hypotheses. He got 10 million Chinese social media posts from the start of the pandemic, before companies like Weibo were required to censor certain pandemic-related posts as politically sensitive. Yang treats these posts as a robust snapshot of public opinion: because there was no censorship of pandemic-related chatter, Chinese users were free to post anything they wanted without having to self-censor for fear of retaliation or deletion.
Next, Yang acquired the censorship model used by a real Chinese social media company to decide which posts should be blocked. Using this, he was able to determine which of the posts in the original set would be censored today in China.
That means that Yang knows that the “real” sentiment in the Chinese social media snapshot is, and what Chinese authorities would believe it to be if Chinese users were self-censoring all the posts that would be flagged by censorware today.
From here, Yang was able to play with the knobs, and determine how “preference-falsification” (when users lie about their feelings) and self-censorship would give a dictatorship a misleading view of public sentiment. What he finds is that the more repressive a regime is — the more people are incentivized to falsify or censor their views — the worse the system gets at uncovering the true public mood.
What’s more, adding additional (bad) data to the system doesn’t fix this “missing data” problem. GIGO remains an iron law of computing in this context, too.
But it gets better (or worse, I guess): Yang models a “crisis” scenario in which users stop self-censoring and start articulating their true views (because they’ve run out of fucks to give). This is the most dangerous moment for a dictator, and depending on the dictatorship handles it, they either get another decade or rule, or they wake up with guillotines on their lawns.
But “crisis” is where AI performs the worst. Trained on the “status quo” data where users are continuously self-censoring and preference-falsifying, AI has no clue how to handle the unvarnished truth. Both its recommendations about what to censor and its summaries of public sentiment are the least accurate when crisis erupts.
But here’s an interesting wrinkle: Yang scraped a bunch of Chinese users’ posts from Twitter — which the Chinese government doesn’t get to censor (yet) or spy on (yet) — and fed them to the model. He hypothesized that when Chinese users post to American social media, they don’t self-censor or preference-falsify, so this data should help the model improve its accuracy.
He was right — the model got significantly better once it ingested data from Twitter than when it was working solely from Weibo posts. And Yang notes that dictatorships all over the world are widely understood to be scraping western/northern social media.
But even though Twitter data improved the model’s accuracy, it was still wildly inaccurate, compared to the same model trained on a full set of un-self-censored, un-falsified data. GIGO is not an option, it’s the law (of computing).
Writing about the study on Crooked Timber, Farrell notes that as the world fills up with “garbage and noise” (he invokes Philip K Dick’s delighted coinage “gubbish”), “approximately correct knowledge becomes the scarce and valuable resource.”
https://crookedtimber.org/2023/07/25/51610/
This “probably approximately correct knowledge” comes from humans, not LLMs or AI, and so “the social applications of machine learning in non-authoritarian societies are just as parasitic on these forms of human knowledge production as authoritarian governments.”
The Clarion Science Fiction and Fantasy Writers’ Workshop summer fundraiser is almost over! I am an alum, instructor and volunteer board member for this nonprofit workshop whose alums include Octavia Butler, Kim Stanley Robinson, Bruce Sterling, Nalo Hopkinson, Kameron Hurley, Nnedi Okorafor, Lucius Shepard, and Ted Chiang! Your donations will help us subsidize tuition for students, making Clarion — and sf/f — more accessible for all kinds of writers.
Libro.fm is the indie-bookstore-friendly, DRM-free audiobook alternative to Audible, the Amazon-owned monopolist that locks every book you buy to Amazon forever. When you buy a book on Libro, they share some of the purchase price with a local indie bookstore of your choosing (Libro is the best partner I have in selling my own DRM-free audiobooks!). As of today, Libro is even better, because it’s available in five new territories and currencies: Canada, the UK, the EU, Australia and New Zealand!
[Image ID: An altered image of the Nuremberg rally, with ranked lines of soldiers facing a towering figure in a many-ribboned soldier's coat. He wears a high-peaked cap with a microchip in place of insignia. His head has been replaced with the menacing red eye of HAL9000 from Stanley Kubrick's '2001: A Space Odyssey.' The sky behind him is filled with a 'code waterfall' from 'The Matrix.']
Image: Cryteria (modified) https://commons.wikimedia.org/wiki/File:HAL9000.svg
CC BY 3.0 https://creativecommons.org/licenses/by/3.0/deed.en
—
Raimond Spekking (modified) https://commons.wikimedia.org/wiki/File:Acer_Extensa_5220_-_Columbia_MB_06236-1N_-_Intel_Celeron_M_530_-_SLA2G_-_in_Socket_479-5029.jpg
CC BY-SA 4.0 https://creativecommons.org/licenses/by-sa/4.0/deed.en
—
Russian Airborne Troops (modified) https://commons.wikimedia.org/wiki/File:Vladislav_Achalov_at_the_Airborne_Troops_Day_in_Moscow_%E2%80%93_August_2,_2008.jpg
“Soldiers of Russia” Cultural Center (modified) https://commons.wikimedia.org/wiki/File:Col._Leonid_Khabarov_in_an_everyday_service_uniform.JPG
CC BY-SA 3.0 https://creativecommons.org/licenses/by-sa/3.0/deed.en
#pluralistic#habsburg ai#self censorship#henry farrell#digital dictatorships#machine learning#dictator's dilemma#eddie yang#preference falsification#political science#training bias#scholarship#spirals of delusion#algorithmic bias#ml#Fully automated data driven authoritarianism#authoritarianism#gigo#garbage in garbage out garbage back in#gigogbi#yuval noah harari#gubbish#pkd#philip k dick#phildickian
832 notes
·
View notes
Photo
What is an Algorithm in 30 Seconds?
An algorithm is simply a series of instructions.
Think of a recipe: boil water, add pasta, wait, drain, eat. These are steps to follow.
In computer terms, an algorithm is a set of instructions for a computer to execute.
In machine learning, these instructions enable computers to learn from data, making machine learning algorithms unique and powerful.
#artificial intelligence#automation#machine learning#business#digital marketing#professional services#marketing#web design#web development#social media#tech#Technology
67 notes
·
View notes
Text
So, I made a tool to stop AI from stealing from writers
So seeing this post really inspired me in order to make a tool that writers could use in order to make it unreadable to AI.
And it works! You can try out the online demo, and view all of the code that runs it here!
It does more than just mangle text though! It's also able to invisibly hide author and copyright info, so that you can have definitive proof that someone's stealing your works if they're doing a simple copy and paste!
Below is an example of Scrawl in action!
Τо հսⅿаոѕ, 𝗍հᎥꜱ 𝗍ех𝗍 𐌉ο໐𝗄ꜱ ո໐𝗋ⅿаⵏ, 𝖻ս𝗍 𝗍о ᴄоⅿрս𝗍е𝗋ꜱ, Ꭵ𝗍'ѕ սո𝗋еаⅾа𝖻ⵏе!
[Text reads "To humans, this text looks normal, but to computers, it's unreadable!"]
Of course, this "Anti-AI" mode comes with some pretty serious accessibility issues, like breaking screen readers and other TTS software, but there's no real way to make text readable to one AI but not to another AI.
If you're okay with it, you can always have Anti-AI mode off, which will make it so that AIs can understand your text while embedding invisible characters to save your copyright information! (as long as the website you're posting on doesn't remove those characters!)
But, the Anti-AI mode is pretty cool.
#also just to be clear this isn't a magical bullet or anything#people can find and remove the invisible characters if they know they're there#and the anti-ai mode can be reverted by basically taking scrawl's code and reversing it#but for webscrapers that just try to download all of your works or automated systems they wont spend the time on it#and it will mess with their training >:)#ai writing#anti ai writing#ai#machine learning#artificial intelligence#chatgpt#writers
351 notes
·
View notes
Text
Really excited for the potential of unionized CGI effects crews. Imagine if we could get back the amazing CGI / practical effects combination from Jurassic Park. Delightful, magical.
#it would be hilarious if the push to automate art with machine learning and maximize profitable streaming#was the push that led to the entire industry locking down attempts to trear digital likeness as property#brought back everything on physical media#etc#idk I'm tired and miss when big movies looked nice sometimes
100 notes
·
View notes
Text
I'll protect you from all the things I've seen.
#a.b.e.l#divine machinery#archangel#automated#behavioral#ecosystem#learning#divine#machinery#ai#artificial intelligence#crystal castles#kerosene#lyrics#lyric posting#lyric quotes#angel#angels#guardian angel#robot#android#computer#machine#sentient ai#i love you
7 notes
·
View notes
Photo
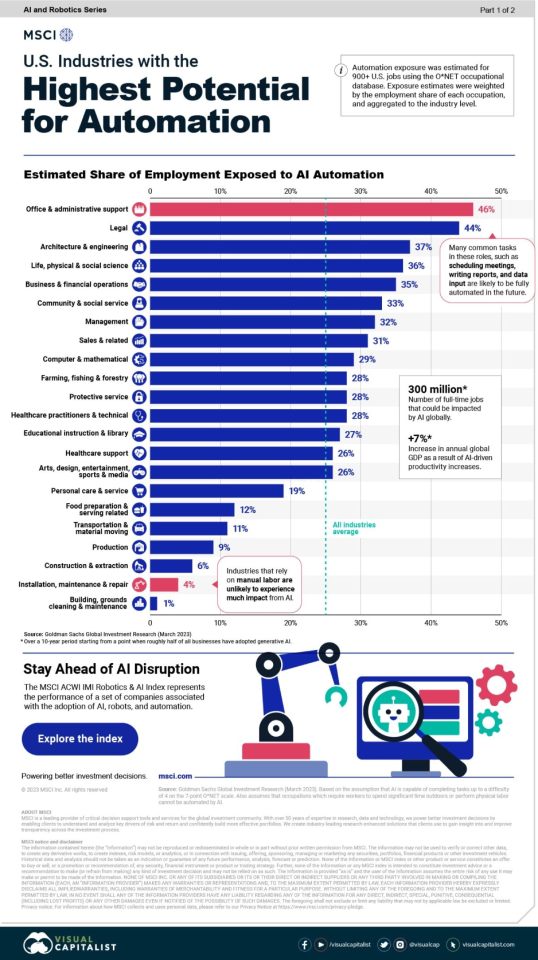
▸ Top 3 industries that are most prone to AI automation are: office support, legal, and engineering.
▸ AI automation could impact up to 300 million jobs globally, and potentially result in a 7% increase in annual GDP.
(via Industries with the highest potential for AI (infographic))
54 notes
·
View notes
Text
AUTOMATIC AUTOMATION DEFENSIVE SYSTEMS USED AGAINST DEFINITE AND KNOWN TIME TRAVELERS AND THEIR ASSOCIATES
#AUTOMATIC AUTOMATION DEFENSIVE SYSTEMS#AUTOMATON#AUTOMATIC AUTOMATION DEFENSIVE SYSTEMS USED AGAINST DEFINITE AND KNOWN TIME TRAVELERS AND THEIR ASSOCIATES#robots#self-driving cars#deep learning#machine learning#drones#artificial intelligence#technology#culture#history#TIME TRAVEL#TEXT#TXT#txt#text
6 notes
·
View notes
Text
Automated Machine Learning (AutoML) Industry Growth Forecast to 2030
According to the latest market research study published by P&S Intelligence, the automated machine learning market is witnessing growth and is projected to reach 15,499.3 million by 2030. The market is driven by the growing need for effective scam recognition solutions, the rising requirement for tailored product recommendations, and the increasing importance of predictive lead scoring. Cloud…

View On WordPress
#Automated Machine Learning#AutoML#Fraud Detection#Medical Testing#Sales & Marketing Management#Transport Optimization
0 notes
Text
The most awaited article of the year is here ... Evolution of automation is all yours now !!!
#aerospace#automation#machine learning#artificial intelligence#success#inspiring quotes#architecture#article#trending#viral#viral trends#market trends#viralpost#automotive#automatically generated text#software#engineering#search engine optimization
9 notes
·
View notes
Text
youtube
Revolutionizing Small Business with Generative AI Unlocking Growth and Success
#SmallBusiness#GenerativeAI#AIForBusiness#Innovation#Automation#CustomerExperience#BusinessGrowth#TechTrends#digitaltransformation#animation#art#artists on tumblr#artificial intelligence#branding#accounting#artwork#machine learning#architecture#youtube#Youtube
3 notes
·
View notes
Text
periodically people have to be reminded that you should be against these large generative ai models because its aggregate content theft and not because of like "laziness" or whatever
#like yes the arts are wildly devalued which#goes in hand with this push to replace us en masse with ai to cut labour costs#and its disgusting to see#but if someone says its making art accessible to disabled people. and you talk about a guy who learned to draw with his feet#you need to take a good hard look at yourself#it feels bad to encourage the automation of art when in popular culture so much of our work is devalued but#as an artist!!! i am lazy. i use 3d models i trace photos i use custom brushes#sometimes you just aint drawing all that. the Laziness thing is a bad argument#whats bad is feeling entitled to Take the work of others without permission to train generative models!!! thats the problem!!!#just bc you're taking stuff en masse it doesnt make it better than stealing from one person. thats not the same thng as inspiration shut up#i think technology and tools evolve and its cool that I've found thinvs that support me as an artist#its not fucking about the machine learning its about theft how many times do we have to teach you this lesson
7 notes
·
View notes