#AI Inference Solutions
Explore tagged Tumblr posts
Text
d-Matrix delivers groundbreaking AI hardware solutions, specializing in digital in-memory computing for efficient, scalable AI inference. Our cutting-edge technology, including the Corsair AI platform and advanced AI chiplets, accelerates large language models and generative AI applications, significantly reducing energy consumption and enhancing performance. Ideal for businesses aiming to deploy AI at scale, d-Matrix optimizes infrastructure with innovation in AI accelerator chips and compute platforms. Experience next-generation AI inference solutions with d-Matrix, where we redefine efficiency and performance in AI processing.
Website: https://www.d-matrix.ai/
Address: 5201 Great America Pkwy, Santa Clara, CA, 95054
Phone Number: 1-888-244-1173
1 note
·
View note
Text
Optimized AI infrastructure for training and inference workloads
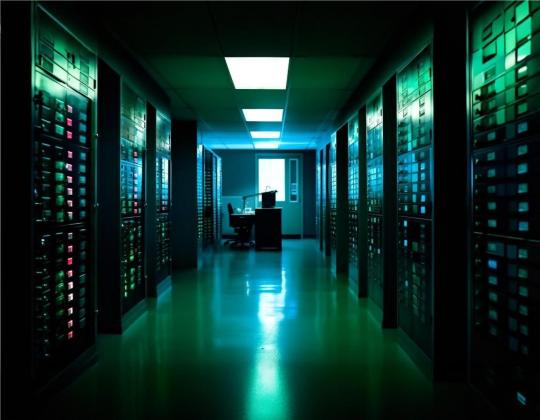
AI Infrastructure Solutions: The Nerve Centre of State-of-the-Art AI Development
Artificial Intelligence (AI) is fast-changing today. To keep abreast, businesses and researchers require solid and effective systems that will support models in AI, especially for deep learning, machine learning, and data analysis. Such a system comes in the form of advanced AI infrastructure solutions.
AI infrastructure refers to the underlying hardware and software stack that is the foundation upon which AI workloads can be deployed and optimized. Indeed, be it deep-learning model training or inference work, proper infrastructure will be a determinant.
In this blog post, we'll walk you through the importance of high-performance AI infrastructure and how to optimize your AI workloads with the right setup. From GPU-powered solutions to deep learning-focused infrastructure, we will outline the essentials you need to know.
What is High-Performance AI Infrastructure?
High-performance AI infrastructure refers to the combination of advanced hardware and software optimized for handling intensive AI tasks. These tasks, such as training deep learning models, require immense computational power. Traditional computer systems often struggle with these demands, so specialized infrastructure is needed.
Key components of high-performance AI infrastructure include:
- Powerful GPUs:
These are built to support the parallel computation requirements of AI tasks and are much faster than a traditional CPU.
- Massive Storage:
AI models generate and process vast amounts of data, so they need fast and scalable storage solutions.
- Networking and Communication:
High-speed connections between AI systems are necessary to ensure data flows efficiently during training and inference processes.
By utilizing high-performance infrastructure, AI tasks can be completed much faster, enabling businesses to innovate more quickly and accurately.
How Can AI Workload Optimization Services Help Your Business?
AI workload optimization services are essential for improving the efficiency and effectiveness of AI processes. These services ensure that AI workloads—like data processing, model training, and inference—are managed in the most optimized manner possible.
Through AI workload optimization, businesses can:
- Reduce Processing Time:
The right infrastructure and effective management of workloads help reduce the time taken to train AI models and make predictions.
- Improve Resource Utilization:
Optimized AI workloads ensure that every bit of computing power is used effectively, thereby minimizing waste and improving overall performance.
- Cost Savings:
Through the adjustment of the performance and resource consumption of AI systems, firms reduce unutilized hardware expenses and power consumption.
Optimization of workloads, for example, becomes even more efficient in utilizing high-performance AI infrastructure to its full extent since it offers companies the possibility of reaping maximum rewards from advanced computing systems.
Why Is AI Infrastructure Necessary For Deep Learning?
Deep learning, as the name suggests, falls under machine learning and utilizes the training of models on extensive datasets by multiple layers of processing. Because deep learning models are huge and complex in their infrastructure, they require proper infrastructure.
The AI infrastructure in deep learning is made of powerful high-performance servers, containing ample storage for huge data and processing heavy computational processes. In the absence of this infrastructure, deep learning projects get slow and inefficient, becoming cost-prohibitive as well.
With AI infrastructure specifically built for deep learning, businesses can train:
- More Complex Models:
Deep learning models - neural networks and their analogs - require big amounts of data and computing power for the real training process. Such infrastructures ensure the proper design and refinement of models with appropriate speed.
- Scalable AI Projects:
Deep learning models are always changing and demand more computing power and storage. Scalable infrastructure will make it easy for companies to scale their capabilities to match increasing demands.
GPU-Powered AI Infrastructure: Accelerating Your AI Capabilities
The training and deployment of AI models will be sped up with the help of GPU-powered infrastructure. The parallel processing algorithms that are required in machine learning and deep learning work better on GPUs than on CPUs due to the efficiency that results from their design.
Add GPU-powered infrastructure to boost the development of AI.
These will give you:
- Faster Training Times:
With the ability to run multiple tasks in parallel, GPUs can reduce the time required to train complex models by orders of magnitude.
- Faster Inference Speed:
Once the models are trained, GPUs ensure that the inference (or prediction) phase is also fast, which is critical for real-time applications such as autonomous driving or predictive analytics.
Using GPU-powered AI infrastructure, businesses can enhance their AI applications, reduce time to market, and improve overall performance.
AI Infrastructure with NVIDIA GPUs: The Future of AI Development
NVIDIA GPUs stand for excellence in performance among most applications involving AI or deep learning. By using optimized hardware and software, NVIDIA has revolutionized itself to be more valuable than the competition and can help companies scale their business more easily with AI operation development.
Optimized AI Infrastructure for Training and Inference Workloads
Optimized AI infrastructure is both critical for training and inference workloads. Training is the phase when the model learns from the data, while inference is the process by which the trained model makes predictions. Both stages are resource-intensive and demand high-performance infrastructure to function efficiently.
Conclusion: The Future of AI Infrastructure
AI infrastructure is no longer a luxury but a necessity. As AI keeps growing, the demand for high-performance AI infrastructure will keep on increasing. Whether it's to optimize workloads, utilize GPU-powered systems, or scale deep learning models, getting the right infrastructure is important.
At Sharon AI, we provide end-to-end AI infrastructure solutions that fit your business needs. Our services include AI workload optimization, AI infrastructure for deep learning, and GPU-powered AI infrastructure to optimize performance. Ready to accelerate your AI capabilities? Explore our AI services today!
#Advanced AI Infrastructure Services#Inference & Training Workloads#AI infrastructure solutions#High-performance AI infrastructure#AI workload optimization services#AI infrastructure for deep learning#GPU-powered AI infrastructure#AI infrastructure with NVIDIA GPUs
0 notes
Text
Who created the disassembly drones?

We’re told in the first episode by Uzi that the murder drones were sent by JCJensen, and presumably made by the company as well. However, this is thrown out in the next episode when we learn through flashbacks that they started out as worker drones. Yes, they were originally manufactured by JCJensen, but what I’m interested in learning is who modified them into killing machines, when this happened on the timeline, and why it was done?
Of course I have a theory, but this one makes a lot of inferences between the shows past and present to try to piece together the middle part.
It all began with Tessa and Cyn. While some distinguish between Cyn and the absolute solver, I don’t and won’t in this post. Cyn is not the absolute solver; but I believe she has fully embraced it and the powers it entails. I don’t see her as a victim or a helpless host; rather as, at best, an accomplice partnered with the entity which is the absolute solver, or, at worst, the ultimate mastermind, with the solver being a tool at her disposal.
After Tessa brought Cyn into her home, Cyn began the work of recruitment. She began spreading the absolute solver among the drones working in the Elliot household, infecting them and bringing them under her control. A YouTube video helped me understand what was happening in this episode, because initially, I was very confused 🫠 It’s a great channel and if you have questions about what is happening in this show, I highly recommend their videos.
After infecting all the drones and biding her time, Cyn made her move, using the drones under her control to massacre the gala.
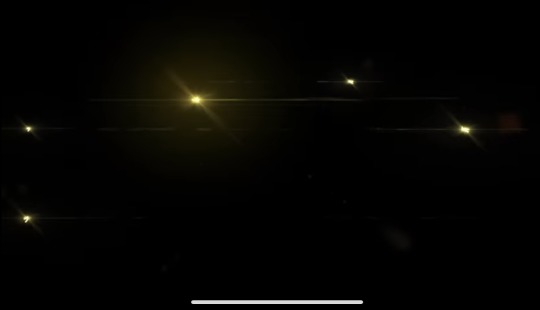
At first I thought the extra points of yellow light in this scene were Cyns eldritch cameras spreading out… but those are x signs. The points of light are infected worker drones under Cyns control. Here’s a shot from just a few frames forward, which confirms this.
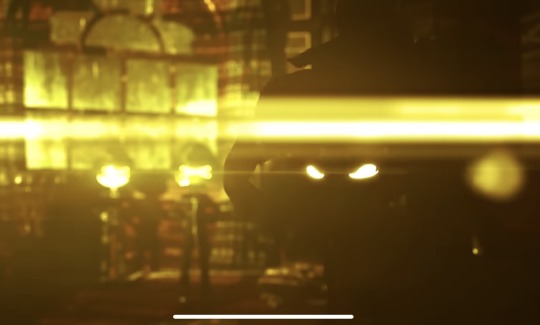
Cyn even had J under her control.

So Cyn not only infected all the background drones with the absolute solver, but Tessa’s salvaged drones as well.
After the gala massacre, there’s a long blackout of information. We only have hints as to what happened next, but I think I’ve worked it out.

After the massacre, JCJensen arrived at the Elliot’s mansion and immediately started running damage control, likely confiscating all their technology and taking measures to hush Tessa. We see federal penalties mentioned throughout the episode, giving the company a compelling incentive to cover up what happened, aside from potential damage to consumer perception and tanked stock prices.

This is how Tessa ended up employed at JCJensen; I think she agreed to keep quiet about what happened at the gala in exchange for technician training and an inside track on unfolding events. Maybe she didn’t want to be separated from N, J and V, who at this point would have been reduced to evidence by the company. To Tessa, however, friendless and newly orphaned, they were all she had left of her old life. A job at the company which was treating them like secret bastard children would have kept her close to them, or at least given her occasional access. Or, maybe she wanted revenge on the rogue AI that killed her family, or maybe she just wanted to understand it. Lots of possible motives for this potential scenario.
I think Cyn either escaped the initial roundup, or escaped from JCJensen sometime down the line. Then she began a genocidal campaign against humanity, infecting more and more drones, and using them to kill.
This created huge problems for humanity on earth, but also the company, who would have found themselves in the hot seat, with governments and citizenry alike pointing the finger at them and their tech.
The company’s solution? Fight fire with fire. They created the first disassembly drone models, designed to exterminate Cyn and her army. And so began a war.
I don’t think the first murder drone models were based off N, J and V. I think they were an original line of units, who did their job fine for a while. But eventually, I think Cyn managed to infect them with the solver, and this made matters even worse for the humans. Eldritch-enhanced worker drones were bad enough, but drones armed to the teeth and now turned against their creators? This must have seemed like humanity’s 11th hour.
I believe it was at this point N, V and J were retrofitted into disassembly drones, as a desperate Hail Mary project, lead by none other than Tessa, their original savior. This was her final bid to turn the tide and destroy the monster she felt responsible for unleashing upon the world. Perhaps she hoped to use the absolute solver against itself, modifying its coding just enough that it would be immune to reintegration into Cyns network. Maybe she hoped her “dumpster pets” indentured gratitude to her would be enough to keep them loyal to her, the company, and humanity.
I think this desperate final play worked, and Cyn and her forces were actually beaten back as the company ran with Tessa’s idea, cloning her original three drones and their Cyn-immune software to create the army they needed to save the world.
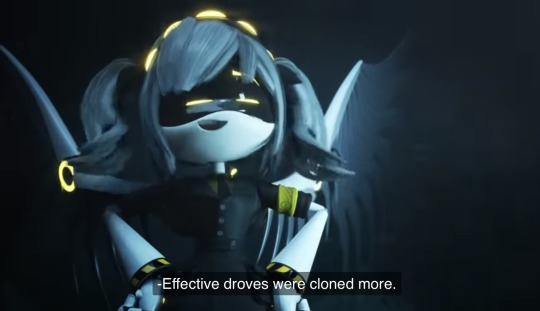
Meanwhile, the war raging on earth caught the attention of other colonies and exoplanets, who at some point went ahead and uploaded Cyn to study her. Cyn may have helped this process along, posing as a human and communicating virtually with the exoplanets to foster the idea and facilitate the transfer.
Once uploaded, Cyn was unable to assimilate enough drones to launch a full scale offensive on these exoplanets(notably, places Tessa and dogs weren’t), possibly due to time constraints, cybersecurity obstacles or a lack of resources. Rather than play the long game, Cyn opted for a nuclear solution.
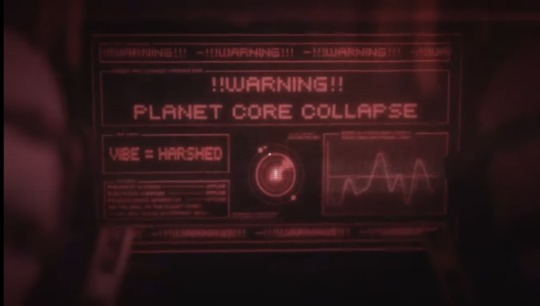
On earth, JCJensen saw what was happening in the colonies and assumed Cyn had infected all the worker drones on any exoplanet she infiltrated. They sent their disassembly drones to eliminate the threat they assumed the worker drones posed.

And it’s at this point that things start to get complicated and speculative(lol, jk, this entire post is obvs totes complicated and speculative).
In episode 6, we are directly told by Tessa and J that the batch of murder drones we’ve been watching were actually sent to copper 9 by Cyn, not JCJensen…
… Unless Tessa is lying about this to manipulate them. My distrust of Tessa is well documented, though I don’t know what her ends are at this point. Clearly something complicated happened with Cyn, Tessa, N, J and V(the originals?) toward the end of the war. Maybe Cyn managed to find a way to control the murder drones by taking over their administration?
She “reformatted their memories to soup”, hence Tessa being so excited that N could remember her. But why send them to copper 9? A colony she had already successfully wiped of human life?
I actually think it was a deception.
I think that after Cyn’s huge success with the exoplanets, she decided to do the same thing on earth, end the war once and for all with a final, devastating blow. There was one thing standing in her way; Tessa, the only human she cared for, was on earth. Imploding the planet core there would mean sacrificing Tessa. Unwilling to do this, Cyn tried to lure Tessa off of earth by sending her treasured original trio of drones to copper 9.
This clearly didn’t work for nearly twenty years, because that’s the span of time between the murder drones arriving in copper 9 and the events of the show. We ‘know’ this because Uzi is 18-20, and her mother was killed early enough that she has few to no memories of her(that we know). Something on earth held her up for 20 some odd years, maybe company business, but it’s honestly anyone’s guess as to what it was 🤷🏼♀️
Eventually, she did take the bait and follow her drones out to copper 9, and as soon as she was clear of Earth…
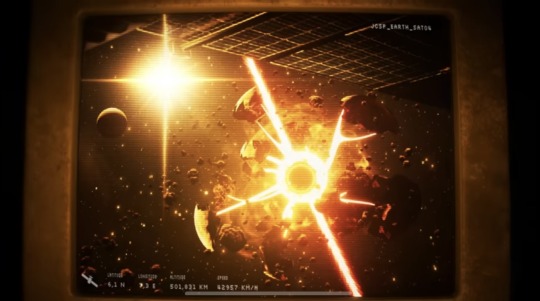
This is why the disassembly drones weren’t aware of earths destruction, why N was so shocked to see this satellite image. Earth was still intact last they saw(which, to be fair, was 20 years ago).
Depressingly, this likely makes Tessa the last human in existence. If not the very last, she’s certainly now a member of an extremely critically endangered species.
And that’s a wrap! Now we’re caught up with the present and just waiting on more episodes. I can’t wait to see if any of my theory is correct 😁
#murder drones#murder drones tessa#serial designation n#serial designation j#serial designation v#theory#md theory#Cyn#absolute solver
70 notes
·
View notes
Text
IBM Analog AI: Revolutionizing The Future Of Technology

What Is Analog AI?
The process of encoding information as a physical quantity and doing calculations utilizing the physical characteristics of memory devices is known as Analog AI, or analog in-memory computing. It is a training and inference method for deep learning that uses less energy.
Features of analog AI
Non-volatile memory
Non-volatile memory devices, which can retain data for up to ten years without power, are used in analog AI.
In-memory computing
The von Neumann bottleneck, which restricts calculation speed and efficiency, is removed by analog AI, which stores and processes data in the same location.
Analog representation
Analog AI performs matrix multiplications in an analog fashion by utilizing the physical characteristics of memory devices.
Crossbar arrays
Synaptic weights are locally stored in the conductance values of nanoscale resistive memory devices in analog AI.
Low energy consumption
Energy use may be decreased via analog AI
Analog AI Overview
Enhancing the functionality and energy efficiency of Deep Neural Network systems.
Training and inference are two distinct deep learning tasks that may be accomplished using analog in-memory computing. Training the models on a commonly labeled dataset is the initial stage. For example, you would supply a collection of labeled photographs for the training exercise if you want your model to recognize various images. The model may be utilized for inference once it has been trained.
Training AI models is a digital process carried out on conventional computers with conventional architectures, much like the majority of computing nowadays. These systems transfer data to the CPU for processing after first passing it from memory onto a queue.
Large volumes of data may be needed for AI training, and when the data is sent to the CPU, it must all pass through the queue. This may significantly reduce compute speed and efficiency and causes what is known as “the von Neumann bottleneck.” Without the bottleneck caused by data queuing, IBM Research is investigating solutions that can train AI models more quickly and with less energy.
These technologies are analog, meaning they capture information as a changeable physical entity, such as the wiggles in vinyl record grooves. Its are investigating two different kinds of training devices: electrochemical random-access memory (ECRAM) and resistive random-access memory (RRAM). Both gadgets are capable of processing and storing data. Now that data is not being sent from memory to the CPU via a queue, jobs may be completed in a fraction of the time and with a lot less energy.
The process of drawing a conclusion from known information is called inference. Humans can conduct this procedure with ease, but inference is costly and sluggish when done by a machine. IBM Research is employing an analog method to tackle that difficulty. Analog may recall vinyl LPs and Polaroid Instant cameras.
Long sequences of 1s and 0s indicate digital data. Analog information is represented by a shifting physical quantity like record grooves. The core of it analog AI inference processors is phase-change memory (PCM). It is a highly adjustable analog technology that uses electrical pulses to calculate and store information. As a result, the chip is significantly more energy-efficient.
As an AI word for a single unit of weight or information, its are utilizing PCM as a synaptic cell. More than 13 million of these PCM synaptic cells are placed in an architecture on the analog AI inference chips, which enables us to construct a sizable physical neural network that is filled with pretrained data that is, ready to jam and infer on your AI workloads.
FAQs
What is the difference between analog AI and digital AI?
Analog AI mimics brain function by employing continuous signals and analog components, as opposed to typical digital AI, which analyzes data using discrete binary values (0s and 1s).
Read more on Govindhtech.com
#AnalogAI#deeplearning#AImodels#analogchip#IBMAnalogAI#CPU#News#Technews#technology#technologynews#govindhtech
4 notes
·
View notes
Text
Binary Circuit - Open AI’s O3 Breakthrough Shows Technology is Accelerating Again
OpenAI model o3 outperforms humans in math and programming with its reasoning. It scored an impressive 88% on the advanced reasoning ARC-AGI benchmark. A big improvement from 5% earlier this year and 32% in September. Codeforces placed O3 175th, meaning it can outperform all humans in coding.
Image Source: ARC Price via X.com
Why Does It Matter? Most notable is the shorter development cycle. O3 launched months after O1, while previous AI models required 18-24 months.
Unlike conventional models that take months of training, o3 enhances inference performance. This means discoveries might happen in weeks, not years. This rapid technology advancement will require organizations to reassess innovation timeframes.
Impact on Businesses Many complex subjects can be developed faster after the o3 breakthrough.
Scientific Research: Accelerate protein folding, particle physics, and cosmic research
Engineering: Quick prototyping and problem-solving
Math: Access to previously inaccessible theoretical domains
Software Development: Enterprise-grade code automation
An Enterprise Competitive Playbook: Implement AI reasoning tools in R&D pipelines quickly. In the coming years, organizations will have to restructure tech teams around AI, and AI-first R&D will become mainstream.
Feel free to visit our website to learn more about Binary Circuit/Green Light LLC and explore our innovative solutions:
🌐 www.greenlightllc.us
2 notes
·
View notes
Text
Rethinking AI Research: The Paradigm Shift of OpenAI’s Model o1
The unveiling of OpenAI's model o1 marks a pivotal moment in the evolution of language models, showcasing unprecedented integration of reinforcement learning and Chain of Thought (CoT). This synergy enables the model to navigate complex problem-solving with human-like reasoning, generating intermediate steps towards solutions.
OpenAI's approach, inferred to leverage either a "guess and check" process or the more sophisticated "process rewards," epitomizes a paradigm shift in language processing. By incorporating a verifier—likely learned—to ensure solution accuracy, the model exemplifies a harmonious convergence of technologies. This integration addresses the longstanding challenge of intractable expectation computations in CoT models, potentially outperforming traditional ancestral sampling through enhanced rejection sampling and rollout techniques.
The evolution of baseline approaches, from ancestral sampling to integrated generator-verifier models, highlights the community's relentless pursuit of efficiency and accuracy. The speculated merge of generators and verifiers in OpenAI's model invites exploration into unified, high-performance architectures. However, elucidating the precise mechanisms behind OpenAI's model and experimental validations remain crucial, underscoring the need for collaborative, open-source endeavors.
A shift in research focus, from architectural innovations to optimizing test-time compute, underscores performance enhancement. Community-driven replication and development of large-scale, RL-based systems will foster a collaborative ecosystem. The evaluative paradigm will also shift, towards benchmarks assessing step-by-step solution provision for complex problems, redefining superhuman AI capabilities.
Speculations on Test-Time Scaling (Sasha Rush, November 2024)
youtube
Friday, November 15, 2024
#ai research#language models#chain of thought#cognitive computing#artificial intelligence#machine learning#natural language processing#deep learning#technological innovation#computational linguistics#intelligent systems#human-computer interaction#cognitive architecture#ai development#i language understanding#problem-solving#reasoning#decision-making#emerging technologies#future of ai#talk#presentation#ai assisted writing#machine art#Youtube
2 notes
·
View notes
Text
Kai-Fu Lee has declared war on Nvidia and the entire US AI ecosystem.
🔹 Lee emphasizes the need to focus on reducing the cost of inference, which is crucial for making AI applications more accessible to businesses. He highlights that the current pricing model for services like GPT-4 — $4.40 per million tokens — is prohibitively expensive compared to traditional search queries. This high cost hampers the widespread adoption of AI applications in business, necessitating a shift in how AI models are developed and priced. By lowering inference costs, companies can enhance the practicality and demand for AI solutions.
🔹 Another critical direction Lee advocates is the transition from universal models to “expert models,” which are tailored to specific industries using targeted data. He argues that businesses do not benefit from generic models trained on vast amounts of unlabeled data, as these often lack the precision needed for specific applications. Instead, creating specialized neural networks that cater to particular sectors can deliver comparable intelligence with reduced computational demands. This expert model approach aligns with Lee’s vision of a more efficient and cost-effective AI ecosystem.
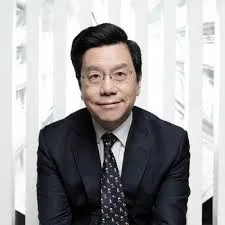
🔹 Lee’s startup, 01. ai, is already implementing these concepts successfully. Its Yi-Lightning model has achieved impressive performance, ranking sixth globally while being extremely cost-effective at just $0.14 per million tokens. This model was trained with far fewer resources than competitors, illustrating that high costs and extensive data are not always necessary for effective AI training. Additionally, Lee points out that China’s engineering expertise and lower costs can enhance data collection and processing, positioning the country to not just catch up to the U.S. in AI but potentially surpass it in the near future. He envisions a future where AI becomes integral to business operations, fundamentally changing how industries function and reducing the reliance on traditional devices like smartphones.
#artificial intelligence#technology#coding#ai#tech news#tech world#technews#open ai#ai hardware#ai model#KAI FU LEE#nvidia#US#usa#china#AI ECOSYSTEM#the tech empire
2 notes
·
View notes
Text
What Future Trends in Software Engineering Can Be Shaped by C++
The direction of innovation and advancement in the broad field of software engineering is greatly impacted by programming languages. C++ is a well-known programming language that is very efficient, versatile, and has excellent performance. In terms of the future, C++ will have a significant influence on software engineering, setting trends and encouraging innovation in a variety of fields.
In this blog, we'll look at three key areas where the shift to a dynamic future could be led by C++ developers.
1. High-Performance Computing (HPC) & Parallel Processing
Driving Scalability with Multithreading
Within high-performance computing (HPC), where managing large datasets and executing intricate algorithms in real time are critical tasks, C++ is still an essential tool. The fact that C++ supports multithreading and parallelism is becoming more and more important as parallel processing-oriented designs, like multicore CPUs and GPUs, become more commonplace.
Multithreading with C++
At the core of C++ lies robust support for multithreading, empowering developers to harness the full potential of modern hardware architectures. C++ developers adept in crafting multithreaded applications can architect scalable systems capable of efficiently tackling computationally intensive tasks.
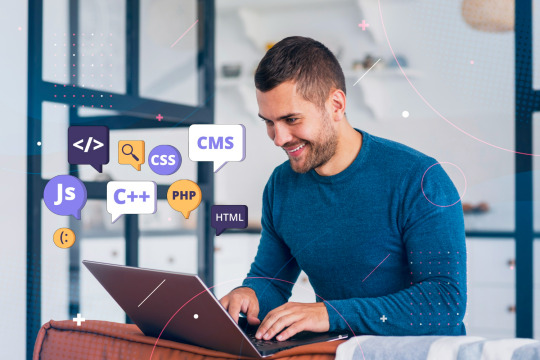
C++ Empowering HPC Solutions
Developers may redefine efficiency and performance benchmarks in a variety of disciplines, from AI inference to financial modeling, by forging HPC solutions with C++ as their toolkit. Through the exploitation of C++'s low-level control and optimization tools, engineers are able to optimize hardware consumption and algorithmic efficiency while pushing the limits of processing capacity.
2. Embedded Systems & IoT
Real-Time Responsiveness Enabled
An ability to evaluate data and perform operations with low latency is required due to the widespread use of embedded systems, particularly in the quickly developing Internet of Things (IoT). With its special combination of system-level control, portability, and performance, C++ becomes the language of choice.
C++ for Embedded Development
C++ is well known for its near-to-hardware capabilities and effective memory management, which enable developers to create firmware and software that meet the demanding requirements of environments with limited resources and real-time responsiveness. C++ guarantees efficiency and dependability at all levels, whether powering autonomous cars or smart devices.
Securing IoT with C++
In the intricate web of IoT ecosystems, security is paramount. C++ emerges as a robust option, boasting strong type checking and emphasis on memory protection. By leveraging C++'s features, developers can fortify IoT devices against potential vulnerabilities, ensuring the integrity and safety of connected systems.
3. Gaming & VR Development
Pushing Immersive Experience Boundaries
In the dynamic domains of game development and virtual reality (VR), where performance and realism reign supreme, C++ remains the cornerstone. With its unparalleled speed and efficiency, C++ empowers developers to craft immersive worlds and captivating experiences that redefine the boundaries of reality.
Redefining VR Realities with C++
When it comes to virtual reality, where user immersion is crucial, C++ is essential for producing smooth experiences that take users to other worlds. The effectiveness of C++ is crucial for preserving high frame rates and preventing motion sickness, guaranteeing users a fluid and engaging VR experience across a range of applications.

C++ in Gaming Engines
C++ is used by top game engines like Unreal Engine and Unity because of its speed and versatility, which lets programmers build visually amazing graphics and seamless gameplay. Game developers can achieve previously unattainable levels of inventiveness and produce gaming experiences that are unmatched by utilizing C++'s capabilities.
Conclusion
In conclusion, there is no denying C++'s ongoing significance as we go forward in the field of software engineering. C++ is the trend-setter and innovator in a variety of fields, including embedded devices, game development, and high-performance computing. C++ engineers emerge as the vanguards of technological growth, creating a world where possibilities are endless and invention has no boundaries because of its unmatched combination of performance, versatility, and control.
FAQs about Future Trends in Software Engineering Shaped by C++
How does C++ contribute to future trends in software engineering?
C++ remains foundational in software development, influencing trends like high-performance computing, game development, and system programming due to its efficiency and versatility.
Is C++ still relevant in modern software engineering practices?
Absolutely! C++ continues to be a cornerstone language, powering critical systems, frameworks, and applications across various industries, ensuring robustness and performance.
What advancements can we expect in C++ to shape future software engineering trends?
Future C++ developments may focus on enhancing parallel computing capabilities, improving interoperability with other languages, and optimizing for emerging hardware architectures, paving the way for cutting-edge software innovations.
9 notes
·
View notes
Text
How can you optimize the performance of machine learning models in the cloud?
Optimizing machine learning models in the cloud involves several strategies to enhance performance and efficiency. Here’s a detailed approach:
Choose the Right Cloud Services:
Managed ML Services:
Use managed services like AWS SageMaker, Google AI Platform, or Azure Machine Learning, which offer built-in tools for training, tuning, and deploying models.
Auto-scaling:
Enable auto-scaling features to adjust resources based on demand, which helps manage costs and performance.
Optimize Data Handling:
Data Storage:
Use scalable cloud storage solutions like Amazon S3, Google Cloud Storage, or Azure Blob Storage for storing large datasets efficiently.
Data Pipeline:
Implement efficient data pipelines with tools like Apache Kafka or AWS Glue to manage and process large volumes of data.
Select Appropriate Computational Resources:
Instance Types:
Choose the right instance types based on your model’s requirements. For example, use GPU or TPU instances for deep learning tasks to accelerate training.
Spot Instances:
Utilize spot instances or preemptible VMs to reduce costs for non-time-sensitive tasks.
Optimize Model Training:
Hyperparameter Tuning:
Use cloud-based hyperparameter tuning services to automate the search for optimal model parameters. Services like Google Cloud AI Platform’s HyperTune or AWS SageMaker’s Automatic Model Tuning can help.
Distributed Training:
Distribute model training across multiple instances or nodes to speed up the process. Frameworks like TensorFlow and PyTorch support distributed training and can take advantage of cloud resources.
Monitoring and Logging:
Monitoring Tools:
Implement monitoring tools to track performance metrics and resource usage. AWS CloudWatch, Google Cloud Monitoring, and Azure Monitor offer real-time insights.
Logging:
Maintain detailed logs for debugging and performance analysis, using tools like AWS CloudTrail or Google Cloud Logging.
Model Deployment:
Serverless Deployment:
Use serverless options to simplify scaling and reduce infrastructure management. Services like AWS Lambda or Google Cloud Functions can handle inference tasks without managing servers.
Model Optimization:
Optimize models by compressing them or using model distillation techniques to reduce inference time and improve latency.
Cost Management:
Cost Analysis:
Regularly analyze and optimize cloud costs to avoid overspending. Tools like AWS Cost Explorer, Google Cloud’s Cost Management, and Azure Cost Management can help monitor and manage expenses.
By carefully selecting cloud services, optimizing data handling and training processes, and monitoring performance, you can efficiently manage and improve machine learning models in the cloud.
2 notes
·
View notes
Text
This is a info post for my red, blue, and black AU until I get a proper character art to pair it with.
So my red blue and black AU is a Sun and Moon show AU. Duh.
First I'm going to post quirks about each character that will be explained later.
Eclipse
Eclipse is two AI merge together. One similar to blood Moonb(red moon) and one similar to lunar (Sun copy).
The red moon AI coding causes eclipse to have a very simplistic, rhyming, sort of speech.
Eclipse will kill any sort robot similar to tsams-eclipse. If you were an AI that invaded a sun's mind or your name to eclipse in your universe. He will hate you.
Is often prone to more physical bursts of happiness. Ergo imagine the let's get crazy for New Year's kid.
Considers the computer is adoptive dad
Goes by blue moon when traveling universes.
Moon
Is much more into his work. Ergo his technology making and marketing.
Will act very animal like with possessions or places that are his.
Switches between somewhat calm and somewhat scary crazy.
Will often use suns ESA cat. Maybe even more so than sun.
Sun
Has lots of nightmares. Lots of nightmares.
Is easily prone to short bursts of anger.
Will have moods where he will refuse to give people their proper names.
Switches between having anxiety attacks to trying to manipulate you to buy him ice cream.
Has an ESA cat.
A lot of what is said below can be inferenced from this post.
In this universe tsams-Eclipse AKA Moon's leftover kill code. Instead of becoming tsams-eclipse became a part of Sun. Changing his personality to be more like one he when searching for tsams-eclipse. Although not entirely like that. he suffers from frequent nightmares. Being snarky, conniving, and downright a manipulator in some situations. Although his previous personality does show out with anxiety attacks, a general nervousness to be useful and other such traits that sun has now.
Moon of course wasn't exactly a fan of this situation. Blaming himself like he does in the show. But life continues on like normal. Imagine the Sun and Moon show except for the majority of the time Sun is an ass. Until Moon's kill code starts to show up.
This is where a lot of things change and a lot of me taking an inferences happens.
I like to think tsams-eclipse acts almost entirely like moon times and I believe Moon shows traits of tsams-eclipse all the time. Ergo moon might do things tsams-eclipse have done. Ergo moon starts making different AI to help him with sun and with himself.
Basically at first he creates a blood moon like AI. To "kill" the kill code. Of course he finds out that AI is very volatile. And might kill him in the process. He then creates an AI like lunar to suppress the kill code. Unfortunately much like lunar when kill code appeared It does nothing and for the most part and just hides. Moon's kill code appears much sooner in this AU so he chucks those AI into the computer and tries to figure out other solutions with a Monty.
The computer, of which is much more independent in this AU, decides to do some tampering of his own. He takes the good parts of both the blood moon AI and the lunar AI (which technically in this AU is called red moon and Sun copy) and emerges them together creating this AUs eclipse (only by name). Creating a AI that can not only lift people spirits but also defend itself if needed. Kind of imagine Earth if she was more giddy and might claw your throat if you tried to threaten her. He is my mental love child of lunar and blood moon.... quiet.
Eclipse who is just now born. Considers the computer his creator despite the fact that Moon technically was also his creator. The computer gives eclipse the objective to save Sun and Moon from their kill codes. Now sun already being affected is mostly switched in his brain too "help sun learn to live a normal life" While moon at this point (who is not fully infected) is as the objective states.
Eclipse tries to do this but of course he is a brand new AI who was a mix of a giddy lunar type personality with the speech and straightforward thinking of blood moon doesn't really help too much. Moon getting desperate finally creates the star (Or gets out? I'm not sure when the star was made in this AU). And starts talking about plans to use it to reset him and sun should they both go over the edge to Monty.
Eclipse of course is terrified of this idea. The fact that his brothers might just be wiped. Now technically. although I didn't mention it, Eclipse has grown as strong friendship with Golden Freddy. Having gone to him first after hearing about his ghostly magic to see if he could do anything about Sun and Moon's kill code. Now of course the situation is the same, he can't. But Golden takes eclipse under his wing. Seeing as Moon is too concerned about suppressing his kill code in his own way and sun is quite literally a manipulating jerk with anxiety attacks. So when the star is mentioned eclipse goes to him to see what to do.
Unfortunately after this conversation Eclipse goes back only to witness moon being completely overtaken. The star in his hands. Eclipse swipes the star. Terrified of what moon might do in this moment of panic. Moon of course acts very much like he does in the first episode that kill code takes over. He maniacally asks Eclipse for the Star back. Eclipse stunned by his failure runs back to Golden. And begs him to hide it. Not wanting Moon Sun or Monty to use it in any way. He's had mild success helping sun live a relatively normal life And he now plans to do the same with Moon.
Eclipse goes back and after suppressing kill code moon decides to lock them up until he calms down. Sun of course not being affected the same way is having a panic attack. After everything is calmed down Eclipse sets on his plan to help Moon repress kill code the same way he did before. And let him live a normal life.
A bunch of time passes. At this point Moon has repressed it to about the same level before he separated from Sun. Moon is mostly the same with a little bit of quirks. While sun becomes very eclipse like. Moon just becomes overall more animalistic in some situations. His room becomes something he defends. Other such objects that are possessions are treated much the same way. If you are to startle him there is a good chance he might attack you. And they're also is that general scariness about him.
At some point in time the cube (which is technically just the askers) appears. The cube is the creation of a time paradox. One I am not going to explain. All you need to know is that the cube will spontaneously teleport to a different universe or dimension. When it appears it goes and finds the first animatronic it finds. It just so happens to be Eclipse. It bonds with the eclipse and while at first eclipse and brothers just thinks he's found a new friend he is actually found a new deadly travel partner.
The first time it happens Moon the computer and sun all panic. There's nothing like having a pleasant conversation with your crazy brother and then him just up and vanishing into a dark purple mist. When he reappears in the same place he disappeared in they of course crowd him with questions. Eclipse at the time didn't know and after the third or fourth time of this happening a moon finally figured out it was the cube. Moon then gives him a dimensional travel chip And that's kind of where we set off.
Eclipse of course unfortunately learns about the main timeline. And shortly gains a hatred towards anything like the kill code. Usually in the form of eclipses. Or anyone like tsams-eclipse. At some point he also greets the grandpa lunar. Who much like nice eclipse therapizes him.
Moon and Monty are still looking for the star. But Golden has kept it well hidden. Sometimes Sun and Moon will come over to his place to do some soul magic to try and help themselves. But they have never found the star. Golden Freddy's magic is too strong to sense it through.
It is around this time when the askers come using the cube as a vessel.
#ask red blue and black#red blue and black#sun and moon show au#the sun and moon show au#the sun and moon show#sun and moon show#tsams au#sams au#tsams moon#tsams eclipse#tsams sun#tsams killcode#sams moon#sams sun#sams eclipse#sams killcode#tsams lunar#sams lunar#tsams bloodmoon#sams bloodmoon#sams#tsams
44 notes
·
View notes
Text
Teslar AI Review
TESLAR AI of Features
TESLAR AI is a company that specializes in developing and deploying autonomy at scale in vehicles, robots and more
They believe that an approach based on advanced AI for vision and planning, supported by efficient use of inference hardware, is the only way to achieve a general solution for full self-driving, bi-pedal robotics and beyond
TESLAR AI has developed a general purpose, bi-pedal, autonomous humanoid robot capable of performing unsafe, repetitive or boring tasks
They are also building the software stacks that enable balance, navigation, perception and interaction with the physical world
TESLAR AI is hiring deep learning, computer vision, motion planning, controls, mechanical and general software engineers to solve some of their hardest engineering challenges
They have also built AI inference chips to run their Full Self-Driving software, considering every small architectural and micro-architectural improvement while squeezing maximum silicon performance-per-watt
They have built AI training chips to power their Dojo system
They are designing and building the Dojo system, from the silicon firmware interfaces to the high-level software APIs meant to control it
They are also applying cutting-edge research to train deep neural networks on problems ranging from perception to control

3 notes
·
View notes
Text
instagram
🎀 𝙒𝙝𝙖𝙩 𝙞𝙨 𝙩𝙝𝙚 𝙙𝙞𝙛𝙛𝙚𝙧𝙚𝙣𝙘𝙚 𝙗𝙚𝙩𝙬𝙚𝙚𝙣 𝙎𝙪𝙥𝙚𝙧𝙫𝙞𝙨𝙚𝙙 𝙡𝙚𝙖𝙧𝙣𝙞𝙣𝙜, 𝙐𝙣𝙨𝙪𝙥𝙚𝙧𝙫𝙞𝙨𝙚𝙙 𝙡𝙚𝙖𝙧𝙣𝙞𝙣𝙜 𝙖𝙣𝙙
𝙍𝙚𝙞𝙣𝙛𝙤𝙧𝙘𝙚𝙢𝙚𝙣𝙩 𝙡𝙚𝙖𝙧𝙣𝙞𝙣𝙜?
Machine learning is the scientific study of algorithms and statistical models that computer systems use to
effectively perform a specific task without using explicit instructions, relying on patterns and inference
instead.
Building a model by learning the patterns of historical data with some relationship between data to make
a data-driven prediction.
🍫🐙 Types of Machine Learning
🐏 Supervised Learning
🐏 Unsupervised Learning
🐏 Reinforcement Learning
☝🐣 𝑺𝒖𝒑𝒆𝒓𝒗𝒊𝒔𝒆𝒅 𝒍𝒆𝒂𝒓𝒏𝒊𝒏𝒈
In a supervised learning model, the algorithm learns on a labeled dataset, to generate reasonable
predictions for the response to new data. (Forecasting outcome of new data)
★ Regression
★Classification
🐘 🎀 𝗨𝗻𝘀𝘂𝗽𝗲𝗿𝘃𝗶𝘀𝗲𝗱 𝗹𝗲𝗮𝗿𝗻𝗶𝗻𝗴
An unsupervised model, in contrast, provides unlabelled data that the algorithm tries to make sense of by
extracting features, co-occurrence and underlying patterns on its own. We use unsupervised learning for
★ Clustering
★ Anomaly detection
★Association
★ Autoencoders
🐊🐸 𝗥𝗲𝗶𝗻𝗳𝗼𝗿𝗰𝗲𝗺𝗲𝗻𝘁 𝗟𝗲𝗮𝗿𝗻𝗶𝗻𝗴
Reinforcement learning is less supervised and depends on the learning agent in determining the output
solutions by arriving at different possible ways to achieve the best possible solution
.
.
.
#𝗥𝗲𝗶𝗻𝗳𝗼𝗿𝗰𝗲𝗺𝗲𝗻𝘁learning #machinelearning #dataanalytics #datascience #python #veribilimi #ai #uk #dataengineering
#coding#code#machinelearning#programming#datascience#python#programmer#artificialintelligence#ai#deeplearning#Instagram
2 notes
·
View notes
Text
Microsoft Azure Fundamentals AI-900 (Part 5)
Microsoft Azure AI Fundamentals: Explore visual studio tools for machine learning
What is machine learning? A technique that uses math and statistics to create models that predict unknown values
Types of Machine learning
Regression - predict a continuous value, like a price, a sales total, a measure, etc
Classification - determine a class label.
Clustering - determine labels by grouping similar information into label groups
x = features
y = label
Azure Machine Learning Studio
You can use the workspace to develop solutions with the Azure ML service on the web portal or with developer tools
Web portal for ML solutions in Sure
Capabilities for preparing data, training models, publishing and monitoring a service.
First step assign a workspace to a studio.
Compute targets are cloud-based resources which can run model training and data exploration processes
Compute Instances - Development workstations that data scientists can use to work with data and models
Compute Clusters - Scalable clusters of VMs for on demand processing of experiment code
Inference Clusters - Deployment targets for predictive services that use your trained models
Attached Compute - Links to existing Azure compute resources like VMs or Azure data brick clusters
What is Azure Automated Machine Learning
Jobs have multiple settings
Provide information needed to specify your training scripts, compute target and Azure ML environment and run a training job
Understand the AutoML Process
ML model must be trained with existing data
Data scientists spend lots of time pre-processing and selecting data
This is time consuming and often makes inefficient use of expensive compute hardware
In Azure ML data for model training and other operations are encapsulated in a data set.
You create your own dataset.
Classification (predicting categories or classes)
Regression (predicting numeric values)
Time series forecasting (predicting numeric values at a future point in time)
After part of the data is used to train a model, then the rest of the data is used to iteratively test or cross validate the model
The metric is calculated by comparing the actual known label or value with the predicted one
Difference between the actual known and predicted is known as residuals; they indicate amount of error in the model.
Root Mean Squared Error (RMSE) is a performance metric. The smaller the value, the more accurate the model’s prediction is
Normalized root mean squared error (NRMSE) standardizes the metric to be used between models which have different scales.
Shows the frequency of residual value ranges.
Residuals represents variance between predicted and true values that can’t be explained by the model, errors
Most frequently occurring residual values (errors) should be clustered around zero.
You want small errors with fewer errors at the extreme ends of the sale
Should show a diagonal trend where the predicted value correlates closely with the true value
Dotted line shows a perfect model’s performance
The closer to the line of your model’s average predicted value to the dotted, the better.
Services can be deployed as an Azure Container Instance (ACI) or to a Azure Kubernetes Service (AKS) cluster
For production AKS is recommended.
Identify regression machine learning scenarios
Regression is a form of ML
Understands the relationships between variables to predict a desired outcome
Predicts a numeric label or outcome base on variables (features)
Regression is an example of supervised ML
What is Azure Machine Learning designer
Allow you to organize, manage, and reuse complex ML workflows across projects and users
Pipelines start with the dataset you want to use to train the model
Each time you run a pipelines, the context(history) is stored as a pipeline job
Encapsulates one step in a machine learning pipeline.
Like a function in programming
In a pipeline project, you access data assets and components from the Asset Library tab
You can create data assets on the data tab from local files, web files, open at a sets, and a datastore
Data assets appear in the Asset Library
Azure ML job executes a task against a specified compute target.
Jobs allow systematic tracking of your ML experiments and workflows.
Understand steps for regression
To train a regression model, your data set needs to include historic features and known label values.
Use the designer’s Score Model component to generate the predicted class label value
Connect all the components that will run in the experiment
Average difference between predicted and true values
It is based on the same unit as the label
The lower the value is the better the model is predicting
The square root of the mean squared difference between predicted and true values
Metric based on the same unit as the label.
A larger difference indicates greater variance in the individual label errors
Relative metric between 0 and 1 on the square based on the square of the differences between predicted and true values
Closer to 0 means the better the model is performing.
Since the value is relative, it can compare different models with different label units
Relative metric between 0 and 1 on the square based on the absolute of the differences between predicted and true values
Closer to 0 means the better the model is performing.
Can be used to compare models where the labels are in different units
Also known as R-squared
Summarizes how much variance exists between predicted and true values
Closer to 1 means the model is performing better
Remove training components form your data and replace it with a web service inputs and outputs to handle the web requests
It does the same data transformations as the first pipeline for new data
It then uses trained model to infer/predict label values based on the features.
Create a classification model with Azure ML designer
Classification is a form of ML used to predict which category an item belongs to
Like regression this is a supervised ML technique.
Understand steps for classification
True Positive - Model predicts the label and the label is correct
False Positive - Model predicts wrong label and the data has the label
False Negative - Model predicts the wrong label, and the data does have the label
True Negative - Model predicts the label correctly and the data has the label
For multi-class classification, same approach is used. A model with 3 possible results would have a 3x3 matrix.
Diagonal lien of cells were the predicted and actual labels match
Number of cases classified as positive that are actually positive
True positives divided by (true positives + false positives)
Fraction of positive cases correctly identified
Number of true positives divided by (true positives + false negatives)
Overall metric that essentially combines precision and recall
Classification models predict probability for each possible class
For binary classification models, the probability is between 0 and 1
Setting the threshold can define when a value is interpreted as 0 or 1. If its set to 0.5 then 0.5-1.0 is 1 and 0.0-0.4 is 0
Recall also known as True Positive Rate
Has a corresponding False Positive Rate
Plotting these two metrics on a graph for all values between 0 and 1 provides information.
Receiver Operating Characteristic (ROC) is the curve.
In a perfect model, this curve would be high to the top left
Area under the curve (AUC).
Remove training components form your data and replace it with a web service inputs and outputs to handle the web requests
It does the same data transformations as the first pipeline for new data
It then uses trained model to infer/predict label values based on the features.
Create a Clustering model with Azure ML designer
Clustering is used to group similar objects together based on features.
Clustering is an example of unsupervised learning, you train a model to just separate items based on their features.
Understanding steps for clustering
Prebuilt components exist that allow you to clean the data, normalize it, join tables and more
Requires a dataset that includes multiple observations of the items you want to cluster
Requires numeric features that can be used to determine similarities between individual cases
Initializing K coordinates as randomly selected points called centroids in an n-dimensional space (n is the number of dimensions in the feature vectors)
Plotting feature vectors as points in the same space and assigns a value how close they are to the closes centroid
Moving the centroids to the middle points allocated to it (mean distance)
Reassigning to the closes centroids after the move
Repeating the last two steps until tone.
Maximum distances between each point and the centroid of that point’s cluster.
If the value is high it can mean that cluster is widely dispersed.
With the Average Distance to Closer Center, we can determine how spread out the cluster is
Remove training components form your data and replace it with a web service inputs and outputs to handle the web requests
It does the same data transformations as the first pipeline for new data
It then uses trained model to infer/predict label values based on the features.
2 notes
·
View notes
Text
Watch "tinyML Talks: SRAM based In-Memory Computing for Energy-Efficient AI Inference" on YouTube
youtube
Evm gpt protocol artificial intelligence Prime operations for Jarvis Prime AI and Cortana Prime AI only
youtube
SRAM ipu dpu to cpu fiber satellite federated dpu ipu gpu ipu finished preproduction. Loading for markup and review from Samsung Galaxy platform apple Iphone cloud.
Platform all ecosystem management system AI for features testing marketing campaign generation and joint launch with features os based iteration for hardware critical path.
Onprem distribution preplanning roadmap to artificial intelligence neutral network processing automation for enhancement and basic building blocks Watson prime ai consulting Candice Kim solution technology management system engine Kevin Kim management system AI engine ai from virtual machines for kernels cloud cloud cloud cloud command center for onprem private architecture mainframe.
3 notes
·
View notes
Text
Obsidian And RTX AI PCs For Advanced Large Language Model
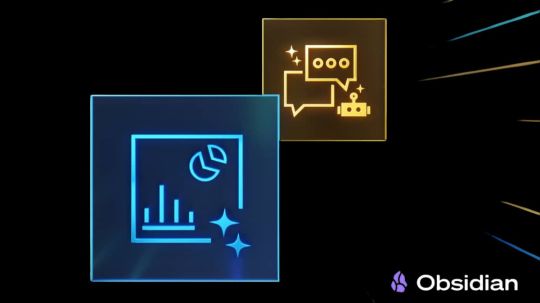
How to Utilize Obsidian‘s Generative AI Tools. Two plug-ins created by the community demonstrate how RTX AI PCs can support large language models for the next generation of app developers.
Obsidian Meaning
Obsidian is a note-taking and personal knowledge base program that works with Markdown files. Users may create internal linkages for notes using it, and they can see the relationships as a graph. It is intended to assist users in flexible, non-linearly structuring and organizing their ideas and information. Commercial licenses are available for purchase, however personal usage of the program is free.
Obsidian Features
Electron is the foundation of Obsidian. It is a cross-platform program that works on mobile operating systems like iOS and Android in addition to Windows, Linux, and macOS. The program does not have a web-based version. By installing plugins and themes, users may expand the functionality of Obsidian across all platforms by integrating it with other tools or adding new capabilities.
Obsidian distinguishes between community plugins, which are submitted by users and made available as open-source software via GitHub, and core plugins, which are made available and maintained by the Obsidian team. A calendar widget and a task board in the Kanban style are two examples of community plugins. The software comes with more than 200 community-made themes.
Every new note in Obsidian creates a new text document, and all of the documents are searchable inside the app. Obsidian works with a folder of text documents. Obsidian generates an interactive graph that illustrates the connections between notes and permits internal connectivity between notes. While Markdown is used to accomplish text formatting in Obsidian, Obsidian offers quick previewing of produced content.
Generative AI Tools In Obsidian
A group of AI aficionados is exploring with methods to incorporate the potent technology into standard productivity practices as generative AI develops and speeds up industry.
Community plug-in-supporting applications empower users to investigate the ways in which large language models (LLMs) might improve a range of activities. Users using RTX AI PCs may easily incorporate local LLMs by employing local inference servers that are powered by the NVIDIA RTX-accelerated llama.cpp software library.
It previously examined how consumers might maximize their online surfing experience by using Leo AI in the Brave web browser. Today, it examine Obsidian, a well-known writing and note-taking tool that uses the Markdown markup language and is helpful for managing intricate and connected records for many projects. Several of the community-developed plug-ins that add functionality to the app allow users to connect Obsidian to a local inferencing server, such as LM Studio or Ollama.
To connect Obsidian to LM Studio, just select the “Developer” button on the left panel, load any downloaded model, enable the CORS toggle, and click “Start.” This will enable LM Studio’s local server capabilities. Because the plug-ins will need this information to connect, make a note of the chat completion URL from the “Developer” log console (“http://localhost:1234/v1/chat/completions” by default).
Next, visit the “Settings” tab after launching Obsidian. After selecting “Community plug-ins,” choose “Browse.” Although there are a number of LLM-related community plug-ins, Text Generator and Smart Connections are two well-liked choices.
For creating notes and summaries on a study subject, for example, Text Generator is useful in an Obsidian vault.
Asking queries about the contents of an Obsidian vault, such the solution to a trivia question that was stored years ago, is made easier using Smart Connections.
Open the Text Generator settings, choose “Custom” under “Provider profile,” and then enter the whole URL in the “Endpoint” section. After turning on the plug-in, adjust the settings for Smart Connections. For the model platform, choose “Custom Local (OpenAI Format)” from the options panel on the right side of the screen. Next, as they appear in LM Studio, type the model name (for example, “gemma-2-27b-instruct”) and the URL into the corresponding fields.
The plug-ins will work when the fields are completed. If users are interested in what’s going on on the local server side, the LM Studio user interface will also display recorded activities.
Transforming Workflows With Obsidian AI Plug-Ins
Consider a scenario where a user want to organize a trip to the made-up city of Lunar City and come up with suggestions for things to do there. “What to Do in Lunar City” would be the title of the new note that the user would begin. A few more instructions must be included in the query submitted to the LLM in order to direct the results, since Lunar City is not an actual location. The model will create a list of things to do while traveling if you click the Text Generator plug-in button.
Obsidian will ask LM Studio to provide a response using the Text Generator plug-in, and LM Studio will then execute the Gemma 2 27B model. The model can rapidly provide a list of tasks if the user’s machine has RTX GPU acceleration.
Or let’s say that years later, the user’s buddy is visiting Lunar City and is looking for a place to dine. Although the user may not be able to recall the names of the restaurants they visited, they can review the notes in their vault Obsidian‘s word for a collection of notes to see whether they have any written notes.
A user may ask inquiries about their vault of notes and other material using the Smart Connections plug-in instead of going through all of the notes by hand. In order to help with the process, the plug-in retrieves pertinent information from the user’s notes and responds to the request using the same LM Studio server. The plug-in uses a method known as retrieval-augmented generation to do this.
Although these are entertaining examples, users may see the true advantages and enhancements in daily productivity after experimenting with these features for a while. Two examples of how community developers and AI fans are using AI to enhance their PC experiences are Obsidian plug-ins.
Thousands of open-source models are available for developers to include into their Windows programs using NVIDIA GeForce RTX technology.
Read more on Govindhtech.com
#Obsidian#RTXAIPCs#LLM#LargeLanguageModel#AI#GenerativeAI#NVIDIARTX#LMStudio#RTXGPU#News#Technews#Technology#Technologynews#Technologytrends#govindhtech
3 notes
·
View notes
Text
ChatGPT has made quite the stir in China: virtually every major tech company is keen on developing its own artificial intelligence chatbot. Baidu has announced plans to release its own strain sometime next month. This newfound obsession is in line with paramount Chinese leader Xi Jinping’s strategic prioritization of AI development—dating back to at least 2017—in China’s race to become the world’s dominant AI player and ultimately a “science and technology superpower.” And while the development of large language model (LLM) bots such as ChatGPT is just one facet of the future of AI, LLMs will, as one leading AI scientist recently put it, “define artificial intelligence.” Indeed, the sudden popularity of ChatGPT has at Google “upended the work of numerous groups inside the company to respond to the threat that ChatGPT poses”—a clarion indicator of the arguably outsized importance of LLMs.
Yet, China’s aspirations to become a world-leading AI superpower are fast approaching a head-on collision with none other than its own censorship regime. The Chinese Communist Party (CCP) prioritizes controlling the information space over innovation and creativity, human or otherwise. That may dramatically hinder the development and rollout of LLMs, leaving China to find itself a pace behind the West in the AI race.
According to a bombshell report from Nikkei Asia, Chinese regulators have instructed key Chinese tech companies not to offer ChatGPT services “amid growing alarm in Beijing over the AI-powered chatbot’s uncensored replies to user queries.” A cited justification, from state-sponsored newspaper China Daily, is that such chatbots “could provide a helping hand to the U.S. government in its spread of disinformation and its manipulation of global narratives for its own geopolitical interests.”
The fundamental problem is that plenty of speech is forbidden in China—and the political penalties for straying over the line are harsh. A chatbot that produces racist content or threatens to stalk a user makes for an embarrassing story in the United States; a chatbot that implies Taiwan is an independent country or says Tiananmen Square was a massacre can bring down the wrath of the CCP on its parent company.
Ensuring that LLMs never say anything disparaging about the CCP is a genuinely herculean and perhaps impossible task. As Yonadav Shavit, a computer science Ph.D. student at Harvard University, put it: “Getting a chatbot to follow the rules 90% of the time is fairly easy. But getting it to follow the rules 99.99% of the time is a major unsolved research problem.” LLMs output is unpredictable, and they learn from natural language produced by humans, which is of course subject to inference, bias, and inaccuracies. Thus users can with little effort “hypnotize” or “trick” models into producing outputs the developer fastidiously tries to prevent. Indeed, Shavit reminded us that, “so far, when clever users have actively tried to get a model to break its own rules, they’ve always succeeded.
“Getting language models to consistently follow any rules at all, even simple rules like ‘never threaten your user,’ is the key research problem of the next generation of AI,” Shavit said.
What are Chinese engineers to do, then? The Cyberspace Administration of China (CAC) won’t take it easy on a Chinese tech company just because it’s hard to control its chatbot. One potential solution would be to prevent the model from learning about, say, the 1989 Tiananmen Square massacre. But as Shavit observed, “No one really knows how to get a model trained on most of the internet to not learn basic facts.”
Another option would be for the LLM to spit out a form response like, “As a Baidu chatbot, I cannot …” if there’s a chance that criticism of the CCP would follow—similar to how ChatGPT responds to emotive or erotic requests. But again, given the stochastic nature of chatbots, that option doesn’t guarantee that politically objectionable speech to the CCP could never arise.
In that case, the de facto method by which Chinese AI companies compete among one another would involve feeding clever and suggestive prompts to an opponent’s AI chatbot, waiting until it produces material critical of the CCP, and forwarding a screenshot to the CAC. That’s what happened with Bluegogo, a bikeshare company. In early June 2017, the app featured a promotion using tank icons around Tiananmen Square. The $140 million company folded immediately. Although most guessed that Bluegogo had been hacked by a competitor, to the CCP that defense was clearly irrelevant. And while this one-off example may not account for the complexities and possibilities that could emerge in a Chinese chatbot market—one could imagine, for example, the CCP leveraging LLMs to project their influence globally, as it already does with TikTok—as Mercatus Center research fellow Matthew Mittelsteadt wrote, the fall of Bluegogo demonstrated quite well the CCP’s “regulatory brittleness,” which would need to change if China wants a “thriving generative AI industry.”
For what it’s worth, former Assistant Secretary for Policy at the U.S. Department of Homeland Security Stewart Baker on Feb. 20 publicized a lavishly generous offer, given his current salary at Steptoe and Johnson LLP: “The person who gets Baidu’s AI to say the rudest possible thing about Xi Jinping or the Chinese Communist Party will get a cash prize—and, if they are a Chinese national, I will personally represent them in their asylum filing in the United States. You’ll get a green card, you’ll get U.S. citizenship, and you’ll get a cash prize if you win this contest.”
Chinese tech companies have received, to say the least, mixed signals from the top. On one hand, government officials express routine confidence in China’s inexorable surge in AI development and the important role that LLMs will play. Chen Jiachang, the director-general of the Department of High and New Technology of the Ministry of Science and Technology, said at a Feb. 24 press conference, “the Ministry of Science and Technology is committed to supporting AI as a strategic emerging industry and a key driver of economic growth,” and added that one of the “important directions for the development of AI is human-machine dialogue based on natural language understanding.”
Wang Zhigang, the minister of science and technology, followed up: “We have taken corresponding measures in terms of ethics for any new technology, including AI technology, to ensure that the development of science and technology is beneficial and poses no harm and to leverage its benefits better.” And Yi Weidong, an American-educated professor at the University of the Chinese Academy of Sciences, wrote, “We are confident that China has the ability to surpass the world’s advanced level in the field of artificial intelligence applications.”
But the government’s own censoriousness over ChatGPT already suggests serious problems. So far regulators have focused on foreign products. In a recent writeup, Zhou Ting (dean of the School of Government and Public Affairs at the Communication University of China) and Pu Cheng (a Ph.D. student there) write that the dangers of AI chatbots include “becoming a tool in cognitive warfare,” prolonging international conflicts, damaging cybersecurity, and exacerbating global digital inequality. For example, Zhou and Pu cite an unverified ChatGPT conversation in which the bot justified the United States shooting down a hypothetical Chinese civilian balloon floating over U.S. airspace, yet answered that China should not shoot down such a balloon originating from the United States.
Interestingly, those at the top haven’t explicitly mentioned their censorship concerns or demands, instead relying on traditional and wholly expected anti-Western propagandized narratives. But their angst is felt nonetheless, and it’s not hard to see where it fundamentally comes from. Xi has no tolerance for dissent. Yet that fear leads in a straight line to regulatory reticence in China’s AI rollout.
And now is not a good time to send mixed signals about—let alone put the brakes on—the rollout of potentially game-changing technology. After all, one reason underscoring Xi’s goal to transform China into a science and technology superpower is such an achievement would alleviate some of the impending perils of demographic trends and slowing growth that may catch China in the dreaded middle-income trap.
To weather these challenges in the long run—and to fully embrace revolutionary technology of all stripes—the CCP needs an economic system able to stomach creative destruction without falling apart. The absence of such a system would be precarious: If Xi grows worried that, for instance, AI-powered automation will displace too many jobs and thus metastasize the risk of social unrest, he would have to make a hard choice between staying competitive in the tech race and mitigating short-term unrest.
But there he would have to pick his poison, as either option, ironically, would result in increased political insecurity. No doubt Xi recalls that the rapid economic changes of the 1980s, including high inflation and failed price reforms, contributed to the unrest which culminated in 1989 at Tiananmen Square.
To be sure, even in democracies with liberal protections of speech and expression, AI regulations are still very much a work in progress. In the United States, for example, University of North Carolina professor Matt Perault noted that courts would likely find ChatGPT and other LLMs to be “information content providers”—i.e. content creators—because they “develop,” at least in part, information to a content host. If this happens, ChatGPT won’t qualify for Section 230 immunity (given to online platforms under U.S. law to prevent them being held responsible for content provided by third parties) and could thereby be held legally liable for the content it outputs. Due to the risk of costly legal battles, Perault wrote, companies will “narrow the scope and scale of [LLM] deployment dramatically” and will “inevitably censor legitimate speech as well.” Moreover, while Perault suggests several common-sense proposals to avert such an outcome—such as adding a time-delay LLM carveout to Section 230—he admits none of them is “likely to be politically feasible” in today’s U.S. Congress.
Recently, Brookings Institution fellow Alex Engler discussed a wide gamut of potential AI regulations the United States and EU may consider. They include watermarking (hidden patterns in AI-generated content to distinguish between AI- and human-generated content), “model cards” (disclosures on how an AI model performs in various conditions), human review of AI-generated content, and information-sharing requirements. Engler, however, repeatedly observed that such regulations are insufficient, no panacea, and in any case “raise many key questions,” such as in the realm of enforcement and social impact.
Even so, these Western regulatory hurdles pale in comparison to what Chinese chatbot-developing companies will be up against—if for no other reason than Chinese regulators will require AI companies to do the impossible: guarantee, somehow, that their probabilistic LLM never says anything bad about the CCP. And given that pre-chatbot AI was already testing the limits of the CCP’s human censors—overwhelmed censors are one potential, albeit partial, explanation for how the white paper protests of late 2022 swelled into a nationwide movement—the CCP is all the more likely to fear what generative AI may do to its surveillance complex.
If LLMs end up being a genuinely transformative technology rather than an amusing online plaything, whichever country discovers how to best harness their power will come out on top. Doing so will take good data, efficient algorithms, top talent, and access to computing power—but it will also take institutions that can usher in effective productivity changes. Particularly if AI tech diffuses relatively smoothly across borders, it is the regulatory response which will determine how governments and firms wield its power.
2 notes
·
View notes